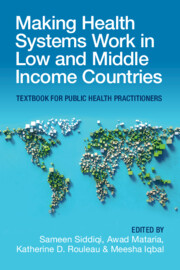
Book contents
- Making Health Systems Work in Low and Middle Income Countries
- Reviews
- Making Health Systems Work in Low and Middle Income Countries
- Copyright page
- Dedication
- Contents
- About the Editors
- Contributors
- Preface
- Section 1 Analyzing Health Systems: Concepts, Components, Performance
- Section 2 Transforming Health Systems: Confronting Challenges, Seizing Opportunities
- Chapter 15 Universal Health Coverage and Beyond
- Chapter 16 Pro-Poor Expansion of Universal Health Coverage
- Chapter 17 Health Insurance for Advancing Universal Health Coverage
- Chapter 18 From Passive to Strategic Purchasing in Low and Middle Income Countries
- Chapter 19 Good Governance and Leadership for Better Health Systems
- Chapter 20 Developing a Balanced Health Workforce
- Chapter 21 Enhancing Equitable Access to Essential Medicines and Health Technologies
- Chapter 22 Health Information and Information Technology
- Chapter 23 Using Health Research for Evidence-Informed Decisions in Health Systems in L&MICs
- Chapter 24 Integrated People-Centered Health Care
- Chapter 25 Strengthening Hospital Governance and Management to Become High-Performing Organizations
- Chapter 26 Improving the Quality and Safety of Health Care in Low and Middle Income Countries
- Chapter 27 Harnessing the Contribution of the Private Health Care Sector toward Public Health Goals
- Chapter 28 Public–Private Partnership in Health Care Services
- Chapter 29 Embedding People’s Voice and Ensuring Participatory Governance
- Chapter 30 Achieving Health-Related Sustainable Development Goals
- Chapter 31 The Determinants of Health Systems
- Chapter 32 Integrating Essential Public Health Functions in Health Systems
- Chapter 33 Engaging in a Health Care Recovery Process
- Chapter 34 Health System Response to the COVID-19 Pandemic
- Chapter 35 Understanding the Global Health Architecture
- Chapter 36 Political Economy of Health Reforms in Low and Middle Income Countries
- Chapter 37 Better Health Systems for Better Outcomes
- Index
- References
Chapter 22 - Health Information and Information Technology
The Path from Data to Decision
from Section 2 - Transforming Health Systems: Confronting Challenges, Seizing Opportunities
Published online by Cambridge University Press: 08 December 2022
- Making Health Systems Work in Low and Middle Income Countries
- Reviews
- Making Health Systems Work in Low and Middle Income Countries
- Copyright page
- Dedication
- Contents
- About the Editors
- Contributors
- Preface
- Section 1 Analyzing Health Systems: Concepts, Components, Performance
- Section 2 Transforming Health Systems: Confronting Challenges, Seizing Opportunities
- Chapter 15 Universal Health Coverage and Beyond
- Chapter 16 Pro-Poor Expansion of Universal Health Coverage
- Chapter 17 Health Insurance for Advancing Universal Health Coverage
- Chapter 18 From Passive to Strategic Purchasing in Low and Middle Income Countries
- Chapter 19 Good Governance and Leadership for Better Health Systems
- Chapter 20 Developing a Balanced Health Workforce
- Chapter 21 Enhancing Equitable Access to Essential Medicines and Health Technologies
- Chapter 22 Health Information and Information Technology
- Chapter 23 Using Health Research for Evidence-Informed Decisions in Health Systems in L&MICs
- Chapter 24 Integrated People-Centered Health Care
- Chapter 25 Strengthening Hospital Governance and Management to Become High-Performing Organizations
- Chapter 26 Improving the Quality and Safety of Health Care in Low and Middle Income Countries
- Chapter 27 Harnessing the Contribution of the Private Health Care Sector toward Public Health Goals
- Chapter 28 Public–Private Partnership in Health Care Services
- Chapter 29 Embedding People’s Voice and Ensuring Participatory Governance
- Chapter 30 Achieving Health-Related Sustainable Development Goals
- Chapter 31 The Determinants of Health Systems
- Chapter 32 Integrating Essential Public Health Functions in Health Systems
- Chapter 33 Engaging in a Health Care Recovery Process
- Chapter 34 Health System Response to the COVID-19 Pandemic
- Chapter 35 Understanding the Global Health Architecture
- Chapter 36 Political Economy of Health Reforms in Low and Middle Income Countries
- Chapter 37 Better Health Systems for Better Outcomes
- Index
- References
Summary
This Chapter describes principles of information management for health systems and the need to focus on key data items required to improve individual and population health. It discusses the collection and analysis of relevant, high-quality data and the importance of agreeing on health programme aims before defining the minimum data set. We review the derivation of health indicators, focusing on WHO indicators. Many indicators rely on linking data from different sources, which requires accurate personal identifiers. Data is useless unless reports based on it can be shared and understood, so data analysts should use different visualization techniques to facilitate and support user decisions such as self-service dashboards. We also review the many high quality, open source, free to use data capture, analysis and data sharing tools that can support health systems, concluding that it is rarely necessary to develop an information system from scratch. Finally, while big data analytics, artificial intelligence and machine learning capture many headlines, health system can achieve much using simple tools to capture relevant, high-quality data and turn it into actionable knowledge to support their decision makers.
Keywords
- Type
- Chapter
- Information
- Making Health Systems Work in Low and Middle Income CountriesTextbook for Public Health Practitioners, pp. 336 - 353Publisher: Cambridge University PressPrint publication year: 2022