Book contents
- Sampling in Judgment and Decision Making
- Sampling in Judgment and Decision Making
- Copyright page
- Contents
- Figures
- Tables
- Contributors
- Part I Historical Review of Sampling Perspectives and Major Paradigms
- Part II Sampling Mechanisms
- Part III Consequences of Selective Sampling
- Part IV Truncation and Stopping Rules
- Part V Sampling as a Tool in Social Environments
- Part VI Computational Approaches
- Index
- References
Part II - Sampling Mechanisms
Published online by Cambridge University Press: 01 June 2023
- Sampling in Judgment and Decision Making
- Sampling in Judgment and Decision Making
- Copyright page
- Contents
- Figures
- Tables
- Contributors
- Part I Historical Review of Sampling Perspectives and Major Paradigms
- Part II Sampling Mechanisms
- Part III Consequences of Selective Sampling
- Part IV Truncation and Stopping Rules
- Part V Sampling as a Tool in Social Environments
- Part VI Computational Approaches
- Index
- References
Summary
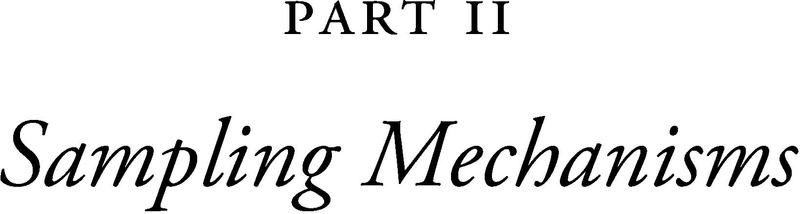
- Type
- Chapter
- Information
- Sampling in Judgment and Decision Making , pp. 113 - 204Publisher: Cambridge University PressPrint publication year: 2023