Book contents
- The Cambridge Handbook of Computational Cognitive Sciences
- Cambridge Handbooks in Psychology
- The Cambridge Handbook of Computational Cognitive Sciences
- Copyright page
- Contents
- Preface
- Contributors
- Part I Introduction
- Part II Cognitive Modeling Paradigms
- Part III Computational Modeling of Basic Cognitive Functionalities
- Part IV Computational Modeling in Various Cognitive Fields
- Part V General Discussion
- Index
- References
Part IV - Computational Modeling in Various Cognitive Fields
Published online by Cambridge University Press: 21 April 2023
- The Cambridge Handbook of Computational Cognitive Sciences
- Cambridge Handbooks in Psychology
- The Cambridge Handbook of Computational Cognitive Sciences
- Copyright page
- Contents
- Preface
- Contributors
- Part I Introduction
- Part II Cognitive Modeling Paradigms
- Part III Computational Modeling of Basic Cognitive Functionalities
- Part IV Computational Modeling in Various Cognitive Fields
- Part V General Discussion
- Index
- References
Summary
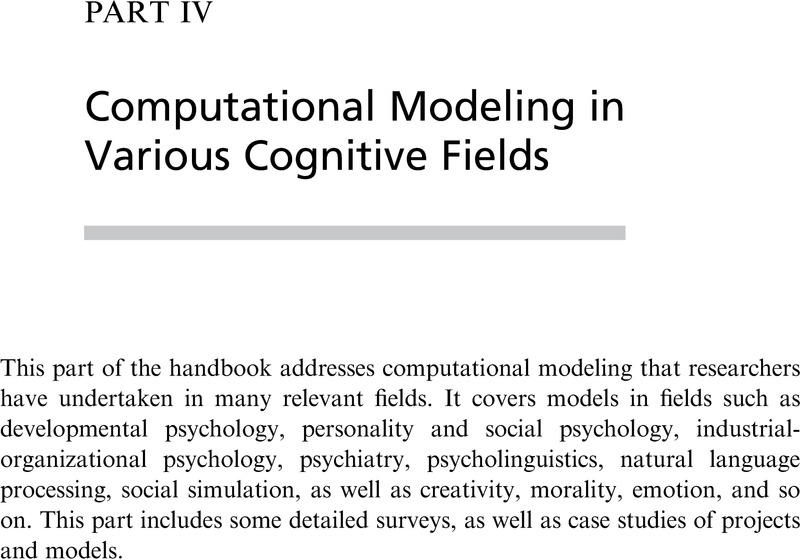
- Type
- Chapter
- Information
- The Cambridge Handbook of Computational Cognitive Sciences , pp. 767 - 1162Publisher: Cambridge University PressPrint publication year: 2023