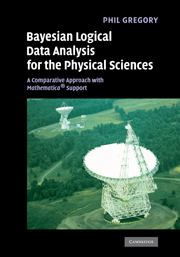
Book contents
- Frontmatter
- Contents
- Preface
- Acknowledgements
- 1 Role of probability theory in science
- 2 Probability theory as extended logic
- 3 The how-to of Bayesian inference
- 4 Assigning probabilities
- 5 Frequentist statistical inference
- 6 What is a statistic?
- 7 Frequentist hypothesis testing
- 8 Maximum entropy probabilities
- 9 Bayesian inference with Gaussian errors
- 10 Linear model fitting (Gaussian errors)
- 11 Nonlinear model fitting
- 12 Markov chain Monte Carlo
- 13 Bayesian revolution in spectral analysis
- 14 Bayesian inference with Poisson sampling
- Appendix A Singular value decomposition
- Appendix B Discrete Fourier Transforms
- Appendix C Difference in two samples
- Appendix D Poisson ON/OFF details
- Appendix E Multivariate Gaussian from maximum entropy
- References
- Index
12 - Markov chain Monte Carlo
Published online by Cambridge University Press: 05 September 2012
- Frontmatter
- Contents
- Preface
- Acknowledgements
- 1 Role of probability theory in science
- 2 Probability theory as extended logic
- 3 The how-to of Bayesian inference
- 4 Assigning probabilities
- 5 Frequentist statistical inference
- 6 What is a statistic?
- 7 Frequentist hypothesis testing
- 8 Maximum entropy probabilities
- 9 Bayesian inference with Gaussian errors
- 10 Linear model fitting (Gaussian errors)
- 11 Nonlinear model fitting
- 12 Markov chain Monte Carlo
- 13 Bayesian revolution in spectral analysis
- 14 Bayesian inference with Poisson sampling
- Appendix A Singular value decomposition
- Appendix B Discrete Fourier Transforms
- Appendix C Difference in two samples
- Appendix D Poisson ON/OFF details
- Appendix E Multivariate Gaussian from maximum entropy
- References
- Index
Summary
Overview
In the last chapter, we discussed a variety of approaches to estimate the most probable set of parameters for nonlinear models. The primary rationale for these approaches is that they circumvent the need to carry out the multi-dimensional integrals required in a full Bayesian computation of the desired marginal posteriors. This chapter provides an introduction to a very efficient mathematical tool to estimate the desired posterior distributions for high-dimensional models that has been receiving a lot of attention recently. The method is known as Markov Chain Monte Carlo (MCMC). MCMC was first introduced in the early 1950s by statistical physicists (N. Metropolis, A. Rosenbluth, M. Rosenbluth, A. Teller, and E. Teller) as a method for the simulation of simple fluids. Monte Carlo methods are now widely employed in all areas of science and economics to simulate complex systems and to evaluate integrals in many dimensions. Among all Monte Carlo methods, MCMC provides an enormous scope for dealing with very complicated systems. In this chapter we will focus on its use in evaluating the multi-dimensional integrals required in a Bayesian analysis of models with many parameters.
The chapter starts with an introduction to Monte Carlo integration and examines how a Markov chain, implemented by the Metropolis–Hastings algorithm, can be employed to concentrate samples to regions with significant probability. Next, tempering improvements are investigated that prevent the MCMC from getting stuck in the region of a local peak in the probability distribution.
- Type
- Chapter
- Information
- Bayesian Logical Data Analysis for the Physical SciencesA Comparative Approach with Mathematica® Support, pp. 312 - 351Publisher: Cambridge University PressPrint publication year: 2005
- 1
- Cited by