Implications
Since this is a position paper, the implications are restricted to what might transpire if sensor technologies were to come into widespread use in the dairy industry. There is a potential for the health and welfare of dairy animals to improve as a result of better, more consistent and more frequent (even continuous) monitoring. This would impact directly and positively on farmer profitability, which could also benefit from a reduced labour requirement, and the industry as a whole could benefit from improved consumer perception as a consequence of better animal wellbeing.
Introduction: changes, capabilities and challenges in dairying
Some knowledge of the local and global dairy industries is needed if one is to appreciate the potential impact of ‘smart technology’. In the latter years of the 20th century, UK dairy farms were small, cows were known individually and incomes were often marginal. Dairy products were, and still are, supermarket ‘loss leaders’, a key part of cheap food policies that proliferated across developed countries (Caraher and Coveney, Reference Caraher and Coveney2004). We worked long hours and, besides attaching milking clusters, one of my regular jobs when our cow numbers increased to more than 100 was the late night walk through the herd looking for the increased activity of cows in heat that would be inseminated the next day. Later, as a lactation scientist, I became actively involved in biological support to the engineering research that developed one of the first automatic milking systems (AMS) (Hillerton et al., Reference Hillerton, Knight, Turvey, Wheatley and Wilde1990), a technology that has come to be regarded as a dairy industry harbinger of what Daniel Beckmans dubbed Precision Livestock Farming (PLF) (Berckmans, 2008 and Reference Berckmans2014; John et al., Reference John, Clark, Freeman, Kerrisk, Garcia and Halachmi2016). What was the point of AMS? Almost certainly it was intended to reduce labour costs, although marketing of such systems has mainly focused on farmer lifestyle and cow choice. What was the chief capability that enabled AMS? In order to locate the teats for cluster attachment, the early robotic arms needed to know which cow they were dealing with so as to access a database of udder coordinates, and so radiofrequency identity (RFID: first patented by Hanton and Leach (Reference Hanton and Leach1974) as an indwelling rumen bolus radiofrequency device) was the key element. Whether directly linked or not, the gradual uptake of AMS has been accompanied by significant increases in the size of dairy farms (Robbins et al., Reference Robbins, von Keyserlingk, Fraser and Weary2016), a change that has been driven in large part by simple economics: as the number of cows increases, the farm’s fixed overhead costs are diluted, especially if AMS allows the expansion to occur without extra labour. The dairy industry is a curious mix of entrepreneurship (adoption of robotics) and extreme conservatism; we were able to demonstrate convincingly that encouraging more frequent attendance at AMS could result in greater lactation persistency and hence enable extended lactations (Pettersson et al., Reference Pettersson, Svennersten-Sjaujna and Knight2011), but the equally clear demonstration that longer lactations can be economically advantageous (De Vries, Reference De Vries2006) has never been accepted by farmers (or perhaps genetics companies!), who still aim to maximise output from AMS by increasing cow numbers, maximising the genetic potential of the individual cow and rebreeding to an annual schedule. These latter two objectives do not fit well together: high-yielding dairy cows suffer from ‘infertility’, that is to say, a reduced chance of rebreeding at around 60 days postpartum, regarded by many as a key benchmark (but see Dobson et al., Reference Dobson, Smith, Royal, Knight and Sheldon2007 for a critical analysis). Technology has come to the fore in addressing this issue and the ‘herd walk-through’ is no longer needed. Cows that show increased activity indicative of estrous will be automatically flagged by a pedometer, collar, ear tag, rumen bolus or other device incorporating an accelerometer. Most importantly, the cost is not prohibitive, due in no small part to very much cheaper computing power (a modern ‘tablet’ contains processing capability that would have cost a theoretical $10B in the 1960s: Michie et al., Reference Michie, Andonovic, Davison, Tachtatzis, Hamilton, Gilroy and Johnsson2020) and microcircuitry that ensures that sensors are small (rumen boluses are now available for sheep and goats, in addition to cattle: Castro-Costa et al., Reference Castro-Costa, Salama, Moll, Aguiló and Caja2015).
Larger herds, less intensive labour input, better reproductive management; these should certainly be regarded as important changes that have occurred in the last few decades driven by economics and assisted by technological capabilities. So, what of challenges? In the developed world, obesity is now commonly (but not yet scientifically) regarded as a greater health risk factor than smoking. This belief can actually be traced back almost to the millennium (Sturm and Wells, Reference Sturm and Wells2002), but the problem shows no signs of abating. Fitness tracking is part of an increasing trend to exercise more and live a better, healthier lifestyle, as recently reviewed by Böhm et al. (Reference Böhm, Karwiese, Böhm and Oberhoffer2019). Lifestyle choices extend to diet, where dairy products are often seen as obesogenic, hugely damaging to the environment and associated with poor animal welfare (see, e.g., Willett et al., Reference Willett, Rockström, Loken, Springmann, Lang, Vermeulen, Garnett, Tilman, DeClerck, Wood, Jonell, Clark, Gordon, Fanzo, Hawkes, Zurayk, Rivera, De Vries, Sibanda, Afshin, Chaudhary, Herrero, Agustina, Branca, Lartey, Fan, Crona, Fox, Bignet, Troell, Lindahl, Singh, Cornell, Reddy, Narain, Nishtar and Murray2019). The first two of these arguments are totally refutable (see, e.g., Guo et al., Reference Guo, Dougkas, Elwood and Givens2018 regarding obesity and for environmental sustainability, official Food and Agriculture Organization (FAO) data (FAO, 2010) showing that dairy production and distribution generate less than 3% of global greenhouse gas emissions). The third challenge is less easy to defend, since animal welfare is a highly subjective issue, but is an area in which sensors could have a very significant and positive impact. These ‘negative’ challenges are real and considerable, but dairying should also be seen as a positive part of another equally large societal challenge, our need to feed an expanding global population (Reynolds et al., Reference Reynolds, Wulster-Radcliffe, Aaron and Davis2015). There are contrasting views in this respect. In the developed world, consumers are encouraged to reduce dairy consumption (Willett et al., Reference Willett, Rockström, Loken, Springmann, Lang, Vermeulen, Garnett, Tilman, DeClerck, Wood, Jonell, Clark, Gordon, Fanzo, Hawkes, Zurayk, Rivera, De Vries, Sibanda, Afshin, Chaudhary, Herrero, Agustina, Branca, Lartey, Fan, Crona, Fox, Bignet, Troell, Lindahl, Singh, Cornell, Reddy, Narain, Nishtar and Murray2019), although the rationale for doing so is not particularly clear; in a nutritional context lower intakes appear to be justified on the basis of (perhaps) doing no harm, rather than doing good. In other parts of the world, the reality is rapid expansion of dairy production capacities; megadairies comprising many tens of thousands of cows are operating in Vietnam and under construction in Russia and China, for instance (Vietnam Investment Review, 2018) whilst a major project co-funded by governments and a major multinational milk processor aims to revolutionise milk production in West Africa (CARE Danmark, 2015). Global consumer demands for dairy products are almost certainly set to expand considerably, FAO predicting a 22% increase globally in the next 10 years with a concentration in India and Pakistan (OECD, 2018). The challenges are clear, and it seems inevitable that technology will be instrumental in enabling sustainable and ethical production standards in very large herds and in areas of the world that have little tradition of organised dairy production.
State of the art in dairy animal sensors
At the time of writing (late 2019), the search term ‘sensors and dairy cows’ yields 6 400 000 Internet hits, and this headline statement, taken from Dairy Global (2017): ‘Sensors used to detect oestrus, lameness, disease and calving are being touted as the next big thing in dairy production. It is not known, however, if these sensor systems actually improve the health and production of dairy herds’. Interestingly, replacing ‘cows’ by ‘animals’ in the search term reduced the number of hits (by 60%!) The take-home messages are clear:
there is a lot of interest in sensors
most of this relates to use in cows
it is not yet easy to justify the investment
Farmers are persuaded that investing in sensors that deliver improved estrous detection is a price worth paying, an analysis borne out by economic modelling (Rutten et al., Reference Rutten, Steeneveld, Inchaisri and Hogeveen2014 and Reference Rutten, Steeneveld, Oude Lansink and Hogeveen2018). By contrast, the same group concluded that sensors for body condition score are not yet a good investment, but may be in the future as the technology improves (Rutten et al., Reference Rutten, Steeneveld, Oude Lansink and Hogeveen2018). This leads to another take-home message:
state of the art currently mainly comprises stand-alone sensors for single applications
some of these (estrous detection in particular) are more mature than others
Perhaps it is not surprising, therefore, that estrous detection sensors dominate the market. In 2017, a colleague working for a manufacturer of sensors was able to identify 22 accelerometer-based commercially available sensor technologies for the neck, ear or leg, all but one of which professed to detect estrous (Thorup, Reference Thorup2017). A year earlier, our own review of the scientific literature identified 12 such products (Caja et al., Reference Caja, Castro-Costa and Knight2016). This should not be taken as an indication of the rate of expansion in the market! Perhaps our colleague did a better job than we did, but more likely this exposes a further take-home message:
many of the commercially available technologies have not yet been independently tested under rigorous scientific conditions
which takes us back to our earlier statement questioning whether sensors (yet) improve health and productivity. It is perhaps relevant that a recent meta-analysis of human activity trackers (sensors) concluded that almost all of a large research base (850/857 studies) were poorly controlled and ‘No evidence was found for the effect of wearable activity trackers, on physical-activity-related outcomes’ (Böhm et al., Reference Böhm, Karwiese, Böhm and Oberhoffer2019). Before considering whether these problems can be overcome such that sensors do have a future, it is important to have a fuller picture of the range of sensors that are available or conceived of.
Sensors fall into three broad categories, as shown in Figure 1. The first is often referred to as ‘Wearable’ sensors since these are found attached to the cow, but I prefer the term ‘At Cow’ which then also includes devices swallowed into the reticulorumen or inserted into the reproductive tract (red zone in Figure 1). By analogy, the second category is ‘Near Cow’ (blue zone in Figure 1) and the third is ‘From Cow’ (green zone in Figure 1). Near Cow includes all remote sensors that could watch, listen to, track, weigh, record or interrogate the cow or its immediate environment and includes elements that are geographically very remote (Global Positioning Satellite technology, GPS and cloud), but still ‘near’ in the sense of enabling real-time interaction. From Cow is the specific category of sensors that could collect and analyse data relating to products that have come from the cow, milk analysis being the prime example but biomarker analysis of body tissues/fluids (e.g., hair, saliva, sweat, nasal secretion, breath, faeces) also being included.
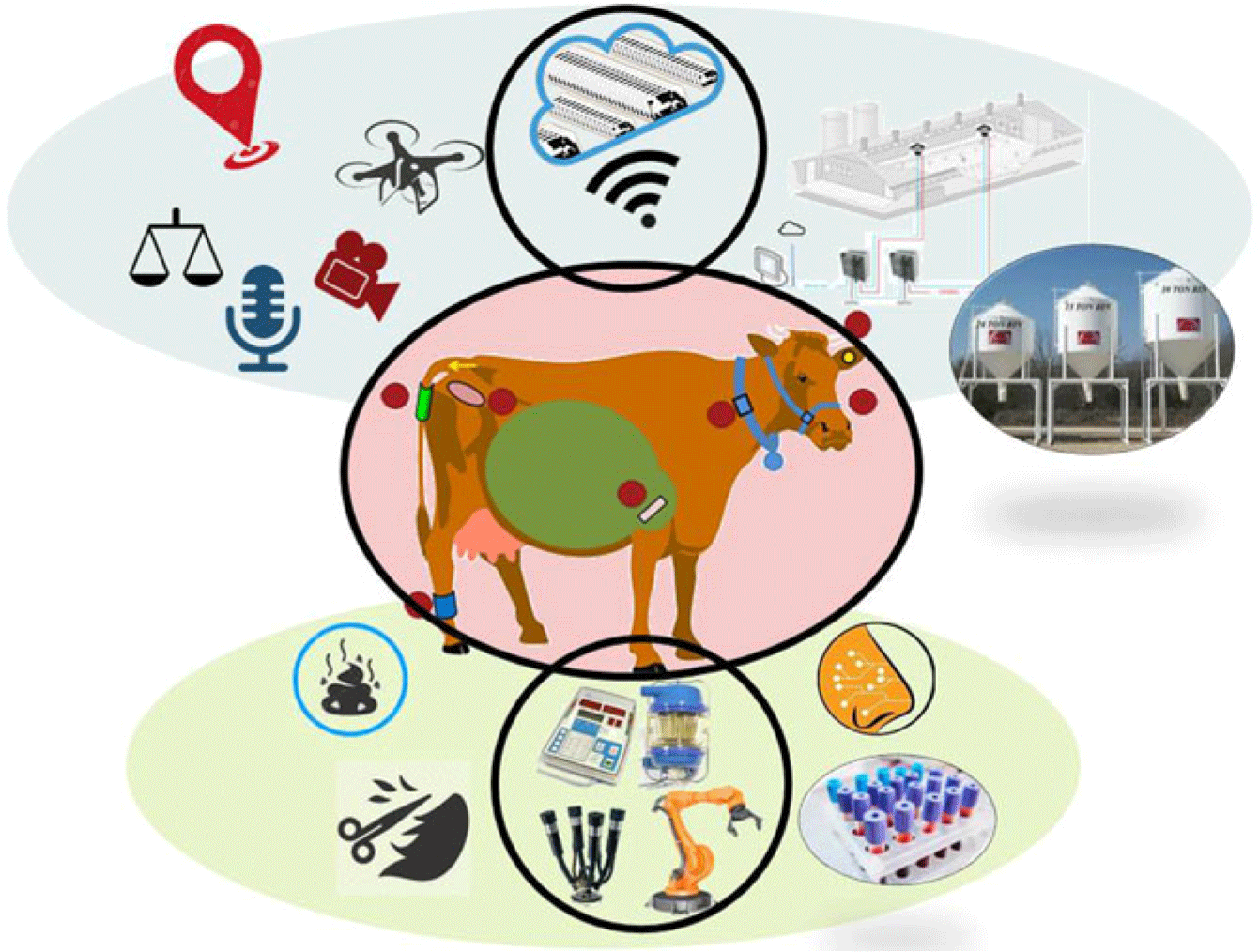
Figure 1 Overview of sensor technologies associated with dairy animals (example is dairy cow but others could apply equally well). The red zone and individual red dots show ‘At Cow’ sensors, chiefly accelerometer based but also in some cases including temperature, heart rate and pH analysis. The blue zone shows ‘Near Cow’ sensors such as video and sound analysis, climate analysis, feed analysis, GPS and interaction with the cloud (the latter two classified as ‘near’ on the basis of enabling real-time analysis). The green zone shows ‘From Cow’ sensors that monitor products coming from the cow (milk, hair, saliva, sweat, nasal secretion, breath, faeces, etc.). The black circles represent the main technologies that are commercially available and in widespread use. A cow is shown as example; many of the technologies are also applicable to other dairy ruminants. The figure is not exhaustive.
I include the potential for robotic collection of biomarker samples in the third category. To the best of my knowledge, this possibility has not yet been considered even at a research level, which leads us to consider which of these sensor technologies are not only commercially available but also in actual widespread use. These are represented by the black circles in Figure 1 and comprise numerous At Cow sensors (very largely accelerometers and/or temperature sensors) that in most cases communicate with cloud-based data management, together with online milk analysis. Automatic milking systems should also be mentioned as a non-sensor technology that has seen widespread adoption (Svennersten-Sjaunja and Pettersson, Reference Svennersten-Sjaunja and Pettersson2008). A comprehensive list of sensor technologies that were available in 2016 is available in tabular form in Caja et al. (Reference Caja, Castro-Costa and Knight2016). Other technologies are commercially available, such as image-based assessment of body condition (Krukowski, Reference Krukowski2009) and udder disease conditions (Castro-Costa et al., Reference Castro-Costa, Caja, Salama, Rovai, Flores and Aguiló2014), force plates for automated BW recording (Dickinson et al., Reference Dickinson, Morton, Beggs, Anderson, Pyman, Mansell and Blackwood2013) and limb disease conditions (Ghotoorlar et al., Reference Ghotoorlar, Ghamsari, Nowrouzian, Ghotoorlar and Ghidary2012) and sensors for monitoring rumen pH and informing on rumen dysfunction (Sato et al., Reference Sato, Mizuguchi, Ito, Ikuta, Kimura and Okada2012). I should add that this list is probably not comprehensive, the references refer to the basic technology rather than necessarily the commercial product and in some cases the scientific case for the technology or product is not established. Further consideration and comparison of a ‘successful adoption’ (estrous detection) and a ‘failed adoption’ (automated weighing) is given by Maltz (Reference Maltz2020). The rumen pH bolus is an interesting case in point. The technology has been available for some years, as have commercial products. However, the sensor is expensive and suffers from a short life due to a combination of the acidic conditions in the rumen and heavy power requirement. As a result, few (if any) farmers have invested directly in such sensors, which are used primarily for research (see companion paper by Dijkstra et al.) or by feed companies or veterinarians offering a service to farmers who have a herd-level nutritional problem. Many more technologies are in research development, such as image-based assessment of individual feeding behaviour (Bloch et al., Reference Bloch, Levit and Halachmi2019), rumen function (Song et al., Reference Song, van der Tol, Groot Koerkamp and Bokkers2019) and heart and respiratory rates (Beiderman et al., Reference Beiderman, Halachmi and Zalevsky2014). Totally, novel sensor modalities under development include laser reflection-based LiDAR for body composition (Huang et al., Reference Huang, Li, Zhu, Fan, Zhang and Wang2018) and micro-Doppler radar for heart and respiratory rate (Michie et al., Reference Michie, Andonovic, Davison, Tachtatzis, Hamilton, Gilroy and Johnsson2020).
Estrous detection
Technologies that require specific mention are RFID and tri-axial accelerometry since these are at the heart of estrous detection. Both have been comprehensively reviewed by Caja et al. (Reference Caja, Castro-Costa and Knight2016). Radiofrequency identity technologies include large high-frequency transponders (e.g., neck collars) that also enable collection and transmission of large data packages as well as the cheaper miniaturised low-frequency devices (ear tags, injectable devices or rumen boluses) that are purely for identity. Both types of transponder are ‘passive’ (i.e., power resides with the receiving element) and the two types are not inter-compatible. Radiofrequency identity technology is optional for most EU dairy cattle farmers, but compulsory for the larger national populations (>600 000 head) of small ruminants as the only way to trace and to build up credible animal inventories.
Tri-axial accelerometers are small, cheap and robust. In addition to estrous detection in cattle, they form the basis of the activity trackers that many of us carry or wear. Clearly, a device that measures changes in motion in three axes is not capable of detecting the main physiological indicators of estrous (pheromones) as a bull would. Furthermore, it is not activity per se that identifies the onset of estrous, rather, it is the change in activity of the individual animal from day to day. Trying to identify which of, say, 200 cows in a herd were in estrous on a specific day using only that day’s data would give very unreliable results. This is a crucial observation that will underpin our later discussion of sensor technology use for wellbeing evaluation; technology is likely to be very much better at identifying change within a cow across time than variation between cows within a herd or between herds (‘benchmarking’) at a single point in time. Accelerometers may be located at various points on the animal (rear ankle, neck, ear, tailhead) as well as in the rumen. All are capable of detecting estrous and some can do more than that, especially if combined with a second sensor modality. Examples are a gyroscope that provides positional information to allow easier detection of lying/standing time, and temperature thermistors that enable calving detection when expelled from the vagina or, as we shall see later, information on rumen function and general health as part of a bolus sensor. For a detailed review of estrous detection using sensors, see Mottram (Reference Mottram2016).
Biomarkers
The third category of sensors identified in Figure 1 comprises biomarkers of one sort or another. In current commercial use, this relates exclusively to milk analysis. Milk can yield important information relating not just to downstream consumer properties (i.e., product quality) but also upstream animal status (Duplessis et al., Reference Duplessis, Santschi, Plante, Bergeron, Lefebvre, Durocher and Cue2019). Systems are available for milk composition (relevant to metabolic status: Leitner et al., Reference Leitner, Merin, Lemberskiy-Kuzin, Bezman and Katz2012; Larsen et al., Reference Larsen, Alstrup and Weisbjerg2016), somatic cell count and conductivity (relevant to udder disease status; Albrechtsen et al., Reference Albrechtsen, Duse, Bennedsgaard, Klaas, Hogeveen and Lam2011) and, in one system, biomarkers of reproductive (Bruinjé et al., Reference Bruinjé, Colazo, Ribeiro, Gobikrushanth and Ambrose2019) and metabolic function. In contrast to this rather restricted commercial usage, the amount of research effort directed to biomarkers of one sort or another is enormous, and beyond the scope of this position paper. Fortunately, the field has been reviewed very recently by de Almeida et al. (Reference de Almeida, Zachut, Hernandez-Castellano, Šperanda, Gabai and Mobasheri2019) in relation to health and welfare and by Zachut et al. (Reference Zachut, Speranda, de Almeida, Gabai, Mobasheri and Hernandez-Castellano2020) in relation to fertility and metabolic regulation. Biomarkers show great promise, but a word of caution is required. The physiological principle underpinning the use of milk conductivity for mastitis detection was first shown in the mid-70s (Linzell and Peaker, Reference Linzell and Peaker1975), and it took 30 years or more for the principle to be put into commercial practice and some studies still question the validity of the approach (Khatun et al., Reference Khatun, Bruckmaier, Thomson, House and García2019).
Sensors for wellbeing
Several of the estrous detection manufacturers now also offer systems (usually add-on modules) that identify (or claim to identify) cows that are at risk of health or welfare problems of one sort or another: statements like ‘your herd’s health and well-being, at your fingertips’ and ‘effective animal health monitoring’ are easy to find on commercial Internet sites. Principle amongst these are mastitis, lameness and metabolic disease detection, and it is probably no coincidence that these are the major health problems afflicting dairy cows. How realistic are the claims? From a scientific point of view, there has been little or no independent validation of which I am aware. Is that necessarily a problem? Consider the case of mastitis. Automatic milking systems is a technology that has been around for some time, and since there is no dairyman to detect udder abnormalities during the milking process, AMS requires a technological approach to mastitis detection. Sensor modalities in use include somatic cell counting (SCC), lactate dehydrogenase concentration and milk conductivity. We have just mentioned scientific concerns about some aspects of this (Khatun et al., Reference Khatun, Bruckmaier, Thomson, House and García2019) but is there any industry-level indication of significant problems? Frössling et al. (Reference Frössling, Ohlson and Hallén-Sandgren2017) documented AMS uptake in Sweden between 2008 and 2011 and showed that AMS was associated with increased mastitis risk (elevated SCC), but that this effect decreased over time and was less important than other factors such as time of year. In Sweden as a whole, AMS use increased but recorded mastitis incidence decreased over the period, which could, of course, reflect either improved udder health or detection failure. In short, the situation is complicated but for this particular example, there is no real evidence of a growing problem. In relation to animal wellbeing as a whole, is this maintenance of status quo satisfactory? Not in my view! Two of the major factors that potentially decrease wellbeing are higher milk yields and larger farms. The first of these has been recognised for many years and is not straightforward (Knight et al., Reference Knight, Alamer, Sorensen, Nevison, Flint and Vernon2004), but it is the second where technology can have real impact, and it should be to improve, rather than to avoid deterioration. The problem is simple. Economics drives the move towards bigger herds and more animals per dairyman (see discussion in Caja et al., Reference Caja, Castro-Costa and Knight2016), so surveillance becomes more difficult. The concept that technology could redress the balance by providing a precise diagnosis of a specific problem in a specific animal at a specific time is attractive, but almost certainly unrealistic. Mastitis detection systems are set to identify cows that may have a problem using algorithms that attempt to balance false positives and false negatives in an optimal way; the final decision that a cow does/does not need treatment is made by a dairyman. The crux is to develop systems that constantly monitor simple (but meaningful) wellbeing-related parameters, looking for change indicative of a suboptimal state (‘constantly’ will be qualified later and should not be confused with ‘frequently’). Lying time is a measurable trait that is almost certainly related to lameness (Maselyne et al., Reference Maselyne, Pastell, Thomsen, Thorup, Hänninen, Vangeyte, Van Nuffel and Munksgaard2017) and is, again, almost certainly how at least one commercial sensor ‘detects’ lameness. The problem is that lying time is also affected by many other factors, so the same false-positive/negative issues apply, and it will probably be several years before the true efficacy of lameness detection systems can be established. Other simple indicators of wellbeing that can be measured using today’s state of the art are feeding, rumination and drinking, and in the next section we will see how this is done and how it could be useful in the future.
Beyond state of the art
The premise of this position paper is that sensor technologies could revolutionise global dairy farming in a positive way, and Figure 2 summarises how that might be achieved. The first priority is to identify an optimistic but achievable target, here defined as Optimized Decision Support. The reasons for this target are twofold:
the focus is on improving overall husbandry, rather than ‘solving’ specific disease problems
it is clear that the technology supports the farmer, and not vice versa.

Figure 2 Beyond state of the art: schematic of research and development routes to optimised sensor-based decision support for dairy animal husbandry. The red shaded routes represent the likely minimum effort required to achieve the objective. R&D = research and development; IOT: Internet of things.
The second priority is to recognise the need for coordinated, integrated and synergistic research and development (R&D) effort from a spectrum of essential disciplines that include a range of animal-related sciences together with an equally broad range of technology-related sciences. This was a major consideration in a recently completed COST Action in technology for dairy animal health and welfare, FA1308 DairyCare (DairyCare, 2019). Sadly, such integration does not always happen: animal scientists met recently at the Ghent Conference of the European Association of Animal Production to discuss (amongst other things) PLF, at exactly the same time that engineers were meeting in Cork to discuss the same issues in the European Conference on PLF.
There are a number of potential R&D routes from current state of the art towards the target which coalesce into two common elements, a need for data integration (considered below) and then a business model for implementation (considered later). Is there a need for new sensors to extend the state of the art? Is there a need for more of the sensors that we already have? I take the view that neither are essential, but both will happen and both are likely to speed progress. On the issue of new sensors, there is a need to work backwards from a target to identify how technology can achieve that target, rather than forwards from a technical possibility to a now redefined target that may not be really what is needed. The example of rumen bolus sensors is relevant. A major manufacturer of rumen pH boluses is currently testing the market for additional biochemical functionalities (ammonium and nitrate) to be added to their sensor. Whilst these may have relevance to researchers investigating nitrogen cycling, the relevance to farmers is difficult to see and the sensor is likely to suffer the same limited lifetime issues as the existing pH sensor. Those with knowledge of physiological rumen function will know that rumenal muscular contractions of three distinct types mix digesta, regurgitate it for rumination and remove methane by eructation. It is our belief that a pressure sensor could record these different contractions and therefore give farmer-relevant information on animal wellbeing (target: impaired rumen function is a ‘first stop’ for veterinary diagnosis) as well as carbon footprint (target: evidence-based low carbon footprint has potential economic value), and with funding from the Hannah Dairy Research Foundation and as a collaboration between Spanish animal scientists and Scottish engineers, we hope to develop such a sensor (Castro-Costa et al., Reference Castro-Costa, Caja, Michie, Andonovic and Knight2019).
Adding more and more of the same sensors to the global dairy sector takes us into the realms of ‘big data’ and ‘the Internet of Things, IoT’ (reviewed by Michie et al., Reference Michie, Andonovic, Davison, Tachtatzis, Hamilton, Gilroy and Johnsson2020). Internet of Things is the concept that ‘things’ (people or animals but also pieces of equipment, vehicles, sensors, etc.) can be connected through local communication wireless networks and the Internet (cloud communication). If large amounts of data are linked together in this way, it potentially becomes something referred to as big data and may accrue increased value. Do current generation dairy animal sensors produce big data? Emphatically no! The size of big data is generally accepted to be in the zetabyte range (zeta is 1021 so a zetabyte is a kilobyte raised to the seventh power) approximating to data from 6bn smartphones. Additional criteria apply; the data should have variety, be obtained in real time or approaching it and be fit for purpose (the four Vs: Volume, Variety, Velocity and Veracity). Accelerometer sensors, for example, can potentially collect very large amounts of data, but the amount that they communicate (transmit to a receiver and thereafter the IoT) is constrained by battery power. To get around this problem, the data are processed locally by the sensor device using algorithms that identify summary features relevant to the trait of interest, and it is this data that is then sent onwards. So the sensor may be sensing constantly, but for practical reasons the measurements are packaged into summaries covering discrete windows of time, corresponding to observation frequency. A typical estrous detection sensor will function effectively sending just 1 or 2 kilobytes of data daily! (Michie et al., Reference Michie, Andonovic, Davison, Tachtatzis, Hamilton, Gilroy and Johnsson2020). In short, big data is a misconception, but on the other hand all of the commercially available sensors are IoT enabled, and in one specific case the manufacturer is heavily targeting this aspect and offers a product that is built around integrating data from very many customers globally into an artificial intelligence (AI) system (Connecterra, 2019).
In current practice, although manufacturers claim to offer ‘complete solutions’, no one sensor system individually offers everything that could be achieved using a full combination of all systems operating together, so for maximum benefit the farmer’s only course of action is to invest in more than one system, shown as ‘Multiple Sensors’ in Figure 2. Furthermore, almost without exception, the different technologies operate ‘stand alone’ and will not communicate with each other, limiting the usefulness of the IoT. However, there may be circumstances in which multiple sensors do achieve a specific objective. Some smartwatch devices produced for golfers employ an accelerometer to detect shots, which works well for drives and fairway shots, but not for putts. Although the device could probably be made more sensitive to overcome this problem, this would introduce another problem, namely false positives. The solution adopted by the manufacturer is to supply an additional accelerometer that attaches directly to the putter, detects the shot and communicates it to the smartwatch. I am not aware of a similar approach being taken by dairy sensor manufacturers, but it could be done.
We have previously mentioned the ‘Combined Sensor’ in relation to, for instance, temperature and activity in a single rumen bolus. This approach will doubtless be repeated, and I would suggest that a single rumen bolus could potentially include sensors detecting activity, temperature, pressure and heartbeat.
Data mining is an increasingly familiar concept in many scientific fields and can be defined simplistically as ‘the process of discovering patterns in large data sets’. The ‘cocktail party effect’ is a good example of what is happening: in a crowded and noisy room your attention is defocused from the background ‘babble’ (which your brain filters out) and focused onto a specific word or words, such as your own name. Another example is the processing of collected sensor data that is done by the device before transmission, where the raw activity data (for an accelerometer) are filtered to identify a ‘signature’ left by the step that the animal has just taken (or the golf shot just made). Steps proved to be relatively easy to find, and data mining algorithms have now advanced to the point where signatures for eating and ruminating can also be identified from several of the commercial accelerometer-based sensors (Michie et al., Reference Michie, Andonovic, Davison, Tachtatzis, Hamilton, Gilroy and Johnsson2020). Another interesting example is rumen temperature. It quickly became obvious that temperature would fall every time that the cow drank, and this signature was crystal clear even to the naked eye. So, wellbeing indicators including feeding, rumination and drinking are all already available.
Beyond data mining employing a ‘static’ algorithm, AI or Machine Learning constantly moves the goalposts, in other words, as more and more data arrive the algorithm automatically adapts or ‘learns’. Cognitive computing is another term for this phenomenon, the goal being to simulate human thought processes in a computerised model. Artificial intelligence has arrived for dairy husbandry management! The firm marketing it is Dutch and their approach is built around existing sensor technology coming from many, many cows around the globe but with the additional input of the farmer adding a simple assessment of what the data mean to him, based on prompts from the system. As the database grows, the system will progressively get better and better at interpreting the data that sensors are generating. This is a long-term prospect; in the shorter term variation in how the farmers assess the data will likely limit gains made in the final interpretation.
Data integration is the next essential stage of the process, and is an area in which a lot could be achieved quickly, were it not for commercial sensitivities that restrict data sharing. All of the commercial systems do already integrate and compare data, in particular that coming from the same individual animal on consecutive days. The principle is simple; the system compares the cow’s current status with her previous status and then with the desired status for an animal at this stage of the lactation cycle, and if these values do not match an alert is raised. Comparison with the rest of the herd is also important; reduced water intake in one cow indicates a possible health problem in that cow, but if it is replicated across the herd it is a supply problem. It is integration of data coming from different sensors manufactured by different companies that is the problem: for understandable reasons, there is often a reluctance to allow access. In addition to these commercial factors, combining and integrating data from a number of sensors is fraught with difficulty, not least being the ‘synchronize watches’ issue. The data sent by a sensor inevitably includes a time stamp, and when different sensors are all sending, it is essential that the time stamps are exactly coordinated.
A variation of this data integration approach was described by Caja et al. (Reference Caja, Castro-Costa and Knight2016), which they called Progressive Integration. The principle is simple. A sensor (proposed as a multifunction rumen bolus, but it need not be) interrogates the cow constantly, and whilst her status remains within predefined ‘normal’ limits she is classified ‘green’. If a potential problem is detected the system internally changes her status to ‘amber’, triggering additional attention from the system (not, at this stage, from the farmer). The example used was that she might now be followed by a drone that was monitoring her interactions with other animals and her environment. If this additional level of attention also raises questions about her status, she moves to ‘red’ and next time that she is milked, shedding gates automatically divert her into the holding area for cows requiring attention from the farmer or veterinarian. To assist their decision, a robotic arm would have taken samples of saliva (for instance) for diagnostic biomarker analysis whilst she was being milked.
Business models
Development of serviceable sensor systems is one thing, commercial adoption is quite another. Unless farmers are persuaded of the benefits they will not invest, and whilst RFID has become a legal requirement in some EU countries, it seems unlikely that legislators will ever go as far as to require the use of husbandry-related sensor systems. Wellbeing-related sensor data could add value to the primary product, but historically it has usually been processors and retailers that have exploited added value, not farmers, and there is little reason to believe that this will change. What is needed, therefore, is a route to market, or business model. A major outcome from the DairyCare COST Action will be a ‘blueprint for action’ (Knight, Reference Knight2020). This will include a novel proposal for how the provision of sensor technologies to dairy farmers might be structured in the future, shown schematically in Figure 3. The commercial sensitivity of data has already been mentioned, but there is also a related issue of data ownership. This arises from the recognition that data have value. To the farmer, the immediate value is the improvement in animal health and performance, and hence profitability. He may also perceive an additional potential value whereby the data are provided to others who would have an interest in it, such as feed and genetics companies, processors, retailers and even the consumer. This is an understandable attitude to take, but a number of pitfalls can be seen. Firstly, the manufacturers of the sensors may well feel that they have a claim to the data. Secondly, whilst many farmers are very business conscious, they would not necessarily have the relevant skill sets or time to fully exploit the value of the data. Thirdly, this approach leaves the farmer in the position of having to invest capital sums in every piece of sensor equipment that may be valuable to him and, furthermore, to reinvest on a regular basis as upgrades become available. Fourthly, the maintenance costs associated with the technologies would fall on the farmer. Fifthly, the costs that will need to be borne by the farmer are rather difficult to predict such that budget and cash flow become a problem. Finally, the farmer has total responsibility for collecting and understanding the data which, as we have said before, may be coming from a number of different systems. With these points in mind, the DairyCare business model deliberately takes a service provider approach, whereby the technologies are never owned by the farmer, but by a service provider. The farmer enters into a contract with the service provider knowing when and how much he will pay. The service provider installs and maintains the technologies that are most relevant for that farmer, purchasing them from a number of different technology developers if necessary. The data are collected by the service provider who becomes responsible for the data integration and decision support steps identified in Figure 2. From the farmer’s point of view, the decision support is exactly that: he does not need to worry about anything other than giving his full attention to those cows that have been identified by the system as being most at need. This relationship between farmer and service provider is the inner loop identified in Figure 3. Given that data do have value, the service provider will be the one to exploit this value by appropriate interaction with the other players identified in the figure, and the potential returns will be reflected in the contract price agreed with the farmer. The further advantage of this sort of approach is that it overcomes the problem that technological approaches might only be affordable, or apply to, the largest farms. In this scenario, a cluster of say, 20 farms of 250 head all instrumented by the same service provider would in effect be equivalent to a single farm of 5000. The nature of the service provider is flexible. It could simply be a commercial company, but equally it could be a farmer cooperative or a national agency such as a breed improvement service.

Figure 3 A potential data-based business model for how sensor-based husbandry support might be introduced to a dairy cow farm (reproduced with permission from Knight, Reference Knight2020). Vet = veterinarian.
Conclusions
The estimated global value of animal biosensor technologies is expected to exceed $20 billion by 2020 (Neethirajan et al., Reference Neethirajan, Huang, Tuteja and Kelton2017) and a large share of that will be in dairy, so it is true to say that sensor systems for dairy husbandry have come a long way in a relatively short time. Since the only market to have been rigorously exploited to date is estrous detection, it is equally true to say that they are still in their infancy in comparison to what could become possible in the future. There is no reason why sensors systems could not find widespread use for health and welfare monitoring, and to do that not just in dairy cows but in other dairy species as well. Technologies capable of measuring relevant parameters such as eating and drinking already exist, and the ability to extract relevant information from complex datasets is growing all the time. The need for technologies that will assist husbandry is growing as dairy farms expand in size, and the potential for wellbeing data to add value to the primary product is there to be exploited. Success will require coordinated effort from a spectrum of biologists, engineers and business experts in areas of R&D and marketing that are identifiable. There is a potential for sensor systems to benefit not just the farmer and their animals, but also other players in the dairy foods chain as well as consumers. Indeed, it is probably not an overstatement to say that sensor-based husbandry support could be a key element of the dairy sector’s vital future contribution to global food security.
Acknowledgements
This article is based upon work from COST Action FA1308 DairyCare, supported by COST (European Cooperation in Science and Technology, www.cost.eu). COST is a funding agency for research and innovation networks. COST Actions help connect research initiatives across Europe and enable scientists to grow their ideas by sharing them with their peers. This boosts their research, career and innovation.
C. H. Knight 0000-0001-9361-2249
Declaration of interest
The author does not receive any commercial or other financial or in-kind support related to the subject matter of this article and therefore declares no conflict of interest.
Ethics statement
Not applicable. No original data or experimentation is presented in this article.
Software and data repository resources
Not applicable. No original data or experimentation is presented in this article.