If neighborhood perceptions can drive selection into and out of certain areas, influence the concentration of social problems, exacerbate negative health outcomes, and steer urban policy, then identifying factors that influence those perceptions is crucial to understanding city life and developing effective urban policy. What shapes how we see city streets? Research has shown that perceptions of disorder are influenced less by outright signs of decay and neglect – e.g., litter, broken windows, graffiti, public nuisances, crime – than by the kinds of people who inhabit a neighborhood. As it was at the turn of the century, when Du Bois (Reference Du Bois[1899] 1996) was writing about Philadelphia, and as it was at midcentury, when Jacobs (Reference Jacobs1961) was writing about New York, race infuses our evaluations of urban neighborhoods. Sampson demonstrates that nonblack residents are more likely to leave the city if they live in neighborhoods where blacks have a growing presence (Reference Sampson2012, 300). Quillian and Pager show that city dwellers’ perceptions of crime are positively associated with the percentage of young black men in their neighborhood, controlling for crime levels and other neighborhood factors (Reference Quillian and Pager2001).
Race casts a long shadow over neighborhood perceptions. What else does? Here, urbanists are surprisingly quiet; and their silence leaves us particularly unprepared to understand the views of residents in racially segregated neighborhoods, where the vast majority of Americans live. Most surprisingly, researchers have neglected to appreciate how housing dynamics shape neighborhood perceptions. When Du Bois (Reference Du Bois[1899] 1996, ch. 15) set out to write about “the environment” of black Philadelphians, he began by analyzing “houses and rent.” Only after reviewing the cost, quality, and spatial organization of housing in the ghetto did he broaden to “sections and wards.” Du Bois recognized that the house and the neighborhood were intimately linked. But this insight was largely lost on the Chicago School, whose scholars came to view neighborhoods as “moral regions” or sites of residential attainment, a preoccupation that neglected the fact that neighborhoods were also markets and largely owned, in the case of the inner city, by landlords who do not live within their borders. As the neighborhood became a core object of social-scientific analysis, the house faded from view. Despite the efflorescence of research on neighborhood effects (Sampson et al. Reference Sampson, Morenoff and Gannon-Rowley2002; Sharkey and Faber Reference Sharkey and Faber2014), we still know relatively little about the role housing dynamics play in shaping the characteristics and perceptions of city blocks.
To understand the link between housing and neighborhood dynamics, this chapter investigates how three housing dynamics – (1) residents’ reasons for moving; (2) their strategies for finding housing; and (3) the quality of their dwelling – influence neighborhood perceptions. Drawing on a novel survey of renters in Milwaukee, it finds that city dwellers who relocated to their neighborhood after an eviction, who found their apartment through a nonprofit or government agency, and who experienced long-lasting housing problems harbored lower evaluations of their neighborhoods. These findings indicate that any theory of the neighborhood will be incomplete without accounting for the influence of housing dynamics.
Forced into a Neighborhood
Social scientists have long remarked that low-income families experience high rates of residential instability without explaining why this is so. Recent research, however, has revealed the high prevalence of eviction in the lives of renters, demonstrating that poor families move so much simply because they are forced to (Desmond, Gershenson, and Kiviat Reference Desmond, Gershenson and Kiviat2015). Over the past decade, low-income families have watched their incomes stagnate while their housing costs have soared. Meanwhile, only one in four families who qualify for housing assistance receives it. These transformations have led to a rapid increase in severely rent-burdened households – according to the American Housing Survey, roughly half of poor renting families spend at least half of their income on housing (Eggers and Moumen Reference Eggers and Moumen2010) – and eviction has become a common occurrence in the lives of low-income families. In Milwaukee, the setting of this study, one in eight renter households experiences an involuntary move every two years (Desmond and Shollenberger Reference Desmond and Shollenberger2015). Nationwide, renters in more than 2.8 million homes believe they will be evicted soon (Desmond Reference Desmond2015).
While middle-class families may exert a good deal of control and intentionality over their mobility decisions, poor families often are forced from their homes.1 In the harried aftermath of eviction, finding subsequent housing consumes renters’ time and attention. Because many landlords reject recently evicted applicants, displaced families often apply to dozens of apartments before being accepted to one, their housing search stretching on for months (Desmond Reference Desmond2016a). When they finally do find subsequent housing, it is often substandard and located in disadvantaged neighborhoods (Desmond et al. Reference Desmond, Gershenson and Kiviat2015; Desmond and Shollenberger Reference Desmond and Shollenberger2015). But when the alternative is homelessness, the priority of finding shelter takes precedence, even if it means moving into a run-down apartment on a dangerous block. As one mother I met during fieldwork put it to her children after their eviction: “We take whatever we can get” (Desmond Reference Desmond2016a).
It is one thing to enter a neighborhood voluntarily; it is quite another to relocate in the exhausting and stressful aftermath of an eviction. Yet no study has investigated the relationship between the circumstances by which families select into a neighborhood and their perceptions of that neighborhood. If many families settle for a place after their eviction, taking “whatever they can get,” we might expect them to have lower evaluations of their neighborhood than those who moved under less trying circumstances. This leads to the following hypothesis:
Hyp. 1. Renters whose previous move was forced will express less favorable views of their neighborhood than renters who entered the neighborhood through more voluntary means.
Finding a Neighborhood
Besides overlooking why families move, conventional accounts of neighborhood selection also tend to ignore how families move (though see Farley Reference Farley1996; Krysan Reference Krysan2008): the multiple ways they locate subsequent housing. “More often,” write Ludwig and collaborators, “we do not know exactly what is driving the [neighborhood] selection process, and we should worry that selection could occur in part on the basis of factors that are not well understood or easily measured” (Reference Ludwig, Liebman, Kling, Duncan, Katz, Kessler and Sanbonmatsu2008, 176). But we can study directly “what is driving the selection process,” treating neighborhood selection as an important topic of inquiry in its own right (Sharkey Reference Sharkey2013). “In examining the sources and social consequences of residential sorting,” Sampson has argued, “we need to conceptualize neighborhood selection not merely as an individual-level confounder or as a ‘nuisance’ that arises independent of social context. Instead, neighborhood selection is part of a process of stratification that situates individual decisions within an ordered, yet constantly changing, residential landscape” (Reference Sampson2008, 217).
The relocation strategies of urban renters may be meaningfully diverse. Some may undertake a search independently, scanning the newspaper, local media sources, or the Internet for housing options. Others may use state, municipal, or nonprofit social-service agencies. Still others may rely on network ties, relocating to neighborhoods because a family member or friend told them about a unit coming available or referred them to a landlord (Rossi Reference Rossi[1955] 1980, 207–10). Could the ways renters find housing influence their neighborhood perceptions?
There is a qualitative difference between finding an apartment through your own efforts or those of your social network and moving into a place found or assigned by a third-party agency, such as the Public Housing Authority. It is the difference between placing yourself and being placed in a neighborhood. In the former instance, renters may have spent more time, money (e.g., application fees), and social capital during their housing search, which may kindle a psychological desire to reap returns on their efforts. Should the neighborhood be unsafe or otherwise distressed, renters who found housing alone or through their social connections have no one to blame other than themselves or their close ties, while renters who found housing through a nonprofit or government agency can blame a third party. These considerations lead to the following hypothesis:
Hyp. 2. Renters who located housing themselves or by relying on social networks will express more favorable views of their neighborhood than renters who found housing through a government or nonprofit agency.
Seeing Your Neighborhood through Cracked Windows
Besides paying attention to the circumstances of city dwellers’ previous moves, and the ways they located subsequent housing, I also consider the condition of a family’s house. Housing quality in the United States has increased significantly over the past decades (Schwartz Reference Schwartz2010). However, some low-income families still live in degrading and dangerous housing conditions. According to the American Housing Survey, 1.2 million renter-occupied units had severe physical problems in 2011 (Desmond Reference Desmond2016a).
For many city dwellers, most of their time spent in a neighborhood is spent in their homes. Poor housing conditions could influence residents’ neighborhood perceptions in at least two ways. First, such conditions could dim their perceptions of the world in general. Studies have linked housing problems to poor mental health outcomes, including depressive symptoms, anxiety, and neurological disorders (Evans, Wells, and Moch Reference Evans, Wells and Moch2003; Shaw Reference Shaw2004); and quasi-experimental evidence suggests that housing improvements can improve mental health outcomes (Curl et al. Reference Curl, Kearns, Mason, Egan, Tannahill and Ellaway2015). Negative mental health outcomes could be a mechanism through which poor housing conditions deflate residents’ perceptions of their neighborhood.
Second, city dwellers with poor housing conditions may spend less time in their homes and thus may be more regularly exposed to neighborhood disorder and crime simply by virtue of heightened neighborhood usage. One way to cope with sinking bathtubs, stopped-up plumbing, and no heat is by spending as little time in your home as possible. As one resident of a low-income trailer park in Milwaukee told me: “My trailer is a hotel. … I sleep there, and that’s about it. I wake up in the morning and leave and go to bed at night, and that’s it.” Housing problems are concentrated in disadvantaged neighborhoods (Desmond Reference Desmond2016a). Residents of those neighborhoods who flee poor housing conditions may find themselves confronting a different set of problems in the form of public disorder or violence. These paired considerations lead to the following hypothesis:
Hyp. 3. Renters living with poor housing conditions will express less favorable views of their neighborhood than renters who live in higher-quality housing.
Data
To test these three hypotheses, this study draws on the Milwaukee Area Renters Study (MARS), an original survey comprised of more than 250 unique questions asked of 1,086 tenants in Milwaukee’s private housing sector (Desmond Reference Desmond2016b).2 From 2009 to 2011, households were selected into MARS through multi-stage stratified sampling. Blocks were randomly selected from strata so as to create a sample generalizable to Milwaukee’s rental population. This sampling strategy drew from 168 of 591 unique block groups, representing 28 percent of Milwaukee block groups. When a block was selected into the sample, interviewers visited every renter-occupied household in it, saturating the targeted areas. To bolster response rate and data quality, surveys were administered in person in English and Spanish by professional interviewers at tenants’ place of residence. For each household, interviewers surveyed an adult leaseholder or, should a leaseholder be unavailable, an adult knowledgeable about household financial matters. According to the most conservative calculation (AAPOR Rate 1), MARS has an 83.4 percent response rate.
After data collection, custom design weights were calculated to reflect the inverse of selection probability, facilitated by a Lahiri (Reference Lahiri1951) procedure, based on the demographic characteristics of Milwaukee’s rental population and adjusted to MARS’s sample size. The Lahiri procedure allows the sampler to select probability samples (with a probability proportional to size) and to compute the selection probabilities for the resulting sample. Selection probabilities are then used to calculate the design weights for the overall sample. I use custom weights when presenting descriptive statistics.
The characteristics of Milwaukee’s residents (Pager Reference Pager2007) and rental market (U.S. Department of Housing and Urban Development 2009) are comparable to those of many U.S. cities. Most low-income city dwellers neither own their homes nor live in public housing (Desmond Reference Desmond2015; Schwartz Reference Schwartz2010). MARS’s focus on the private rental market, then, reflects the experiences of the vast majority of low-income families.3 That said, it is important to bear in mind that the MARS sample excludes homeowners, and the extent to which these findings apply to other cities remains to be seen.
Main Outcome Variables
This study relies on two measures of neighborhood perception: the degree to which renters trust their neighbors and the amount of concentrated suffering renters believe to be within their neighborhood.
Perceived Trust. Cultivating social capital and collective efficacy on the local level depends in large part on the degree to which neighbors find one another trustworthy. Studies have shown that social trust not only serves as the foundation for civic engagement and reciprocal exchanges that help families make ends meet (Putnam Reference Putnam2001; Sampson Reference Sampson2012); it also is linked to individual health outcomes and other indicators of well-being (Kawachi et al. Reference Kawachi, Kennedy, Lochner and Prothrow-Stith1997). Accordingly, I measured renters’ neighborhood perceptions through the question: “How much do you trust people in your neighborhood?” Responses were recorded on a five-point scale ranging from “not at all” to “a great deal.” For ease of interpretation, neighborhood trust is reported as a binary variable. Renters were considered to trust their neighbors if they reported trusting people in their neighborhood “quite a bit” or “a great deal.”
Perceived Suffering. I also observed the degree to which renters believe social problems were found in their neighborhood (Sampson Reference Sampson2012). Each respondent was asked: “While you have been living in this neighborhood, have any of your neighbors ever: (1) been evicted; (2) been in prison; (3) been in an abusive relationship; (4) been addicted to drugs; (5) had their children taken away by social services; or (6) had a close family member or friend murdered?” This measure allowed me to observe what kind of neighborhood renters believed themselves to be living in: one relatively free of hardship, violence, and vice or one brimming over with disadvantage. I treat this measure as a count variable (score 0–6).
Explanatory Variables
Forced Moves. To assess if renters’ neighborhood perceptions were influenced by the nature of their previous move – the housing-related circumstances that brought them to their current neighborhood – I examined if that move was induced by eviction, foreclosure, or building condemnation (Desmond and Shollenberger Reference Desmond and Shollenberger2015). These are moves that were involuntary or forced, initiated by landlords or city officials (e.g., code inspectors), and involved situations where tenants had no choice other than to relocate. Forced moves are distinct not only from voluntary moves, intentional and uncoercive relocations often carried out to gain residential advantage, but also from responsive moves, motivated by housing or neighborhood conditions such as rent hikes, a deterioration in housing quality, or escalating neighborhood violence. Because retrospective data are most reliable when limited to a recent recall period (Beckett et al. Reference Beckett, DaVanzo, Sastry, Panis and Peterson2001), I only recorded involuntary moves that occurred within two years prior to the survey. Doing so had the added benefit of conservatively biasing the estimated effect of eviction toward zero, since renters who had lived in their neighborhood for more than two years and whose previous move was involuntary were not classified as recently evicted.
Housing Search Strategies. I observed how tenants found their current residence through the question: “How did you find this place? Was it through: (a) a friend; (b) a family member; (c) a [nonprofit] agency; (d) a newspaper, Redbook, Bluebook;4 (e) a ‘for rent’ sign; (f) the Internet; (g) the Housing Authority; (h) some other way?” I organized responses into three categories: network-based searches that relied on kin, friends, or other social ties; agency-based searches that relied on the Housing Authority or nonprofit organizations; and individual searches in which tenants located housing themselves by relying on print media or the Internet, or by calling on “for rent” signs. Nearly all renters in our sample (97 percent) found housing exclusively through one of these types of searches.5 Although renters may have searched for housing in multiple ways, this measure records the technique that led them to the dwelling they inhabited at the time of the survey.
Housing Problems. To measure housing quality, renters were asked if they had experienced any of the following problems in their current residence in the year prior to being interviewed: at least three days with (a) a broken stove or other appliance; (b) a broken window; (c) a broken exterior door or lock; (d) mice, rats, or other pests; or (e) exposed wires or other electrical problems; or at least 24 hours with (f) no heat; (g) no running water; or (h) stopped up plumbing. Responses were summed.
Controls
All models control for a number of demographic attributes related to neighborhood perception, including respondents’ race and ethnicity, gender, and age (Hartnagel Reference Hartnagel1979; Quillian and Pager Reference Quillian and Pager2001). I also observed respondents’ highest level of education, a stable measure of socioeconomic status (Sampson Reference Sampson2012; Soss and Jacobs Reference Soss and Jacobs2009). I accounted for family status by observing if each respondent lived with minor children and was the only adult in the household. Living alone or with children could influence one’s views of their community (Kimbro and Schachter Reference Kimbro and Schachter2011; Klinenberg Reference Klinenberg2012).
Renters who experienced recent setbacks might also harbor more negative views of their community. Accordingly, I observed if renters lost their job or experienced relationship dissolution within the previous two years. Although cost-burdened renters need not experience a major setback to invite eviction, accounting for recent job losses and breakups allowed me to observe the relationship between involuntary moves and neighborhood perceptions, conditioning on other recent shocks that could also color renters’ views.
Next, I controlled for several factors related to respondents’ time and experiences in their neighborhood. Long-standing residents might view their community in a different light than new arrivals (Highton Reference Highton2000). Accordingly, I observed how long each respondent had lived in her or his neighborhood. In a similar vein, I controlled for the distance (in miles) between renters’ current and previous addresses. Moving long distances, such as relocating from across the city or another city entirely, could influence one’s views of their current community in stronger ways than moving short distances. Additionally, because renters’ neighborhood experiences and perceptions are steered by their relationships with people in their community (Glynn Reference Glynn1986; Stack Reference Stack1974), I observed how many of a respondent’s “closest family members/friends” lived in her or his neighborhood.
The address of each MARS respondent was geo-coded using ArcGIS and an associated road network database. I then assigned each residence to a census block group, my neighborhood metric. In Milwaukee, the population of the average block group was 1,135 in 2010. Each block group was then linked to aggregate data from the 2010 U.S. Census and crime records from the Milwaukee Police Department. I controlled for neighborhood poverty rate: the percentage of people in a census block group below the poverty line. This is a straightforward measure of concentrated disadvantage (Sampson Reference Sampson2012; Wilson Reference Wilson1987). As discussed later, results are robust to other community-level measures, including a neighborhood disadvantage composite variable.
To account for missing data prior to estimation, I conducted multiple imputation (m = 10). Values for missing data were estimated using regression equations that relied on all in-sample variables as predictors (Allison Reference Allison2002). Where appropriate, logit, ordinal logit, and negative binomial models were used, depending on the type of imputed variable. By and large, MARS has very little missing data. The average variable in our sample was missing only 1.2 percent of observations. Findings hold across imputed and non-imputed datasets. Summary statistics for all variables are presented in Table 7.1.
Table 7.1: Weighted Summary Statistics
Mean | SD | Min | Max | Count | |
---|---|---|---|---|---|
Perceived Trust | 2.88 | 1.18 | 1 | 5 | 1,055 |
Perceived Suffering | 0.91 | 1.32 | 0 | 6 | 1,021 |
Previous Move was Forced | 0.10 | 0 | 1 | 1,063 | |
Found Housing through Network | 0.51 | 0 | 1 | 1,063 | |
Found Housing through Agency | 0.05 | 0 | 1 | 1,063 | |
Found Housing through Self | 0.44 | 0 | 1 | 1,063 | |
Number of Lasting Housing Problems | 0.80 | 1.14 | 0 | 9 | 1,063 |
Black Renter | 0.34 | 0 | 1 | 1,060 | |
Hispanic Renter | 0.14 | 0 | 1 | 1,060 | |
White Renter | 0.46 | 0 | 1 | 1,060 | |
Other Race Renter | 0.06 | 0 | 1 | 1,060 | |
Female Renter | 0.62 | 0 | 1 | 1,062 | |
College Graduate | 0.40 | 0 | 1 | 1,074 | |
Age | 38.78 | 14.68 | 15 | 91 | 1,053 |
Months in Neighborhood | 48.44 | 75.88 | 0 | 635 | 1,037 |
Miles from Previous Residence | 47.33 | 309.35 | 0.006 | 5,408 | 1,032 |
Minor Children in Household | 0.43 | 0 | 1 | 1,060 | |
Only Adult in Household | 0.47 | 0 | 1 | 1,063 | |
Neighborhood Strong Ties | 1.91 | 2.43 | 0 | 26 | 1,044 |
Recent Job Loss | 0.19 | 0 | 1 | 1,049 | |
Recent Relationship Dissolution | 0.23 | 0 | 1 | 1,061 | |
Neighborhood Poverty Rate | 0.12 | 0.15 | 0 | 0.89 | 1,062 |
Observed Disorder | 0.04 | 0 | 1 | 1,036 | |
Violent Crime Rate | 0.12 | 0.12 | 0 | 0.87 | 1,056 |
Neighborhood Disadvantage | −0.51 | 0.79 | −1.451 | 2.99 | 1,056 |
Note: Milwaukee Area Renters Study, N = 1,086.
Methods
I use regressions to examine the relationship between housing dynamics and neighborhood perceptions. When estimating neighborhood trust (0, 1), I rely on logistic regression. To investigate the association between housing dynamics and perceptions of concentrated suffering (a count variable), I employ negative binomial models. Along with the controls listed earlier, models include block-group fixed effects to account for time-invariant neighborhood factors potentially correlated with renters’ perceptions of their community (Allison Reference Allison2009). The identification strategy of the multivariate analyses, then, conditions both on time-variant ecological indicators of disadvantage (through neighborhood-level coefficients) and time-invariant indicators (through neighborhood fixed effects), facilitating comparisons between similar renters in similar neighborhoods who differ with respect to the reasons they entered the neighborhood, how they located their housing, and the quality of dwelling they inhabit.
To address treatment selection, I also employ propensity score matching. Applying experimentalist logic to observational data, this technique compares renters matched along several observable characteristics but who differ by whether they were exposed to a treatment: in this case, one of the three housing dynamics of interest (Rosenbaum and Rubin Reference Rosenbaum and Rubin1983). To predict renters’ propensity for (1) selecting into the neighborhood after a forced move (e.g., eviction) and (2) finding housing through an agency, I included the following characteristics in the matching algorithm: race, age, gender, education, recent job loss, and recent relationship dissolution, as well as indicators for whether the tenant is the only adult in the household or lives with minor children. In addition to these characteristics, when predicting renters’ propensity for (3) experiencing any lasting housing problem (here, a binary outcome), I also included how many months they had lived in the neighborhood, the miles between their current and previous residence, neighborhood-based strong ties, and the neighborhood poverty rate.
Descriptive Patterns: Forced Mobility, Search Strategies, and Housing Problems
Looking strictly at moves that occurred within the previous two years, I found that the prior move for 1 in 10 renters in Milwaukee was a forced relocation. A nontrivial percentage of renting families, then, selected into their current home and community through an involuntary dislocation from their previous neighborhood. This was the case for 17 percent of Hispanic renters, 10 percent of black renters, and 9 percent of white renters – a disparity driven in large part by the high rate of landlord foreclosures in predominantly Latino neighborhoods during the study period (Desmond and Shollenberger Reference Desmond and Shollenberger2015). Fifteen percent of renters living in neighborhoods with high concentrations of poverty (where at least 40 percent of residents lived below the poverty line) came to their neighborhoods after an eviction, compared to 9 percent of renters residing in low-poverty areas (where less than 20 percent of residents lived in poverty).
As displayed in Figure 7.1, considerable differences appear in perceived neighborhood trust and suffering between renters who selected into their community after a forced move and those who did not. Compared to renters who had relocated to their neighborhood after a recent eviction, other renters were twice as likely to report trusting people in their neighborhood “quite a bit” or “a great deal.” This difference is statistically significant (p = 0.007). Recently evicted movers were also far more likely to perceive suffering in their neighborhood (27 percent), compared to non-forced movers or long-term stayers (21 percent).
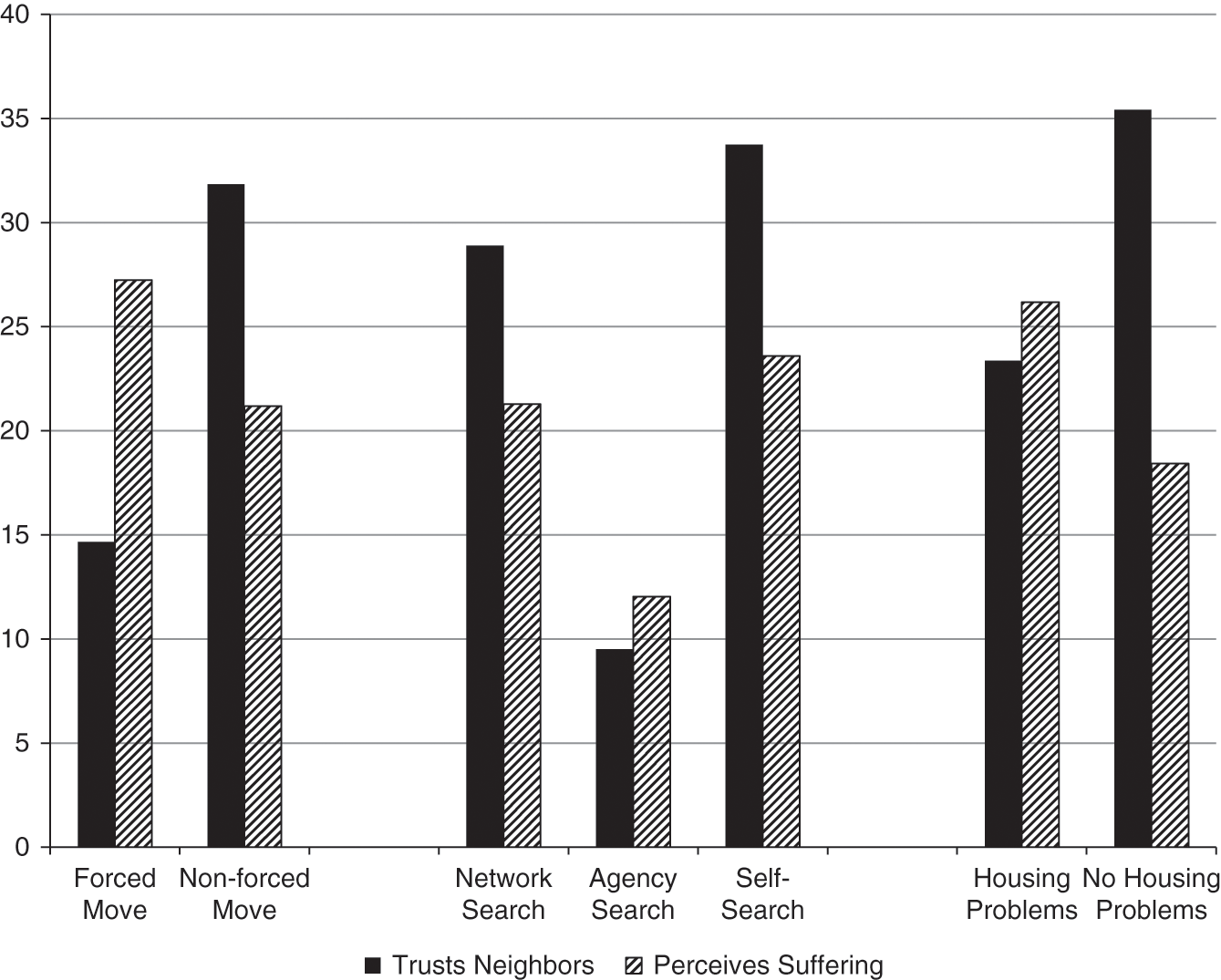
Figure 7.1 Perceived Neighborhood Trust and Suffering by Reasons for Moving, Housing Search Strategies, and Housing Problems (weighted percentages). Renters were considered to have housing problems if they reported at least one lasting issue. Renters were considered to trust their neighbors if they reported trusting people in their neighborhood “quite a bit” or “a great deal.” Renters were considered to perceive suffering if they reported that their neighbors had experienced two or more adverse events. Milwaukee Area Renters Study, N = 1,086.
With respect to locating new housing, most Milwaukee renters (51 percent) found their current housing through a network connection: a friend, family member, church attendee, coworker, or other social tie. An additional 44 percent found their housing by themselves, through searching the newspaper or Internet, or spotting a “for rent” sign. Only 5 percent of renters found their housing through a government or nonprofit agency.
While roughly 58 percent of black renters found housing through social networks, the same was true for 50 percent of Hispanic renters and only 41 percent of white renters. The majority of white renters (54 percent) and 49 percent of Hispanic renters located housing through an independent search. Roughly 34 percent of black renters relied on a self-guided search. A small share of renters – roughly 5 percent of white renters, 8 percent of black renters, and less than 1 percent of Hispanic renters – relied on agencies. The vast majority of tenants who located housing through network ties relied on kin and friends. In sharp contrast to research suggesting that black job seekers receive less help from social ties than other groups (Smith Reference Smith2007), I found that black house seekers receive more.
White, black, and Hispanic renters who searched for housing independently did so differently. Roughly 48 percent of whites who found housing on their own relied on the Internet, and 33 percent found housing after spotting a “for rent” sign. A total of only three Hispanic households who undertook a self-guided search used the Internet. The majority of them (55 percent) found housing through “for rent” signs. Only 15 percent of black households who executed an independent search relied on the Internet. A third found housing through “for rent” signs and an additional third through the newspaper or other print media. Except for white renters, looking for rental housing was largely an un-digital affair.
Figure 7.1 indicates that the ways renters found housing might influence how they perceive their neighborhoods. The difference is especially acute when it comes to trusting one’s neighbors. Roughly a third of renters who located housing independently reported high levels of neighborhood trust; the same was true for 29 percent of renters who located housing through social networks. However, only 9.5 percent of renters who found housing through an agency reported high levels of neighborhood trust, a statistically significant difference when compared to non-agency search methods (p = 0.04). Surprisingly, the reverse pattern was observed with respect to perceived suffering, with renters who found housing independently and through networks being roughly twice as likely to report suffering in their communities, compared to renters who relied on agencies to locate housing.
Regarding housing quality, 44 percent of Milwaukee renters reported experiencing at least one significant and lasting housing problem. Twenty-one percent of renters reported one problem; 16 percent reported two; and 7 percent reported three or more. Black and Hispanic renters were more likely to live in poor-quality housing, with 52 percent and 42 percent, respectively, experiencing any housing problem, compared to 37 percent of white renters. Housing problems affected renters across the city, particularly those in poor communities. Forty-two percent of renters in neighborhoods with poverty rates below 20 percent reported housing problems, compared to 58 percent of renters in all other neighborhoods.
While more than a third of renters who experienced no housing problems reported high levels of trust in their neighbors, the same was true for less than a quarter of those who experienced at least one housing problem, a statistically significant difference (p = 0.03). Likewise, while 18 percent of renters who lived in decent conditions perceived suffering in their community, 26 percent of those who reported at least one lasting housing problem did.
Multivariate Models
To further examine these patterns in a multivariate framework, I employed logistic and negative binomial regression analyses. Table 7.2 displays the results. Separate models were estimated for each of the three explanatory variables: eviction, housing search methods, and housing problems. Models 1 and 3 include the full suit of control variables; Models 2 and 4 also employ neighborhood fixed effects.
Table 7.2: Logistic and Negative Binomial Regression Models Estimating Neighborhood Perceptions
Neighborhood Trust | Perceived Suffering | |||
---|---|---|---|---|
(1) | (2) | (3) | (4) | |
Previous Move Was Forced | −0.360 | −0.171 | 0.241* | 0.192 |
(0.234) | (0.259) | (0.106) | (0.123) | |
Found Housing through Agency | −1.406* | −1.236* | −0.245 | −0.196 |
(0.549) | (0.567) | (0.205) | (0.210) | |
Found Housing through Networks | −0.075 | 0.095 | −0.043 | −0.068 |
(0.156) | (0.173) | (0.083) | (0.090) | |
Lasting Housing Problems | −0.250*** | −0.271*** | 0.174*** | 0.185*** |
(0.066) | (0.074) | (0.023) | (0.027) |
Note: Milwaukee Area Renters Study, N = 1,086. Logistic regression models are used when estimating perceived trust; negative binomial regression models are used when estimating perceived suffering. Separate regressions were used to estimate the association between eviction, housing search methods, and housing problems on neighborhood perceptions. Models 2 and 4 include neighborhood fixed effects. All models control for months in neighborhood; miles from previous residence; the race, age, gender, and education of respondents; if respondents live with other adults or children; if respondents recently experienced job loss or relationship dissolution; how many of each respondent’s strong ties live in the neighborhood; and the neighborhood (block group) poverty rate.
* p < 0.05; ** p < 0.01; *** p < 0.001 (two-tailed)
Table 7.2 shows that renters whose previous move was forced reported significantly higher levels of perceived neighborhood suffering, all else equal. A previous eviction is estimated to increase the likelihood that renters will perceive suffering in their neighborhood by 27 percent. However, the association between eviction and perceived suffering becomes insignificant when neighborhood fixed effects are introduced. No model documented a statistically significant relationship between eviction and neighborhood trust.
I did, however, document such a relationship when the explanatory variable was housing search strategies. Specifically, renters who located housing through agencies expressed lower levels of neighborhood trust. All else equal, finding housing through an agency is predicted to reduce renters’ levels of neighborhood trust by 71 percent, a finding robust to neighborhood fixed effects. No relationship between housing search strategies and perceived neighborhood suffering was documented.
My findings also indicate that housing problems are associated with lower levels of perceived neighborhood trust and higher levels of perceived neighborhood suffering. After controlling for several relevant factors and including neighborhood fixed effects, each lasting housing problem a renter experiences is expected to decrease her or his odds of trusting neighbors by 24 percent and increase her or his level of perceived suffering by 20 percent. Renters who lived with more housing problems thought less of their neighborhoods.
All else equal, older renters and those with at least some college education reported higher levels of neighborhood trust, while Hispanic renters expressed lower levels of perceived suffering (results available upon request). Renters who had lived in the neighborhood longer and who counted more of their neighbors among their closest family members and friends reported higher levels of perceived suffering. This suggests that those who spend more time in a neighborhood and who are intimately connected with their neighbors may have a heightened sensibility of the adversity surrounding them. That housing dynamics remained a significant and substantially large predictor of more negative neighborhood perceptions after a number of relevant controls were introduced indicates that the circumstances that led families to select into a neighborhood, how they selected in, and the conditions of their home are critically important to understanding how they see their local community.
Robustness Checks
Across models, the neighborhood poverty rate was negatively associated with perceived trust and positively associated with perceived suffering, indicating that renters living in more economically disadvantaged neighborhoods harbor dimmer views of their community. To test whether the results were robust to alternative ecological specifications, I replicated the fixed effects models displayed in Table 7.2, replacing block-group poverty rate with three alternative neighborhood-level measures. The first was Observed Disorder, a binary variable that takes the value of 1 if survey interviewers documented abandoned buildings and litter on a respondent’s street. Renters’ perception of their community might be dragged down if they lived on streets displaying visible signs of distress.
I also substituted neighborhood poverty with Violent Crime rates, the latter being among the most important indicators of neighborhood disadvantage (Sampson Reference Sampson2012; Wilson Reference Wilson1987) and may affect cognitive functioning (Margolin and Gordis Reference Margolin and Gordis2000; Sharkey and Sampson Reference Sharkey, Sampson, Schutt, Keshavan and Seidman2015) Drawing on data supplied by the Milwaukee Police Department, I estimated each neighborhood’s violent crime rate as the sum of all counts of homicide, kidnapping, assault, arson, robbery, and weapon-related incidents per 100 people in the year a renter was surveyed.
Last, I created the composite variable, Neighborhood Disadvantage, via factor analysis, loading seven block-group characteristics onto a single scale: median household income, violent crime rate, and the percentages of families below the poverty line, of the population under 18, of residents with less than a high school education, of residents receiving public assistance, and of vacant housing units. This measure provides a more comprehensive estimate of neighborhood quality.
Separate models were estimated for each neighborhood-level control. The results are displayed in Table 7.3. In addition to using neighborhood fixed effects, these models include all individual- and household-level control variables employed in Table 7.2. The results are robust to alternative neighborhood specifications. Across all models, renters who worked with an agency to find housing reported lower levels of neighborhood trust, and those living in substandard conditions expressed more negative views of their local community.
Table 7.3: Fixed Effects Models with Alternative Neighborhood Controls
Observed Disorder | Violent Crime | Neighborhood Disadvantage | |
---|---|---|---|
Neighborhood Trust | |||
Previous Move Was Forced | −0.177 | −0.145 | −0.126 |
(0.267) | (0.260) | (0.260) | |
Found Housing through Agency | −1.104+ | −1.251* | −1.252* |
(0.574) | (0.568) | (0.570) | |
Lasting Housing Problems | −0.251** | −0.270*** | −0.270*** |
(0.074) | (0.074) | (0.074) | |
Perceived Suffering | |||
Previous Move Was Forced | 0.200 | 0.182 | 0.180 |
(0.124) | (0.124) | (0.123) | |
Found Housing through Agency | −0.207 | −0.201 | −0.191 |
(0.216) | (0.211) | (0.210) | |
Lasting Housing Problems | 0.190*** | 0.182*** | 0.183*** |
(0.027) | (0.027) | (0.027) |
Note: Milwaukee Area Renters Study, N = 1,086. Logistic regression models are used when estimating perceived trust; negative binomial regression models are used when estimating perceived suffering. These models include all individual- and household-level control variables used in Table 7.2. Observed Disorder = 1 if survey interviewers noticed abandoned buildings and litter on a given block. Violent Crime is the sum of all counts of homicide, kidnapping, assault, arson, robbery, and weapon-related incidents (categories based on Incident-Based Reporting codes) per 100 people per year. Neighborhood Disadvantage is a composite variable created via factor analysis, with seven block-group characteristics loading onto a single scale: median household income, violent crime rate, and the percentages of families below the poverty line, of the population under 18, of residents with less than a high school education, of residents receiving public assistance, and of vacant housing units.
+ p < 0.1; * p < 0.05; ** p < 0.01; *** p < 0.001 (two-tailed)
As a final robustness check, I employed propensity score matching to estimate differences in neighborhood trust and perceived suffering by eviction, agency-based housing searches, and experiencing at least one housing problem. The results are displayed in Table 7.4. The findings indicate that agency-based searches and housing problems are negatively related to neighborhood trust, while previous involuntary moves and housing problems are positively associated with perceived suffering.
Table 7.4: Propensity Score Matching Estimates, Average Treatment Effects
Neighborhood Trust | Perceived Suffering | |
---|---|---|
Previous Move Was Forced | 0.041 | 0.348* |
(0.061) | (0.173) | |
Found Housing through Agency | −0.224*** | −0.277 |
(0.036) | (0.198) | |
Lasting Housing Problems | −0.098** | 0.619*** |
(0.031) | (0.113) |
Note: Milwaukee Area Renters Study, N = 1,086. Here, Lasting Housing Problems is a binary variable, with 1 indicating having experienced any problems.
* p < 0.05; ** p < 0.01; *** p < 0.001 (two-tailed)
Discussion
Most of the chapters in this volume focus on housing markets and their regulation, with the primary actors being real estate investors, policy makers and enforcers, and homeowners. This study, by contrast, increased the magnification to focus on the relationship between housing and neighborhood dynamics in an American city, understood through the experiences of urban renters, many of whom live below the poverty line. An analysis of a unique dataset of Milwaukee renters found that those who had relocated to their neighborhood after an eviction or other kind of involuntary displacement, located their apartments through a government or nonprofit agency, or experienced multiple housing problems saw their neighborhood in a lesser light. With the exception of the finding pertaining to the estimated effect of eviction on neighborhood perceptions, the results of this study are robust to multiple measures of neighborhood quality as well as to neighborhood fixed effects.
What mechanisms help explain these patterns? Consider, first, the relationship between eviction and negative neighborhood perceptions. This study found some evidence that renters whose previous move was involuntary reported higher levels of perceived suffering, although this finding was not robust to neighborhood fixed effects specifications. Eviction can be a demoralizing process involving families being forced from a community in which they were invested to a neighborhood they consider undesirable (Desmond Reference Desmond2016a). If processed through the court system, an eviction comes with a record, which can result in families moving into worse neighborhoods and substandard housing (Desmond and Shollenberger Reference Desmond and Shollenberger2015). Even if evicted families relocate to equivalent housing and similar neighborhoods, they may still feel that their surroundings are of lower quality because they were accepted under acute duress; after all, the effect of eviction on local trust remained after controlling for neighborhood poverty rate and housing quality. Alternatively, eviction can affect one’s mental health, heightening depressive symptoms and stress levels (Desmond and Kimbro Reference Desmond and Kimbro2015), which could cast a pall over renters’ outlooks in general, including their views on the local community.
A more puzzling finding, however, concerns the observation that renters who located their housing through a government or nonprofit agency had a dimmer view of their neighborhoods. These renters did live in more disadvantaged neighborhoods: the poverty rate for the average agency-assisted renter was 28 percent, compared to 11 percent for all other renters. However, the link between locating housing through a third party and lower levels of community trust remained after conditioning on several ecological characterizes and including neighborhood fixed effects. When attempting to understand this pattern, it is important to recognize, first, that the vast majority of these renters (91 percent) did not receive housing assistance. So this finding should not be interpreted as reflecting the perceptions of voucher holders. On the contrary, two-thirds of renters who located housing through a government or community organization relied on a nonprofit agency; and roughly 40 percent sought help from the Housing Authority. By and large, then, most renters who located housing through a government or nonprofit agency did not receive additional help, like rent assistance, and relied on nonprofit organizations.
To further investigate possible dynamics beneath this pattern, I examined the reasons these renters offered as to why they moved into their neighborhoods. Some renters who relied on agencies to find housing expressed a lack of options when it came to neighborhood choice. “This is what the Housing Authority had open,” one renter said. “Couldn’t find anywhere else to go,” said another. As these comments attest, finding housing through an agency can be a stressful and rushed experience, owing to time limits affixed to assistance and limited staff capacity. This may influence how renters see the neighborhood into which they eventually select.
This finding suggests a pair of implications for policy makers. First, expanding a city’s stock of temporary housing would allow housing organizations to operate with more slack. When the alternative is homelessness, an organization assisting a family in need is more likely to identify housing options quickly and to encourage clients to accept whatever is available. However, if that family were able to stay in temporary housing for some duration of time, organizations could provide families more housing options. This could boost low-income families’ neighborhood quality and their level of community trust. Agencies assisting families in crisis operate under considerable pressure not only because those families have an acute need to be housed quickly, but also because many landlords turn away assisted renters. Landlords in most states are not obligated to accept families with housing vouchers, for example, and many make inferences about the quality of tenants based on their relationships with government or nonprofit agencies (DeLuca, Garboden, and Rosenblatt Reference DeLuca, Garboden and Rosenblatt2013). A second policy implication from this study, then, has to do with expanding and enforcing source-of-income discrimination laws, which would increase the housing and neighborhood options of low-income, assisted families.
Besides renters’ motivations for moving and strategies for locating housing, the quality of their dwellings also appears to color their neighborhood perceptions. Renters who experienced more housing problems were far less likely to trust their neighbors and more likely to perceive suffering around them. As suggested earlier, a potential explanation for this finding pertains to neighborhood usage. Renters living in worse housing conditions might spend less time in their homes and more on the street than those in higher-quality housing. If this were the case, we might expect housing problems to be positively associated with indicators of neighborhood involvement or exposure. Following this line of thought, I replicated the fixed effects negative binomial regression model displayed in Table 7.2 on a new outcome: Local Assistance, a measure of how meaningfully engaged renters were with their neighbors. Respondents were asked: “While you have been living in this neighborhood, have you ever helped a neighbor (1) pay bills or buy groceries; (2) get a job; (3) fix their house or their car; (4) by supporting them emotionally, as they went through a hard time; or (5) by watching their kids?” Answers were summed to create a measure of local assistance (min = 0, max = 5). The models found that renters who experienced more housing problems reported higher levels of local assistance (b = 0.086; p < 0.001). This finding is robust to the three alternative measures of neighborhood quality discussed earlier.
Material hardship could be the underlying cause of both heightened exposure to housing problems and neighborhood engagement, relied on to make ends meet. All else equal, renters who live in disadvantaged neighborhoods report higher levels of local assistance (Desmond and An 2015). However, I observed a significant association between housing problems and neighborhood engagement net of indicators of material hardship, such as having experienced a recent job loss or breakup, as well as controls for the length of time and the number of strong ties in a community and neighborhood fixed effects. Renters who have experienced more housing problems report higher levels of neighborhood involvement, compared to similar renters in similar neighborhoods. This suggests that housing problems spur community exposure, as renters leave their homes to escape degrading conditions or to address such conditions by seeking help from neighbors.
This study’s identification strategy pertains to similar renters in similar neighborhoods having different housing-related experiences that influence how they perceive their local community. Those perceptions are steered not only by objective neighborhood disadvantage, like crime, but also by multiple housing dynamics: why city dwellers moved, how they found subsequent housing, and the conditions of their dwelling. A neighborhood’s outlook, whether it will improve or decline, depends in significant part on how its residents see it. If people see their community as a special, cherished thing, they will work to protect and improve it (Fischel, Chapter 1, this volume). However, if they fear their neighborhood or become ashamed of it, they will look for ways to leave or burrow behind locked doors, ignoring the streets around them (Rosenblatt and DeLuca Reference Rosenblatt and DeLuca2012). When neighbors work together, they can improve their community and drive down crime by establishing effective local practices or lobbying elected representatives. “Our failures with city neighborhoods are, ultimately, failures in localized self-government,” wrote Jane Jacobs. “And our successes are successes at localized self-government” (Reference Jacobs1961, 114).
A prerequisite for successful self-management is the cultivation of a palpable optimism about the capacity of the local community (McAdam Reference McAdam1982; Piven and Cloward Reference Piven and Cloward1977), a belief in and familiarity with one’s neighbors. But negative neighborhood perceptions can compromise a community’s civic efficacy and thwart community-based organizing (Desmond and Travis Reference Desmond and Travis2016; Sampson Reference Sampson2012), directly contributing to neighborhood decline through depopulation (when families move out) and disinvestment (when families withdraw from their own community). It follows, then, that those hoping to identify ways to cultivate collective efficacy and raise people’s expectations of their community should strive to apprehend why people see their neighborhood in this or that light.
The findings of this study indicate that if we wish to understand neighborhood perceptions, we have to pay attention to housing dynamics. A long-standing interest in housing discrimination and gentrification notwithstanding (Massey and Denton Reference Massey and Denton1993; South and Crowder Reference South and Crowder1997), research on neighborhood effects largely overlooks housing dynamics. Because city dwellers’ immediate environment – the house – is fundamentally linked to their broader ecological surroundings, future investigations into the role housing markets play in shaping neighborhood perceptions and local dynamics could advance urban studies in significant ways. This chapter has contributed to uniting the sociology of housing and of neighborhoods, but much work remains undone. Indeed, many chapters in this volume reflect the tendency of research on housing markets to ignore neighborhood characteristics, which in turn are critical to understanding market dynamics. For example, despite the fact that racial residential segregation directly contributed to the foreclosure crisis (Rugh and Massey Reference Rugh and Massey2010), scholarship on the crisis trains most of its attention on regulatory failures and financial instruments.
If housing and neighborhood dynamics are bound together in a tight knot, then policy interventions focused on one will likely have an effect on the other. The findings of this chapter indicate that initiatives designed to prevent eviction, for example, not only could promote family stability and well-being, but may also promote community investment since renters that select into neighborhoods under more voluntary circumstances see their communities in a more positive light. Similarly, if housing problems color renters’ perceptions of their neighborhoods, then initiatives designed to improve housing quality could benefit not just individuals but communities as well.
Negative neighborhood perceptions can thwart community cohesion, impede the formation of social capital, spur residential turnover, and invite social problems. Addressing these issues by confronting such perceptions requires understanding the factors that deeply influence how we see our communities. When it comes to promoting neighborhood trust and pride, a good place to start is the home.
Author’s Note
I thank Kathleen Cagney, Lee Anne Fennell, Benjamin Keys, and participants at the Kreisman Initiative on Housing Law and Policy, University of Chicago Law School, for critical feedback on earlier drafts. This research was supported by the John D. and Catherine T. MacArthur Foundation, through its “How Housing Matters” initiative.