The postpartum period is a high-risk time for the development of a mental disorder. Depression, anxiety and related disorders (such as obsessive–compulsive disorder or post-traumatic stress disorders) are collectively the most common, with prevalence ranging from 7 to 20%, depending on the sample.Reference Vigod and Stewart1–Reference Falah-Hassani, Shiri and Dennis3 These common postpartum mental health (PMH) disorders can impair activities of daily living such as eating and sleeping and have a negative impact on social and occupational functioning.Reference Howard, Molyneaux, Dennis, Rochat, Stein and Milgrom2 Parent–infant interactions can also be affected, with implications for long-term socioemotional difficulties in children.Reference Yonkers, Simone and Ross4,Reference Alan Stein, Goodman, Rapa, Rahman, McCallum and Howard5 Preventing and treating common PMH disorders is therefore a major public health priority.
Various psychological interventions can prevent common PMH disorders from developing, as can antidepressant use in individuals with a history of the illness.Reference Howard, Molyneaux, Dennis, Rochat, Stein and Milgrom2,Reference Dennis and Dowswell6 These interventions are most effective though among those at high risk; they may be minimally effective or ineffective in prevention in individuals who are at low risk.Reference Howard, Molyneaux, Dennis, Rochat, Stein and Milgrom2 So, the ability to quantify a person's risk for developing a common PMH disorder has clinically actionable implications. For example, an individual-level estimate of risk could help with decisions about when to devote time (and/or financial resources) to psychotherapy, or when to recommend preventive antidepressant use.
Clinical prediction models can facilitate evidence-based clinical decision-making at the point of care.Reference Lee, Bang and Kim7 Many models have an associated points-based risk-scoring system – a clinical risk index – to allow for a rapid individual-level risk assessment.Reference Austin, Lee, D'Agostino and Fine8 A clinical risk index does not aim to predict with absolute certainty whether the future event will occur. Rather, it provides a framework for intervention options to be recommended based on an individual's calculated risk for the outcome.Reference Leslie, Lix, Langsetmo, Berger, Goltzman and Hanley9 Well-known clinical risk indices include the Framingham Risk Score (FRS) for heart disease and the Fracture Risk Assessment Tool (FRAX) for fragility fractures.Reference Sullivan, Massaro and D'Agostino10,Reference McCloskey, Harvey, Johansson, Lorentzon, Liu and Vandenput11 Randomised controlled trials (RCTs) have shown that use of these indices can improve patient outcomes, and they are recommended globally by various clinical practice guidelines.Reference Shepstone, Lenaghan, Cooper, Clarke, Fong-Soe-Khioe and Fordham12
Previous attempts to create risk indices for postpartum depression using key risk factors such as prior psychiatric illness and major stressors such as obstetrical and neonatal complications, financial and marital difficulties and inadequate social supportReference Howard, Molyneaux, Dennis, Rochat, Stein and Milgrom2 have yielded moderate predictive capacity,Reference Nielsen, Videbech, Hedegaard, Dalby and Secher13,Reference Webster, Hall, Somville, Schneider, Turnbull and Smith14 similar to that of indices such as the FRAX and FRS.Reference Sullivan, Massaro and D'Agostino10,Reference McCloskey, Harvey, Johansson, Lorentzon, Liu and Vandenput11 These indices have not been taken up into clinical practice, however, and so have not been examined to determine at what level of risk preventive intervention(s) might improve outcomes. This may be because the variables required to calculate these risk indices include patient self-reported life stressors, social support and subsyndromal depressive symptoms. This means that provider and patient time is required for data acquisition and entry at the point of care. No risk indices have been derived or validated at the population level, none have also been inclusive of other common PMH disorders, such as postpartum anxiety, and none have used only variables that could be automatically extracted from routinely collected information in a health record.
In a population-based cohort in Ontario, Canada's largest province for which data on common PMH disorder risk factors and diagnoses are routinely recorded in health administrative data-sets, we aimed to develop a clinical risk index that could estimate a postpartum person's specific risk for developing a common PMH disorder in the 1 year postpartum using data easily collectable at the time of birth and to conduct an internal validation of the risk index.
Method
Study design and data sources
This population-based cohort study leveraged linked health administrative and clinical registry data collected in Ontario, Canada, between 2012 and 2015. Ontario has a universal publicly funded health insurance system that includes obstetrical care and physician-based mental healthcare for all Ontario residents. Data were analysed at ICES (formerly the Institute for Clinical Evaluative Sciences), an independent non-profit research organisation that holds population-based data to analyse healthcare use and effectiveness in Ontario, and where patient records are de-identified and linked using unique encoded identifiers. ICES data-sets contain complete and accurate sociodemographic information, including age, neighbourhood-level income, immigration status, and physicians’ and hospital-based diagnoses (Supplementary Table 1, available at https://dx.doi.org/10.1192/bjp.2023.74)Reference Juurlink, Croxford, Chong, Austin, Tu and Laupacis15,Reference Williams, Sellors, Grootendorst, Willison, Goel, Anderson, Blacksterin-Hirsch, Fooks and Naylor16 and are linked the Better Outcomes Registry Network (BORN), which uses the standardised BORN Information System (BIS) to collect clinical data on all Ontario births and has built-in checks for validity to verify the data at data entry.Reference Sprague, Dunn, Fell, Harrold, Walker and Kelly17 BIS data for each birth in 2012–2014 are linked to other health administrative data with a success rate of 93%.Reference Fell18
Participants
Postpartum individuals (female based on their provincial health card) who delivered a live infant in or outside of hospital from 1 April 2012 to 31 March 2014 were identified. As the aim of the risk index was to predict a new or recurrent illness (i.e. one that could be prevented with intervention), not to identify those already in treatment for their conditions, those who had received care for any mental illness from a physician during the 2 years before delivery were excluded, as were those with psychotic disorders diagnosed from the earliest available data in the health administrative databases.Reference Kurdyak, Lin, Green and Vigod19 For individuals with multiple deliveries during the study period, we randomly selected one delivery per person to contribute to the cohort, as opposed to selecting the first delivery during the study period, so as to avoid over-selection of primiparous deliveries into the cohort.
Outcome
The primary outcome was a common mental health disorder diagnosed on a visit to a physician in an out-patient or in-patient setting during the first year postpartum.Reference Howard, Molyneaux, Dennis, Rochat, Stein and Milgrom2 Eligible diagnoses were major depressive episodes (including those associated with major depressive disorder and bipolar disorder) and any anxiety or related disorder (e.g. obsessive–compulsive disorder or post-traumatic stress disorder) (Supplementary Table 2). We required: (a) two or more out-patient visits with a diagnosis of an eligible disorder (to avoid ‘rule-out’ visits) or (b) at least one hospital admission or emergency department visit with a diagnosis of an eligible disorder, during the outcome period. The sensitivity and specificity of identifying common mental health disorders in primary care are 80.7% and 97.0% respectively, compared with chart diagnoses (positive and negative predictive values 84.9% and 96.0% respectively).Reference Marrie, Walker, Graff, Lix, Bolton and Nugent20
Risk index variables
All variables were captured either in Ontario health administrative data or in Ontario's standardised antenatal reporting forms that make up part of the BIS data-set, to maximise potential for scale and spread of any risk index created. Variables were grouped as: (a) sociodemographic (maternal age, parity, neighbourhood income quintile, location of residence, primary language, immigration status); (b) pre-pregnancy (e.g. prior psychiatric diagnoses, hospital admissions); (c) pregnancy (smoking, alcohol or substance use during pregnancy, intimate partner violence in pregnancy, prenatal care provider type and number of prenatal visits, pregnancy complications); (d) labour and delivery; and (e) child (sex, number of fetuses, gestational age at birth, size for gestational age, Apgar score, newborn complications, skin-to-skin contact, breastfeeding, newborn taken into care (‘newborn apprehension’) by child welfare services after delivery). Missing data were modelled as levels in variable categories, as applicable. A full list of variables is given in Supplementary Table 3.
Analysis
A split-sample methodology was used to create and then validate the predictive model. The cohort was randomly divided into two groups to create a larger ‘development’ data-set and a smaller ‘validation’ data-set, at a 2:1 ratio.
Model development
We first characterised all potential risk index variables and their relationships with the outcome, using logistic regression to generate odds ratios (OR) and 95% confidence intervals (CI). Next, we developed a predictive model by creating a series of logistic regression models in which potential risk index variables were sequentially added from each of the five variable groups described above.Reference Altman and Royston21,Reference David and Hosmer22 The log likelihood test (−2LL) determined whether added variables increased the model's predictive capacity compared with a simpler version beyond what would be expected with more variables. To build the most parsimonious model, variables were removed if the absolute effect size (parameter estimate) was <0.05 and their removal did not reduce the C-statistic by >0.001 or change the effect size of another covariate by >10%. C-statistics were generated to characterise model discrimination in both the development and validation data-sets.
We conducted two sets of additional analyses to finalise decisions about what model to convert into a risk index. First, replicating procedures from the primary analysis, separate models were built for (a) depressive episodes and (b) anxiety and related disorders. If individual diagnostic models outperformed the main model, we planned to create separate risk indices. Second, to determine whether an empirical machine learning approach would have improved predictive ability over the manual modelling approach, we entered all potential variables into a random forest classifier.Reference Breiman23 If this were to substantially outperform the logistic modelling procedure, this might have additionally supported creating an online automated risk calculator system for jurisdictions where perinatal care data are routinely entered electronically. To conduct this analysis, a random forest classifier utilising the full data-set (development and validation combined, n = 228 134) was created using the randomforest package in R version 3.1.2 for Linux (2014). For each decision tree, observations were randomly sampled with replacement to form a training set (63.2% of the full data-set) and those not selected (out-of-bag sample) were used as a validation set. Predictor variables (Supplementary Table 3) were specified in the same way as in the logistic models, including categories for missing values, except maternal age, which was linear and continuous. The number of variables randomly sampled as candidates at each split in a decision tree was the square root of the number of variables in the data-set, rounded down (e.g. 12 variables in Model 5, containing all 5 variable groups). Trees were grown until the out-of-bag error rate stabilised to within 0.01%, ranging from 600 trees (Model 2) to 760 trees (Model 5). A receiver operating characteristic (ROC) curve and C-statistic (area under ROC curve) were computed for the out-of-bag sample using the prediction and performance functions of the ROCR package in R.
Risk index
Once the final model was established, we converted it into a risk index.Reference Sullivan, Massaro and D'Agostino10 There were several steps involved in this procedure. Using the regression coefficient estimates of the multivariable model, the variables were organised into categories so that we could assign a reference value (midpoints and distributions were examined to generate categorisations for continuous variable; we then calculated the distance of each category of a variable (in regression units) from the reference category. Finally, assigning the reference category a point value of 0, we computed a point value for every category. This conversion of model parameters into integers was done to create a situation where the risk index could be easily computed in multiple settings, including by pen and paper or a simple electronic calculator, and thus be applicable equitably in lower and higher resource settings.
We then generated for each score of the risk index: (a) the probability (or risk) for a common PMH disorder; (b) the expected and observed probabilities of the outcome, which if similar would indicate adequate calibration; and (c) the sensitivity, specificity and positive and negative predictive values if the score were to be used as a screening ‘cut-off’. Although we did not have an external sample in which to conduct validation, we conducted additional cross-validation. As Ontario is a large geographical area with community supports and health services organised regionally, generalisability of the model could differ by region. Socioeconomic status also varies across the Province. We therefore conducted a set of ‘leave-one-out’ analyses by maternal region of residence and income in the validation sample. To do this, we assigned individuals to one of five regions based on the first letter of their postal code (Eastern Ontario, Western Ontario, Northern Ontario, ‘Golden Horseshoe’ wrapping around Lake Ontario, and Toronto, Ontario) and to one of five neighbourhood income quintiles, which are defined by linkage of the first three postal code digits to income data from census files for that neighbourhood. We then conducted ‘leave-one-group-out’ analyses by region and separately by neighbourhood income quintile. For each fold, each listed group was iteratively left out of the model, and the ‘final model’ was fit to the remaining four groups. The fitted model was then used to predict the outcome in the left-out group.
With the exception of the machine learning analayses, all analyses were conducted using SASⓇ version 9.4 for Unix (2014).
Results
From the 280 225 live births in Ontario during the study period, about 4.1% were excluded owing to invalid maternal identifiers for data linkage (n = 11 432), or inability to link maternal to infant data or maternal death before discharge from the delivery hospital admission (n = 76). Of the remaining 268 717, there were 16 846 (6.3%) births excluded owing to lack of Ontario Health Insurance Plan (OHIP) eligibility in the 2 years prior to delivery, which would have precluded measurement of pre-delivery covariates, and 79 (<0.1%) births excluded owing to non-Ontario residency, which would have precluded use of OHIP data to measure the PMH outcome. Additional exclusions were 1365 (0.5%) with severe mental illness at any time prior to index and 16 657 (6.2%) who had received mental healthcare in the 2 years before delivery. For the 5636 pregnancies with multiple deliveries during the study period, we randomly selected one per person, resulting in a final cohort of 228 134 women (n = 152 362 and n = 75 772 randomly assigned to the development and validation samples respectively).
About 6.0% (n = 13 608) of the cohort developed a common PMH disorder, including 3392 (1.5%) with a depressive episode, 11 262 (5.0%) with an anxiety or related disorder and 1046 (0.5%) with both. PMH disorders were usually first diagnosed in the out-patient setting (88.1%), followed by the emergency department (10.3%) or on hospital admission (1.6%). Those with common PMH disorders were slightly younger than those without (mean 29.9, s.d. = 5.8 v. 30.6, s.d. = 5.3 years), more likely to be primiparous (45.2% v. 42.0%) and less likely to be recent immigrants (16.7% v. 27.2%) (Table 1). They were more likely to report having previously experienced postpartum depression (4.4% v. 1.4%), any depression (15.3% v. 4.4%) and any anxiety disorder (13.8% v. 4.3%). They were more likely to report smoking (15.0% v. 10.2%), use of non-prescribed substances (3.0% v. 1.4%) and intimate partner violence in pregnancy (2.7% v. 1.5%). Pregnancy complications (16.0% v. 13.9%), preterm birth (8.2% v. 6.8%) and low Apgar scores (<7 at 1 or 5 min) (10.5% v. 7.6%) were also more common in this group.
Table 1 Selected characteristics of n = 228 134 individuals with and without a common postpartum mental health (PMH) disorder
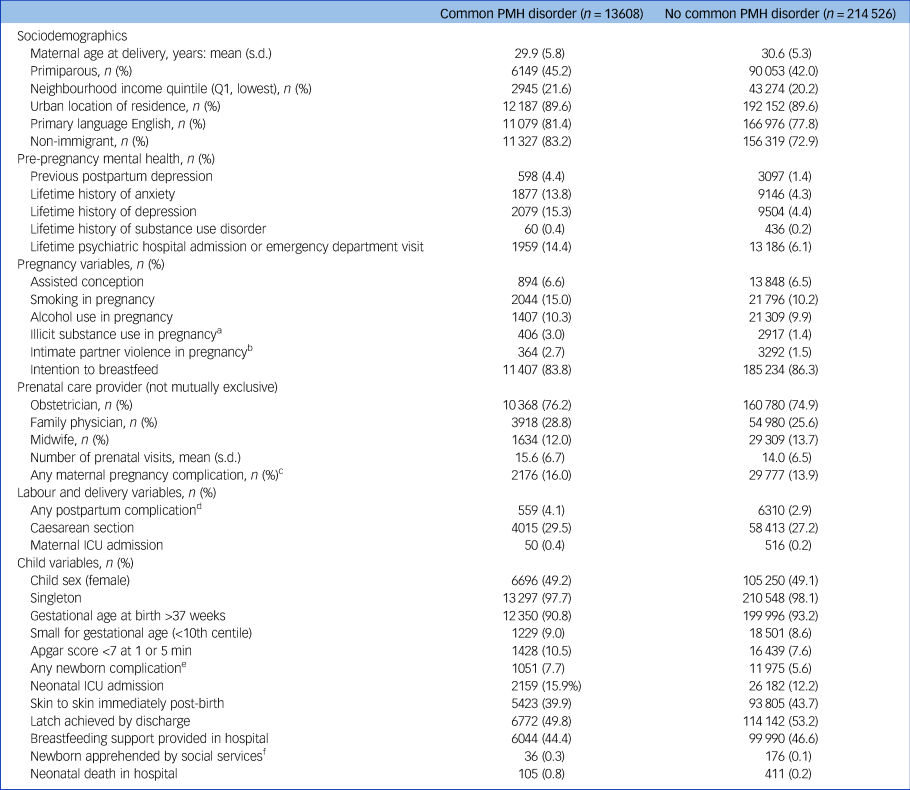
ICU, intensive care unit.
a. Cannabis, cocaine, gas, hallucinogen, methadone, narcotic, opioid, other.
b. Data missing for 58 187 (25.5%) women.
c. Hypertensive disorders of pregnancy (gestational pre-existing + pre-eclampsia; pre-eclampsia; eclampsia; haemolysis, elevated liver enzymes and low platelet count (HELLP syndrome)), diabetes mellitus in pregnancy (type 1, type 2, gestational diabetes\insulin, gestational diabetes\no insulin), hyperemesis gravidarum, anaemia, bleeding, preterm premature rupture of membranes (PPROM), PROM).
d. Late postpartum haemorrhage, uterine atony, hysterectomy, abdominal incision infection, perineal laceration, pulmonary embolus, mastitis, thrombophlebitis, urinary tract infection, postpartum fever, postpartum perineal hematoma.
e. Neonatal abstinence syndrome, congenital malformation, infection, intraventricular haemorrhage, persistent fetal circulation, respiratory distress syndrome, seizure, sepsis.
f. Apprehended denotes that the newborn is taken into the care of social services.
In the development sample (n = 152 362), predictive capacity was low when only sociodemographics were included (5 variables, C-statistic of 0.586), but improved with the addition of pre-pregnancy mental health (9 variables, C = 0.655) and pregnancy variables (15 variables, C = 0.685) (Supplementary Table 4). Adding labour/delivery and then child variables led to slight improvements in discriminative capacity, albeit with a large number of additional variables (20 variables, C = 0.687, and 23 variables, C = 0.690, respectively) (Supplementary Table 4). As more variables are not advantageous when the objective is to create a risk index that can be easily computed in most healthcare contexts, the final model was simplified to maintain its discriminative capacity with the minimum number of variables. This led to a final model of 16 variables (C = 0.688) (Table 2). The model had similar discriminative capacity in the validation data-set (C = 0.686).
Table 2 Balanced logistic regression model in the development cohort (n = 152 362) predicting common postpartum mental health disorder, with the 16 variables presented using fully adjusted odds ratios (OR) and 95% confidence intervals

Random forest model results yielded only slightly increased discriminative capacity in the validation sample compared with the clinical risk index (Supplementary Table 5). Logistic regression models constructed specifically for depressive episodes yielded a higher discriminative capacity in the development sample (C = 0.733) but this increase in discriminative capacity was not borne out in the validation sample (C = 0.698). Models constructed specifically for anxiety and related disorders yielded discriminative capacity similar to that observed in the main models (development C = 0.683; validation C = 0.680). Since neither the random forest model nor the diagnosis-specific models had improved performance, we retained the main model for the risk index.
When converting the final model into a risk index, we created the acronym ‘PMH CAREPLAN’ as a useful mnemonic for remembering its variables in the risk index that potentially would be used to plan an individual's PMH care: (P) prenatal care provider; (M) mental health diagnosis history and medications during pregnancy; (H) psychiatric hospital admissions or emergency department visits; (C) conception type and complications; (A) apprehension of newborn by child services; (R) region of maternal origin; (E) extremes of gestational age at birth; (P) primary maternal language; (L) lactation intention; (A) maternal age (at delivery); and (N) number of prenatal visits (Table 3). As very few observations occurred at the extremes and estimates of outcome risk were unstable below 0 and over 39, scores were left- and right-trimmed to 0 and 39. Expected probability of a common PMH disorder using the PMH CAREPLAN index ranged from 1.56% at a score of less than 6 to 40.5% at a score of 39 or above, and the relationship appeared linear (Fig. 1).

Fig. 1 Distribution of risk scores in the development cohort (n = 152 362) and observed common postpartum mental health (PMH) disorder rate and 95% confidence interval (CI) associated with each point on the risk index.
Table 3 PMH CAREPLAN index (range 0 to 39) for risk of common postpartum mental health disorders, with points assigned to values within the 16 variables in the index
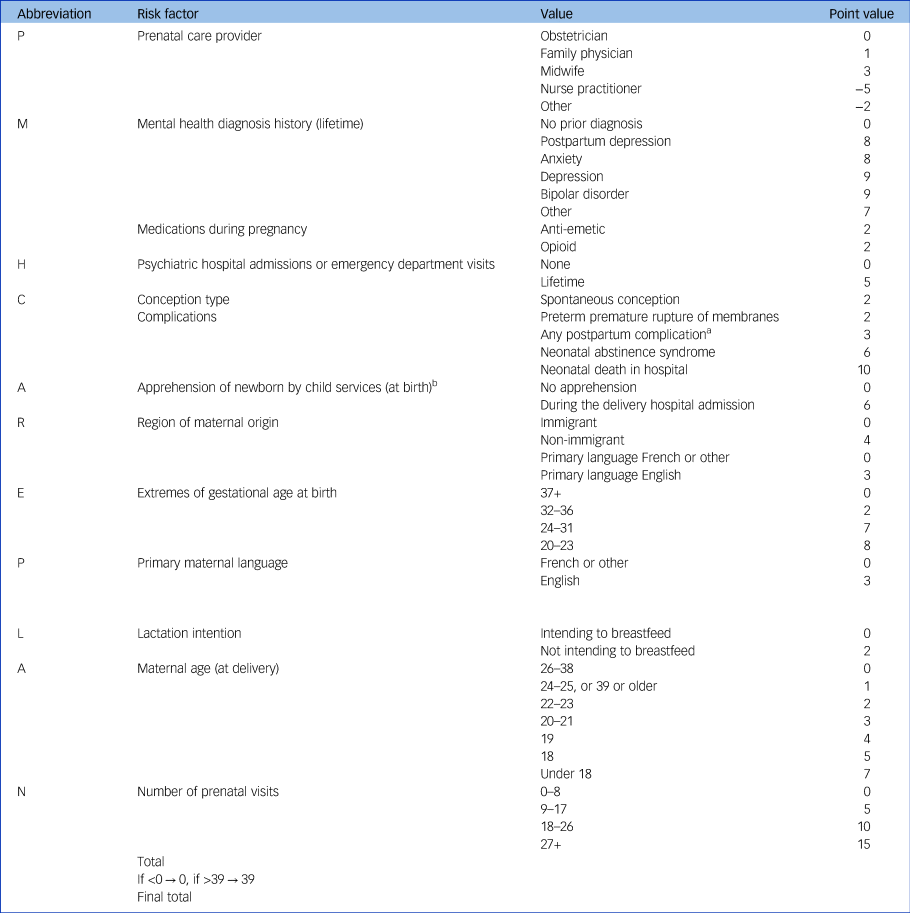
a. Postpartum complications are: late postpartum haemorrhage, uterine atony, hysterectomy, abdominal incision infection, perineal laceration, pulmonary embolus, mastitis, thrombophlebitis, urinary tract infection, postpartum fever, postpartum perineal hematoma.
b. The newborn is taken into the care of social services.
The expected probability of a common PMH disorder was within the 95% confidence interval observed for all scores in both samples (except for a score of 39 or above, where the expected rate was above the 95% confidence interval of the observed probability), indicating adequate calibration (Supplementary Table 6). In the validation sample, when the scores were treated as screening cut-offs, the best ‘trade-off’ of sensitivity/specificity (0.620/0.654) appeared to be at a threshold score of 17 or above. The higher the cut-off score (or threshold), the greater the specificity and the lower the sensitivity; given the low overall risk, almost all thresholds were associated with low positive predictive values and high negative predictive values (examples of various cut-off scores for both the development and validation samples are in Supplementary Table 7). In the leave-one-out analyses, discriminative capacity ranged from 0.65 to 0.71 and 0.67 to 0.69 based on provincial region of residence and the neighbourhood income quintile respectively (Supplementary Table 8).
Discussion
We developed and internally validated a predictive model quantifying the risk for receiving a diagnosis of a common mental health disorder in the first postpartum year in a population-based sample. The model approached 70% in its ability to discriminate between those who would or would not develop a common PMH disorder over their first year postpartum, a discriminative capacity that was reasonably robust to leave-one-out analyses based on maternal region of residence and income level. The PMH CAREPLAN is the first published risk score in this area that considers all common PMH disorders, not only depressive episodes. It is also the first to use a physician-based clinical diagnosis, where outcome specificity is likely to be greater than that of prior risk indices where a diagnosis was assigned based on a cut-off on a self-report symptom scale.Reference Webster, Pritchard, Creedy and East24
Strengths and limitations
The model had similar discriminative capacity to previous studies, all of which predicted postpartum depression specifically (e.g. C = 0.71 in validation studiesReference Nielsen, Videbech, Hedegaard, Dalby and Secher13,Reference Webster, Hall, Somville, Schneider, Turnbull and Smith14 and C = 0.77 in a development-only sampleReference Webster, Pritchard, Creedy and East24), and were survey-based and required depressive symptom scale input early postpartum. The predictive capacity of the current model could perhaps be improved by including both routinely collected and self-report variables that provide more information on clinical symptomatology and on the social context. For example, certain risk factors were not measurable in our data-sets and would have to be collected by self-report, such as subsyndromal symptoms and psychosocial stressors (e.g. life stress, low social support).Reference Yonkers, Simone and Ross4 Further, knowledge about certain postnatal factors might have improved predictive capacity, such as length of parental leave, nature of social support over time or other factors that occur postnatally that would not have been known at the time of delivery. However, advantages of the current model are that it generates a reasonable estimate of risk with routinely collected clinical data alone and that it uses data that are available at the time of delivery, which is an opportune time for identifying individuals who may benefit from additional monitoring or targeted intervention, given that all individuals are in contact with healthcare professionals.
Clinical and research implications
Although improving predictive capacity is a laudable goal, most risk indices do not rely on perfect prediction of a future state for actionable results.Reference McCloskey, Harvey, Johansson, Lorentzon, Liu and Vandenput11,Reference Gates, Pillay, Theriault, Limburg, Grad and Klarenbach25 For example, clinical practice guidelines recommend preventive treatment with osteoporosis medications when the 10-year risks of a major fracture or hip fracture exceed 20% and 3% respectively based on the FRAX index.Reference McCloskey, Harvey, Johansson, Lorentzon, Liu and Vandenput11 In a trial of FRAX screening, individuals were offered preventive treatment with osteoporosis medications based on their 10-year risk level; in the screened group preventive treatment uptake was much higher and risk of hip fracture was reduced by 28%, lending support to the use of the index.Reference Shepstone, Lenaghan, Cooper, Clarke, Fong-Soe-Khioe and Fordham12 With PMH CAREPLAN, one could risk stratify based on the 1-year risk for a common PMH disorder to develop, and evaluate evidence-based psychological or pharmacological interventions accordingly for whether screening with the risk index improves the uptake of preventive interventions and reduces the risk for a PMH disorder to develop. One could also test interventions based on a cut-off or threshold level of risk. Where the threshold risk level is set for an intervention would depend on tolerance for missing cases and/or for false-positive results. At the cut-off score of 17 or above that optimises the balance between sensitivity and specificity, about 62% of cases would be identified, with a false-positive rate of about 35%. This is likely to be a reasonable threshold for evaluation of monitoring strategies or of lower-cost preventive interventions with low potential for harm (i.e. where it is not highly problematic if some people receive the intervention who may not have needed it). In contrast, a higher cut-off score optimising specificity might be more appropriate for evaluating interventions with more potential for adverse effects (e.g. medications).
Prior to testing interventions, however, external validation is recommended. Although the prevalence of common PMH disorders (~6%) in this study is consistent with previous population-level estimates, using health administrative data limited our ability to capture individuals who did not come to medical attention. This means that the model could perform differently when diagnoses are ascertained systematically for an entire population. This might be particularly important because we found that being a recent immigrant and not speaking English as the primary language were protective factors in our model. Although this may reflect a healthy immigrant effect and/or the protective nature of postpartum cultural rituals that increase social support, the cultural and language barriers faced by immigrants can increase risk for common PMH disorders and reduce access to healthcare.Reference Guintivano, Manuck and Meltzer-Brody26,Reference DiBari, Yu, Chao and Lu27 It is also important to note that the PMH CAREPLAN risk index focuses on risk for common postpartum mental disorders (depressive, anxiety and related disorders such as obsessive–compulsive and trauma- and stressor-related disorders).Reference Howard, Molyneaux, Dennis, Rochat, Stein and Milgrom2 These common PMH disorders are to be distinguished from the more rare but severe postpartum mental disorders such as postpartum psychosis, bipolar disorder and primary psychotic disorders, whose distinct clinical, epidemiological and biological risk factors would likely necessitate a separate risk index building process.Reference Jones, Chandra, Dazzan and Howard28
Summary and next steps
The PMH CAREPLAN risk index creates a framework for clinically actionable risk stratification that could assist patients and providers in determining an individual's level of risk for common postpartum mental health disorders and direct them to appropriate intervention. After evaluation of its utility at optimising the uptake of preventive treatments and reducing risk for developing a common PMH disorder, it could be easily integrated into almost any type of antenatal or postpartum care setting. Future research to externally validate and refine the PMH CAREPLAN index and to explore the effectiveness of preventive interventions at varying levels of risk will be critical to its use in the clinical setting.
Supplementary material
Supplementary material is available online at https://doi.org/10.1192/bjp.2023.74.
Data availability
The data for this study are not publicly available owing to restrictions related to the Personal Health Information Privacy Act of Ontario in its permissions for data use. However, detailed data-set creation and analytic plans (including health administrative diagnostic codes used and SAS® coding) are available from the corresponding author on request.
Acknowledgements
Parts of this material are based on data and/or information compiled and provided by the Canadian Institute for Health Information (CIHI) and Immigration, Refugees and Citizenship Canada's Permanent Resident Database (IRCC) current to 31 May 2017. However, the analyses, conclusions, opinions and statements expressed herein are those of the authors and not necessarily those of the CIHI or IRCC. This document used data adapted from the Statistics Canada Postal Code Conversion File, which is based on data licensed from Canada Post Corporation. This work was supported by the ICES, which is funded by an annual grant from the Ontario MOH and MLTC. The opinions, results and conclusions reported in this paper are those of the authors and are independent from the funding sources. No endorsement by ICES or the Ontario MOH or MLTC is intended or should be inferred. This study is based in part on data provided by Better Outcomes Registry and Network (BORN), part of the Children's Hospital of Eastern Ontario. The interpretation and conclusions contained herein do not necessarily represent those of BORN Ontario.
Author contributions
S.N.V. formulated the research question, designed the study, created the analytic plan and drafted the article. N.U. contributed to formulation of the research question and analytic plan and drafting of the article. A.C. contributed to formulation of the research question, study design and analytic plan, analysed the data and edited the article. C.-L.D., A.G., B.D.T., M.W. and H.K.B. contributed to formulating the research question, designing the study, creating the analytic plan, and edited the article. All authors approved the final version of the manuscript.
Funding
This work received funding from the Canadian Institute of Health Research (CIHR PCG-155466).
Declaration of interest
S.N.V. receives royalties from UpToDate Inc for authorship of materials related to depression and pregnancy.
eLetters
No eLetters have been published for this article.