Book contents
- Frontmatter
- Dedication
- Contents
- Notation
- Preface
- 1 Introduction
- 2 Limiting Spectral Distributions
- 3 CLT for Linear Spectral Statistics
- 4 The Generalised Variance and Multiple Correlation Coefficient
- 5 The T2-Statistic
- 6 Classification of Data
- 7 Testing the General Linear Hypothesis
- 8 Testing Independence of Sets of Variates
- 9 Testing Hypotheses of Equality of Covariance Matrices
- 10 Estimation of the Population Spectral Distribution
- 11 Large-Dimensional Spiked Population Models
- 12 Efficient Optimisation of a Large Financial Portfolio
- Appendix A Curvilinear Integrals
- Appendix B Eigenvalue Inequalities
- References
- Index
- References
References
- Frontmatter
- Dedication
- Contents
- Notation
- Preface
- 1 Introduction
- 2 Limiting Spectral Distributions
- 3 CLT for Linear Spectral Statistics
- 4 The Generalised Variance and Multiple Correlation Coefficient
- 5 The T2-Statistic
- 6 Classification of Data
- 7 Testing the General Linear Hypothesis
- 8 Testing Independence of Sets of Variates
- 9 Testing Hypotheses of Equality of Covariance Matrices
- 10 Estimation of the Population Spectral Distribution
- 11 Large-Dimensional Spiked Population Models
- 12 Efficient Optimisation of a Large Financial Portfolio
- Appendix A Curvilinear Integrals
- Appendix B Eigenvalue Inequalities
- References
- Index
- References
Summary
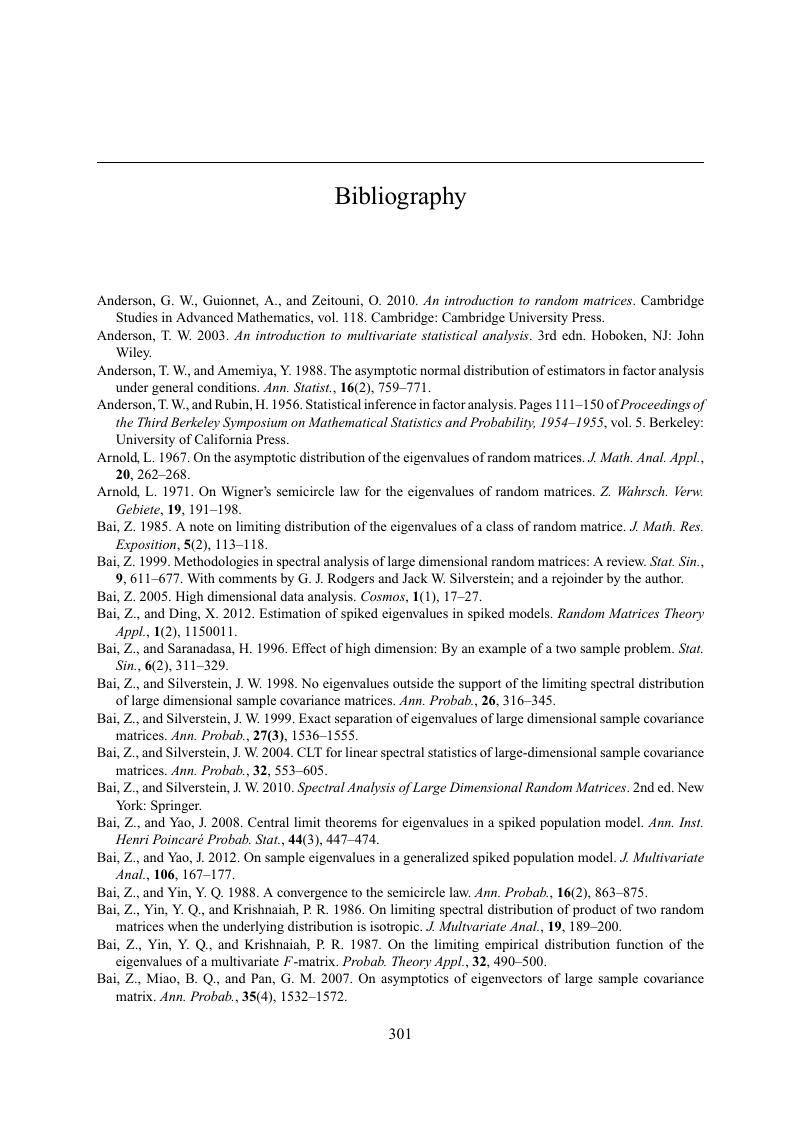
- Type
- Chapter
- Information
- Publisher: Cambridge University PressPrint publication year: 2015