Book contents
- Frontmatter
- Contents
- List of contributors
- Preface
- 1 Inference and estimation in probabilistic time series models
- I Monte Carlo
- 2 Adaptive Markov chain Monte Carlo: theory and methods
- 3 Auxiliary particle filtering: recent developments
- 4 Monte Carlo probabilistic inference for diffusion processes: a methodological framework
- II Deterministic approximations
- III Switching models
- IV Multi-object models
- V Nonparametric models
- VI Agent-based models
- Index
- Plate section
- References
4 - Monte Carlo probabilistic inference for diffusion processes: a methodological framework
from I - Monte Carlo
Published online by Cambridge University Press: 07 September 2011
- Frontmatter
- Contents
- List of contributors
- Preface
- 1 Inference and estimation in probabilistic time series models
- I Monte Carlo
- 2 Adaptive Markov chain Monte Carlo: theory and methods
- 3 Auxiliary particle filtering: recent developments
- 4 Monte Carlo probabilistic inference for diffusion processes: a methodological framework
- II Deterministic approximations
- III Switching models
- IV Multi-object models
- V Nonparametric models
- VI Agent-based models
- Index
- Plate section
- References
Summary
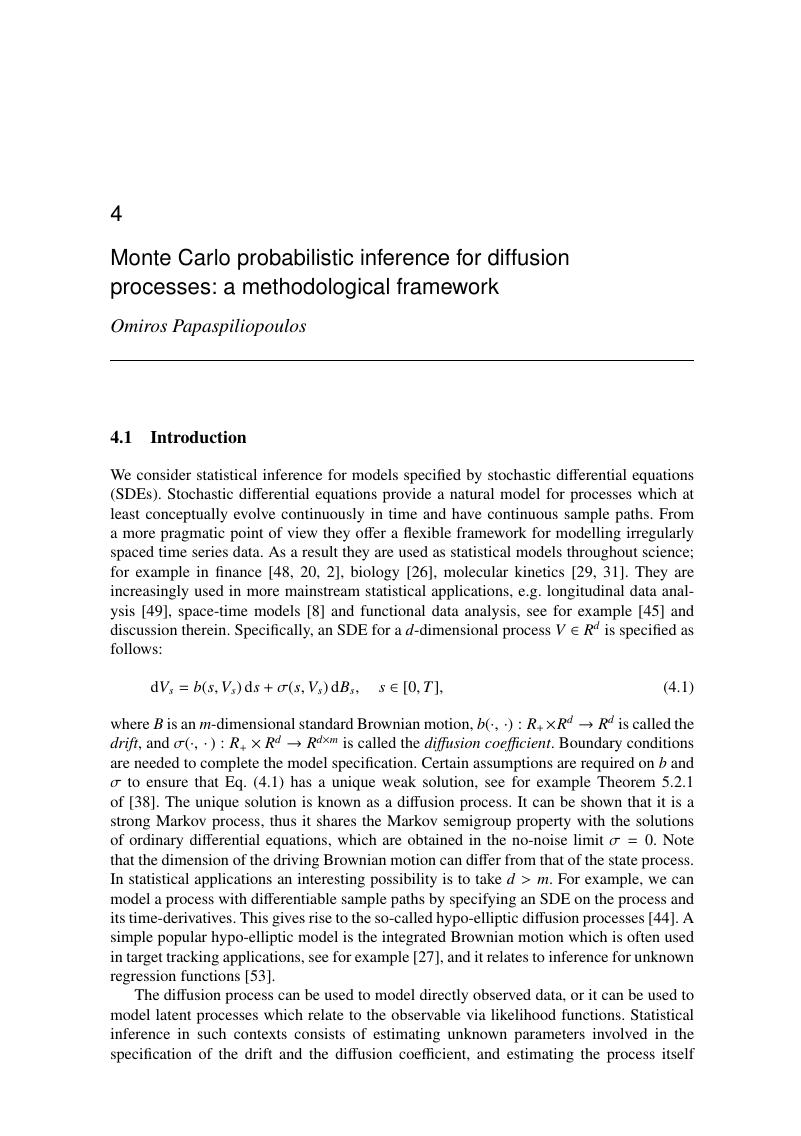
- Type
- Chapter
- Information
- Bayesian Time Series Models , pp. 82 - 103Publisher: Cambridge University PressPrint publication year: 2011
References
- 7
- Cited by