315 results
7 - Computational Approaches to Language
-
- Book:
- The Philosophy of Theoretical Linguistics
- Published online:
- 25 April 2024
- Print publication:
- 02 May 2024, pp 157-182
-
- Chapter
- Export citation
Extreme heat wave sampling and prediction with analog Markov chain and comparisons with deep learning
-
- Journal:
- Environmental Data Science / Volume 3 / 2024
- Published online by Cambridge University Press:
- 27 March 2024, e9
-
- Article
-
- You have access
- Open access
- HTML
- Export citation
Chapter 3 - Worlds Otherwise
- from Part II - Probable Realisms
-
- Book:
- The Art of Uncertainty
- Published online:
- 29 February 2024
- Print publication:
- 07 March 2024, pp 109-151
-
- Chapter
- Export citation
Models of mild cognitive deficits in risk assessment in early psychosis
-
- Journal:
- Psychological Medicine , First View
- Published online by Cambridge University Press:
- 04 March 2024, pp. 1-12
-
- Article
- Export citation
5 - Genetic Testing for Suicide Risk Assessment
-
- Book:
- Practical Ethics in Suicide
- Published online:
- 15 February 2024
- Print publication:
- 22 February 2024, pp 82-101
-
- Chapter
- Export citation
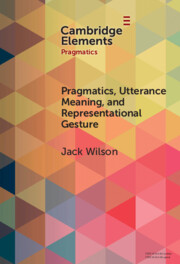
Pragmatics, Utterance Meaning, and Representational Gesture
-
- Published online:
- 02 February 2024
- Print publication:
- 29 February 2024
-
- Element
- Export citation
Application of ultrasound multimodal imaging in the prediction of cervical tuberculous lymphadenitis rupture
-
- Journal:
- Epidemiology & Infection / Volume 152 / 2024
- Published online by Cambridge University Press:
- 30 January 2024, e28
-
- Article
-
- You have access
- Open access
- HTML
- Export citation
7 - Beyond Disciplinary Prediction
-
- Book:
- The Time of Global Politics
- Published online:
- 23 November 2023
- Print publication:
- 07 December 2023, pp 198-233
-
- Chapter
- Export citation
Changing trends in the global burden of mental disorders from 1990 to 2019 and predicted levels in 25 years
-
- Journal:
- Epidemiology and Psychiatric Sciences / Volume 32 / 2023
- Published online by Cambridge University Press:
- 07 November 2023, e63
-
- Article
-
- You have access
- Open access
- HTML
- Export citation
Are predictions of bovine tuberculosis-infected herds unbiased and precise?
-
- Journal:
- Epidemiology & Infection / Volume 151 / 2023
- Published online by Cambridge University Press:
- 20 September 2023, e165
-
- Article
-
- You have access
- Open access
- HTML
- Export citation
Chapter 7 - Observational Research: Case-Control and Cohort Designs
-
- Book:
- Research Design in Clinical Psychology
- Published online:
- 25 August 2023
- Print publication:
- 07 September 2023, pp 237-278
-
- Chapter
- Export citation
Developmental pathway for first onset of depressive disorders in females: from adolescence to emerging adulthood
-
- Journal:
- Psychological Medicine / Volume 54 / Issue 4 / March 2024
- Published online by Cambridge University Press:
- 29 August 2023, pp. 753-762
-
- Article
- Export citation
The aerodynamic force estimation of a swept-wing UAV using ANFIS based on metaheuristic algorithms
-
- Journal:
- The Aeronautical Journal / Volume 128 / Issue 1322 / April 2024
- Published online by Cambridge University Press:
- 23 August 2023, pp. 739-755
-
- Article
- Export citation
Predicting dairy herd resilience on farms with conventional milking systems
-
- Journal:
- Journal of Dairy Research / Volume 90 / Issue 3 / August 2023
- Published online by Cambridge University Press:
- 11 September 2023, pp. 273-279
- Print publication:
- August 2023
-
- Article
-
- You have access
- Open access
- HTML
- Export citation
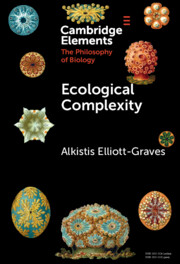
Ecological Complexity
-
- Published online:
- 20 July 2023
- Print publication:
- 10 August 2023
-
- Element
-
- You have access
- HTML
- Export citation
Are markets more accurate than polls? The surprising informational value of “just asking” – CORRIGENDUM
-
- Journal:
- Judgment and Decision Making / Volume 18 / 2023
- Published online by Cambridge University Press:
- 30 June 2023, e21
-
- Article
-
- You have access
- Open access
- HTML
- Export citation
Predicting antipsychotic-induced weight gain in first episode psychosis – A field-wide systematic review and meta-analysis of non-genetic prognostic factors
-
- Journal:
- European Psychiatry / Volume 66 / Issue 1 / 2023
- Published online by Cambridge University Press:
- 06 June 2023, e42
-
- Article
-
- You have access
- Open access
- HTML
- Export citation
Clinical epidemiology and a novel predicting nomogram of central line associated bloodstream infection in burn patients
-
- Journal:
- Epidemiology & Infection / Volume 151 / 2023
- Published online by Cambridge University Press:
- 23 May 2023, e90
-
- Article
-
- You have access
- Open access
- HTML
- Export citation
4 - Telling the Boiling Frog What He Needs to Know
- from Part I - Science
-
- Book:
- Five Times Faster
- Published online:
- 19 March 2023
- Print publication:
- 06 April 2023, pp 28-44
-
- Chapter
- Export citation