INTRODUCTION
In some conflicts, participants are motivated by material considerations—the spoils of war, future political power, and so on. In others, the motivation is ideological or psychological. How do different types of motivation influence the success or failure of collective action? How does the nature of the good being sought in the process of popular dissent affect how both rebellion and repression work? Does a state’s repression policy vary depending on the primary motivations of the rebels? We develop a model of endogenous collective action and endogenous repression to study how different types of individual motivation affect participation, states’ repressive policies, and the mechanisms by which state violence affects political contention.Footnote 1
We adopt the standard conception of state repression as any action by the state that “raises the contenders’ cost of collective action” (Tilly Reference Tilly1978, 100; also see Davenport Reference Davenport2007; Earl Reference Earl2011). Thus, in our model, higher levels of repression are represented by the state imposing higher costs of mobilizing for rebellion.
We compare two broad types of rebel motivations: psychological and material. The psychological motivations we consider have to do with concerns like emotion, identity, and the quest for justice, which have been much discussed in the literature on why people rebel (Aytaç and Stokes Reference Aytaç and Stokes2019; Balcells Reference Balcells2012; Blattman and Miguel Reference Blattman and Miguel2010; Lawrence Reference Lawrence2017; Opp and Ruehl Reference Opp and Ruehl1990; Toft and Zhukov Reference Toft and Zhukov2015; Pearlman Reference Pearlman2013; Reference Pearlman2018; Wood Reference Wood2003; Zhukov and Talibova Reference Zhukov and Talibova2018). For instance, Wood (Reference Wood2003) finds that rebels in El Salvador were motivated by psychological rewards ranging from vengeance to the opportunity to be “part of the making of history” (18–9). The material motivations we consider have to do with the rents associated with political and territorial control, which are also widely discussed (Dal Bó and Dal Bó Reference Dal Bò and Bò2011; Dube and Vargas Reference Dube and Vargas2013; Ellis Reference Ellis1999; Hirshleifer Reference Hirshleifer1991; Humphreys and Weinstein Reference Humphreys and Weinstein2008;Weinstein Reference Weinstein2007). For example, Weinstein (Reference Weinstein2007) and Humphreys and Weinstein (Reference Humphreys and Weinstein2008) find that many rebel fighters in Sierra Leone were motivated by opportunities for looting, drug sales, and other material gains.
The difference between material and psychological motivations, in our approach, hinges on whether or not the rewards from successful rebellion are rivalrous. Material goods are rivalrous in our model because materially motivated rebels must share the spoils of victory. In contrast, psychologically motivated rebels need not divide the fruits of victory, as one person’s feelings of having achieved justice or having been part of history need not detract from another’s feelings of the same.
Our model provides insights that advance our understanding of both the mechanisms by which state violence affects political contention and why certain types of policy responses to public demands may or may not be successful. In particular, the model establishes four primary results. We show the following, all else being equal:
-
(1) Strategic governments repress psychologically motivated groups more than materially motivated groups.
-
(2) Psychologically motivated groups are less effective at achieving their goals than are materially motivated groups.
-
(3) Repression has a larger marginal effect on the efficacy of psychologically motivated groups than of materially motivated groups—materially motivated rebels are harder to discourage.
-
(4) At the endogenous repression level chosen by governments, psychologically motivated groups are less effective at achieving their goals than are materially motivated groups.
Thus, the model yields predictions about the amount of repression against different types of rebel groups, the efficacy of that repression, and the likelihood of success of differentially motivated movements.
In addition to providing new insight into rebellion and repression, our model’s implications point to three complications for empirical studies of the efficacy of repression. These complications may help shed light on why the empirical literature on repression finds such highly variable results (for reviews, see Davenport Reference Davenport2007; Earl Reference Earl2011). The first complication involves causal inference. If governments repress psychologically motivated groups more than materially motivated groups (result 1) and psychologically motivated groups are less effective than materially motivated groups, all else equal (result 2), then rebel motivations are a confounder in attempts to estimate the efficacy of repression. The second complication involves heterogeneous treatment effects. Our model implies that the effect of repression depends on rebel motivations (result 3). Thus, estimates of the efficacy of repression are sensitive to the sample of conflicts being studied. And, moreover, if repression is more frequently used against psychologically motivated groups (result 1), the set of cases where we observe repression and can estimate its efficacy will overrepresent cases where it is particularly efficacious. The third complication is methodological. If repression is relatively rare, then with finite data, all else equal, the effect of repression will be more precisely estimated in conflicts with a relatively high level of repression (so that the treated group is closer in size to the untreated group). This will affect the set of conflicts for which we can find statistically significant evidence of the efficacy of repression, and is important to take into consideration when considering how to interpret the results of a meta-analysis (whether formal or implicit in a reading of the literature) that weights estimates by the inverse of their precision. We provide a detailed discussion and numerical illustrations later in the text.
Although our focus is on how motivations affect rebellion and repression, it is worth noting that our model has some broader implications as well.
First, our results on which types of groups are most affected by repression also apply to the effects of exogenous economic shocks or other nonrepressive changes that influence participation costs—just as endogenous repression decisions by the state affect those costs. Nonrepressive changes in the opportunity costs of mobilization have the same effect in the model as repression. For instance, better economic opportunities increase the opportunity costs of participation and, thus, have heterogeneous effects that are similar to those of repression. Counterintuitively, then, the model implies that positive economic shocks will decrease rebel efficacy and that the effect of such shocks is greater on psychologically motivated groups than on materially motivated groups. Indeed, the literature features conflicting empirical results on the effect of economic conditions on mobilization (see, e.g., the discussion in Bazzi and Blattman Reference Bazzi and Blattman2014). The literature suggests different potential explanations—for example, based on the distribution of opportunity cost shocks in the population (Dube and Vargas Reference Dube and Vargas2013) or informational mechanisms (Shadmehr and Boleslavsky Reference Shadmehr and Boleslavsky2022)—but does not take rebel motivations into account.
Second, we provide an extension that considers the resiliency of rebel groups to early setbacks. In a dynamic setting, we show that movements whose members are psychologically rather than materially motivated are better able to turn early failures, resulting from repression, into future successes. This is because the committed core that is often left behind by repression (Bursztyn et al. Reference Bursztyn, Cantoni, Yang, Yuchtman and Zhang2021; Lawrence Reference Lawrence2017; Pearlman Reference Pearlman2021; Wood Reference Wood2003) is better able to spark future mobilization when motivations are psychological—see also Diani and McAdam (Reference Diani and McAdam2003). This result provides an important caveat to the implication of our earlier analysis suggesting that, all else equal, psychologically motivated rebels are less effective and more easily repressed than are materially motivated rebels. The extension shows that, although groups whose members are psychologically rather than materially motivated are better deterred by repression, they are also better able to turn early failures into future successes.
Third, the need to coordinate for collective action is not unique to rebellion. Many of the same issues arise in analyzing mobilization for nonviolent protest and even for government-sponsored war. Therefore, our analysis may apply to such behavior as well.
The Nature of Material and Psychological Motivations
In our conceptualization, material and psychological motivations share an important feature and also differ in an important respect. The common feature is that both are contingent on the success of the movement.Footnote 2 Rewards being contingent on success is important because it implies a force pushing for strategic complementarities—if an agent believes other agents are more likely to participate, and therefore the rebellion is more likely to succeed, that agent believes they are more likely to enjoy benefits from having participated. The point of divergence is that material rewards are rival goods, whereas psychological rewards are nonrival. When rewards are material, as the movement becomes larger, success is more likely, but the rewards to each individual conditional on success are smaller. When rewards are psychological, as the movement becomes larger, success is more likely and rewards don’t change. Why these similarities and distinctions?
Our focus on success-contingent motivation is consistent with Rasler’s (Reference Rasler1996) “value expectancy” model, and her empirical evidence supports the associated implications (148). As Rasler (Reference Rasler1996) argues, “Value-expectancy models assert that people will rebel if they become convinced that dissent will achieve the collective good (Finkel, Muller, and Opp Reference Finkel, Muller and Opp1989; Klandermans Reference Klandermans1984; Muller and Opp Reference Muller and Opp1986). If the value of the collective good (e.g., overthrow of the Shah’s government) is combined with a high expectation of success, people are likely to participate in mass actions” (134).
Material benefits take various forms, including direct payments, protection, opportunities for looting, and promises of future economic spoils. Consider a few examples from the literature. Popkin (Reference Popkin1979) describes offering peasants material rewards for mobilizing as a crucial recruitment strategy for Vietnamese insurgents. Ross (Reference Ross2006) summarizes the extensive literature showing that the presence of lootable diamonds helps to motivate and sustain rebellion. Several studies show evidence that rebel violence in conflicts ranging from Colombia to Chad to the Republic of Congo is motivated by the desire to capture control over valuable oil resources (Dube and Vargas Reference Dube and Vargas2013; Englebert and Ron Reference Englebert and Ron2004; Humphreys Reference Humphreys2005). Shapiro (Reference Shapiro2013) presents documentary evidence of disputes within al Qaeda around various members’ material compensation. And Goodwin and Skocpol (Reference Goodwin and Skocpol1989, 494) argue that quite broadly “it is the on-going provision of such collective and selective goods, not ideological conversion in the abstract, that has played the principal role in solidifying social support for guerrilla armies.”
Of course, some material resources, such as the looting while fighting or monthly salaries, may be enjoyed by participants even during the course of a failing campaign. A conflict in which such rewards were unlimited (and, thus, nonrival) and unrelated to success would not be well described by our model. But in the typical case, material resources are scarce and a losing movement will eventually be unable to keep providing such material resources. Moreover, many economic benefits of rebellion, such as the rents from oil production discussed above, are only available if the rebels can successfully hold oil-producing territory. And, of course, economic spoils are finite. They must be divided among the participants in the victorious movement, thus the disputes documented by Shapiro (Reference Shapiro2013). Therefore, it is natural to think that they are rival—the larger the movement, the less each participant expects to receive. This assumption is consistent with a large literature arguing that there is often conflict among rebels when the rebellion is materially motivated—see Fjelde and Nilsson (Reference Fjelde and Nilsson2012) for a discussion and evidence.
The nature of psychological benefits has long been the subject of debate. Early work emphasized purely expressive motives (Davies Reference Davies1962; Geschwender Reference Geschwender1967; Gurr Reference Gurr1970). But later studies showed that even psychologically motivated individuals account for the likelihood of success and the costs of participation when deciding whether to mobilize (McAdam Reference McAdam1999; Tarrow Reference Tarrow2011; Tilly Reference Tilly1978; Reference Tilly2008).Footnote 3 In particular, movements with no prospect of success are unlikely to be sustainable because the costs of participation exceed the psychological benefits. Later studies confirmed this insight and developed a success-contingent conception of psychological and ideological rewards. Wood’s (Reference Wood2003) notion of pleasure-in-agency captures psychological rewards associated with participating in a successful movement. Wood defines pleasure-in-agency as “the positive effect associated with self-determination, autonomy, self-esteem, efficacy, and pride that come from the successful assertion of intention” (235). Based on extensive fieldwork and building on the historical and sociological literature, Wood found that agents motivated by psychological rewards both account for the likelihood of success and act strategically: pleasure-in-agency is “a frequency-based motivation: it depends on the likelihood of success, which in turn increases with the number participating (Schelling Reference Schelling1978; Hardin Reference Hardin1982)” (235–6). Such findings suggest that whether psychological rewards derive from ideology, the satisfaction of “being part of the making of history,” justice, honor, or vengeance, the net benefit is positive only if the movement succeeds (Aytaç and Stokes Reference Aytaç and Stokes2019; Morris and Shadmehr Reference Morris and Shadmehr2017; Pearlman Reference Pearlman2018; Petersen Reference Petersen2001; Wood Reference Wood2003). By contrast with the material setting, the satisfaction from implementing an ideological vision, achieving justice, or being part of history is not diminished for being shared. Thus, it is natural to think of psychological rewards as nonrival—as more people join the movement, the likelihood of success increases, with no diminution in individual rewards conditional on success.
Capturing these ideas—especially the rivalrous nature of material rewards—requires an analysis with multiple people considering whether or not to mobilize. This gives rise to coordination considerations: whether one individual wishes to participate depends on her beliefs about how many others will participate. The dual presence of coordination concerns and congestion externalities (due to rivalrous material rewards) significantly complicates the strategic environment, precluding the application of standard models. Almost all models of protest and revolution feature pure coordination considerations with no congestion externalities (Boix and Svolik Reference Boix and Svolik2013; Casper and Tyson Reference Casper and Tyson2014; Chen, Lu, and Suen Reference Chen, Lu and Suen2016; Correa Reference Correa2021; Edmond Reference Edmond2013; Loeper, Steiner, and Stewart Reference Loeper, Steiner and Stewart2014; Nandong Reference Nandong2020; Rundlett and Svolik Reference Rundlett and Svolik2016; Tyson and Smith Reference Tyson and Smith2018).Footnote 4 The complexity arises because there is a force for strategic complementarity (when more people mobilize, the chances of success are higher) and a force for strategic substitutes (when more people mobilize, the rewards of victory are smaller). Consequently, for example, as a citizen becomes more optimistic about the likelihood of regime change, her incentives to participate may, paradoxically, fall. The analysis of these competing forces and their interactions with repression requires a formal model that incorporates both forces in a tractable manner. We provide such a model and analysis.
MODEL OF REBELLION
We start with a model of rebellion with fixed repressive capacity. Within that model we characterize equilibrium and analyze the efficacy of repression as comparative statics. We then add an earlier stage in which the government chooses repressive capacity and characterize perfect Bayesian equilibria of this augmented game. In the model of rebellion, there is a continuum of citizens of size
$ a>0 $
, indexed by
$ i\in \left[0,a\right] $
. Citizens simultaneously decide whether to participate in a rebel movement. The rebellion succeeds if and only if the fraction of rebels in the population exceeds the state of the world,
$ \theta $
, which captures the strength of the status quo regime.Footnote
5 Letting
$ m\in \left[0,a\right] $
be the size of the rebels, the fraction of rebels in the population is
$ \frac{m}{a} $
.
The payoff of a citizen who does not rebel is normalized to 0. A citizen who rebels pays a cost
$ c\in \left(0,1\right) $
. If the rebellion succeeds, a citizen who participated receives a payoff
$ {u}^j $
,
$ j\hskip0.35em =\hskip0.35em p,\hskip0.3em m $
, where
$ {u}^p $
is the reward in the setting with psychological rewards and
$ {u}^m $
is the reward in the setting with material rewards. Psychological rewards are normalized to 1, and material rewards are normalized to
$ \frac{a}{m} $
so that if the rebellion succeeds, the total available rewards in both settings is a. Figure 1 represents the payoffs.
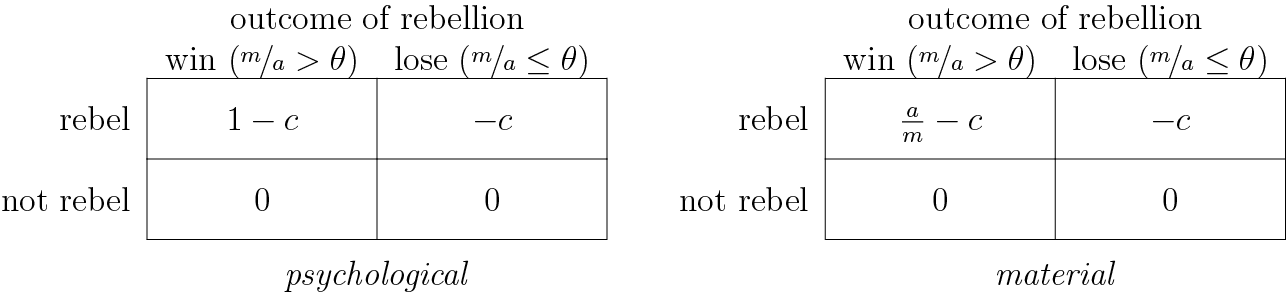
Figure 1. Psychological versus Material Rewards
Note: The size of the population is a, the size of rebel movement is
$ m\le a $
, the cost of participation is c, and the regime’s strength is
$ \theta $
. The left panel captures movements with psychological rewards: net rewards from participation do not depend on how many participate. The right panel captures movements with material rewards: net rewards from participation fall with more participation because participants must share the spoils.
The state of world is uncertain, and citizens share a common prior that
$ \theta $
is distributed on ℝ according to an improper uniform distribution. Each citizen i receives a noisy private signal
$ {x}_i\hskip0.35em =\hskip0.35em \theta +{\sigma \varepsilon}_i $
, where
$ \theta $
and
$ {\varepsilon}_i $
s are distributed independently, with ε
i ~ F and the corresponding probability density function
$ f $
. We assume
$ f $
is log concave with full support on ℝ.
Complete Information Benchmark
We begin with the complete information benchmark in which the regime’s strength
$ \theta $
is known. (All proofs are in the appendix.)
Proposition 1. The setting with psychological rewards and the setting with material rewards both have the same pure strategy equilibria:
-
• If
$ \theta \ge 1 $ , there is a unique equilibrium in which no one rebels, the regime survives, and each citizen receives 0.
-
• If
$ \theta \hskip0.2em <0 $ , there is a unique equilibrium in which everyone rebels, the regime collapses, and everyone receives 1.
-
• In between, both equilibria coexist.
Proposition 1 implies that the settings with psychological and material rewards generate the same outcomes. However, this complete information setting is misleading. Of course, it abstracts from information frictions that exist in the real world. Moreover, it has two problematic properties: (1) there are multiple equilibria, which makes empirical prediction difficult, and (2) equilibrium outcomes are insensitive to parameters of the model like the costs of rebellion. The introduction of incomplete information addresses both issues.
Equilibrium
We now analyze our incomplete information model of rebellion. The left panel in Figure 1 is the quintessential regime change game (Angeletos, Hellwig, and Pavan Reference Angeletos, Hellwig and Pavan2007; Morris and Shin Reference Morris and Shin1998; Reference Morris, Shin, Dewatripont, Hansen and Turnovsky2003).Footnote
6 In it, equilibrium is characterized by two thresholds
$ \left({x}^p,{\theta}^p\right) $
so that a citizen i with signal
$ {x}_i<{x}^p $
rebels and the regime collapses if and only if
$ \hskip0.35em \theta <{\theta}^p $
. These thresholds are determined by the indifference (optimality) and belief consistency conditions:

Because each citizen rebels whenever her signal of the regime’s strength is below a threshold, for any given regime strength
$ \theta $
, the aggregate size of the rebellion as a fraction of the population is
$ \Pr \left({x}_i<{x}^p,|,\theta \right) $
. Naturally, the size of the rebellion is decreasing in the regime’s strength, implying that the regime collapses below a threshold of regime strength and survives above it. Thus, that critical threshold (which we call
$ {\theta}^p $
) is exactly the size of the rebellion at that critical threshold.
How do we find this critical rebellion size? Because a citizen rebels whenever her belief about the likelihood of success is larger than the cost of rebelling, to find the size of the rebellion at the critical threshold we need to know the distribution of these beliefs at that critical threshold. As Shadmehr (Reference Shadmehr2019a) discusses in detail, when there is no prior knowledge about
$ \theta $
,Footnote
7 the distribution of these beliefs about the likelihood of success at the critical threshold is uniformly distributed on [0,1] among citizens. Thus, the size of the rebellion as a fraction of the population is the probability that a random citizen’s belief is above the rebellion cost, 1–c. That is,

The nature of strategic interactions is a pure coordination problem. The game is a standard global game of regime change, where the actions of citizens are always strategic complements: when one citizen believes that others are more likely to rebel, her incentives to join the rebellion increase because the rewards remain the same, but the likelihood of success increases.
In contrast, the game in the right panel of Figure 1 is not a pure coordination game. In this game, when a citizen believes that others are more likely to rebel, her incentives to protest may fall because, although success is more likely, the limited rewards from that success will be shared among a larger group so that each participant will expect to receive fewer rewards conditional on success. That is, the game does not feature global strategic complements due to congestion externalities. In particular, for a given level of regime strength,
$ \theta $
, the net payoff from revolting versus not revolting is

This net payoff is nonmonotone in the size of the rebellion m. It jumps up from 0 to
$ \frac{a}{m}-\hskip0.2em c $
at
$ \frac{m}{a}\hskip0.35em =\hskip0.35em \theta $
(the threshold at which regime change succeeds) but then falls smoothly to 1–c as more people join the movement. Therefore, the best response to a monotone strategy is not monotone in general, and monotone equilibria may not exist. The source of this complication, relative to the psychological rewards setting, is that the expected rewards do not boil down to the likelihood of success because higher chances of success also imply a larger rebellion size, which in turn, implies a smaller reward for each participant. That is, when a citizen receives a lower signal, she updates that the regime is weaker and the size of the rebellion larger. This updating increases her assessment of the chances of success but reduces her assessment of the reward conditional on that success (the citizen updates
$ {\mathbf{1}}_{\left\{\theta <m/a\right\}} $
upward, but
$ \frac{a}{m} $
downward). Despite this nonmonotonicity, Proposition 2 shows that our assumptions are enough to deliver the existence and uniqueness of symmetric monotone equilibria.Footnote
8
Proposition 2. The setting with psychological rewards has a unique equilibrium in which the rebellion succeeds if and only if the strength of the regime is below a threshold
$ {\theta}^p=\hskip0.35em 1-\hskip0.1em c $
. The setting with material rewards has a unique equilibrium in which the rebellion succeeds if and only if the strength of the regime is below a threshold
$ {\theta}^m\hskip0.35em =\hskip0.35em {e}^{-c} $
.
Proposition 2 implies that
$ {\theta}^m>{\theta}^p $
. Because total rewards in the material setting are divided among rebel participants and some citizens always choose not to rebel in equilibrium due to information frictions, the equilibrium incentives are stronger in the material rewards setting.
With these equilibrium characterizations in hand, we can turn to our main topic of interest: the differential efficacy of repression against materially versus psychologically motivated rebel groups and its empirical implications. But, before doing so it is worth commenting on some features of our model.
Comments on the Model
Several natural questions arise from our basic setup. The first is what happens if people are motivated by some mix of psychological and material motivations. We analyze this question in Online Appendix A, showing that the results in the mixed case lie in between the results for the pure material and pure psychological cases we consider in the main text.
The second is about the robustness of results to the assumption of an improper uniform prior. We make that assumption, which is standard in the global games literature (Morris and Shadmehr Reference Morris and Shadmehr2017), to introduce strategic uncertainty while maintaining tractability that allows us to focus on the question of interest—the interactions of different motivation types and state repression. We are not focused on the effects of information per se. That said, in Online Appendix B, we show that the results are robust to other informational assumptions. In particular, we show that the same results obtain for any smooth prior in the limit when the noise becomes vanishingly small. We then provide numerical examples for a standard normal prior for the case of both a uniform distribution of noise and a standard normal distribution of noise. Finally, we provide additional numerical examples for the effect of a public signal about the regime strength (
$ \theta $
) in both settings with psychological and material rewards.
The third is about whether our results are sensitive to the normalization that total material and psychological rewards are equal at full participation and that, therefore, individual psychological rewards are less than individual material rewards for less than full participation. To address this concern, in Online Appendix C we show the robustness of our results to a variant of the model where individual material rewards are given by
$ k\frac{a}{m}, $
for
$ k>0 $
and
$ \frac{c}{k}\in \left(0,1\right) $
. Finally, it is worth commenting on a few other features of payoffs in our model.
We have assumed that rewards are contingent on participating. This is distinct from rewards that are gained by every citizen if the regime falls—in the language of Olson (Reference Olson1965) and Tullock (Reference Tullock1971), our rewards are selective/private benefits. As we argued extensively in the Introduction, we think the idea of participation-contingent rewards is substantively appropriate in both our material and psychological rewards settings. But it is also worth noting that our results are robust to adding rewards that are not participation contingent. In particular, as our model has a continuum of individuals, each individual regards their personal contribution to the probability of success as negligible. This implies that any reward (or cost) that does not depend on whether a person participates cannot affect that person’s participation decisions. Thus, introducing additional rewards that are not contingent on participation would not alter our results.
It is also worth noting that we do not directly include costs that a citizen might suffer should she fail to participate in a rebellion that ultimately succeeds. Such costs are, of course, quite substantively plausible. But, notice, success-contingent costs associated with not participating are mathematically equivalent (with opposite sign) to success-contingent benefits associated with participating. So our model captures the substantive effects of such costs, without adding an additional parameter to directly represent them.
EFFICACY OF REPRESSION
In this section, we ask how the efficacy of repression differs when deployed against groups with material versus psychological motivations. For this analysis, we continue to treat repression as a parameter, examining its efficacy through a comparative static analysis. In the next section, we leverage these results to characterize the level of repression chosen by a strategic government.
We represent the idea of an increase in repression with an increase in the cost of rebellion, c. This corresponds to the standard conception of state repression as any action by the state “which raises the contender’s cost of collective action” (Tilly Reference Tilly1978, 100; Davenport Reference Davenport2007; Earl Reference Earl2011). Of course, repression may raise both a citizen’s direct costs of rebelling and a citizen’s direct benefit from rebelling due to a sense of injustice or a desire for vengeance (Aytaç and Stokes Reference Aytaç and Stokes2019; Davenport Reference Davenport2007; Earl Reference Earl2011; Lawrence Reference Lawrence2017; Pearlman Reference Pearlman2018; Shadmehr and Boleslavsky Reference Shadmehr and Boleslavsky2022; Siegel Reference Siegel2011; Wood Reference Wood2003). In our model, the cost c is, in fact, the ratio of the costs to benefits of rebellion. Representing increased repression with an increase in c means that even though both the numerator and the denominator may increase, we assume the direct cost–benefit ratio is increasing. This is consistent with the standard view of higher repression as reducing political opportunities (Davenport Reference Davenport2007; Earl Reference Earl2011; McAdam Reference McAdam1999; Tarrow Reference Tarrow2011; Tilly Reference Tilly1978; Reference Tilly2006).
Proposition 3. The equilibrium regime change threshold is less responsive to repression in the material rewards setting than in the psychological rewards setting:
$ \frac{\partial {\theta}^p}{\partial c}<\frac{\partial {\theta}^m}{\partial c}<0 $
.
Proposition 3 shows how the likelihood of success in differently motivated groups responds to variations in repression levels. The direct effect of higher rebellion costs is to reduce incentives to protest in both settings. But there is also a strategic effect: a citizen recognizes that higher costs of mobilization mean that others have less incentive to rebel and adjusts her behavior accordingly. In the psychological rewards setting, this further reduces the incentive to rebel because the likelihood of success is lower. This strategic effect is weaker in the material rewards setting and may offset parts of the direct effect (if actions are strategic substitutes at equilibrium). The reason is that even though the likelihood of success is lower, the size of the rebellion is also smaller so that if the rebellion succeeds each participant receives a larger reward. Due to this strategic effect, the likelihood of success in the material rewards setting is less sensitive to increases in the direct costs of rebellion. Thus, repression is less effective against groups whose members are materially motivated.
It is also worth noting that, because we model repression as increasing the costs of mobilization, our results apply to any change in the world that affects these costs, whether due to repression or otherwise. For instance, the model predicts that a positive economic change that increases wages and, thus, the opportunity costs of mobilization has the same effects as repression—reducing mobilization. Moreover, just like repression, the effects of economic shocks will be heterogeneous so that, perhaps counterintuitively, economic shocks have a bigger effect on mobilization among psychologically motivated rebels than among materially motivated rebels.
THE GOVERNMENT’S REPRESSION DECISION
In this section, we consider a government choosing how much to invest in repression. As Balcells and Stanton (Reference Balcells and Stanton2021) highlight, not all instances of government repression and violence are intentional (as opposed to collateral damage) or even a matter of policy (as opposed to practice [Wood Reference Wood2018]). However, intentional government policies of repression constitute an important category of repression and violence (Balcells and Stanton Reference Balcells and Stanton2021; Davenport Reference Davenport1995; Reference Davenport2007; Earl Reference Earl2011), and our analysis focuses on this type of state repression.
To study the government’s decision, we add an earlier stage to our base model, in which the government chooses a level of repressive capacity, c, prior to information being revealed about regime strength.Footnote 9 The government cares about two things: it wants to reduce the risk of a successful rebellion and it bears direct costs for engaging in repression. In particular, the government’s objective is to minimize a combination of the regime change threshold and the costs of investment in repressive capacity:

where
$ C(0)\hskip0.20em =\hskip0.20em {C}^{\prime }(0)\hskip0.20em = 0 $
,
$ {C}^{\prime }(1)\hskip-0.1em >\hskip-0.1em 1 $
, and
$ 0<{C}^{\prime }(c),\hskip0.20em {C}^{{\prime\prime} }(c) $
for all
$ c>0 $
, captures the costs of a repression level c for the government.Footnote
10
Let
$ {c}^j $
,
$ j\hskip0.35em =\hskip0.35em p,\hskip0.35em m $
, be the regime’s choice of repression when the rebels are psychologically and materially motivated, respectively.
Proposition 4. The government chooses a higher repression level against psychologically motivated groups than against materially motivated groups:
$ {c}^p>{c}^m $
. Moreover, in equilibrium with the endogenous choice of government repression, the equilibrium regime change threshold is higher in the material rewards setting than in the psychological rewards setting:
$ {\theta}^p\left({c}^p\right)<{\theta}^m\left({c}^m\right) $
.
Proposition 4 says two things. First, all else equal, a strategic government engages in more repression against a psychologically motivated rebel group than against a materially motivated rebel group. Second, even with endogenous repression, psychologically motivated groups are less likely to succeed than are materially motivated groups.
The intuition builds on the earlier analysis. We showed that higher repression has a larger marginal effect on reducing the probability of successful rebellion when motivations are psychological rather than material (Proposition 3). This implies that the government obtains a larger marginal benefit from applying repression against psychologically motivated groups than against materially motivated groups. As a result, the government represses psychologically motivated groups more (
$ {c}^p>{c}^m $
).
Moreover, for a given (exogenous) level of repression, the equilibrium regime change threshold is higher in the material rewards setting than in the psychological rewards settings (Proposition 2). That is, all else equal, psychologically motivated groups are less likely to succeed in overturning the status quo than are materially motivated groups. Accounting for the government’s strategic choice of repression reinforces this result because, as we just discussed, states use more repression against psychologically motivated groups.
DISCUSSION AND EMPIRICAL IMPLICATIONS
We have established four primary results:
-
1. Strategic governments repress psychologically motivated groups more than materially motivated groups (Proposition 4).
-
2. All else equal (e.g., repression held constant), psychologically motivated groups are less effective at achieving their goals than are materially motivated groups (Proposition 2).
-
3. Repression has a larger marginal effect on the efficacy of psychologically motivated groups than of materially motivated groups. (Proposition 3).
-
4. At the equilibrium level of repression chosen by governments, psychologically motivated groups are less effective at achieving their goals than are materially motivated groups (Proposition 4).
Each of these is an empirical implication in its own right. Thus, the model yields testable hypotheses about both the amount of repression we should expect to see used against different types of rebel groups (implication 1), the efficacy of that repression (implication 3), and the likelihood of success of differentially motivated rebel movements (implications 2 and 4). Moreover, as discussed earlier, the model also has implications about the (heterogeneous) effects of economic or other nonrepressive changes that affect the costs of mobilization. Nonrepressive changes in the opportunity costs of mobilizing (e.g., increases in economic opportunity) have the same effect in the model as does repression; thus, the model also implies that positive economic shocks will decrease rebel efficacy and that the effect of such shocks is greater on psychologically motivated groups than on materially motivated groups.
In addition to these positive implications, the model also highlights some complications for empirically estimating the effect of repression, which may shed light on why the empirical literature finds conflicting and varied results—what Davenport (Reference Davenport2007) refers to as the punishment puzzle. Here we consider three such complications.
The first complication has to do with the way rebel motivations complicate causal inference. Consider, for instance, a regression of either mobilization or rebel success on repression. Points 1 and 2, together, show that motivations are a confounder in such a regression. Holding repression fixed, materially motivated groups have higher levels of mobilization and are more likely to succeed than are psychologically motivated groups. But they also face less repression than psychologically motivated groups. Thus, those regressions will return correlations that are overestimates of the efficacy of repression—some of the negative correlation between the measure of repression and that of protest success is due to systematic baseline differences in mobilization or likelihood of success among rebel groups that face more or less repression, not due to the effect of the repression itself.
A theoretical version of this observation is illustrated in Figure 2. The figure shows the equilibrium regime change threshold on the vertical axis and the equilibrium level of repression on the horizontal axis. The higher the regime change threshold, the more likely the rebel group is to succeed. In the figure, all psychologically motivated groups face repression
$ {c}^p $
and all materially motivated groups face repression
$ {c}^m $
. But, for any given level of repression, psychologically motivated groups also face a lower regime change threshold (i.e., are less likely to succeed). Thus, motivation is correlated with both repression and likelihood of success and, so, is a confounder if we want to interpret the correlation between repression and success as an estimate of the causal effect of repression.

Figure 2. Regime Change Threshold as a Function of Repression
Note: Some of the observed correlation between rebel success and repression is the result of baseline differences coming from the correlation between motivations and repression rather than the causal effect of repression.
Of course, in real data, other parameter values will vary, so not all conflicts with the same motivations will experience the same level of repression or the same regime change threshold. Figure 3 illustrates what such data might look like. The data in the figure are generated as follows. For each type of motivation, the level of repression in any given observation is the equilibrium level plus mean-zero noise. And the regime change threshold is the equilibrium regime change threshold from the model, given that level of repression and the type of motivations, plus (independent) mean-zero noise.

Figure 3. Simulated Data Based on the Theoretical Model
Note: Data simulated from the model show that motivation is a confounder in the regression of the regime change threshold on repression and that repression has heterogeneous treatment effects.
The problem of confounding is evident in the figure. Both the outcome (regime change threshold) and treatment (level of repression) are correlated with motivations (represented by the different colored data points). In conflicts with psychologically motivated rebels (black dots), repression tends to be high and the regime change threshold tends to be low. In conflicts with materially motivated rebels (gray dots), repression tends to be low and the regime change threshold tends to be high. The resulting bias can be seen directly in the left-hand panel of the figure. The dashed line represents the simple regression of regime change threshold on repression—its slope estimates the correlation. The solid lines represent the regression of regime change threshold on repression and a dummy variable for the two possible types of rebel motivation. Because this regression controls for motivation and there are no other confounders, the slope of these lines (which are parallel by construction) estimates the average treatment effect of repression.Footnote 11 The solid lines are shallower than the dashed line. This means that the correlation overestimates (in magnitude) the average treatment effect.
A second complication concerns heterogeneous treatment effects. Suppose an empirical study credibly estimates the effect of repression for some set of cases. Result (3) says that repression has heterogeneous treatment effects on rebellion, depending on rebel motivations. In particular, repression has a bigger effect when rebels are psychologically motivated than when they are materially motivated. This is evident in the right-hand panel of Figure 3. In that figure, the lines represent the regression of regime change threshold on repression for each type of conflict separately. As there are no other confounders in our simulations, the slopes of these regression lines estimate the average treatment effect of repression in each type of conflict. The regression line for psychologically motivated conflicts is steeper than the regression line for materially motivated conflicts, reflecting the heterogeneous treatment effects. Thus, estimates of the efficacy of repression will be sensitive to the sample of conflicts being studied. That is, a study that happens to focus on a region of the world or type of conflict where, for instance, most groups are psychologically motivated is expected to get a different estimate of the effects of repression than a study that happens to focus on a region of the world or type of conflict where most groups are materially motivated.
The third complication is more methodological. Many of the most convincing empirical studies of the efficacy of repression employ a difference-in-difference design—for example, comparing changes in rebel activity in locations within a country that did and did not experience repression within a given period (e.g., Benmelech, Berrebi, and Klor Reference Benmelech, Berrebi and Klor2015; Condra and Shapiro Reference Condra and Shapiro2012; Dell and Querubin Reference Dell and Querubin2018; Kocher, Pepinsky, and Kalyvas Reference Kocher, Pepinsky and Kalyvas2011; Lyall Reference Lyall2009; Rozenas and Zhukov Reference Rozenas and Zhukov2019). In such studies, repression is typically relatively rare; that is, there is not repression in most regions during most periods. That implies that, in a within-country design, most units are “untreated” during most periods. But our result (1) suggests this will be less true for conflicts involving psychologically motivated groups—in those conflicts, the untreated and treated groups will be more evenly balanced because repression is used more often. In a finite sample, this means that (all else being equal), estimates of the efficacy of repression will be more precise in settings with psychologically motivated groups than in settings with materially motivated groups. Thus, we are more likely to find statistically significant evidence of the efficacy of repression in psychologically motivated conflicts not only because it is in fact more effective in such conflicts but also because it is more precisely estimated. And, moreover, a meta-analysis (whether formal or implicit from a reading of the literature) that weights estimates by the inverse of their precision will overestimate the average efficacy of repression because it will put excess weight on estimates from conflicts with psychologically motivated groups which, according to result (3), are the settings where repression is most effective.
EXTENSION: RESILIENCE TO REPRESSION AND THE COMMITTED CORE
Our paper focuses on the strategic interactions between motivations in collective action and the choice of repression by the state. However, to show broader applications and the flexibility of our framework, we now extend the base model to consider the resiliency of rebel groups to early setbacks. In particular, we now turn to a dynamic question: which type of movement is more resilient to repression in the long run given that even successful repression often leaves behind a committed core that will attempt to resurrect the movement in the future?
Many movements do not succeed or fail in a single episode. A movement that initially appears to have been defeated may resurface later when another political opportunity arises (McAdam, Tarrow, and Tilly Reference McAdam, Tarrow and Tilly2001; Tarrow Reference Tarrow2011). Moreover, in such instances, the experience of earlier repression often creates a core of deeply committed participants. For instance, Rasler (Reference Rasler1996) highlights how, in the short run, government repression appears to have succeeded in putting down the protests that preceded the Iranian revolution but that in the longer run the movement inspired by these protests rose back up on the foundation built by the committed core. Wood (Reference Wood2003) and Lawrence (Reference Lawrence2017) emphasize how the desire for justice or vengeance can create such a committed core, focusing on the cases of El Salvador and Morocco, respectively. Bursztyn et al. (Reference Bursztyn, Cantoni, Yang, Yuchtman and Zhang2021) and Pearlman (Reference Pearlman2021) highlight the ways in which social ties can contribute to the creation of a committed core, with a focus on the cases of Hong Kong and Syria, respectively (see also Diani and McAdam Reference Diani and McAdam2003).
To study how early failure that leaves a committed core in place affects the ultimate likelihood of success, we extend the model to two periods and normalize the population size to 1. To study these dynamics, we abstract away from endogenous repression, although it is straightforward to add it along the lines of the analysis above. The stage game in the first period is identical to the previous setting. If the rebellion succeeds, the game ends. However, if the rebellion fails in the first period, in the second period citizens again play a similar regime change game. However, now there is a committed core: a fraction
$ 1-a\in \left(0,1\right) $
of citizens who will surely participate in the second-period rebellion. Thus, there are two differences between periods 1 and 2: in the second period, a fraction 1 – a of citizens are committed to the rebellion and citizens have the additional common knowledge that the regime survived the first period. To ease exposition, we focus on normal distributions of noise so that
$ F\hskip0.35em =\hskip0.35em N\left(0,1\right) $
.
In period 2, each citizen has three pieces of information: her signal from the first period, her signal from the second period, and the fact that the regime has survived. Because conditional expectations of normally distributed variables are linear, a citizen’s private information in period 2 is effectively the average of her private signals in periods 1 and 2. Let
$ {x}_2 $
be that average. We refer to this average signal as a citizen’s private signal in period 2.
As before, we focus on symmetric monotone equilibria. In period
$ 1 $
, a citizen rebels if and only if her private signal is below a threshold
$ {x}_1^{\ast } $
. In period 2, a fraction 1 – a of citizens will be committed and rebel and a citizen from the remaining group rebels if and only if her private signal
$ {x}_2 $
is below a threshold
$ {x}_2^{\ast } $
. As in the static setting, there is no equilibrium in which a citizen always revolts:
$ {x}_t^{\ast }<\infty $
. If the regime survives the first period, this implies
$ \theta \ge 0\hskip-0.1em $
. Thus, there could be an equilibrium in which
$ {x}_2^{\ast}\hskip0.35em =\hskip0.35em -\infty $
and only the fraction 1 – a of (committed) citizens rebel. We focus on the interesting case of finite cutoff equilibria, so that
$ {x}_2^{\ast}\in \mathbb{R} $
.
Because a single citizen’s action does not change the outcome (each individual is too small to make a nonnegligible difference), in the first period the equilibrium behavior of citizens is the same as in the static game. Let
$ {\theta}_t^j $
,
$ j\in \left\{p,m\right\} $
, and
$ t\hskip0.35em =\hskip0.35em 1,\hskip0.2em 2 $
be the period t equilibrium regime change threshold in the settings with psychological (
$ j\hskip0.35em =\hskip0.35em p $
) and material (
$ j\hskip0.35em =\hskip0.35em m $
) rewards, respectively. Let
$ {x}_t^p $
and
$ {x}_t^m $
be the corresponding equilibrium citizen cutoffs. From our earlier analysis,
$ {\theta}_1^p\hskip0.35em =\hskip0.35em 1-\hskip0.1em c $
and
$ {\theta}_1^m\hskip0.35em =\hskip0.35em {e}^{-c} $
. In the second period, in the setting with material rewards, any pair of cutoffs
$ \left({\theta}_2^m,{x}_2^m\right) $
that satisfies the following belief consistency and indifference conditions constitutes an equilibrium:


In the second period, in the setting with psychological rewards, any pair
$ \left({\theta}_2^p,{x}_2^p\right) $
that satisfies the following conditions constitutes an equilibrium:


These equilibrium conditions reflect the two differences between period 2 and period 1. The information content of the regime’s survival is reflected in conditioning on
$ \theta >{\theta}_1^j $
,
$ j\in \left\{p,m\right\} $
, in the indifference conditions. The emergence of a committed rebel core is represented by the term 1 – a in the belief consistency conditions.
It is bad news for the rebels that the regime survived the first period: they’ve learned that
$ \Pr (\theta <{\theta}_1^j)\hskip0.35em =\hskip0.35em 0 $
,
$ j\in \left\{p,m\right\} $
. This may prevent rebellion in period 2 altogether (finite-cutoff equilibria may not exist). But if citizens’ private information is sufficiently precise, they effectively discard the relatively imprecise information that
$ \theta \ge {\theta}_1^j $
,
$ j\in \left\{p,m\right\} $
: compared with their precise private information, this public information receives little weight in their Bayesian updating. Cross-period informational linkages have been studied elsewhere in the literature (Angeletos, Hellwig, and Pavan Reference Angeletos, Hellwig and Pavan2007). To focus on the new insight that the effect of a committed core depends on motivations, in our theoretical results, we abstract away from the informational linkage across periods—letting the noise in the second period’s private signals become vanishingly small. We then provide numerical examples with information linkages (i.e., learning) between periods and discuss the effect of informational linkage on the dynamic.Footnote
12
Proposition 5. Suppose the noise in the second period’s private signals becomes vanishingly small, and we focus on the largest equilibrium. Conditional on failure in the first period, the chances of success is higher in the psychological rewards setting than in the material rewards setting.
The logic is as follows. A failure in the first attempt creates a committed core. This group of committed participants increases the likelihood of success in both settings, but the effect is weaker in the material rewards setting because, conditional on success, the group that will share the rewards is surely larger than the size of the committed members. Combining Propositions 2 and 5 implies that movements with psychological rewards are less likely to succeed in the first period, but conditional on a failure in the first period, they are more likely to succeed than are movements with material rewards. This result resonates with the finding in Shadmehr and Bernhardt (Reference Shadmehr and Bernhardt2019) that it is more difficult for a movement to begin organically (without a revolutionary vanguard), but movements that begin organically are more likely to succeed.
In this analysis, because citizens have very precise private information in the second period, they do not need to rely on the informational content of the failure in the first period. Consequently, similar results hold if the regime’s strength is independent across periods, an assumption that fits settings in which sufficient time has passed since the first revolt. But suppose there are genuine informational linkages across periods; can our findings continue to hold?
To see the forces at work once information linkages are reintroduced, recall that regime change is more likely in the material rewards setting in the first period (
$ {\theta}_1^m>{\theta}_1^p $
). Therefore, upon observing that the regime has survived the rebellion, citizens in the material rewards settings infer that the regime is stronger than citizens in the psychological rewards settings do. That is, the direct informational effect of early failures makes citizens more pessimistic about the likelihood of success in the material rewards settings than in the psychological rewards setting, reinforcing the effect of committed core. Thus, there is reason for optimism that the overall finding that rebellions characterized by psychological motivations will be more resilient following early failures is robust. Below we show computational results consistent with that intuition.
Lemma 1 in the proof of Proposition 5 shows how equilibrium regime change thresholds can be calculated away from the limit of vanishingly small noise. Suppose
$ a\hskip0.35em =\hskip0.35em 0.8 $
and
$ c\hskip0.35em =\hskip0.35em 0.2 $
so that
$ {\theta}_1^p\hskip0.35em =\hskip0.35em 1-c\hskip0.45em =\hskip0.35em 0.8 $
and
$ {\theta}_1^m\hskip0.35em =\hskip0.35em {e}^{-c}\hskip0.70em \approx \hskip0.70em 0.82 $
. Moreover, suppose the noise is normally distributed with the standard deviation
$ \sigma $
. When
$ \sigma \hskip0.35em =\hskip0.35em 0.01 $
, we have:
$ {\theta}_2^p\hskip0.35em \approx \hskip0.35em 0.84 $
and
$ {\theta}_2^m\hskip0.35em \approx \hskip0.35em 0.85 $
. Thus, consistent with Proposition 5,
$ {\theta}_2^p-\hskip0.1em {\theta}_1^p\hskip0.35em \approx \hskip0.35em 0.04>{\theta}_2^m-\hskip0.1em {\theta}_1^m\hskip0.35em \approx \hskip0.35em 0.03 $
—the presence of a committed core has a larger effect in the setting with psychological rewards. Conditional on failure in the first period, the chance of success is higher in the psychological rewards setting than in the material rewards setting.
Now, suppose we increase the noise to
$ \sigma \hskip0.35em =\hskip0.35em 0.02 $
, further moving away from the limiting case of vanishingly small noise. We still have
$ {\theta}_1^p\hskip0.35em =\hskip0.35em 1-\hskip0.1em c\hskip0.35em =\hskip0.35em 0.8 $
and
$ {\theta}_1^m\hskip0.35em =\hskip0.35em {e}^{-c}\hskip0.35em \approx \hskip0.35em 0.82 $
. In the second period of the psychological rewards setting, we have
$ {\theta}_2^p\hskip0.35em \approx \hskip0.35em 0.838>{\theta}_1^p $
and the revolution might succeed in the second period after failing in the first period. By contrast, in the second period of the material rewards setting, the likelihood of success is 0. This case is a stark example of our finding that conditional on failure in the first period, the chance of success is higher in the psychological rewards setting than in the material rewards setting.
Our findings also do not depend critically on the assumption of a uniform prior. To see this, consider an example with a normal prior about
$ \theta $
.Footnote
13 Suppose that
$ a\hskip0.35em =\hskip0.35em 0.6 $
,
$ c\hskip0.35em =\hskip0.35em 0.7 $
, and θ ~ N(0,2) and that noise is independent and identically distributed across citizens and periods according to
$ N\left(\mathrm{0,0.25}\right) $
. Now,
$ {\theta}_1^p\hskip0.35em \approx \hskip0.35em 0.305 $
and
$ {\theta}_1^m\hskip0.35em \approx \hskip0.35em 0.505 $
. As in our previous example, there is still a finite threshold in the psychological motivations setting,
$ {\theta}_2^p\hskip0.35em \approx \hskip0.35em 0.511 $
, and a positive probability of the revolution succeeding in the second period. But in the material motivations case, the revolution will surely fail in the second period, again providing a stark illustration of our result. Thus, in all these examples, allowing for an information linkage across periods strengthens the result that conditional on failure in the first period, the chance of success is higher in the psychological rewards setting than in the material rewards setting.
It is worth relating this dynamic extension of our model to two strains of the literature.
First, we distinguish our approach to modeling the dynamics of rebellion and repression from that of Tsebelis and Sprague (Reference Tsebelis and Sprague1989) and Francisco (Reference Francisco2009). Those analyses adopt phenomenological models of predator–prey from mathematical biology. Tsebelis and Sprague (Reference Tsebelis and Sprague1989) posit that revolutionary activity R and state repression C follow two linear differential equations
$ dR/ dt\hskip0.35em =\hskip0.35em -{a}_1R+{b}_1C+{d}_1,\hskip0.2em dC/ dt\hskip0.35em =\hskip0.35em {a}_2R\hskip0.1em -\hskip0.1em {b}_2C+{d}_2 $
, where
$ {a}_i,\hskip0.2em {b}_i,\hskip0.2em {d}_i $
, and
$ i\hskip0.35em =1,\hskip0.2em 2 $
are constants. Francisco (Reference Francisco2009) considers the nonlinear Lotka-Volterra equations, with interaction terms
$ R\times C $
instead of C. Methodologically, they focus on phenomenological modeling in which aggregate behavior is assumed to follow a form of differential equation. In contrast, in our approach, actors make intentional decisions to achieve their goals given their constraints. As Tsebelis and Sprague (Reference Tsebelis and Sprague1989) argue, their approach “alienates strategic choices by historical actors from the process in which they participate in favor of some impersonal logic of revolutionary processes” (555–6). Thus, although we focus on strategic interactions, “agency is immaterial to the formalism” (Tsebelis and Sprague Reference Tsebelis and Sprague1989, 555) that they use. Substantively, our models share the feature that, all else equal, earlier mobilization facilitates later mobilization. The size of this effect as well as the effect of repression are captured by the constants of their equations. Thus, although variations in motivation, strategic interactions, and endogenous choices of mobilization and repression are collapsed in those constants in their models, we attempted to study these features.
Second, we compare the role played by the committed core in our model with that played by revolutionary vanguards in models of rebellion. Our model of the committed core is most similar to models of vanguards as “early risers”—antigovernment activists who come to the streets early to inspire regime change (Kuran Reference Kuran1991; Lohmann Reference Lohmann1994; Shadmehr and Bernhardt Reference Shadmehr and Bernhardt2019; Tarrow Reference Tarrow2011). Indeed, this is precisely the effect of our committed core in the version of our model with psychological motivations. However, as the analysis above shows, the effects of the committed core are different with material incentives. In particular, the presence of congestion externalities creates offsetting effects—a committed core makes victory more likely but reduces the expected rewards of such a victory. These competing effects have not previously been discussed in the literature on vanguards.
CONCLUSION
We explored the interaction between rebel motivations, rebel mobilization, and government repression. We showed that movements with material rewards are less affected by repression and face less repression, whereas movements with psychological rewards are more resilient to repressive efforts that result in early failures but leave behind a committed core. A primary ingredient of the underlying logic is the rivalry of material rewards versus the nonrivalry of psychological rewards—the other main ingredient is strategic uncertainty, as Propositions 1 and 2 reveal. The effects of these motivations are complicated by the presence of coordination concerns among citizens. We show that, because material rewards must be shared upon success, repression has less influence on movements with material motives: such changes decrease the likelihood of success, but by decreasing participation they also increase the rewards of success in movements with material motives. The model yielded both positive empirical predictions and results about confounding and heterogeneous treatment effects that may help reveal why empirical studies find inconsistent results.
In addition to these empirical implications, these insights have policy implications. Policy makers should be more concerned about the existence of a committed core or vanguard when confronting movements with psychological and ideological motives rather than material motives. And, for this reason they should be more cautious about the long-run efficacy of early victories when facing a group whose members are psychologically or ideologically motivated, as they are more resilient to such early failures than are movements using primarily material motives. By contrast, policy makers should recognize that movements motivated by material reward are more resilient to repression. Combining these observations suggests the optimal policy that seeks to reduce the chances of success are qualitatively different across movements whose members are differentially motivated. When dealing with movements whose members are motivated by psychological or ideological rewards, the focus should be on increasing the costs of participation. In contrast, in dealing with movements whose members are materially motivated, it might be more effective to focus on reducing the material rewards that participants hope to gain should the movement succeed.
Our analysis also suggests avenues for future research. First, we introduced incomplete information into our model as a form of equilibrium selection, which allowed us to focus on how the efficacy of different types of repression depends on motivations. But we largely abstracted away from the substantive effects of information itself. Future work might explore how different sources of uncertainty interact with motivations and repression. Second, our results suggest hypotheses about the heterogeneous treatment effects of both repression and economic shocks that have not been investigated but which we hope will motivate future empirical work.
SUPPLEMENTARY MATERIALS
To view supplementary material for this article, please visit http://doi.org/10.1017/S0003055422000600.
DATA AVAILABILITY STATEMENT
Research documentation and data that support the findings in this study are openly available at the American Political Science Review Dataverse: https://doi.org/10.7910/DVN/TE9U5S.
ACKNOWLEDGMENTS
We received helpful feedback from Dan Bernhard, Anthony Fowler, and Adam Zelizer.
CONFLICT OF INTEREST
The authors declare no ethical issues or conflicts of interest in this research.
ETHICAL STANDARDS
The authors affirm this research did not involve human subjects.
APPENDIX: PROOFS
Proof of Proposition 1: When
$ \theta <1 $
, the regime collapses if almost all citizens rebel so that
$ m\hskip0.35em =\hskip0.35em a $
. Thus, when
$ \theta <1 $
, a citizen rebels if she believes that almost all others will rebel, because her payoff from rebelling will be
$ 1-c>0 $
. When
$ \theta \ge 0 $
, the regime survives if almost no citizen rebels so that
$ m\hskip0.35em =\hskip0.35em 0 $
. Thus, when
$ \theta \ge 0 $
, a citizen does not rebel if she believes that almost no other citizen will rebel, because her payoff from rebelling will be
$ -c<0 $
.
Proof of Proposition 2: We look for symmetric monotone equilibria in which a citizen rebels if and only if her signal is below a finite threshold
$ {x}_i<{x}^m $
. A monotone strategy implies that the regime collapses if and only if
$ \theta <{\theta}^m $
, where

A citizen with signal
$ {x}_i $
rebels if and only if her expected payoff from rebellion exceeds its costs:

If a symmetric finite threshold equilibrium exists, the marginal citizen who receives the threshold signal
$ {x}_i\hskip0.35em =\hskip0.35em {x}^m $
must be indifferent between rebelling or not. Moreover, as Shadmehr (Reference Shadmehr2019b, Lemma 3) shows, when there is no prior information about
$ \theta $
, the marginal citizen believes that the size of the rebellion is uniformly distributed. Thus, the marginal citizen’s expected payoff from rebellion and the indifference condition become

Combining this with (7) yields
$ -\log \hskip-0.1em \left({\theta}^m\right)\hskip0.35em =\hskip0.35em c $
, so that the unique equilibrium regime change threshold is

Alternatively, let
$ z(\hat{\theta})\hskip0.35em =\hskip0.35em \Pr (\theta <\hat{\theta}|{x}_i\hskip0.35em =\hskip0.35em {x}^m) $
, with
$ {z}^m\hskip0.35em =\hskip0.35em \Pr \left(\theta <{\theta}^m|{x}_i\hskip0.35em =\hskip0.35em {x}^m\right) $
. Because there is no prior information about
$ \theta $
:
$ \Pr \left(\theta <{\theta}^m|{x}_i\hskip0.35em =\hskip0.35em {x}^m\right)\hskip0.35em =\hskip0.35em 1-\Pr \left({x}_i<{x}^m|\theta \hskip0.35em =\hskip0.35em {\theta}^m\right) $
. Thus,
$ 1-{z}^m\hskip0.35em =\hskip0.35em {\theta}^m $
. Moreover, the left-hand side of the inequality (8) can be rewritten in terms of z so that the indifference condition becomes

Because
$ 1-{z}^m\hskip0.35em =\hskip0.35em {\theta}^m $
, this shows
$ {\theta}^m\hskip0.35em =\hskip0.35em {e}^{-c} $
.
It remains to show that the best response to a monotone strategy is also monotone. Let
$ \pi \left(\theta \right)\hskip0.35em =\hskip0.35em {\mathbf{1}}_{\left\{\theta <{\theta}^m\right\}}\cdot \frac{1}{\Pr \left({x}_j\hskip0.1em <\hskip0.1em {x}^m|\theta \right)}-c $
. If
$ c\in \left(0,1\right) $
, then
$ \pi \left(\theta \right) $
has a single-crossing property. Because
$ \left(x,\theta \right) $
satisfies the monotone likelihood ration property, by Karlin’s theorem (Shadmehr Reference Shadmehr2019b, Online Appendix), single-crossing property holds under the integral transformation in (8) and the best response to a monotone strategy is also monotone. This means that the marginal citizen with signal
$ {x}_i\hskip0.35em =\hskip0.35em {x}^m $
must be indifferent between rebelling or not.
Proof of Proposition 3: From Proposition 2,

where the inequality follows from
$ {e}^c>1 $
for
$ c>0 $
.
Proof of Proposition 4: From Proposition 2, the government’s marginal benefit of raising repression level c is (weakly) decreasing in both settings, with
$ \frac{\partial {\theta}^p}{\partial c}<\frac{\partial {\theta}^m}{\partial c}<0 $
. Thus, the government’s optimal repression level is interior, and
$ {c}^p>{c}^m $
. Moreover, from Proposition 2,
$ {\theta}^p(c)<{\theta}^m(c) $
. Thus,
$ {\theta}^p\left({c}^p\right)<{\theta}^p\left({c}^m\right)<{\theta}^m\left({c}^m\right) $
.
Proof of Proposition 5: First, we prove a lemma that characterizes finite cutoff equilibria.
Lemma 1
$ {\theta}_2^m>\max \left\{{\theta}_1^m,1-a\right\} $
is an equilibrium regime change threshold of the material rewards setting if and only if it satisfies

and
$ {\theta}_2^p>\max \left\{{\theta}_1^p,1-a\right\} $
is an equilibrium regime change threshold of the psychological rewards setting if and only if it satisfies

Proof of Lemma 1: We first consider the material rewards setting. From Equation 4, the marginal citizen’s net expected payoff from rebellion is

Thus,

where the last equality follow from Equation 3. Substituting from (13) into (4) yields

Substituting
$ {x}_2^m $
from (3) into (14) yields

Similarly for the psychological rewards setting, from Equation 6, the marginal citizen’s net expected payoff from rebellion is

Thus, any
$ \left({\theta}_2^p,{x}_2^p\right) $
that satisfies the following equations constitutes an equilibrium.

Substituting
$ {x}_2^p $
from the indifference condition into the belief consistency yields

Lemma 2

Proof of Lemma 2: When
$ {\theta}_2^m>\max \left\{{\theta}_1^m,1-a\right\} $
, we have

Thus, the right-hand side of (11) approaches
$ {e}^{- ac} $
so that the largest crossing of the 45-degree line approaches
$ {e}^{- ac} $
:

Similarly,

Lemma 2 reflects that when noise in private signals is small, citizens discard their public information. Thus, the informational channel is shut down in the limit. From Lemma 2 and Proposition 2,

And,
$ {\Delta}^m<{\Delta}^p $
if and only if
$ \frac{e^{-c}-{e}^{- ac}}{c- ac}>-1 $
, which is true because
$ {e}^{-x} $
is strictly decreasing and convex with
$ {\left.\frac{de^{-x}}{dx}\right|}_{x\hskip0.35em =\hskip0.35em 0}\hskip0.35em =\hskip0.35em -1 $
.
To calculate conditional probabilities, suppose θ~G, while we recognize that
$ G\hskip0.35em =\hskip0.35em U\left[-l,u\right] $
is a uniform distribution, where
$ l,\hskip0.2em u>0 $
are large. We want to show that

Because G is uniform,

Because the right-hand side is increasing in
$ u $
, it suffices to show that the inequality holds for
$ u\hskip0.35em =\hskip0.35em 1 $
—that is,

which holds for all
$ a,\hskip0.2em c\in \left(0,1\right) $
.
Comments
No Comments have been published for this article.