Introduction
The opioid epidemic has been a growing national public health crisis over the past two decades. In 2020, it was estimated that approximately 2.7 million US individuals had an opioid use disorder (OUD) (Substance Abuse and Mental Health Services Administration, 2022). In 2021, 107622 individuals died of drug overdose, with opioids accounting for more than 75 percent (84 741) of all overdose deaths (National Center for Health Statistics, 2022).
Studies on the epidemiology of OUD have focused on the role of psychosocial and environmental risk factors for the development and maintenance of OUD (Strain, Reference Strain2022). For example, childhood maltreatment (e.g. sexual, physical, or emotional abuse/neglect) has been identified as a risk factor for OUD (Santo et al., Reference Santo, Campbell, Gisev, Tran, Colledge, Di Tanna and Degenhardt2021; Somer, Reference Somer2019), as have social or family environments that allow for substance misuse (Webster, Reference Webster2017). Psychiatric disorders such as major depressive disorder (MDD) (Na, Scodes, Fishman, Rotrosen, & Nunes, Reference Na, Scodes, Fishman, Rotrosen and Nunes2022b) and posttraumatic stress disorder (PTSD) (Somer, Reference Somer2019), which often co-occur among individuals with OUD, are additional risk factors for OUD (Strain, Reference Strain2022). Furthermore, socioeconomic factors such as low educational attainment (e.g. not graduating from high school), and low income have been identified as key risk factors for both OUD and opioid overdose deaths (Altekruse, Cosgrove, Altekruse, Jenkins, & Blanco, Reference Altekruse, Cosgrove, Altekruse, Jenkins and Blanco2020).
In addition to psychosocial and environmental factors, genetic factors are a significant contributor to OUD risk. The impact of family history of OUD and other substance use on OUD risk is well documented (Grant et al., Reference Grant, Saha, Ruan, Goldstein, Chou, Jung and Hasin2016). Genome-wide association studies (GWAS) have identified genetic variants linked to OUD risk (Deak & Johnson, Reference Deak and Johnson2021; Gelernter & Polimanti, Reference Gelernter and Polimanti2021; Zhou et al., Reference Zhou, Rentsch, Cheng, Kember, Nunez, Sherva and Gelernter2020a), including the mu-opioid receptor gene locus (OPRM1). Since 2022, large-scale GWAS efforts have greatly increased the discovery of OUD-related risk loci including 14 loci identified in a study of OUD (Kember et al., Reference Kember, Vickers-Smith, Xu, Toikumo, Niarchou, Zhou and Kranzler2022) and 19 loci in a study of OUD that leveraged information from other substance use disorders (SUDs) using the multi-trait analysis of GWAS (MTAG) approach (Deak et al., Reference Deak, Zhou, Galimberti, Levey, Wendt, Sanchez-Roige and Gelernter2022). Polygenic risk scores (PRS) derived from large GWAS are increasingly being used to quantify the association between genetic variation and OUD risk. For example, the MTAG analysis (Deak et al., Reference Deak, Zhou, Galimberti, Levey, Wendt, Sanchez-Roige and Gelernter2022) yielded a PRS that accounted for 3.8% of the variance in OUD diagnosis (Deak et al., Reference Deak, Zhou, Galimberti, Levey, Wendt, Sanchez-Roige and Gelernter2022).
Understanding the interplay between genetic liability and psychosocial and environmental factors in psychiatry (Mullins et al., Reference Mullins, Power, Fisher, Hanscombe, Euesden, Iniesta and Lewis2016) could account for more variance than either set of factors individually. This could enhance the identification of individuals at risk for various disorders. Emerging research has investigated PRS-by-environment correlates in psychiatric phenotypes including MDD (Mullins et al., Reference Mullins, Power, Fisher, Hanscombe, Euesden, Iniesta and Lewis2016), bipolar disorder (Polimanti et al., Reference Polimanti, Kaufman, Zhao, Kranzler, Ursano, Kessler and Gelernter2018), and suicidal thoughts (Na et al., Reference Na, De Angelis, Nichter, Wendt, Krystal, Southwick and Pietrzak2022a). However, to our knowledge, no study has examined the genetic susceptibility for OUD in the context of environmental and psychosocial factors. Approaches using PRS derived from large-scale GWAS to investigate gene-environment interactions could advance our understanding of the biopsychosocial etiology of OUD and identify modifiable psychosocial and environmental targets for clinical and policy interventions.
We analyzed data from the Yale-Penn 3 cohort (Deak et al., Reference Deak, Zhou, Galimberti, Levey, Wendt, Sanchez-Roige and Gelernter2022) to evaluate the following aims: (1) to examine the main effect of an OUD PRS derived from an MTAG analysis (Deak et al., Reference Deak, Zhou, Galimberti, Levey, Wendt, Sanchez-Roige and Gelernter2022) after adjusting for psychosocial and environmental risk factors for OUD; (2) to measure the effects of the interaction of OUD PRS with the psychosocial environment (i.e. education level [Altekruse et al., Reference Altekruse, Cosgrove, Altekruse, Jenkins and Blanco2020], early exposure to traumas [Santo et al., Reference Santo, Campbell, Gisev, Tran, Colledge, Di Tanna and Degenhardt2021] and substances [Webster, Reference Webster2017]) and psychiatric disorders (i.e. MDD, PTSD [Strain, Reference Strain2022]) in relation to opioid dependence (OD), and (3) to evaluate the gain in predictive power when genetic and clinical data are combined. The psychosocial and environmental factors that were examined as potential moderators were selected based on previous literature on risk factors for OUD and OD (Altekruse et al., Reference Altekruse, Cosgrove, Altekruse, Jenkins and Blanco2020; Na et al., Reference Na, Scodes, Fishman, Rotrosen and Nunes2022b; Santo et al., Reference Santo, Campbell, Gisev, Tran, Colledge, Di Tanna and Degenhardt2021; Somer, Reference Somer2019; Strain, Reference Strain2022; Webster, Reference Webster2017).
Methods
Participants
Data were analyzed from 1958 European-ancestry (EUR) adults who participated in the Yale-Penn Study 3 cohort, a study focused on the genetics of SUDs and related conditions. Data for Yale-Penn 1 and Yale-Penn 2 (Zhou et al., Reference Zhou, Rentsch, Cheng, Kember, Nunez, Sherva and Gelernter2020a, Reference Zhou, Sealock, Sanchez-Roige, Clarke, Levey, Cheng and Gelernter2020b) were included in the OUD meta-GWAS used to create the PRS and thus the target sample was restricted to the Yale-Penn 3 sample to prevent overlap. Participants were recruited from five US sites (Gelernter et al., Reference Gelernter, Kranzler, Sherva, Koesterer, Almasy, Zhao and Farrer2014). All participants were interviewed by trained interviewers using the Semi-Structured Assessment for Drug Dependence and Alcoholism (SSADDA), which offers a comprehensive diagnostic assessment of psychiatric and SUDs and information on respondents' psychosocial and environmental characteristics (Pierucci-Lagha et al., Reference Pierucci-Lagha, Gelernter, Chan, Arias, Cubells, Farrer and Kranzler2007). The study protocol was approved by the Institutional Review Board at each site and all participants provided written informed consent.
Genotyping
Genotyping of the Yale-Penn cohorts has been described previously (Sherva et al., Reference Sherva, Wang, Kranzler, Zhao, Koester, Herman and Gelernter2016). Briefly, genotyping of Yale-Penn 3 was completed in the Gelernter laboratory at Yale School of Medicine using the Illumina multi-ethnic global array containing 1 779 819 single nucleotide polymorphisms. Following genotyping and quality control, imputation was performed using Minimac3 (Das et al., Reference Das, Forer, Schönherr, Sidore, Locke, Kwong and Fuchsberger2016) as incorporated in the Michigan Imputation Server (https://imputationserver.sph.umich.edu) (Michigan imputation server: Free next-generation genotype imputation service) and the Haplotype Reference Consortium reference panel (McCarthy et al., Reference McCarthy, Das, Kretzschmar, Delaneau, Wood, Teumer and Durbin2016). Standard quality control procedures were used, which included restricting variants to those with a minor allele frequency > 0.01, a Hardy–Weinberg Equilibrium p value > 1.0 × 10–6, and an imputation quality (INFO score) > 0.8.
Polygenic Risk Score
The OUD PRS calculated in the current study was based on summary statistics from a GWAS of OUD and related SUDs using MTAG (Deak et al., Reference Deak, Zhou, Galimberti, Levey, Wendt, Sanchez-Roige and Gelernter2022). Given evidence of high positive genetic correlation (r g = 0.77–0.82) between GWAS meta-analyses of OUD, alcohol use disorder (Zhou et al., Reference Zhou, Sealock, Sanchez-Roige, Clarke, Levey, Cheng and Gelernter2020b), and cannabis use disorder (Johnson et al., Reference Johnson, Demontis, Thorgeirsson, Walters, Polimanti, Hatoum and Agrawal2020), a joint-analysis of these three SUDs using MTAG was performed (Turley et al., Reference Turley, Walters, Maghzian, Okbay, Lee, Fontana and Benjamin2018). MTAG (Turley et al., Reference Turley, Walters, Maghzian, Okbay, Lee, Fontana and Benjamin2018) is an approach that can increase GWAS statistical power for a trait of interest, in this case OUD, by including closely related traits to enhance genetic discovery. Using a leave-one-out approach (excluding Yale-Penn 3 from the OUD GWAS meta-analysis), the MTAG-based OUD PRS was a stronger predictor of OD in Yale-Penn 3 subjects than the OUD-only PRS (Deak et al., Reference Deak, Zhou, Galimberti, Levey, Wendt, Sanchez-Roige and Gelernter2022) and thus was selected as the PRS predictor for the current analysis (Deak et al., Reference Deak, Zhou, Galimberti, Levey, Wendt, Sanchez-Roige and Gelernter2022).
The OUD PRS was calculated for Yale-Penn 3 participants using PRS-CS (Ge, Chen, Ni, Feng, & Smoller, Reference Ge, Chen, Ni, Feng and Smoller2019) and summed across chromosomes and standardized using PLINK (Purcell et al., Reference Purcell, Neele, Todd-Brown, Thomas, Ferreira, Bender and Sham2007). Related individuals (second-degree relatives or closer) in Yale-Penn 3 were identified and one individual from each relationship pair was excluded from the analysis, which yielded N = 1958. Variants included in the PRS were limited to those in HapMap 3 (International HapMap, 3 Consortium, 2010). The 1000 genomes EUR reference panel was used (1000 Genome Project Consortium et al., Reference Auton, Brooks, Durbin, Garrison, Kang and Abecasis2015) across analytic steps.
The OUD PRS was then included in the respective models described below, which covaried age, sex, and the first 10 within-ancestry genetic principal components.
Phenotypic traits studied
Opioid dependence
Lifetime DSM-IV OD (American Psychiatric Association, 1994) was assessed using the SSADDA (Pierucci-Lagha et al., Reference Pierucci-Lagha, Gelernter, Chan, Arias, Cubells, Farrer and Kranzler2007). Interviewing methods and the diagnostic reliability of the SSADDA have been described previously (Pierucci-Lagha et al., Reference Pierucci-Lagha, Gelernter, Chan, Arias, Cubells, Farrer and Kranzler2007). The OD diagnosis demonstrated excellent test-retest (κ = 0.94) and interrater (κ = 0.91) reliability (Pierucci-Lagha et al., Reference Pierucci-Lagha, Gelernter, Chan, Arias, Cubells, Farrer and Kranzler2007). Studies have also shown a high kappa value between OD and DSM-5 OUD (Boscarino et al., Reference Boscarino, Rukstalis, Hoffman, Han, Erlich, Ross and Stewart2011).
Sociodemographic variables
Age, sex (male v. female), partnered status (partnered v. not partnered), annual household income ($ 0–9999, $ 10 000–19 999, $ 20 000–29 999, $ 30 000–39 999, $ 40 000–49 999, $ 50 000–74 999, $ 75 000–99 999, $ 100 000–149 999, $ 150 000 or more/year) data were collected.
Potential moderating variables
Potential moderators of the OUD PRS association with OD were selected based on identified risk factors for OUD and genetic studies of OD and OUD (Altekruse et al., Reference Altekruse, Cosgrove, Altekruse, Jenkins and Blanco2020; Na et al., Reference Na, Scodes, Fishman, Rotrosen and Nunes2022b; Santo et al., Reference Santo, Campbell, Gisev, Tran, Colledge, Di Tanna and Degenhardt2021; Somer, Reference Somer2019; Strain, Reference Strain2022; Webster, Reference Webster2017). We evaluated interactions between OUD PRS and the following variables in relation to OD based on previous findings: education level (Altekruse et al., Reference Altekruse, Cosgrove, Altekruse, Jenkins and Blanco2020), quality of relationship with primary caregiver (Caspers, Cadoret, Langbehn, Yucuis, & Troutman, Reference Caspers, Cadoret, Langbehn, Yucuis and Troutman2005), early exposure to traumas (Santo et al., Reference Santo, Campbell, Gisev, Tran, Colledge, Di Tanna and Degenhardt2021), early substance use in the household (Webster, Reference Webster2017), and MDD and PTSD diagnoses (Strain, Reference Strain2022). Variables that may be prone to reverse causation (i.e. a result of OUD), such as income, employment status, relationship status and quality were excluded from the interaction analyses.
Education was assessed with the question: ‘What is the highest grade in school you completed?’ Responses were categorized as (1) less than high school (1–11 year(s)); (2) high school graduate (12 years); (3) some college (13–15 years); and (4) bachelor's degree or higher (⩾16 years).
Quality of early relationship with primary caregiver was assessed with the following question: ‘How would you describe the quality of your relationship with your main caregiver up to age 13?’ Response options were Excellent, Very good, Good, Fair, or Poor.
Witnessed violence before 13 was assessed with the question: ‘Did you ever witness or experience a violent crime, like a shooting or a rape, by age 13?’ Response options were No or Yes.
Sexual abuse before 13 was assessed with the question: ‘By the time you were age 13, were you ever sexually abused?’ Response options were No or Yes.
Physical abuse before 13 was assessed with the question: ‘By the time you were age 13, were you ever beaten by an adult so badly that you needed medical care, or had marks on your body that lasted for more than 30 days?’ Response options were No or Yes.
Substance use in household before 13 was assessed with the question: ‘Were you ever aware of adults in your household, or your older siblings, drinking enough to get drunk, or using drugs or alcohol, by the time you were 13?’ Response options were No or Yes.
Lifetime MDD diagnosis was assessed with the SSADDA, which uses the DSM-IV lifetime diagnosis algorithm for the disorder (American Psychiatric Association, 1994).
Lifetime PTSD diagnosis was assessed with the SSADDA, which uses the DSM-IV lifetime diagnosis algorithm for the disorder (American Psychiatric Association, 1994).
Other substance dependence
Lifetime DSM-IV alcohol, cannabis, cocaine, and other dependence (American Psychiatric Association, 1994) were assessed using the SSADDA (Pierucci-Lagha et al., Reference Pierucci-Lagha, Gelernter, Chan, Arias, Cubells, Farrer and Kranzler2007). Interviewing methods and the diagnostic reliability of the SSADDA have been described previously (Pierucci-Lagha et al., Reference Pierucci-Lagha, Gelernter, Chan, Arias, Cubells, Farrer and Kranzler2007).
Data analysis
Data analyses proceeded in three steps. First, we conducted chi-square and univariate analyses of variance to compare sociodemographic, psychosocial, environmental and psychiatric characteristics by OD status. Second, we correlated the OUD PRS with potential moderating variables. Third, we conducted a multivariable logistic regression analysis to examine associations between OUD PRS, environmental and psychosocial factors, and their interaction in relation to the presence or absence of OD. These analyses were adjusted for age, sex, top 10 within-ancestry principal components, interactions of OUD PRS by age and sex, and significant environmental and psychosocial factors by age and sex. A backward elimination estimation approach was employed to identify significant (likelihood ratio p value < 0.05) OUD PRS × environment and psychosocial interaction terms for the 8 potential moderating variables (i.e. education level, quality of relationship with primary caregiver, witnessed trauma, sexual abuse, physical abuse, and substance use in household before age 13, lifetime MDD diagnosis, and lifetime PTSD diagnosis). Predicted probabilities of OD were then computed and plotted to illustrate significant interaction effects. Lastly, a relative importance analysis (Tonidandel & LeBreton, Reference Tonidandel and LeBreton2010) was conducted to quantify the relative variance in OD that was explained by each of the statistically significant variables identified in the regression analysis.
Results
The average age of participants (n = 1958) was 41.5 years (s.d. = 15.1: range 16–84), 52.0% were female, and 21.5% (n = 420) had OD. Table 1 presents descriptive statistics of the sample by OD status. After Bonferroni correction, group differences were observed for all of the study variables (p < 0.05/16 = 0.0031). Individuals with OD were younger and more likely to be male, had fewer years of education and lower household income, and were less likely to be partnered. They were also more likely to have witnessed violence, been sexually or physically abused, report substance use in the household before age 13, and a lower quality of relationship with their primary caregiver and lifetime diagnoses of MDD and PTSD.
Table 1. Sociodemographic, trauma, and psychiatric characteristics by opioid dependence status
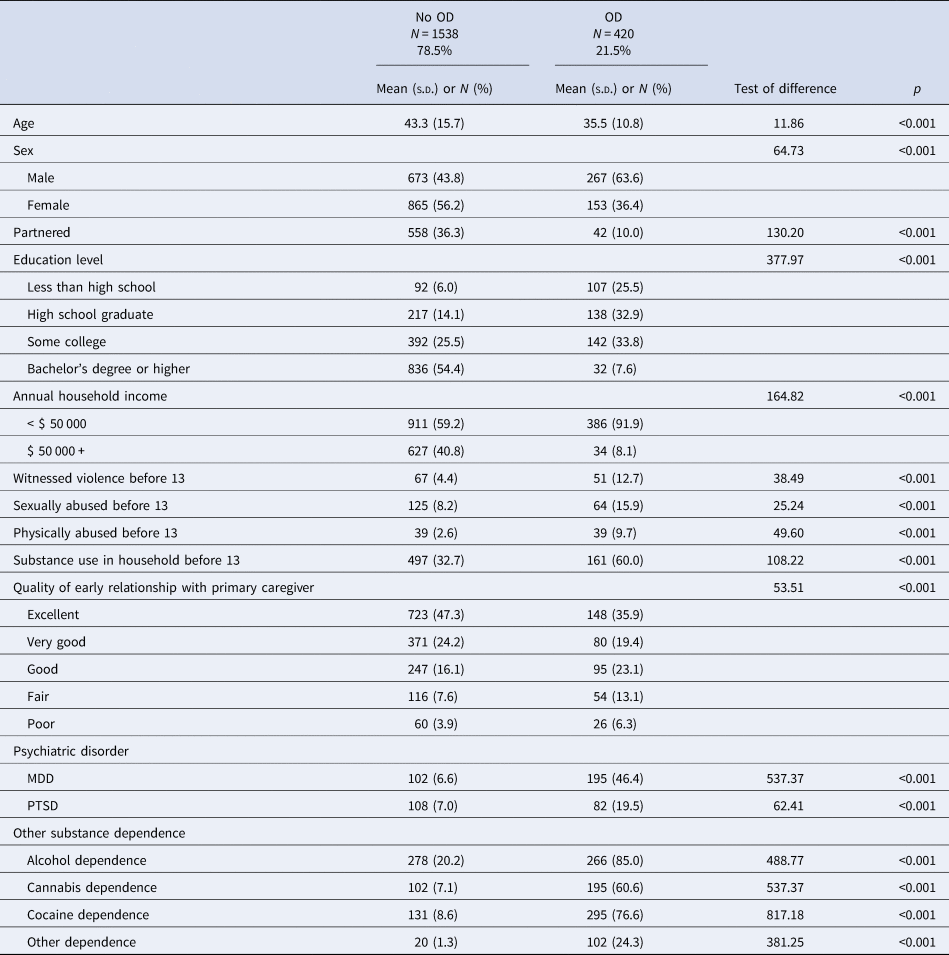
Note. MDD, major depressive disorder; OD, opioid dependence; PTSD, posttraumatic stress disorder; SUD, substance use disorder.
Results of multivariable logistic regression analysis
As shown in Table 2, OUD PRS, male sex, substance use in household before age 13, and PTSD were positively associated with OD, whereas age, education level, household income, and being partnered were negatively associated. The Nagelkerke R 2 of the initial multivariable model without interaction terms was 0.468.
Table 2. Results of multivariable logistic regression analyses in relation to opioid dependence
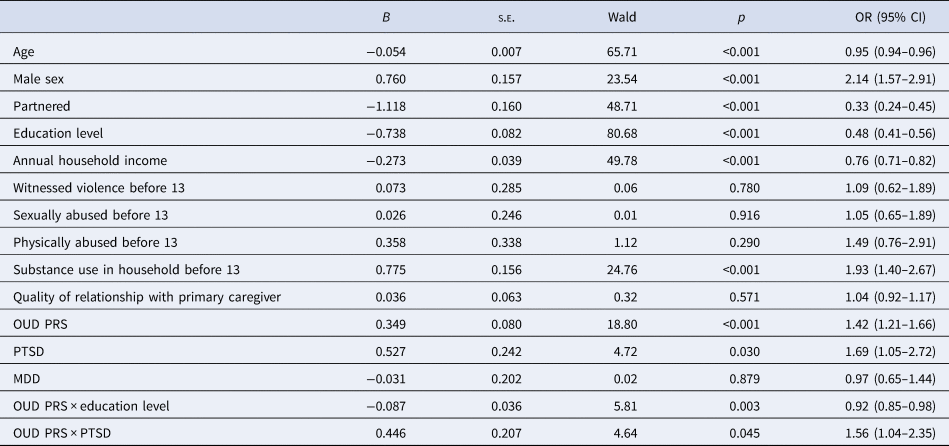
Note. Adjusted for the top 10 within-ancestry principal components.
CI, confidence interval; OR, odds ratio; OUD, opioid use disorder; MDD, major depressive disorder; PTSD, posttraumatic stress disorder; s.e., standard error.
Figure 1 shows results of a relative importance analysis. Annual household income and education level explained 25.9 and 22.7% of the total explained variance of the multivariable model (R 2 = 0.468), respectively. Age explained 18.7%, and partnered status explained 15.3% of this variance. OUD MTAG PRS explained 8.0% of this variance, and sex and substance use in the household before age 13 explained 5.7% and 3.6%, respectively.
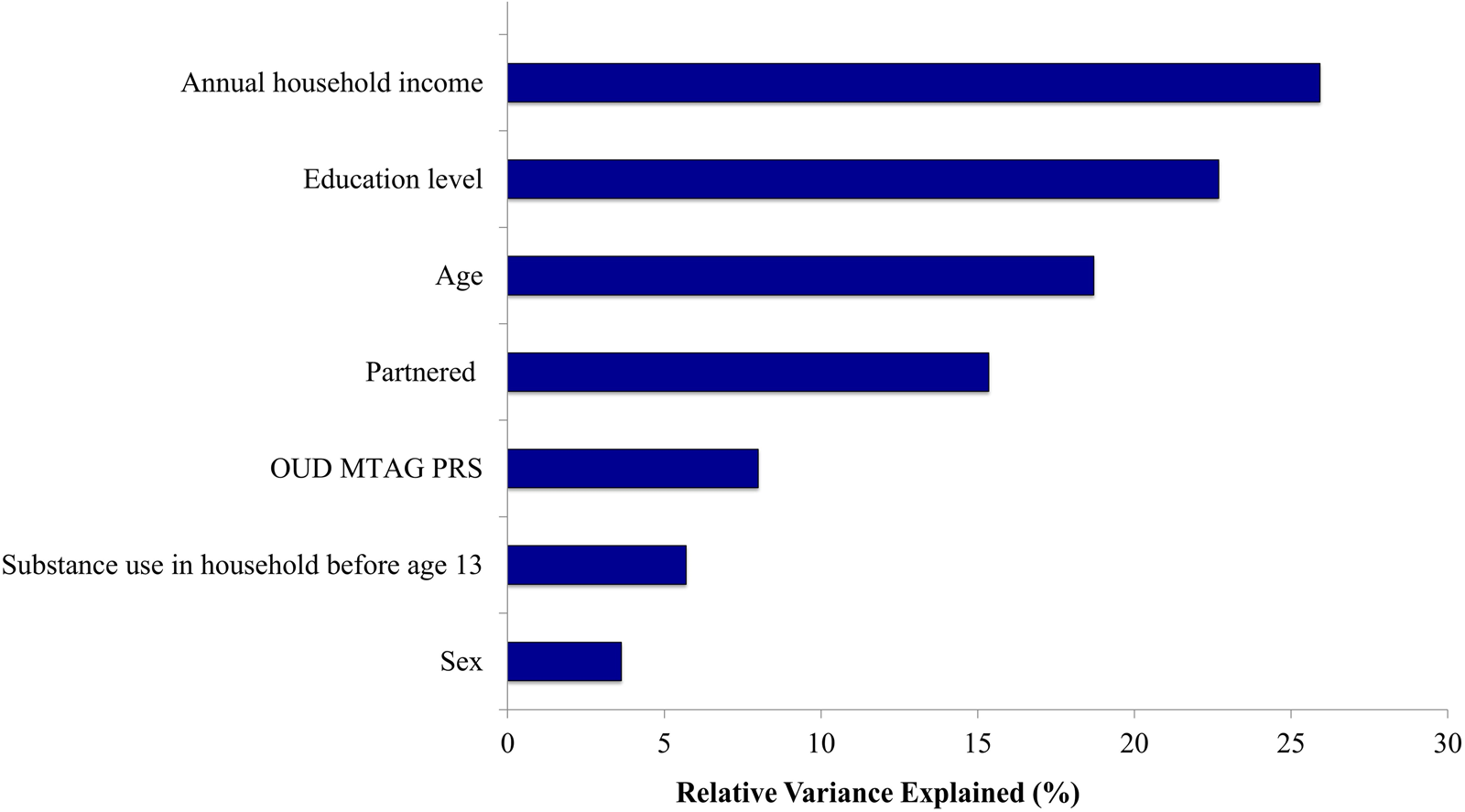
Figure 1. Results of relative importance analysis in relation to opioid dependence.
Note. OUD, opioid use disorder; MTAG, multi-trait analysis of genome-wide association studies; PRS, polygenic risk scores.
Relative variance explained reflects the proportion of the total variance explained in the multivariable model without interaction terms (R 2 = 0.468).
Interactions of OUD PRS × psychosocial and environmental factors
Two psychosocial and environmental factors – education level (p = 0.003) and lifetime PTSD diagnosis (p = 0.045) – showed significant interaction effects with OUD PRS in relation to OD after adjusting for all other variables in the multivariable model. Other potential moderating variables were not retained in the final model (all p > 0.05).
Figure 2 shows the probability of OD by education level and OUD PRS separately, and the interaction between OUD PRS and education level. The highest probability of OD was observed among individuals with higher OUD PRS and less than a high school education. Specifically, among individuals with higher OUD PRS, those with higher education were less likely to have an OD diagnosis (odds ratio [OR] 0.92, 95% confidence interval [CI] 0.85–0.98). For example, among individuals in the highest OUD PRS quartile (highest genetic risk), those with a Bachelor's degree or higher education had a 5-fold lower probability of OD relative to those with less than high school education (12.3% v. 62.4%).
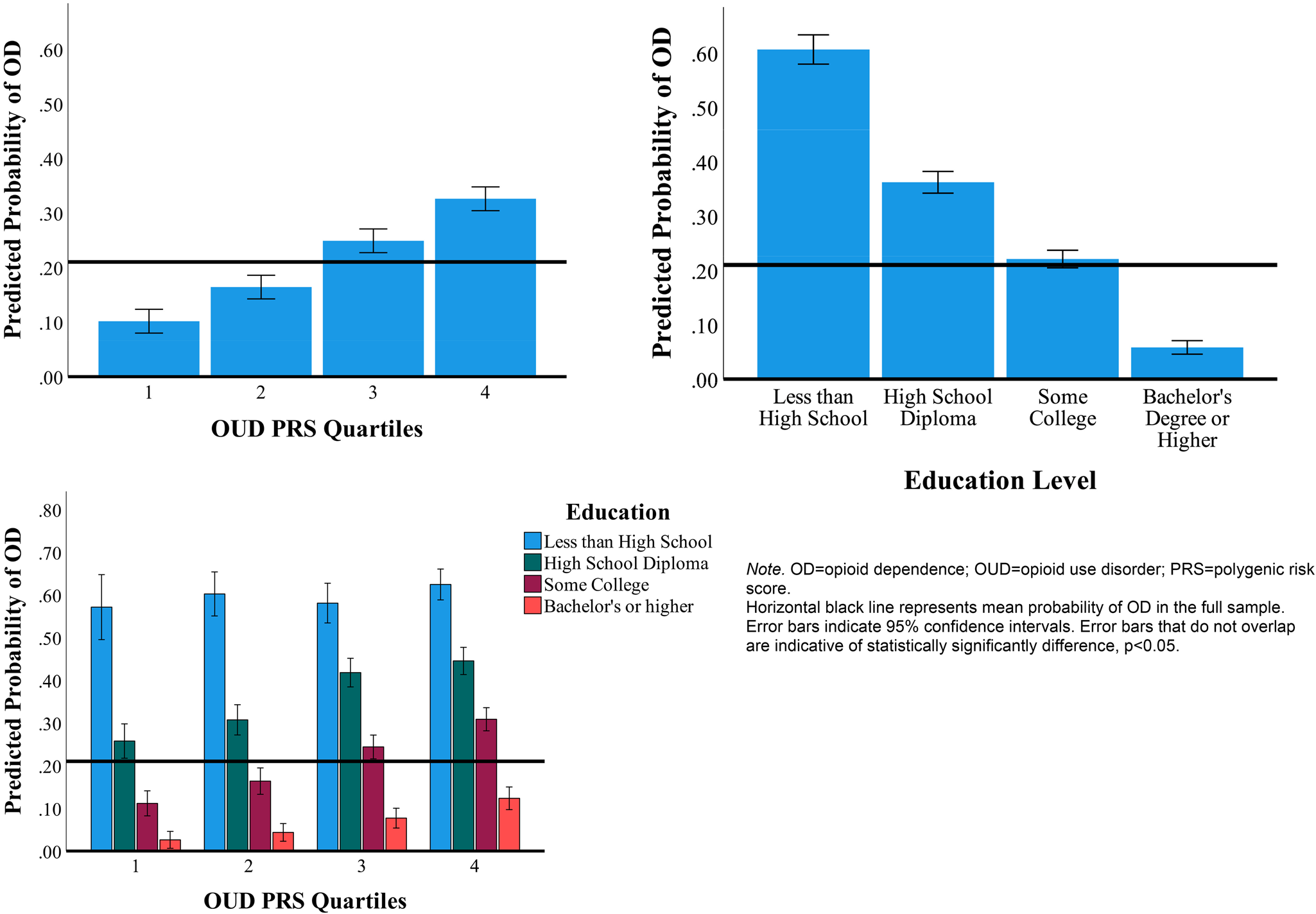
Figure 2. Probability of opioid dependence by OUD PRS, education level, and OUD PRS-by-education.
Note. OD, opioid dependence; OUD, opioid use disorder; PRS, polygenic risk score. Horizontal black line represents mean probability of OD in the full sample. Error bars indicate 95% confidence intervals. Error bars that do not overlap are indicative of statistically significantly difference, p < 0.05. Highest PRS quartiles indicate highest genetic risk for OUD, whereas lowest PRS quartiles indicate lowest genetic risk for OUD.
Figure 3 shows the interaction between OUD PRS and PTSD, which showed the highest probability of OUD among individuals with high OUD PRS and PTSD. Specifically, among individuals with higher OUD PRS, those with PTSD were more likely to have OD than those without PTSD (OR 1.56, 95% CI 1.04–2.35). For example, among individuals in the highest OUD PRS quartile (highest genetic risk), those with PTSD had 48% higher probability of OD relative to those without PTSD (46.4% v. 31.4%).
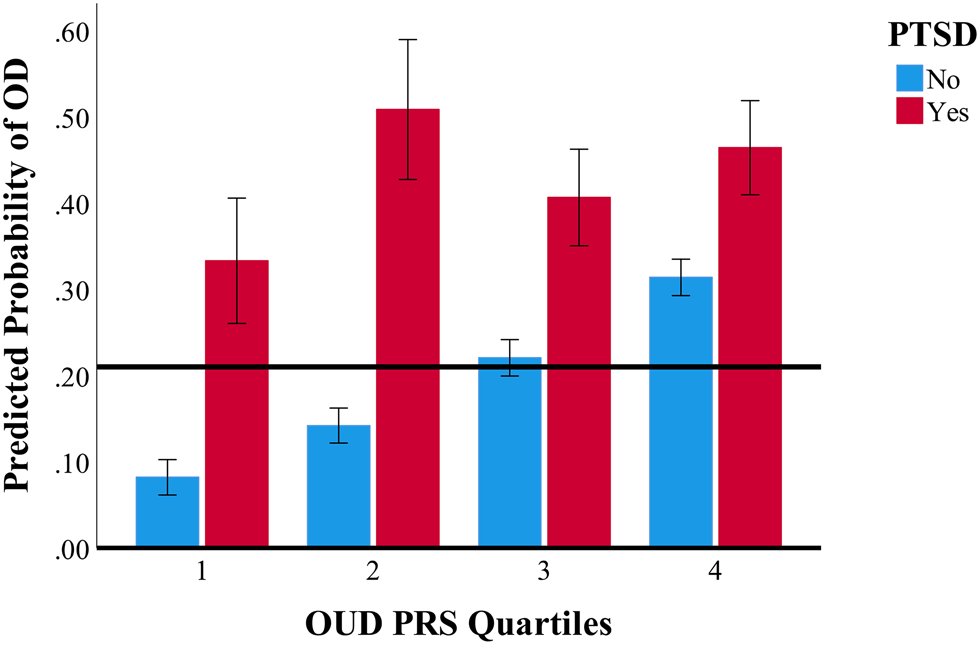
Figure 3. Interaction of PTSD and OUD PRS in relation to the probability of opioid dependence.
Note. OD, opioid dependence; OUD, opioid use disorder; PRS, polygenic risk score; PTSD, posttraumatic stress disorder.
Horizontal black line represents mean probability of OD in the full sample.
Error bars indicate 95% confidence intervals. Error bars that do not overlap are indicative of statistically significantly difference, p < 0.05. Highest PRS quartiles indicate highest genetic risk for OUD, whereas lowest PRS quartiles indicate lowest genetic risk for OUD.
Discussion
We found that OUD PRS derived from a large scale MTAG analysis (Deak et al., Reference Deak, Zhou, Galimberti, Levey, Wendt, Sanchez-Roige and Gelernter2022) was positively associated with risk for OD, even after adjusting for known psychosocial risk factors for OD and OUD. In addition, we identified two independent psychosocial/environmental correlates that moderated the effect of OUD PRS on risk for OD – education level and lifetime PTSD diagnosis.
The significant main effect of the OUD PRS on OD risk aligns with previous literature demonstrating cumulative effects of common genetic variants on risk for OD (Crist, Reiner, & Berrettini, Reference Crist, Reiner and Berrettini2019). This association remained significant after accounting for major sociodemographic (i.e. age, sex, education level, household income, partnered status) and psychiatric risk factors (i.e. childhood adversities, co-occurring psychiatric disorders such as MDD and PTSD). To our knowledge, this is the first study that observed a persistent association between OUD PRS and risk for OD after controlling for major psychosocial and environmental variables. This finding demonstrates the potential utility of OUD PRS in identifying individuals at increased genetic risk for OUD in both clinical and community-based settings, though larger, more informative GWAS of OUD are needed to maximize the variance in OUD risk accounted for by PRS.
Education moderated the deleterious effect of OUD PRS in relation to OD risk. Previously, lower education level, in particular a failure to graduate from high school, was identified as a key risk factor for OUD, fatal drug use, and opioid overdose deaths (Altekruse et al., Reference Altekruse, Cosgrove, Altekruse, Jenkins and Blanco2020; Martins, Sampson, Cerda, & Galea, Reference Martins, Sampson, Cerda and Galea2015; Schepis, Teter, & McCabe, Reference Schepis, Teter and McCabe2018). For example, in a study of 14 553 young adult respondents, OUD was most prevalent among those who dropped out of high school (Schepis et al., Reference Schepis, Teter and McCabe2018). A study of 4 million survey responses from the 2008–2015 National Death Index found that relative to adults with graduate degrees, those with only a high school diploma had more than double the risk of death by opioid overdose (hazard ratio = 2.48, 95% CI 2.00–3.06). In addition, the lack of a Bachelor's degree has been highlighted as a key determinant of what they call ‘deaths of despair (i.e. deaths by drug overdose, alcohol-related diseases, and suicide)’ (Case & Deaton, Reference Case and Deaton2020). An implication of our finding that education moderated the effect of OUD PRS on OD risk is that interventions and/or policy measures that increase retention in school could help to mitigate the risk for OD, especially among individuals with higher polygenic liability for OUD.
Among individuals with higher OUD PRS, those with PTSD had a higher risk of OD. The high co-occurrence of OUD and PTSD has been shown in numerous studies (Dahlby & Kerr, Reference Dahlby and Kerr2020; Jones & McCance-Katz, Reference Jones and McCance-Katz2019; Mills, Teesson, Ross, & Peters, Reference Mills, Teesson, Ross and Peters2006). Many explanations for the link between the two disorders have been posited, including the ‘self-medication hypothesis (Dahlby & Kerr, Reference Dahlby and Kerr2020),’ in which an individual with PTSD attempts to alleviate his or her PTSD symptoms by using opioids. In a study that matched 4025 individuals exposed to opioid analgesics from the National Epidemiologic Survey on Alcohol and Related Conditions III, PTSD was linked to increased risk of OUD after exposure to opioid analgesics (Hassan, Le Foll, Imtiaz, & Rehm, Reference Hassan, Le Foll, Imtiaz and Rehm2017). Based on these findings, it has been suggested that clinicians should monitor closely when prescribing opioids to individuals with a history of PTSD (Wilcox, Reference Wilcox2017). Thus, in the future, OUD PRS may have utility in personalizing prescribing patterns for those with PTSD. However, given the cross-sectional nature of our data, this finding should be interpreted with caution, as it is also possible that OD could have contributed to the development of PTSD (Danovitch, Reference Danovitch2016).
Each of the environmental covariates retained in the final model explained more variance in OD than did OUD PRS. The relative importance analysis revealed that the selected environmental factors, such as annual household income and education level, explained on average 3-fold greater variance than the OUD PRS. Putting together all available information allows for accurate risk predictions based mostly on environmental covariates, with an additional contribution by the genetic data. Thus, current claims of clinically meaningful genetic risk prediction in OUD should be viewed skeptically. We showed previously that a commercially-available kit purporting to predict OUD risk clinically on the basis of a small number of ‘candidate gene’ variants, does not predict OUD at all, but does predict ancestry (Hatoum et al., Reference Hatoum, Wendt, Galimberti, Polimanti, Neale, Kranzler and Agrawal2021). This result is not surprising as our genome-wide genetic data do not yet account for a clinically meaningful proportion of OD risk despite comprising many more variants – and especially, many more variants shown to be significantly associated with the target trait – than commercially-available kits.
This study has several limitations. First, due to the cross-sectional nature of the study, it is not possible to ascertain the causal and temporal relations among the factors examined. However, given that most OD cases develop after high school, it is likely that educational attainment and early life adversities preceded the onset of the disorder, though it is possible that PTSD developed after its onset. Second, the relatively small size of the target sample limited the statistical power to detect other significant main effects or environmental moderators. Relatedly, to preserve statistical power, only interactions between OUD PRS and significant environmental variables with age and sex were force-entered into the multivariable model; further research in larger samples that include all possible PRS-by-covariate and environmental variable-by-covariate interactions(Keller, Reference Keller2014) is needed to evaluate the robustness of the identified environmental moderators of OUD PRS in predicting OD. Third, the measures of environmental and psychosocial factors utilized in this study were based on self-report, and largely binary and retrospective in nature. Future study using a more structured, multidimensional measure is needed. Fourth, the study sample was comprised entirely of EUR individuals due to lack of sufficient non-EUR GWAS data available for PRS analysis. Additionally, the Yale-Penn study 3 cohort was intentionally designed to recruit individuals with SUDs. Thus, it is unclear whether the results of the study can be generalized either to non-EUR populations or to the general population of EUR individuals. Larger PRS-by-environment interaction studies are needed in more diverse, population-based samples.
Conclusion
Notwithstanding these limitations, results of this study showed that OUD polygenic risk was associated with OD even after considering a number of known major risk factors for OD and OUD. Further, lower education and the presence of a PTSD diagnosis were also associated with OD and moderated the association between OUD PRS and OD. Our results demonstrate the potential utility of examining OUD PRS in combination with psychosocial and environmental factors to understand the biopsychosocial etiology of OD and to develop prediction models for the disorder. Research is needed to replicate and extend these findings to other samples, elucidate the biopsychosocial mechanisms linking OUD PRS to risk for OD, and evaluate the efficacy of clinical and policy measures targeting key moderators of OUD PRS in mitigating risk for OD.
Acknowledgment
This work was supported by NIH grants R01DA054869 (JG), R01 DA051906 (JG), R01 AA026364 (JG), and T32 AA028259 (JDD); and by funding from the Department of Veterans Affairs Office of Research and Development, USVA, grants I01CX001849, I01BX003342, I01BX004820, I01 CX001734, and 1IK1CX002532-01 (PJN) and the VA Cooperative Studies Program study, no. 575B. This research is based on data from the Million Veteran Program, Office of Research and Development, Veterans Health Administration, and was supported by award #CSP575B, MVP004, and MVP025. This publication does not represent the views of the Department of Veterans Affairs or the United States Government.
Competing interests
In the past three years, Dr Na has received royalties from Wolters Kluwer. Dr Kranzler is a member of scientific advisory boards for Dicerna Pharmaceuticals, Sophrosyne Pharmaceuticals, Clearmind Medicine, and Enthion Pharmaceuticals; a consultant to Sophrosyne Pharmaceuticals; and a member of the American Society of Clinical Psychopharmacology's Alcohol Clinical Trials Initiative, which during the past three years was supported by Alkermes, Dicerna, Ethypharm, Lundbeck, Mitsubishi, and Otsuka. Drs. Kranzler and Gelernter are named as inventors on PCT patent application #15/878640 entitled: ‘Genotype-guided dosing of opioid agonists,’ filed 24 January 2018 and issued on 26 January 2021 as U.S. Patent No. 10900082. Dr Gelernter is paid for his editorial work on the journal Complex Psychiatry. Drs. Deak and Pietrzak report no competing interests.