Introduction
Adolescence and young adulthood are periods marked by significant changes in youths’ lives, and many youth start to experiment with substances (Arnett, Reference Arnett2000). Despite some decreases in recent years, many adolescents experiment with tobacco, alcohol, or cannabis, with substantial percentages progressing into regular use. Worldwide, about 15% of individuals below age 18 years smoke (WHO, 2017). Over a quarter of adolescents aged 15–19 years drink alcohol, and almost half of those engage in heavy episodic drinking (WHO, 2018). The annual prevalence of cannabis use among youths aged 15–16 years is 14% in Europe and 12% in the Americas (UNODC, 2018). To some degree, these increases in substance use during adolescence and young adulthood can be understood as part of normal development, in which young people want to obtain a wide range of experiences before acquiring adult norms and behaviors (Arnett, Reference Arnett2000). However, among users of tobacco, alcohol, and cannabis, the chances of developing dependence may be as high as 67%, 23%, and 9%, respectively (Lopez-Quintero et al., Reference Lopez-Quintero, Cobos, Hasin, Okuda, Wang, Grant and Blanco2011). Serious (mental) health risks have been associated with long-term use of these substances (Hall et al., Reference Hall, Patton, Stockings, Weier, Lynskey, Morley and Degenhardt2016) and the associated disease burden is substantial (Degenhardt et al., Reference Degenhardt, Whiteford, Ferrari, Baxter, Charlson, Hall and Vos2013; Ezzati, Lopez, Rodgers, Vander Hoorn, & Murray, Reference Ezzati, Lopez, Rodgers, Vander Hoorn and Murray2002).
When trying to understand the emergence of substance use behavior among young adults, research has proposed a dynamic cascade developmental model (Dodge et al., Reference Dodge, Malone, Lansford, Miller, Pettit and Bates2009). This model proposes that adolescent substance use develops through a complex of child and environmental factors that influence each other over the course of development. Important environmental risk factors that play a role during adolescence concern parental factors. Parents are significant role models for their children, and parental modeling of substance use predicts adolescent substance involvement (Li, Pentz, & Chou, Reference Li, Pentz and Chou2002). Adolescence is a period marked by increased need for individuation and independence, which is associated with decreases in parental monitoring (Lionetti et al., Reference Lionetti, Palladino, Moses Passini, Casonato, Hamzallari, Ranta and Keijsers2019) as well as with temporary perturbations in parent–child relationships (De Goede, Branje, & Meeus, Reference De Goede, Branje and Meeus2009). Both parental monitoring and the parent–child relationship may predict higher adolescent substance use. For example, having parents that are less inquisitive about the whereabouts of their child (i.e., low parental monitoring) predicts affiliation with deviant peers (Dodge et al., Reference Dodge, Malone, Lansford, Miller, Pettit and Bates2009), which in turn predicts substance use (Rai et al., Reference Rai, Stanton, Wu, Li, Galbraith, Cottrell and Burns2003). Likewise, having parents that know less about the child's activities is associated with adolescent risk behavior, including alcohol use (Waizenhofer, Buchanan, & Jackson-Newsom, Reference Waizenhofer, Buchanan and Jackson-Newsom2004) and smoking (Harakeh, Scholte, Vermulst, de Vries, & Engels, Reference Harakeh, Scholte, Vermulst, de Vries and Engels2004). The parent–child relationship might directly and indirectly influence adolescents’ substance use. One study reported that high parental support was related to lower adolescent substance use, and that this relationship was mediated by cognitive self-control (Wills, Resko, Ainette, & Mendoza, Reference Wills, Resko, Ainette and Mendoza2004). In addition, a low-quality parent–child relationship has been associated with cannabis use (Creemers et al., Reference Creemers, Harakeh, Dick, Meyers, Vollebergh, Ormel and Huizink2011), smoking, and alcohol use (Simons-Morton, Haynie, Crump, Eitel, & Saylor, Reference Simons-Morton, Haynie, Crump, Eitel and Saylor2001; Visser, de Winter, & Reijneveld, Reference Visser, de Winter and Reijneveld2012).
There is evidence that if such parental risk factors are operating during adolescence, their effects on substance use can last well into young adulthood (for reviews, see Ryan, Jorm, & Lubman, Reference Ryan, Jorm and Lubman2010; Stone, Becker, Huber, & Catalano, Reference Stone, Becker, Huber and Catalano2012). As an example, one study found that low parental monitoring, warmth, and high parental alcohol use in adolescence predicted binge drinking in early adulthood, 7 years later (Donaldson, Handren, & Crano, Reference Donaldson, Handren and Crano2016). Many mechanisms seem to underlie such longitudinal associations. Parental warmth and monitoring have been found to prospectively influence substance use norms and beliefs, as well as increase self-regulation skills and decrease susceptibility to peer influence (Baker & Hoerger, Reference Baker and Hoerger2012; Lac, Alvaro, Crano, & Siegel, Reference Lac, Alvaro, Crano and Siegel2009; Ryan et al., Reference Ryan, Jorm and Lubman2010; Van Ryzin, Fosco, & Dishion, Reference Van Ryzin, Fosco and Dishion2012; Yang, Schaninger, & Laroche, Reference Yang, Schaninger and Laroche2013). As another example, exposure to parental alcohol use prospectively predicted more positive expectancies and attitudes toward alcohol (Smit, Voogt, Otten, Kleinjan, & Kuntsche, Reference Smit, Voogt, Otten, Kleinjan and Kuntsche2020), and being exposed to smoking in the household predicted lower perceived harm of tobacco a year later, which in turn predicted future smoking initiation (Rodriguez, Romer, & Audrain-McGovern, Reference Rodriguez, Romer and Audrain-McGovern2007).
Genetic vulnerability also plays a role in the aetiology of substance use. Heritability estimates from family studies are moderate to high, with the exact estimate depending on developmental period (with lower estimates for youngsters) and whether the behavior constitutes normative use or abuse/dependence (with higher estimates for the latter; Ducci & Goldman, Reference Ducci and Goldman2012; Hopfer, Crowley, & Hewitt, Reference Hopfer, Crowley and Hewitt2003; Mbarek et al., Reference Mbarek, Milaneschi, Fedko, Hottenga, de Moor, Jansen and Vink2015; Verweij et al., Reference Verweij, Zietsch, Lynskey, Medland, Neale, Martin and Vink2010; Vink, Willemsen, & Boomsma, Reference Vink, Willemsen and Boomsma2005). Molecular genetic studies have sought to trace these estimates back to specific genetic variants. Genome-wide association studies (GWAS) have identified many variants of small effect. The variance in a trait explained by all measured genetic variants together (single nucleotide polymorphisms (SNP)-based heritability) is not as high as heritability estimates based on twin research, with the most recent GWAS, for instance, showing a SNP-based heritability of 4% for alcohol use per week, 8% for cigarettes per day (Liu et al., Reference Liu, Jiang, Wedow, Li, Brazel, Chen and Psychiatry2019), and 11% for cannabis initiation (Pasman et al., Reference Pasman, Verweij, Gerring, Stringer, Sanchez-Roige, Treur and Vink2018). Based on GWAS findings, polygenic scores (PGS) can be created to predict genetic risk of substance use in an independent group of individuals. Such scores count and weigh the number of risk alleles from each individual (by their effect estimates from GWAS), creating a personal genetic risk score.
Risk factors interact with each other on multiple levels (Dodge et al., Reference Dodge, Malone, Lansford, Miller, Pettit and Bates2009; Masten, Reference Masten2006). In Gene×Environment interaction (GxE), genetic risk amplifies, diminishes, or even reverses the effect of environmental risk. Although there has been some research into GxE with parent factors in substance use, most have used the (single) candidate–gene method, which has been largely abandoned because most used underpowered designs and findings did not replicate in subsequent GWAS (Border et al., Reference Border, Johnson, Evans, Smolen, Berley, Sullivan and Keller2019; Duncan & Keller, Reference Duncan and Keller2011). Few PGS studies have been conducted to test GxE with parenting factors. One showed that low parental knowledge was more likely to lead to alcohol problems when genetic risk was high (Salvatore et al., Reference Salvatore, Aliev, Edwards, Evans, Macleod, Hickman and Dick2014b). Likewise, a PGS study testing externalizing behavior (including substance use) showed that low parental monitoring predicted externalizing behavior more strongly when genetic risk was high (Salvatore et al., Reference Salvatore, Aliev, Bucholz, Agrawal, Hesselbrock, Hesselbrock and Kramer2014a). Lastly, one study found that parental monitoring (in combination with low peer substance use) buffered for the effect of a smoking cessation PGS on smoking and cannabis use (Musci, Uhl, Maher, & Ialongo, Reference Musci, Uhl, Maher and Ialongo2015). These studies in general seem to align with the differential susceptibility framework, stating that genetic predisposition can amplify or buffer for the effects of adverse environments (Belsky & Pluess, Reference Belsky and Pluess2009). As in the previous example, having both genetic risk for substance use as well as being exposed to some risk-enhancing parenting characteristic leads to a higher risk of substance use than either of these factors alone. This pattern is the one most often found in studies testing GxE in substance use, although there are also many studies that do not detect GxE effects (Pasman, Verweij, & Vink, Reference Pasman, Verweij and Vink2019).
Another form of gene–environment interplay is gene–environment correlation (rGE), where an individual's genetic risk shows a relation to the level of exposure to environmental risk variables. There are different possible sources for rGE, including evocative rGE, where a genetically influenced trait elicits some response from the environment, or active rGE where such a trait influences what kind of environment someone selects for themselves. Passive rGE arises through shared genetic factors between parents and offspring, leading to overlap between the parenting environment and the offspring's genetic make-up. These rGE phenomena could explain why twin studies have traditionally shown significant heritability for parenting and other family environment variables (Deater-Deckard, Fulker, & Plomin, Reference Deater-Deckard, Fulker and Plomin1999; Elkins, McGue, & Iacono, Reference Elkins, McGue and Iacono1997; Jang, Vernon, Livesley, Stein, & Wolf, Reference Jang, Vernon, Livesley, Stein and Wolf2001; Pérusse, Neale, Heath, & Eaves, Reference Pérusse, Neale, Heath and Eaves1994; Plomin, Reiss, Hetherington, & Howe, Reference Plomin, Reiss, Hetherington and Howe1994). Non-twin rGE studies in the context of parenting and substance use are scarce. One study found a genetic factor for substance use to be related to ‘contextual risk” (including family functioning and the parent–child relationship; Hicks et al., Reference Hicks, Johnson, Durbin, Blonigen, Iacono and McGue2013). Another study found that offspring smoking is influenced both by their own as well as their parents’ genetic predisposition, an effect that is likely mediated through modeling of parental smoking (Kong et al., Reference Kong, Thorleifsson, Frigge, Vilhjalmsson, Young, Thorgeirsson and Stefansson2018). rGE effects can make it hard to distinguish the effects from genetic risk factors and the parenting environment. In addition, they can hamper the detection and interpretation of GxE. It has been demonstrated mathematically that rGE can even lead to spurious GxE findings (Dudbridge & Fletcher, Reference Dudbridge and Fletcher2014).
The current study aims to expand knowledge of GxE and rGE mechanisms in the effects of genetic risk and parent environment on substance use, thereby using PGS as measures of genetic risk and incorporating GxE and rGE in a single model to assess their relative contribution. Investigating GxE and rGE is crucial, as these effects can confound the effects of both genetic and environmental factors. For example, if not explicitly modeled, GxE and rGE can present as main effects of G or E in twin research, leading to an overestimation of either effect (Purcell, Reference Purcell2002), and genetic association studies can pick up on environmental signal in the case of rGE (Selzam et al., Reference Selzam, Ritchie, Pingault, Reynolds, O'Reilly and Plomin2019). Disentangling direct, interactive, and correlational mechanisms can provide directions for future intervention studies, for example showing the merits of intervening in parental behavior to prevent genetic vulnerability from coming to expression, or showing which genetic pathways are causally related to substance use independently from environmental confounders. Using prospective data from the TRacking Adolescents’ Individuals Lives Survey (TRAILS), we study the joint effects of genetic risk and different parent factors during adolescence (parental involvement, parental substance use, parent–child relationship quality) on substance use in young adulthood (alcohol use, smoking, cannabis use).
Method
This study's preregistration can be found on Open Science Framework (OSF) (https://osf.io/wv3kb), as well as a section on divergences from the original plan (https://osf.io/ge389/). A description of the analysis scripts can be found in Supplementary Materials 2 (Supplementary Tables S5–S8), and all scripts are published on OSF (https://osf.io/36a7 m/) as well as on GitHub (https://github.com/joellepasman/TRAILS_substanceuse/).
Participants
Data were derived from the ongoing TRAILS, which has been described in detail elsewhere (Oldehinkel et al., Reference Oldehinkel, Rosmalen, Buitelaar, Hoek, Ormel, Raven and Hartman2014). We used data from the first five waves, collected every two years from 2000 to 2013 (population cohort) and 2003–2016 (high-risk cohort). For N = 1,842 (N = 1,354 from the population cohort and N = 498 from the high-risk cohort) adolescents’ genetic samples were available. After genetic quality control and excluding individuals that had no data on parental characteristics, N = 1,645 European-ancestry, unrelated individuals (47.1% female) remained. Average age at Wave 1 was 11.1 years (SD = 0.54, range 10.0–12.6) and at outcome 22.2 years (SD = 0.66, range 20.7–24.1). At Wave 1 (age 11), 84.5% had never or only once drunk alcohol, 94.4% had never or once smoked a cigarette, and 99.6% had never or once used marijuana. Average age at initiation of these substances were M = 14.8, M = 14.8, and M = 16.1, respectively (see Supplementary Table S1).
Genotyping
At Wave 3, blood samples were collected in the adolescents. DNA was isolated and genotyped on a Golden Gate Illumina BeadStation 500 platform and using the HumanCytoSNP-12 BeadChip (Illumina Inc., San Diego, CA, USA). The genotype data (SNPs) were merged, checked for concordance for overlapping SNPs, and imputed against the 1,000 Genomes Project Phase 3 global reference panel. All quality control steps were performed with PLINK v1.07 and v1.9 (Chang et al., Reference Chang, Chow, Tellier, Vattikuti, Purcell and Lee2015; Purcell et al., Reference Purcell, Neale, Todd-Brown, Thomas, Ferreira, Bender and Sham2007). SNPs with a call rate below 95%, a minor allele frequency (MAF) below .05, missingness rates above 5%, and a Hardy–Weinberg disequilibrium p value below 1E−06 were excluded. Individuals with more than 5% missingness on SNP data and individuals from non-European ancestry were filtered out. In order to prevent familial clustering of effects, we excluded one of each pair of family-related individuals (closer than third degree). In order to control for population stratification effects, ten principal components for ancestry were created using multidimensional scaling. Alleles were aligned with 1,000 Genomes, excluding SNPs that had MAFs deviating more than 0.15 from the reference set. Following these cleaning, quality control and selection procedures N = 7,781,794 SNPs and N = 1,645 individuals remained.
Polygenic scores
For the genetic predictor variables, PGS were created. As source GWAS we used the largest studies available to date: from the Liu et al. (Reference Liu, Jiang, Wedow, Li, Brazel, Chen and Psychiatry2019) GWAS we used summary statistics on having smoked on a regular basis (N = 1,232,091), cigarettes per day (N = 337,334), and alcohol consumption in glasses per week (N = 941,280); for cannabis we used summary statistics on lifetime cannabis use from Pasman et al., Reference Pasman, Verweij, Gerring, Stringer, Sanchez-Roige, Treur and Vink2018 (excluding the TRAILS sample, N = 183,539). In order to use information on both smoking initiation and cigarettes per day for the smoking PGS we used multitrait analysis of GWAS (MTAG). MTAG jointly analyzes two or more genetically correlated traits, aggregating their signal and boosting power to detect genetic associations (Turley et al., Reference Turley, Walters, Maghzian, Okbay, Lee, Fontana and Furlotte2018).
PGS are created by summing an individual's risk alleles per locus, weighted by the effect size as found in the source GWAS. However, these weights are not randomly distributed across the genome, due to interdependence between variants (linkage disequilibrium, LD). We used the GCTA-SBLUP tool (Robinson et al., Reference Robinson, Kleinman, Graff, Vinkhuyzen, Couper, Miller and Visscher2017) to adjust the weights for the LD structure within the genome. As reference data for the LD structure we used a random sample of 10,000 European ancestry UK-Biobank participants, selecting a subset of high-quality HapMap 3 SNPs for computational efficiency. We used SNP-based heritability estimates retrieved from the original publications to estimate the model (4% for alcohol use and 11% for cannabis initiation; for the MTAG smoking phenotype we used 8%, which was the estimate for both smoking initiation and cigarettes per day). LD with SNPs more than 1Mb up- or downstream was ignored. In SBLUP it is not necessary to choose arbitrary p value cut-offs or estimate what proportion of the genome should be considered in the PGS (as is necessary using in other methods); rather the whole genome is integrated in the score. In the final step the SBLUP-corrected variant weights were used to create individual-level PGS with the software tool PLINK (Chang et al., Reference Chang, Chow, Tellier, Vattikuti, Purcell and Lee2015).
Measures
Survey items used to measure all nongenetic variables are summarized in Table 1. The earliest measurement point of each variable was included as predictor variable. The parent predictors included measures of parental involvement, consisting of parental monitoring (control, solicitation, and child disclosure) and parental knowledge (Stattin & Kerr, Reference Stattin and Kerr2000). Parental involvement variables were measured at Wave 3 (age 16) and were all based on child-report. Parental substance use was measured at Wave 1 (age 11) using parent-report and included measures of smoking, lifetime cannabis use, and addiction to any substance other than nicotine. Measures of the parent–child relationship at Wave 1 (age 11) included child-reported warmth and rejection (subscales from the EMBU-C, Swedish acronym for “My Memories of Upbringing”; Markus, Reference Markus2003). If for a measure data on both parents were available (this was the case for 97.9% of the parent data), these were averaged. All measures were scored in the direction that we expected would correlate positively with substance use (e.g., positively for parental rejection, negatively for parental warmth; see Table 1).
Table 1. Measures of phenotypical predictors and outcomes (included in the models as observed variables or as indicators of latent variables)
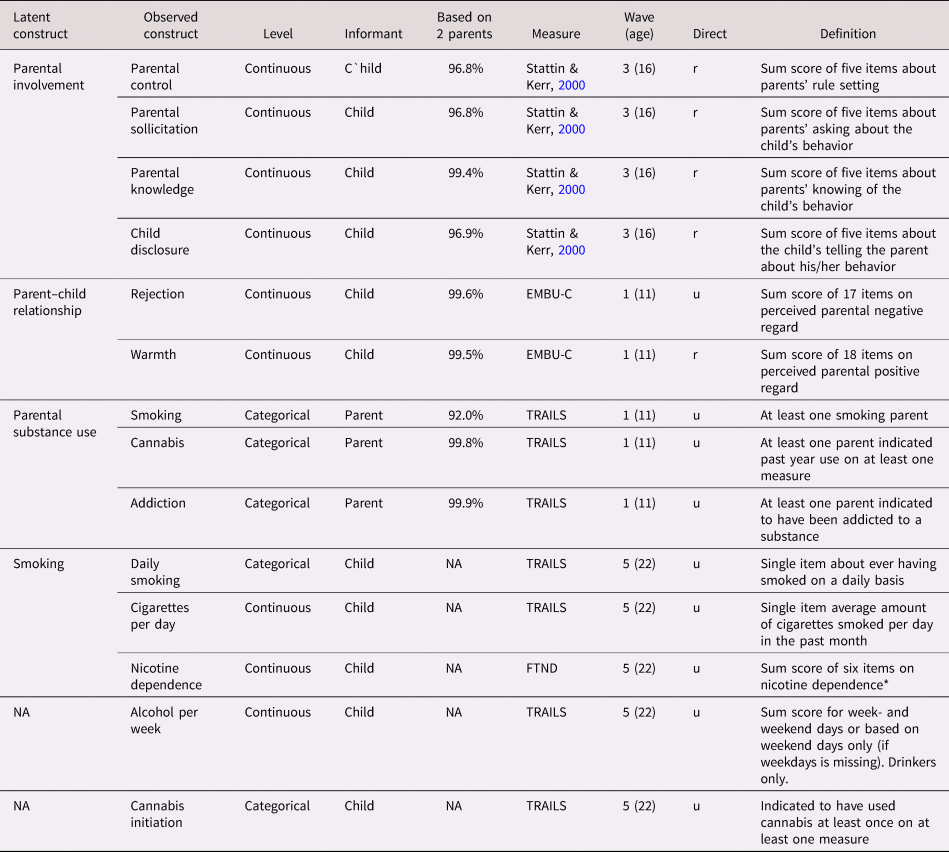
Note: NA = not applicable because the model included the observed (rather than a latent) variable; EMBU-C = Swedish acronym for “My Memories of Upbringing”; TRAILS = TRacking Adolescents’ Individual Lives Survey; FTND = Fagerström Test of Nicotine Dependence. Based on both parents: the proportion of the responses that could be based on reports from or on both the mother and the father. Continuous = Likert response scale analyzed on a continuous scale (i.e., all questions had answering categories).
Direct = direction; all predictors were coded such that it was hypothetically positively related to substance use; “u” (unchanged) indicates the raw scores were used; “r” (reversed) indicates where the scale was reversed
The child's substance use outcomes were measured in young adulthood at Wave 5 (age 22). For smoking, we focused on daily smoking (yes/no), cigarettes per day, and nicotine dependence; for alcohol use we used glasses per week (nondrinkers were excluded); for cannabis, we used cannabis initiation (yes/no). These outcomes were the most similar to the traits measured in the discovery GWAS that were used to create the PGS.
Analyses
We sought to summarize the parent variables within underlying constructs. Using exploratory factor analyses (EFA) in Mplus 8.3 (Muthén & Muthén, Reference Muthén and Muthén1998–2017), it was tested whether the parent variables clustered in the hypothesized latent constructs (parental involvement, parent–child relationship quality, and parental substance use). For the smoking outcomes, we tested whether the three variables clustered in a single smoking factor. With the results from the EFAs, a measurement model was defined, which was used in the structural model.
Using Mplus, we created three separate structural equation models (SEMs) for the three substance use outcomes. We used full information maximum likelihood (FIML) using the maximum likelihood estimator with robust standard errors (MLR) to control for missing data and nonnormality. First, the direct effects of the parental factors and PGS on young-adult substance use were assessed (Model 1, purple arrow in Figure 1). Second, the moderating effects of the PGS (GxE) were added (Model 2, blue arrow). The latent variable interactions between the parent factors and the PGS were computed using the XWITH statement. Significant interactions were followed up with simple slope analysis (Stride, Gardner, Catley, & Thomas, Reference Stride, Gardner, Catley and Thomas2015). Third, the gene-environment correlation pathways were added (rGE), while the moderating effects of the genetic factors were deleted (Model 3, yellow arrow). Note that although these paths are called “correlations,” we modeled them as a directional pathway (one-headed arrow), to investigate the effect of the PGS on parenting and not vice versa. Fourth, the GxE and rGE pathways were included in the same model, to assess their net effects (Model 4). Control variables included age, sex, and ten genetic PCs for ancestry (controlling for genetic similarities arisen because of subgroups of different ancestry within the Dutch population).

Figure 1. The conceptual model of the interplay between genetic and parent factors in the development of substance use, with the blue arrow indicating the Gene×Environment interaction path and the yellow indicating the gene-environment correlation path.
The fit of the four models was determined using commonly used model fit statistics, with acceptable fit defined as root mean square error of approximation (RMSEA) < .08 (MacCallum, Browne, & Sugawara, Reference MacCallum, Browne and Sugawara1996), comparative fit index (CFI) > .90, and Tucker–Lewis Index (TLI) > .90 (Iacobucci, Reference Iacobucci2010). To compare the models, the Akaike information criterion (AIC) and Bayesian information criterion (BIC) were used, which are suitable for comparing nonnested models. AIC and BIC differences of >2 and >10, respectively, are thought to be a strong indication for model fit improvement (in case of a decrease) or deterioration (in case of an increase; Burnham & Anderson, Reference Burnham and Anderson1998; Raftery, Reference Raftery1995). If AIC and BIC disagreed on what was the best fitting model, we prioritized BIC (Nylund, Asparouhov, & Muthén, Reference Nylund, Asparouhov and Muthén2007). In the models including latent variable interactions, and models combining categorical indicators and categorical outcomes, only AIC and BIC, but not CFI, TLI, and RMSEA are computed. Moreover, in models combining categorical indicators with categorical outcomes, CFI, TLI, and RMSEA cannot be computed in Mplus with the MLR estimator. In these models we used the WLSMV (weighted least square mean and variance-adjusted) estimator to compute these fit indices. For individual path parameters we adopted a conventional p value threshold of p < .05. The separate tests for outcomes and parental predictors were not strictly independent and only models with adequate fit parameters were interpreted, foregoing the necessity of stringent correction for multiple testing (Smith & Cribbie, Reference Smith and Cribbie2013).
Results
Parent characteristics were reasonably normally distributed, although parental warmth was high on average, and only few parents reported recent cannabis use or lifetime substance addiction (Table 2). The quarter of the young adults that indicated to ever have smoked, daily smoked eight cigarettes per day on average in the past four weeks and had a low to moderate nicotine dependence score. Participants drank about eight glasses of alcohol per week and almost 60% indicated to have used cannabis. There were high correlations between parent variables and substance use outcomes, and between the PGS and covariates and other traits (Supplementary Table S3).
Table 2. Descriptive statistics for observed variables (before standardization and imputation). For the continuous variables, minimum, maximum, M, and SD are given. For categorical variables the “control’ (reference) group, the “case” group, and the percentage individuals belonging to the “case” group are given

Note: N = sample size before imputation, min = minimum value (for questionnaire scores, the minimum score that was possible to achieve), max = maximum value (for questionnaire scores, the maximum score that was possible to achieve), M = mean, % = percentage for cases, SD = standard deviation, NA = SD for dichotomous variable is not applicable.
* Reported only for current smokers. Cigarettes per day was categorized from 0 = less than 1 cigarettes, 1 = 1–5 cigarettes, 2 = 6–10 cigarettes, 3 = 11–20 cigarettes, 4 = 21–30 cigarettes, and 5 = more than 30 cigarettes.
Measurement model
The exploratory factor analysis of the parent variables showed that the best fitting solution included three factors (see Table 3). The four-factor solution had better fit, but the parsimony and the interpretability of the structure decreased (i.e., there was a factor with only one indicator). We selected the three-factor solution which showed clustering in the hypothesized constructs of parental involvement (indicated by parental control, solicitation, knowledge, and child disclosure), parental substance use (smoking initiation, cannabis initiation, and lifetime addiction), and the parent–child relationship (parental rejection and warmth). We constructed the latent parent–child relationship factor by constraining the two factor loadings to be equal to ensure model identification. Parental alcohol use had no loadings larger than 0.1 on any factor and was excluded from further analysis. Although parental cigarettes per day did load on the parental substance use factor, we excluded this variable because simultaneously using categorical and continuous indicators in one factor led to computational issues. Excluding these variables resulted in the solution presented in Table 4. This model showed good fit, RMSEA = 0.05, CFI = 0.97, TLI = 0.91. Variance explained in the observed variables by the factors ranged from 21.4% (for parental knowledge) to 62.3% (for parental solicitation), with an average of 42.2%. All factor loadings were significant, although the loading of parental knowledge on the first factor was low and this variable also loaded on the second factor. Because of the theoretical similarity to the variables in the first factor we decided to keep this variable in the first factor in the subsequent analyses. One of the most frequently observed modification suggestions was to add the correlation between parental knowledge and child disclosure. Reasoning that these concepts should be related, we added this correlation in all relevant models.
Table 3. Results for the exploratory factor analysis of the parenting variables. Fit indices per solution are provided. To the right side of the table are the χ2 for the difference between the models, with p < .05 indicating significant improvement with respect to the previous model with one factor less

Note: *indicates poor fit according to CFI/TLI < .90, RMSEA ≥ .08
CFI = comparative fit index; RMSEA = root mean square error of approximation; TLI = Tucker–Lewis Index
Table 4. Factor loadings (standard errors) for the best factor solution for the parenting variables (three factors) from the exploratory factor analyses (EFA).
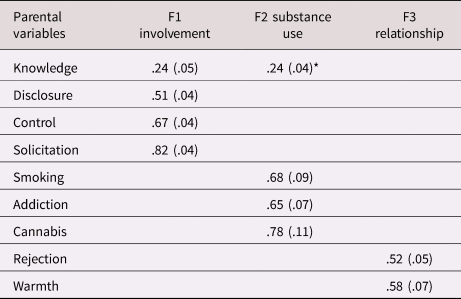
Note. *This cross loading was removed in subsequent models; knowledge was forced to load on F1.
The EFA indicated fit would improve further if the correlation between parental disclosure and knowledge in the first factor was allowed; this path was added in the subsequent structural equation models (SEM) analyses. Presented here are significant loadings (p < .05) with a value > .20.
For the young adult latent smoking factor, there were three indicators. Thus, the only possible factor solution contained one factor. All indicators loaded significantly on the smoking factor in the EFA, with 0.97 for daily smoking, 0.70 for cigarettes per day, and 0.81 for nicotine dependence. Fit indices were not interpretable because the model was just identified.
Structural equation models
Smoking factor
For each of the three parents factor separately we tested main, GxE, rGE, and total effects; the parameters are presented in Table 5 (refer to Supplementary Table S2 for parameter estimates for paths in the best fitting model). Model fit only reached acceptable levels when the parental factors were regressed on the covariates sex and age. We added these paths in all subsequent models for all outcomes (as the same was observed for alcohol per week and cannabis initiation). The effect of sex on smoking was not significant; the effect of age showed higher smoking levels in older individuals. The smoking PGS significantly predicted young adult smoking (R 2 = 4.8% for PGS).
Table 5. Model fit indices for each of the model steps. In bold the best fitting model per outcome according to the Akaike information criterion (AIC)/Bayesian information criterion (BIC)
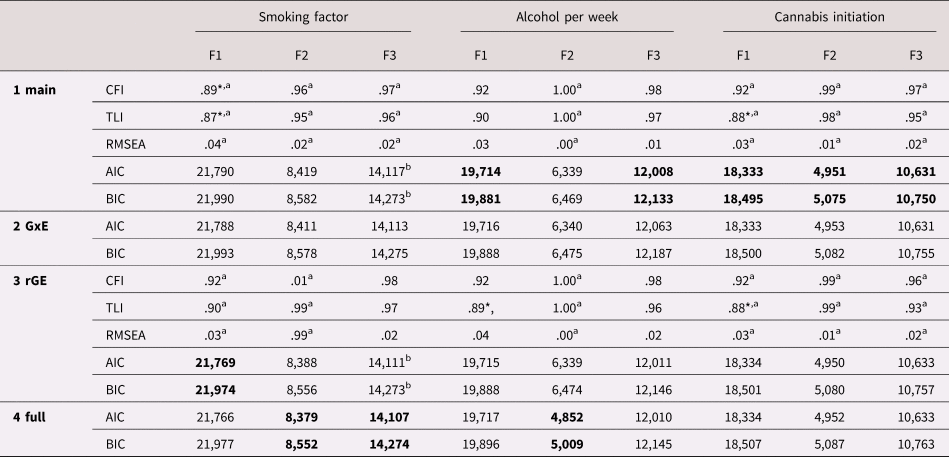
Note: F1 = parental involvement, F2 = parental substance use, F3 = parent–child relationship.
Comparative fit index (CFI), Tucker–Lewis Index (TLI), and root mean square error of approximation (RMSEA) indices are available only for the main and gene–environment correlation (rGE) models, as they cannot be computed for models containing latent interactions.
* Indicates poor fit according to CFI/TLI < .90, RMSEA ≥ .08.
a For these estimates we used weighted least square mean and variance (WLSMV) adjusted estimator, because they are not available with maximum likelihood estimator with robust standard errors (MLR) and categorical variables (indicators or outcome variables). AIC and BIC are based on the MLR estimator to allow for comparisons between the four different models.
b For these MLR models the model estimation reached a saddle point; however, model estimation (including standard errors) terminated normally, allowing for normal interpretation.
With parental involvement as predictor, the model excluding GxE and including rGE showed the best fit (Model 3). There was a main effect of parental involvement in mid-adolescence (such that higher involvement led to lower smoking in young adulthood) and an rGE between the young adult's smoking PGS and parental involvement (such that high genetic risk was associated with low parental involvement). Variance explained in the smoking factor by these paths was 13%.
With parental substance use, the full model (including GxE and rGE; Model 4) showed the best fit. Simple slope analysis suggested that parental substance use in early adolescence significantly predicted young adulthood smoking when the PGS was low (1SD below the mean; b = .07, SE = .02, p = .002, β = .18), but that this effect became stronger when the PGS was high (1SD above the mean; b = .19, SE = .09, p = .036, β = .48). It needs to be noted that although significant in the standardized model results, the GxE effect exceeded the p = .05 threshold in the unstandardized model results (due to a different computation of SE), suggesting this effect should be interpreted with caution. There was significant rGE between parental substance use and the smoking PGS. Together, these effects explained 14% of the variance in smoking.
With the parent–child relationship, again the full model showed the best fit (Model 4). A worse parent–child relationship in early adolescence was associated with more smoking in young adulthood. The GxE suggested that this relationship might become stronger when the young adult had a high PGS, but this effect was not significant (β = .10, p = .057). There was significant rGE between the parent–child relationship and the young adult's PGS. All paths together explained 10% of variance in the smoking factor.
The three best fitting smoking models are presented in Figure 2(a). Note that the analyses were conducted separately per parent factor but are summarized in one figure. Summarizing, there were significant positive main effects of the PGS and all parent factors on smoking, there was significant positive rGE between the PGS and all parent factors, and GxE with parental substance use. GxE with the parent–child relationship did not reach significance.
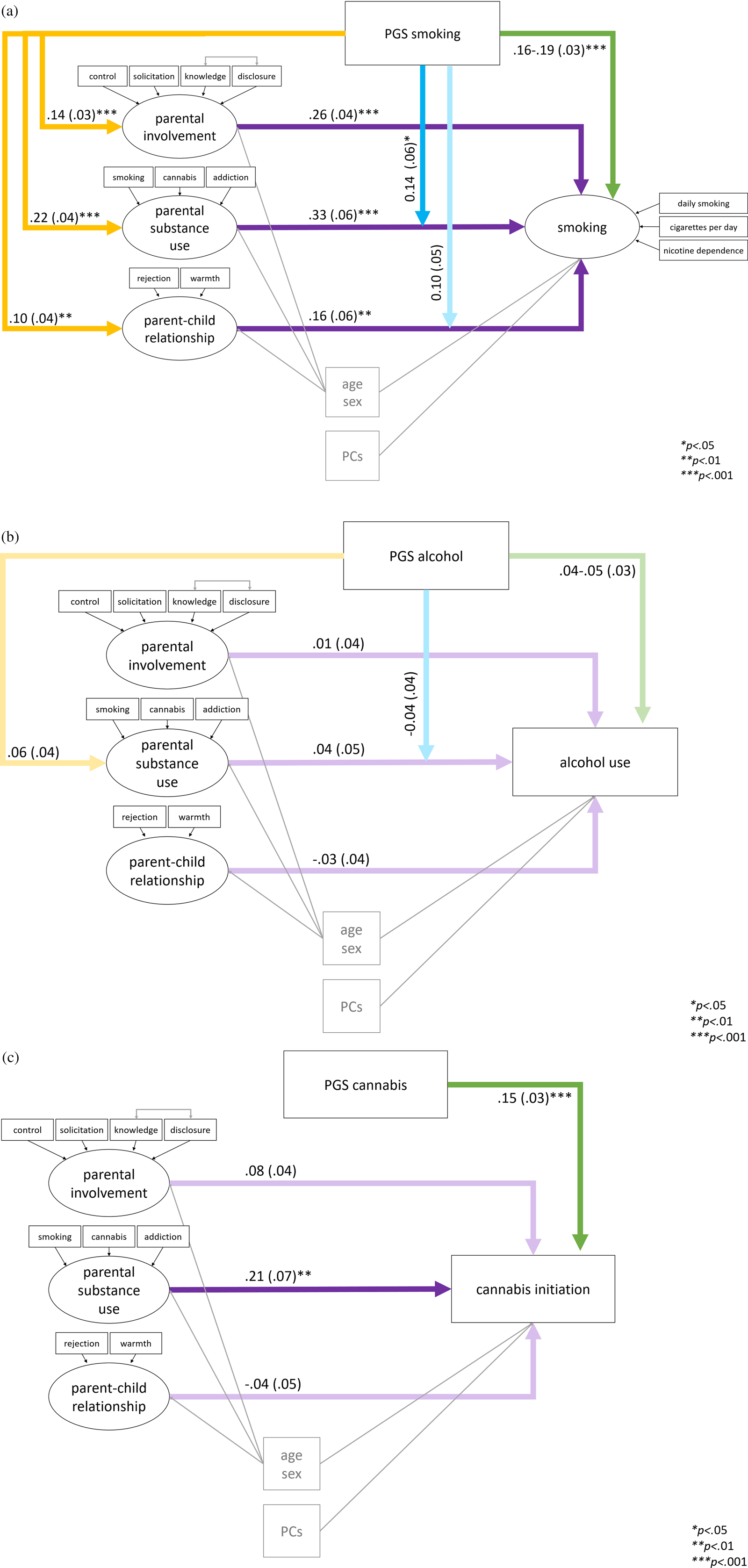
Figure 2. Standardized estimates β (with standard errors) from the best fitting structural equation models of parent factors and polygenic scores (PGS) predicting (a) smoking (complete outcome data N = 1,315); (b) alcohol per week (complete N = 1,122); and (c) cannabis initiation (complete N = 1,299). Note that the models presented in one figure were tested separately per parent factor due to nonconvergence when all models were included at once; these figures are summaries of the separate analyses.
Alcohol per week
For alcohol per week, the same main, GxE, rGE, and full models were tested separately for the three parent factors. With parental involvement and the parent–child relationship as predictors the main models showed the best fit (Model 1). Although with parental substance use the full model including rGE and GxE showed superior fit (Model 4), these paths were not significant. The alcohol PGS did not significantly predict young adult alcohol per week (p = .069-.108 in the main effect models; R 2 = 0.3% for PGS only). In addition, none of the early or mid-adolescence parenting factors predicted young adult alcohol per week (p = .460-.850). The best fitting models for alcohol per week are summarized in Figure 2(b). The variance explained in alcohol per week by all paths was 12% for all three models. Sex effects (β = .32-.34) might have contributed strongly to the explained variance, showing that males used significantly more alcohol than females. Age had no significant effect on alcohol per week.
Cannabis initiation
Finally, models assessing the main, GxE, rGE, and total effects of the three parent factors on cannabis initiation were tested. Cannabis initiation was significantly predicted by the cannabis PGS (R 2 = 2.3% for PGS only), see Figure 2(c). For all parent factors, the main model excluding rGE and GxE were the best fitting models (Model 1). Low parental involvement in mid-adolescence did not significantly increase chances for cannabis initiation in young adulthood (β = .08, odds ratio (OR) = 1.70. p = .064). Parental substance use in young adolescence did have a significant effect, such that it was associated with a higher chance of cannabis initiation. There was no effect of the parent–child relationship in young adolescence. No evidence for rGE or GxE was found. In the models with parental substance use and parent–child relationship there was a significant effect of sex, such that males had a higher chance of having used cannabis. In all models there was a positive effect of age.
Discussion
This 11-year longitudinal study investigated the effect of and interplay between genetic risk and parental factors during adolescence in predicting substance use in young adulthood. Results indicated that young adult substance use is driven by a complex interplay between genetic and parental factors during early and middle adolescence, especially for smoking. Smoking was predicted by genetic risk (PGS), parental involvement, parental substance use, and the parent–child relationship. The effect of parental substance use was further augmented by the PGS (GxE). In addition, there was evidence of gene–environment correlation between the parent factors and the smoking PGS (rGE). Alcohol use per week was not predicted by genetic risk, parent factors, or their interplay. Cannabis initiation was predicted by genetic risk and parental substance use separately, but not by any interplay between those.
Main effects of genetic and parent factors
Polygenic scores
The PGS for smoking behavior based on smoking initiation and cigarettes per day was a significant predictor of a latent factor for smoking behavior in young adults. Likewise, the cannabis PGS significantly predicted its own phenotype. However, the alcohol PGS did not predict alcohol use. This might be due to the fact that the PGS was based on GWAS in older adults, whose data were collected some time ago (Liu et al., Reference Liu, Jiang, Wedow, Li, Brazel, Chen and Psychiatry2019). Although the smoking PGS was based on the same sample, it also contained information on lifetime use, whereas the alcohol phenotype only captured current use. Alcohol consumption rates have been declining in Europe (World Health Organization, 2018) and attitudes toward alcohol seem to become slowly more negative in the Western world (Keyes et al., Reference Keyes, Schulenberg, O'Malley, Johnston, Bachman, Li and Hasin2012; Livingston & Callinan, Reference Livingston and Callinan2017; Looze et al., Reference Looze, Raaijmakers, Bogt, Bendtsen, Farhat, Ferreira and Pickett2015). These shifting attitudes (van Laar et al., Reference van Laar, Cruts, van Miltenburg, Strada, Ketelaars, Croes and Meijer2020) could have resulted in changes in the genetic risk profile. In addition, there are indications that the genetic contribution to alcohol use increases with age, and that environmental factors are more important for this behavior in adolescents and young adults (Hopfer et al., Reference Hopfer, Crowley and Hewitt2003; van Beek et al., Reference van Beek, Kendler, de Moor, Geels, Bartels, Vink and Boomsma2012). Finally, the alcohol use GWAS found low SNP-based heritability (4% of the variance in alcohol use was explained by all GWAS SNPs). PGS in general already tend to explain small proportions of variance, especially when SNP-heritability is low. Power for the alcohol PGS could have been higher if heritable traits such as alcohol dependence or abstinence were included, like we did for the smoking PGS. However, the nature of these traits is quite different. For smoking, initiation, quantity of use, and dependence are closely related and have rather similar prevalence (with 67% of initiators becoming dependent, Lopez-Quintero et al., Reference Lopez-Quintero, Cobos, Hasin, Okuda, Wang, Grant and Blanco2011), whereas for alcohol use initiation rates are high in the western population, but prevalence of dependence is rather low (WHO, 2018). Including genetic information on abstinence and dependence would therefore have increased heterogeneity and could have lowered power even further.
Parental involvement and the parent–child relationship
Lower parental involvement (comprising knowledge, control, solicitation, and child disclosure) in middle adolescence significantly predicted smoking behavior (comprising daily smoking, cigarettes per day, and nicotine dependence) in young adulthood. This is in line with previous literature showing cross-sectional effects of low parental monitoring (Rai et al., Reference Rai, Stanton, Wu, Li, Galbraith, Cottrell and Burns2003) and low parental knowledge on the children's whereabouts (Harakeh et al., Reference Harakeh, Scholte, Vermulst, de Vries and Engels2004). Likewise, a lower quality parent–child relationship (comprising higher rejection and lower warmth) in young adolescence significantly predicted higher young adult smoking levels, while controlling for the effects of parental substance use. This is in line with some previous literature (Harakeh et al., Reference Harakeh, Scholte, Vermulst, de Vries and Engels2004; Piko & Balázs, Reference Piko and Balázs2012). There are several possible explanations for these effects. Harakeh et al. (Reference Harakeh, Scholte, Vermulst, de Vries and Engels2004) reported that a good parent–child relationship led to negative smoking attitudes and high refraining self-efficacy regardless of parenting smoking status, and this in turn led to lower current and future smoking. A good parent–child relationship has been associated with better mental health and self-control (Ackard, Neumark-Sztainer, Story, & Perry, Reference Ackard, Neumark-Sztainer, Story and Perry2006; Phythian, Keane & Krull, Reference Phythian, Keane and Krull2008). In addition, adolescents with a good relationship with their parents might be more inclined to follow smoking rules set by their parents.
In contrast to some previous studies (Burdzovic Andreas, Pape, & Bretteville-Jensen, Reference Burdzovic Andreas, Pape and Bretteville-Jensen2016; Ryan et al., Reference Ryan, Jorm and Lubman2010; Visser et al., Reference Visser, de Winter and Reijneveld2012), we found no effect of parental involvement and the parent–child relationship on alcohol consumption and cannabis initiation. Possibly, parent behaviors during middle adolescence are less likely to exert effects across longer time-frames (i.e., in young adulthood) for these substances. Alcohol use might also be something that is less likely to be under strict parental control, as this represents more normative, socially acceptable behavior (Maciejewski et al., Reference Maciejewski, Keijsers, van Lier, Branje, Meeus and Koot2019). Furthermore, specific parenting practices, such as alcohol and cannabis rule setting, could be more important predictors for alcohol and cannabis use (Engels & Bot, Reference Engels and Bot2006; Vermeulen-Smit, Verdurmen, Engels, & Vollebergh, Reference Vermeulen-Smit, Verdurmen, Engels and Vollebergh2015).
Parental substance use
Higher levels of parental substance use in early adolescence (comprising binary measures of current smoking, recent cannabis use, and lifetime addiction) significantly predicted higher levels of smoking and higher chances of cannabis initiation in young adulthood. These effects might be direct modeling effects, such that offspring imitate observed parental substance use, or indirect modeling effects, for example through attitude formation and rule setting (Engels & Bot, Reference Engels and Bot2006). We did not find an effect of the parental substance use factor on alcohol use, presumably because this factor did not include parental alcohol use. In addition, modeling effects might be less strong for alcohol which is predominantly used in the peer context, especially by older adolescents (Goncy & Mrug, Reference Goncy and Mrug2013).
Age and sex
Considering covariates, it is interesting to see that age had a significant positive effect on cannabis initiation and smoking behavior, even though the age variability in the sample was low. This suggests that these years in young adulthood comprise a sensitive period in the development of substance use where much change is occurring. This is in line with previous literature showing different trajectories of change and development in this period (Bachman, Wadsworth, O'Malley, Johnston, & Schulenberg, Reference Bachman, Wadsworth, O'Malley, Johnston and Schulenberg2013). We observed that males consumed more alcohol and had higher chances of cannabis initiation, consistent with estimates in the general population (Centraal Bureau voor de Statistiek, 2020). We observed no sex differences in smoking after controlling for the other factors in the model, even though population statistics suggest such a difference exists (Leefstijlmonitor, 2020). This suggests that sex differences might be mediated by differences in parent factors. Interestingly, there were significant associations between parent factors and sex, such that males experienced lower parental involvement and a lower parent–child relationship quality, and higher levels of parental substance use in the cannabis initiation model (see Supplementary Table S4). This is in line with previous reports of small differences in parenting behavior towards sons versus daughters, that could be due to gender roles in society and gender stereotypes (Endendijk, Groeneveld, Bakermans-Kranenburg, & Mesman, Reference Endendijk, Groeneveld, Bakermans-Kranenburg and Mesman2016). Though outside of the scope of this study, future research could further explore these effects.
Gene x Environment interaction (GxE)
One of nine tested GxE paths reached significance at a conventional p < .05 threshold. There was positive GxE between parental substance use and the PGS on smoking. Although the models containing GxE showed the best fit for the parent–child relationship on smoking and for parental substance use on alcohol per week, these GxE paths did not reach significance and the effects were small. In addition, the negative direction of the GxE in the alcohol model is not in line with a pattern where environmental risk amplifies genetic risk, as is the most commonly found GxE pattern (Pasman et al., Reference Pasman, Verweij and Vink2019).
The effect of parental substance use on smoking was enlarged when genetic risk for smoking was high. This direction is in line with differential susceptibility frameworks, which state that the effect of an environmental factor can be amplified when genetic vulnerability is high (Belsky & Pluess, Reference Belsky and Pluess2009). Such an effect would contribute to the likelihood that smoking becomes widespread in families and would suggest that especially individuals that are at risk genetically would benefit from prevention targeted at parental substance use. An alternative explanation might be that this effect is driven by the overlap in genetic risk for smoking between parents and offspring. However, we tested this by bringing the gene–environment correlation (rGE) between parental substance use and the offspring's smoking PGS into the model, and this did not change the GxE effect. Thus, parental substance use affected smoking and magnified the effect of genetic risk on smoking independently of genetic overlap with the young adult. Still, because the effect was small and was the only one to reach significance in the tested models caution must be taken in the interpretation.
Although it is possible that GxE effects are specific to smoking and parental substance use only, there are alternative explanations for the fact that only this GxE path was significant. The smoking analyses are likely to be the most powerful. We used a multivariate, more informative approach to compute the smoking PGS. The smoking outcome likewise used information from multiple traits. In addition, the parental substance use factor had the largest main effect (which is relevant in this case as the PGS augmented this main effect). If the parental substance use factor had included a measure of alcohol use, it might have been more likely to have an effect in the alcohol models. Although we conducted power analyses and power was deemed sufficient to detect GxE also in the other models (see preregistration), it is possible that we were overly optimistic in choosing parameters for this analysis. This certainly seems likely for the alcohol analyses, where the PGS did not predict its own phenotype. Another explanation as to why GxE effects tested with PGS are generally difficult to detect is that GWAS only test direct associations between variants and outcomes, and would not detect variants that increase vulnerability to environmental circumstances per se (Fox & Beevers, Reference Fox and Beevers2016). In addition, there is a possibility that individual variants included in the PGS interact or correlate with environmental exposures in different directions, cancelling out an overall interaction effect.
Gene–environment correlation (rGE)
For the smoking models, there was significant rGE between the PGS and all parent factors. rGE between the smoking PGS and parental substance use likely stems from genetic overlap between parent and offspring (“passive” rGE, Knafo & Jaffee, Reference Knafo and Jaffee2013; Plomin, DeFries, & Loehlin, Reference Plomin, DeFries and Loehlin1977). Beside passive rGE driven by transmitted parental alleles, there can be evocative or reactive rGE, that could also arise from nontransmitted alleles (“genetic nurturing,” Kong et al., Reference Kong, Thorleifsson, Frigge, Vilhjalmsson, Young, Thorgeirsson and Stefansson2018). Possibly, the association between the smoking PGS and parental involvement arose through such processes. For instance: certain SNPs are associated with smoking; smoking in turn could lead to parental disapproval, lower parental involvement, and lower relationship quality; and this would result in a correlation between the smoking SNPs and a negative parent environment. For the effect of the relationship quality and parent substance use evocative processes are a less plausible explanation in the current study, since these were measured at age 11, when virtually no adolescent had initiated smoking. For parental involvement (measured at age 16), evocative processes may well have played a role. Alternatively, there may be pleiotropic smoking SNPs that influence some other behavior which in turn elicits a response in the parents. For instance, SNPs important for smoking have also been associated with attention-deficit hyperactivity disorder (ADHD; Liu et al., Reference Liu, Jiang, Wedow, Li, Brazel, Chen and Psychiatry2019), which commonly develops at a much earlier age than smoking behavior, and can elicit negative parenting behaviors, including lower parental warmth and less solicitation (Glatz, Stattin, & Kerr, Reference Glatz, Stattin and Kerr2011). Pleiotropy is the rule rather than the exception for SNPs associated with complex behavior (Lee et al., Reference Lee, Anttila, Won, Feng, Rosenthal and Smoller2019). A combination of passive and evocative processes might also exist, for instance such that transmitted smoking SNPs give rise to ADHD-like behavior in the parent, resulting in ineffective parenting behaviors (Mokrova, O'Brien, Calkins, & Keane, Reference Mokrova, O'Brien, Calkins and Keane2010). Still, all of these explanations are speculative; there might also be genetic overlap with some phenotype that would elicit an opposite response. Future genetic nurturing (Kong et al., Reference Kong, Thorleifsson, Frigge, Vilhjalmsson, Young, Thorgeirsson and Stefansson2018) or Mendelian randomization studies could further disentangle underlying causal mechanisms.
It needs to be noted that GxE and rGE effects did not share much variance and adding them in one model did not change either effect. It is interesting to see that these effects operate independently, as previous research has cautioned for bias introduced by rGE when testing GxE (e.g., Pasman et al., Reference Pasman, Verweij and Vink2019). By testing both effects simultaneously, it became clear that rGE is independent from and at least as prominent as GxE in smoking, and is as such deserving more research attention.
No rGE effects were detected for the alcohol and cannabis models, which could be due to lower power of these PGSs, or may represent a real difference for these substances. For instance, smoking may be more potent to elicit negative reactions from the parents (evocative rGE) than alcohol use, which is more socially accepted, or cannabis initiation, which may be more likely to occur outside of the parents’ awareness. For alcohol use, the low SNP-heritability may also have contributed. Other factors being equal, traits with a higher heritability are more likely to show overlap between parents and offspring (passive rGE).
Strengths and limitations
This is the first PGS study to our knowledge to investigate the main effects and complex interplay between genetic and parental factors during adolescence to understand substance use in young adults. The advantage of our use of SEM was that we could model directional paths (which makes sense in the case of genetic predictors that cannot be influenced by other parameters in the model) and test the relative contributions of main, rGE, and GxE effects. In addition, the use of latent factors enabled us to leverage the wealth of information that was present in the TRAILS dataset. Effects were compared across different parenting characteristics and different substance use outcomes. We employed powerful and up-to-date PGS methods and summary statistics from the largest GWAS available to date.
Limitations of this study include the computational constraints of SEM which made it impossible to include all parent factors in a single model, or similarly, to look at all substance use outcomes simultaneously. Thus, unique contributions to substance use and interdependency between parent factors and substance use outcomes could not be tested. In addition, it would have been informative to include parental PGS within the model, to test for actual parent–offspring genetic overlap and tease apart the rGE effects. For instance, overlap in certain parent–offspring genotypes would provide support for passive rGE effects, whereas differences would indicate other rGE mechanisms. Unfortunately, parental genotypes were not available. Similarly, given the sex differences that we found both in outcomes and parental predictors (Supplementary Tables S2 and S3), it would have been interesting to conduct multigroup analyses and formally test sex differences. However, given the small effect sizes under investigation, we lacked power to do so. Given the interdependent nature of the tested models (and our initial plan to test all effects within a single model, see preregistration and Supplementary Table S3) we did not deem it necessary to correct for multiple testing. It needs to be noted that some of the p values reported would not have survived such corrections; these effects have to be interpreted with caution. We note that we did not control for baseline levels of substance use in our models. Our design was not focused on strictly time-ordered developmental or causal processes, but rather aimed to provide insight into lasting associations between substance use and parenting behaviors. Thus, many causal pathways, including evocative processes, could explain the associations we observe. Further, although we conducted power analyses, effect sizes might have been smaller than anticipated. We only found GxE and rGE effects for smoking, which had the most powerful PGS (based on MTAG) and strongest outcome measure (latent factor with multiple smoking behavior indicators), suggesting that power might have been an issue in the other models. The low SNP-based heritability in some of the source GWAS suggests that the power of the PGS may have been limited. In addition, power might have been limited by selective attrition between baseline and Wave 5 of participants of lower socioeconomic status (de Winter et al., Reference de Winter, Oldehinkel, Veenstra, Brunnekreef, Verhulst and Ormel2005; Huisman et al., Reference Huisman, Oldehinkel, de Winter, Minderaa, de Bildt, Huizink and Ormel2008; Ormel et al., Reference Ormel, Oldehinkel, Sijtsema, van Oort, Raven, Veenstra and Verhulst2012), a factor that has previously been associated with substance use (Johnson, Hicks, McGue, & Iacono, Reference Johnson, Hicks, McGue and Iacono2009; Patrick, Wightman, Schoeni, & Schulenberg, Reference Patrick, Wightman, Schoeni and Schulenberg2012). As a more general limitation, it needs to be noted that we only included individuals of European ancestry in our genetic analyses; as discovery GWAS are still largely unavailable for other ethnic groups, currently PGS research can only reliably be conducted in European samples.
Conclusions and Future Directions
Summarizing, we found that high genetic risk, low parental involvement, high parental substance use, and a low-quality parent–child relationship predicted smoking and cannabis initiation, but not alcohol use.
For smoking, the effect of genetic risk was enlarged by parental substance use. In addition, genetic risk for smoking was associated with lower parental involvement, higher parental substance use, and a lower quality parent–child relationship. In addition, we showed that rGE and GxE operated relatively independently from each other and are unlikely to be captured when not modeled explicitly. Our findings that parent behavior influences substance use both directly and through indirect genetic pathways suggest that parents are an important target point for intervention, especially for smoking behaviors. Future studies should aim to identify causal genetic pathways that operate independently from environmental circumstances, to provide clues for underlying biological mechanisms and potentially provide targets for pharmacogenetic interventions. Further elucidating pathways of genetic risk will provide more clues as to where prevention and intervention can be aimed to break the causal chain.
Supplementary Material
The supplementary material for this article can be found at https://doi.org/10.1017/S095457942100081X
Acknowledgments
This research is part of the TRacking Adolescents’ Individual Lives Survey (TRAILS). Participating centers of TRAILS include various departments of the University Medical Center and University of Groningen, the University of Utrecht, the Radboud Medical Center Nijmegen, and the Parnassia Group, all in the Netherlands. We are grateful to everyone who participated in this research or worked on this project to and make it possible.
Author Contributions
Dominique Maciejewski and Jacqueline M. Vink contributed equally.
Funding Statement
TRAILS has been financially supported by various grants from the Netherlands Organization for Scientific Research (NWO), ZonMW, GB-MaGW, the Dutch Ministry of Justice, the European Science Foundation, the European Research Council, BBMRI-NL, and the participating universities.
KJHV and AA were supported for this work by the Volksbond Rotterdam.
Conflicts of Interest
None