Book contents
- Frontmatter
- Contents
- Preface
- 1 Probability basics
- 2 Estimation and uncertainty
- 3 Statistical models and inference
- 4 Linear models, least squares, and maximum likelihood
- 5 Parameter estimation: single parameter
- 6 Parameter estimation: multiple parameters
- 7 Approximating distributions
- 8 Monte Carlo methods for inference
- 9 Parameter estimation: Markov Chain Monte Carlo
- 10 Frequentist hypothesis testing
- 11 Model comparison
- 12 Dealing with more complicated problems
- References
- Index
- References
References
Published online by Cambridge University Press: 12 July 2017
- Frontmatter
- Contents
- Preface
- 1 Probability basics
- 2 Estimation and uncertainty
- 3 Statistical models and inference
- 4 Linear models, least squares, and maximum likelihood
- 5 Parameter estimation: single parameter
- 6 Parameter estimation: multiple parameters
- 7 Approximating distributions
- 8 Monte Carlo methods for inference
- 9 Parameter estimation: Markov Chain Monte Carlo
- 10 Frequentist hypothesis testing
- 11 Model comparison
- 12 Dealing with more complicated problems
- References
- Index
- References
Summary
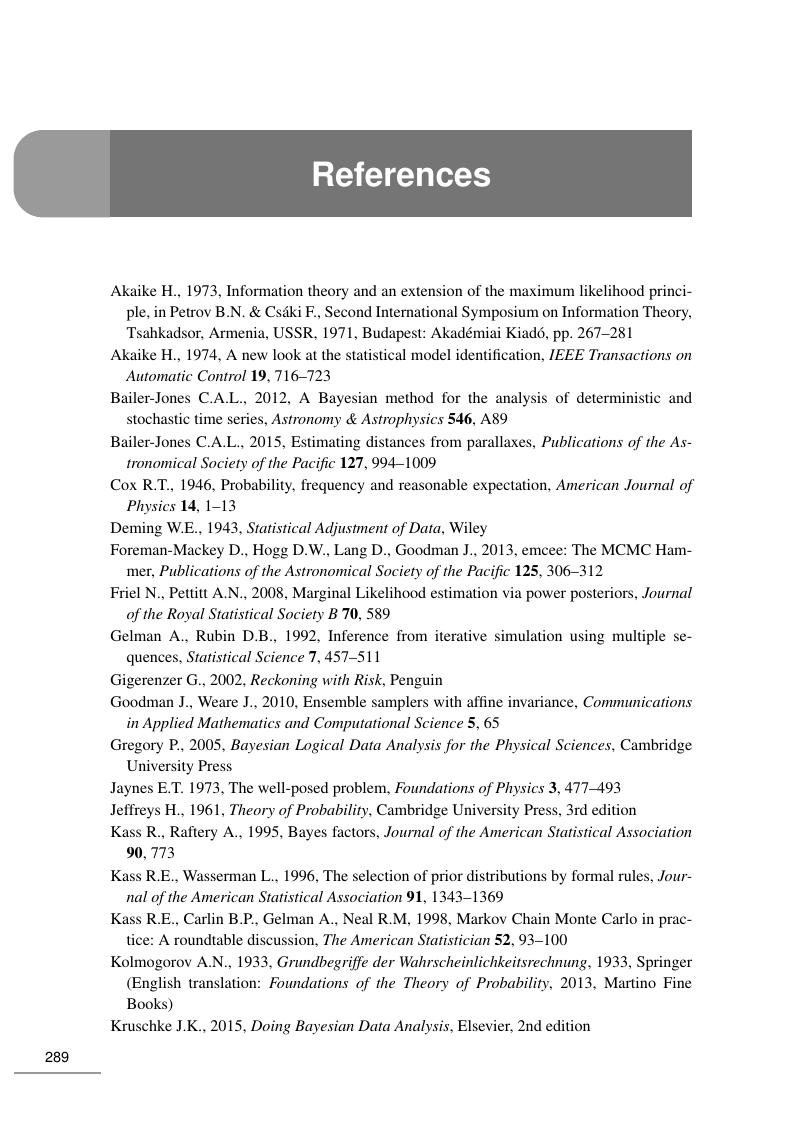
- Type
- Chapter
- Information
- Practical Bayesian InferenceA Primer for Physical Scientists, pp. 289 - 290Publisher: Cambridge University PressPrint publication year: 2017