References
Published online by Cambridge University Press: 28 April 2022
Summary
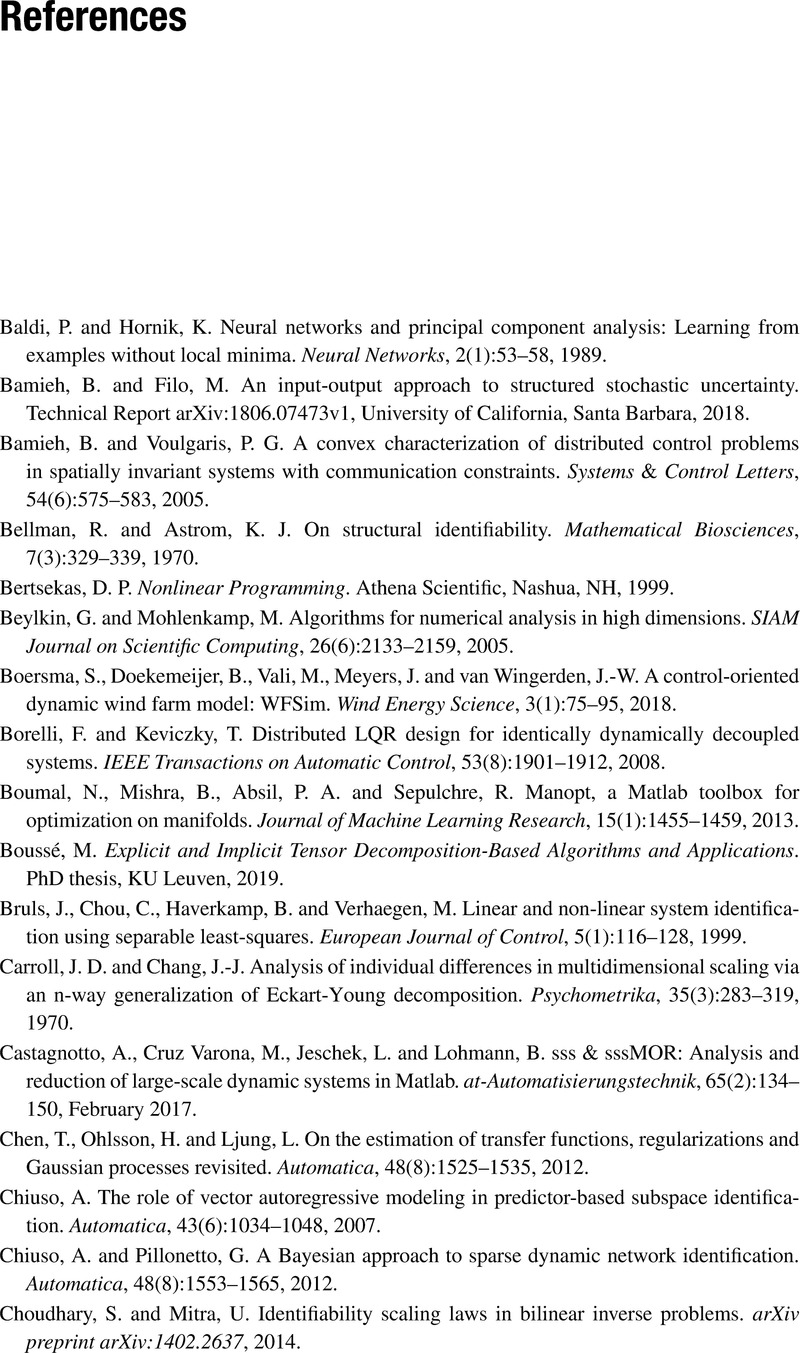
- Type
- Chapter
- Information
- Data-Driven Identification of Networks of Dynamic Systems , pp. 257 - 265Publisher: Cambridge University PressPrint publication year: 2022