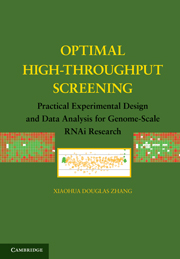
References
Published online by Cambridge University Press: 03 May 2011
Summary
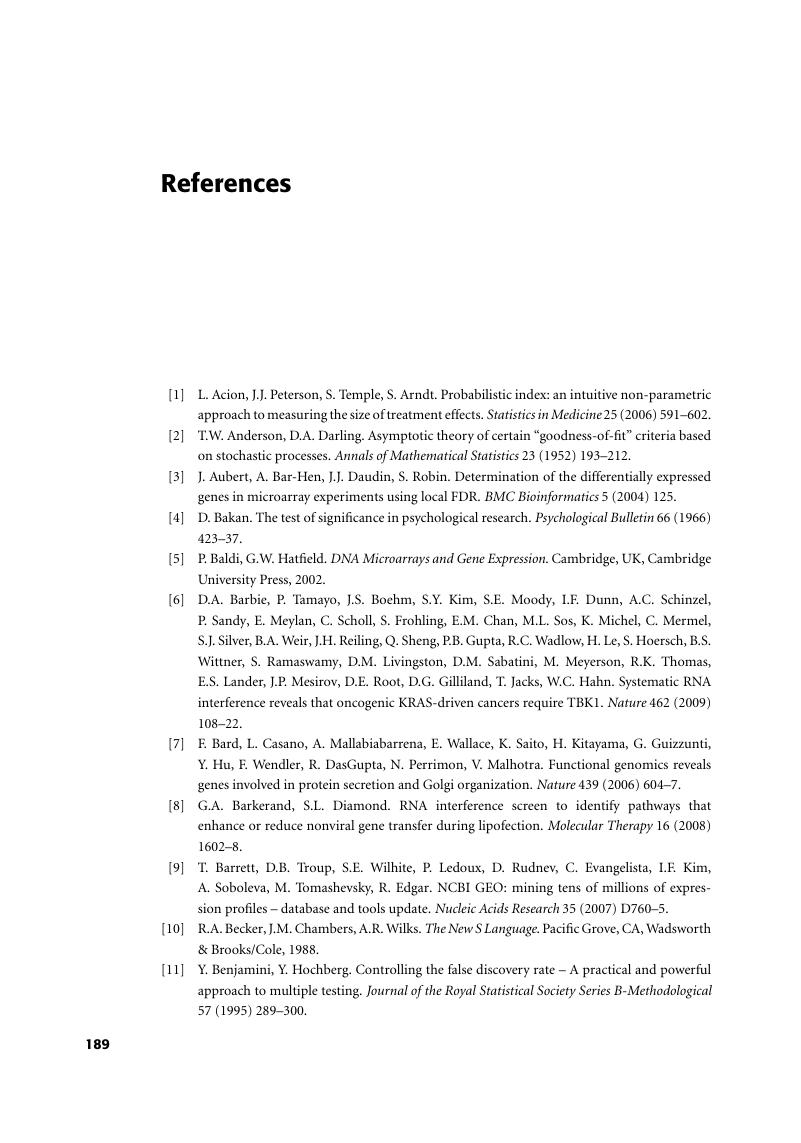
- Type
- Chapter
- Information
- Optimal High-Throughput ScreeningPractical Experimental Design and Data Analysis for Genome-Scale RNAi Research, pp. 189 - 200Publisher: Cambridge University PressPrint publication year: 2011