Book contents
- Frontmatter
- Contents
- Preface
- 1 Introduction
- 2 Fixed-Effects Models
- 3 Models with Random Effects
- 4 Prediction and Bayesian Inference
- 5 Multilevel Models
- 6 Stochastic Regressors
- 7 Modeling Issues
- 8 Dynamic Models
- 9 Binary Dependent Variables
- 10 Generalized Linear Models
- 11 Categorical Dependent Variables and Survival Models
- Appendix A Elements of Matrix Algebra
- Appendix B Normal Distribution
- Appendix C Likelihood-Based Inference
- Appendix D State Space Model and the Kalman Filter
- Appendix E Symbols and Notation
- Appendix F Selected Longitudinal and Panel Data Sets
- References
- Index
- References
References
Published online by Cambridge University Press: 05 September 2012
- Frontmatter
- Contents
- Preface
- 1 Introduction
- 2 Fixed-Effects Models
- 3 Models with Random Effects
- 4 Prediction and Bayesian Inference
- 5 Multilevel Models
- 6 Stochastic Regressors
- 7 Modeling Issues
- 8 Dynamic Models
- 9 Binary Dependent Variables
- 10 Generalized Linear Models
- 11 Categorical Dependent Variables and Survival Models
- Appendix A Elements of Matrix Algebra
- Appendix B Normal Distribution
- Appendix C Likelihood-Based Inference
- Appendix D State Space Model and the Kalman Filter
- Appendix E Symbols and Notation
- Appendix F Selected Longitudinal and Panel Data Sets
- References
- Index
- References
Summary
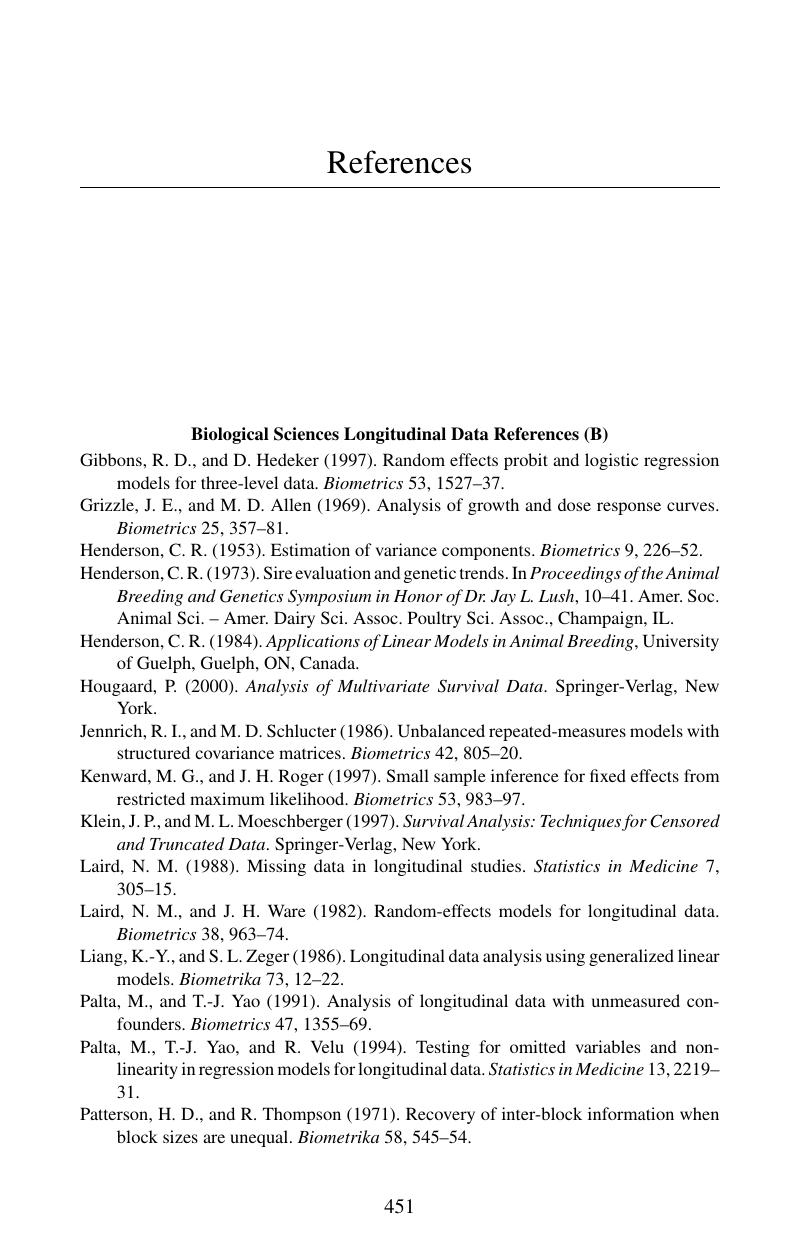
- Type
- Chapter
- Information
- Longitudinal and Panel DataAnalysis and Applications in the Social Sciences, pp. 451 - 462Publisher: Cambridge University PressPrint publication year: 2004