References
Published online by Cambridge University Press: 09 April 2021
Summary
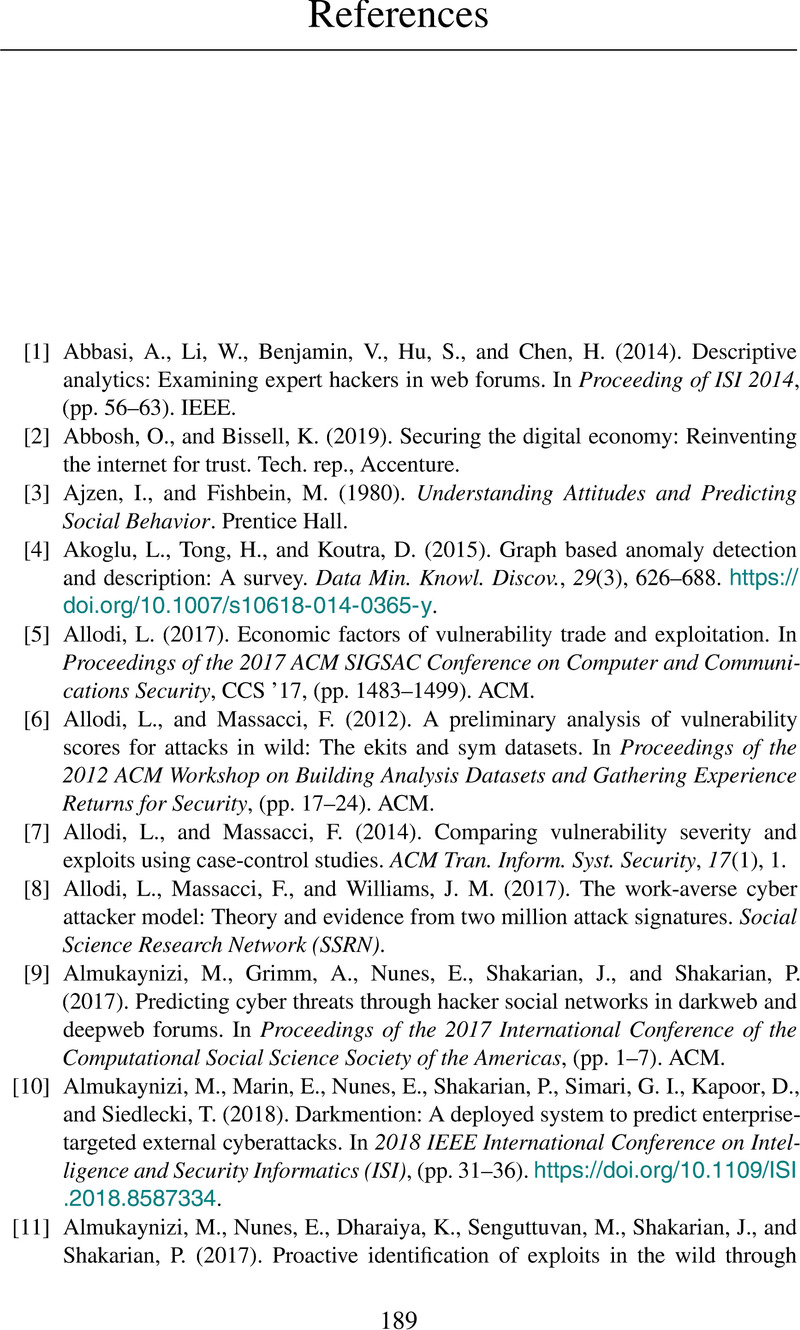
- Type
- Chapter
- Information
- Exploring Malicious Hacker CommunitiesToward Proactive Cyber-Defense, pp. 189 - 202Publisher: Cambridge University PressPrint publication year: 2021