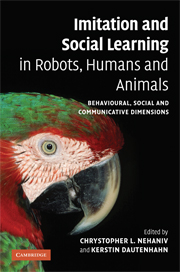
Book contents
- Frontmatter
- Contents
- List of plates
- List of figures
- List of tables
- List of contributors
- Introduction: the constructive interdisciplinary viewpoint for understanding mechanisms and models of imitation and social learning
- Part I Correspondence problems and mechanisms
- Part II Mirroring and ‘mind-reading’
- Part III What to imitate?
- Part IV Development and embodiment
- Part V Synchrony and turn-taking as communicative mechanisms
- Part VI Why imitate? – Motivations
- Part VII Social feedback
- 18 Training behavior by imitation: from parrots to people … to robots?
- 19 Task learning through imitation and human–robot interaction
- Part VIII The ecological context
- Index
- Plate section
- References
19 - Task learning through imitation and human–robot interaction
Published online by Cambridge University Press: 10 December 2009
- Frontmatter
- Contents
- List of plates
- List of figures
- List of tables
- List of contributors
- Introduction: the constructive interdisciplinary viewpoint for understanding mechanisms and models of imitation and social learning
- Part I Correspondence problems and mechanisms
- Part II Mirroring and ‘mind-reading’
- Part III What to imitate?
- Part IV Development and embodiment
- Part V Synchrony and turn-taking as communicative mechanisms
- Part VI Why imitate? – Motivations
- Part VII Social feedback
- 18 Training behavior by imitation: from parrots to people … to robots?
- 19 Task learning through imitation and human–robot interaction
- Part VIII The ecological context
- Index
- Plate section
- References
Summary
Introduction
Human–robot interaction is a rapidly growing area of robotics. Environments that feature the interaction of humans and robots present a number of challenges involving robot learning (imitative) and interactive capabilities. The two problems are tightly related since, on the one hand, social interaction is often an important aspect of imitation learning and, on the other, imitative behavior enhances a robot's social and interactive capabilities. In this work we present a framework that unifies these issues, providing a natural means for robots to interact with people and to learn from interactive experiences.
We focus on two major challenges. The first is the design of robot social capabilities that allow for engagement in various types of interactions. Examples include robot teachers (David and Ball, 1986), workers, team members (Matsui et al., 1997), museum tour-guides (Thrun et al., 1999), toys (Michaud and Caron, 2002), and emotional companions (Breazeal, 2002; Cañamero and Fredslund, 2000). Designing control architectures for such robots presents various, often domain-specific, challenges.
The second challenge we address is endowing robots with the ability to learn through social interaction with humans or other robots, in order to improve their performance and expand their capabilities. Learning by imitation (Dautenhahn and Nehaniv, 2002; Hayes and Demiris, 1994; Schaal, 1997) provides a most natural approach to this problem; methods using gestures (Voyles and Khosla, 1998), natural language (Lauria et al., 2002), and animal “clicker training” (Kaplan et al., 2002) have also been successfully applied.
- Type
- Chapter
- Information
- Imitation and Social Learning in Robots, Humans and AnimalsBehavioural, Social and Communicative Dimensions, pp. 407 - 424Publisher: Cambridge University PressPrint publication year: 2007
References
- 6
- Cited by