Book contents
- The Handbook of Medical Image Perception and Techniques, Second Edition
- Reviews
- The Handbook of Medical Image Perception and Techniques
- Copyright page
- Dedication
- Contents
- Contributors
- 1 Medical Image Perception
- Part I Historical Reflections and Theoretical Foundations
- Part II Science of Image Perception
- Part III Perception Metrology
- Part IV Clinical Performance Assessment
- Part V Computational Perception
- Part VI Applied Perception
- 28 Optimization of 2D and 3D Radiographic Imaging Systems
- 29 Display Optimization from a Physics Perspective
- 30 Display Optimization from a Perception Perspective
- 31 Perception and Training
- 32 Ergonomics 2.0: Fatigue in Medical Imaging
- 33 Perception Issues in Pathology
- 34 Medical Image Perception from a Clinical Perspective
- 35 Future of Medical Image Perception
- Index
- References
31 - Perception and Training
from Part VI - Applied Perception
Published online by Cambridge University Press: 20 December 2018
- The Handbook of Medical Image Perception and Techniques, Second Edition
- Reviews
- The Handbook of Medical Image Perception and Techniques
- Copyright page
- Dedication
- Contents
- Contributors
- 1 Medical Image Perception
- Part I Historical Reflections and Theoretical Foundations
- Part II Science of Image Perception
- Part III Perception Metrology
- Part IV Clinical Performance Assessment
- Part V Computational Perception
- Part VI Applied Perception
- 28 Optimization of 2D and 3D Radiographic Imaging Systems
- 29 Display Optimization from a Physics Perspective
- 30 Display Optimization from a Perception Perspective
- 31 Perception and Training
- 32 Ergonomics 2.0: Fatigue in Medical Imaging
- 33 Perception Issues in Pathology
- 34 Medical Image Perception from a Clinical Perspective
- 35 Future of Medical Image Perception
- Index
- References
Summary
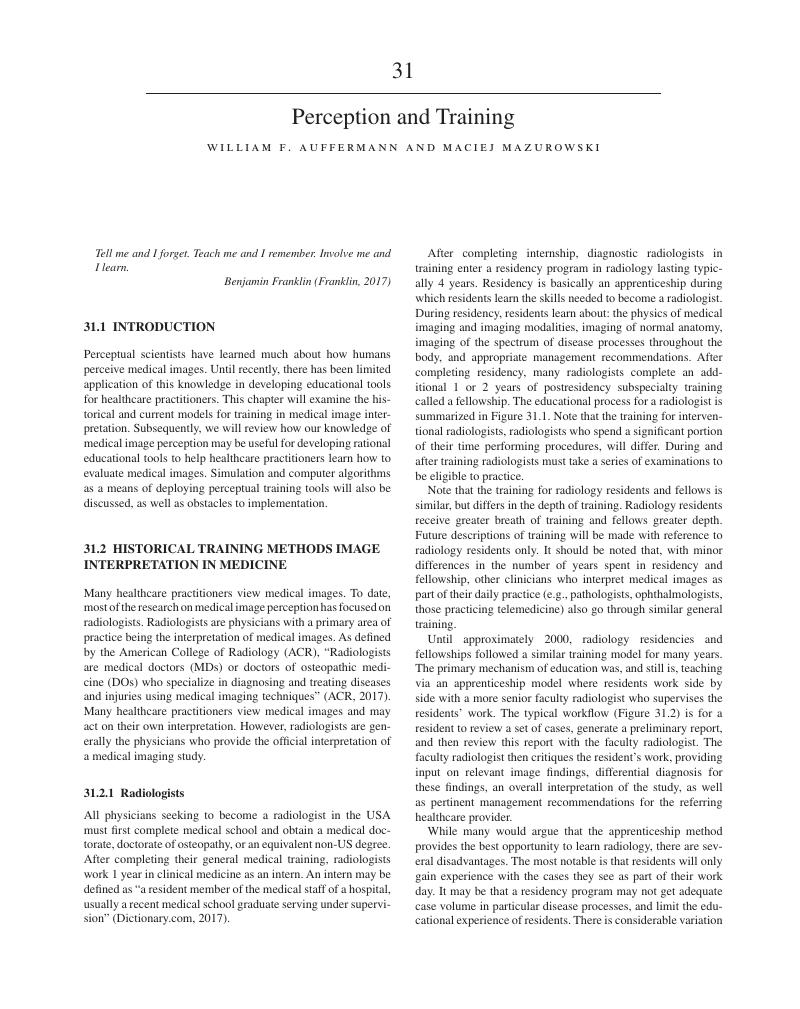
- Type
- Chapter
- Information
- The Handbook of Medical Image Perception and Techniques , pp. 470 - 482Publisher: Cambridge University PressPrint publication year: 2018
References
- 1
- Cited by