Introduction
Climate change threatens the achievement of the sustainable development goals (SDGs), such as ensuring food security for all and promoting sustainable agriculture (SDG 2), empowering women and girls everywhere (SDG 5), and promoting actions at all levels to combat climate change (SDG 12). The livelihoods of rural households in sub-Saharan Africa are particularly vulnerable to climate change (Juana et al. Reference Juana, Kahaka and Okurut2013; Intergovernemental Panel on Climate Change [IPCC] 2014). Degradation of land resources combined with an increase in the frequency of extreme events climatic (higher temperatures, more frequent water stress, more pronounced floods, more violent early season winds) lead to a decline in agricultural production (Intergovernemental Panel on Climate Change [IPCC] 2014). This increased climate variability would make agricultural activities less productive. Farmers need to implement various adaptation strategies to reduce the negative impacts of climate change and maintain their livelihoods. Climate change adaptation strategies affect many different sectors of society and can vary significantly in terms of approach and impact on rural household livelihoods.
The impact of climate change on agricultural production threatens food security and income generation in Burkina Faso. The rural population of this country, like those of other sub-Saharan African countries, faces socioeconomic challenges triggered by climate change. Agriculture is the most vulnerable sector, as it contributes 40% of the Gross Domestic Product and is the main source of income and livelihoods for more than 90% of rural women (MECV 2007; Romero et al. Reference Romero, Belemvire and Saulière2011). In rural areas of Burkina Faso, where most agricultural production takes place, women farmers assume the triple responsibility of domestic, agricultural, and non-agricultural burden. In fact, women are responsible for providing the household with food, drinking water, and firewood. On farms, they weed, plow, sow, pick, and harvest agricultural products. In many rural areas of Burkina Faso and in some sub-Saharan African countries, social constructs confine women to the second tier of society with specific tasks (reproduction, housework, etc.) and limited access to resources (Doubogan et al. Reference Doubogan, Yotto and Yabi2016). Despite having multiple tasks, they have significantly less access to the resources and services they need to increase their productivity and income and reduce their domestic tasks (Fonds Internationnal de Développement Agricole [FIDA] 2011). Rural women farmers remain the most vulnerable to the negative impacts of climate change due to their high levels of poverty, traditional-socially assigned roles, and the discrimination they face (unequal distribution of rights, resources, and power, and repressive cultural rules and norms) (Romero et al. Reference Romero, Belemvire and Saulière2011; Doubogan et al. Reference Doubogan, Yotto and Yabi2016). Land managed by women is generally of lower quality than land managed by men (Romero et al. Reference Romero, Belemvire and Saulière2011). Several studies show that a women farmer is less likely to adopt adaptive practices to climate change than a man farmer (Deressa et al. Reference Deressa, Hassan, Ringler, Alemu and Yesuf2009; Yegbemey et al. Reference Yegbemey, Yabi, Tovignan, Gantoli and Haroll Kokoye2013; Diendéré Reference Diendéré2019).
The various factors that influence the choice of climate change adaptation strategies have been the subject of several studies. However, most of the prior investigations have only considered gender as a factor that can influence adaptive capacity, without focusing on women’s adaptation strategies per se (Deressa et al. Reference Deressa, Hassan, Ringler, Alemu and Yesuf2009; Diendéré Reference Diendéré2019; Yegbemey et al. Reference Yegbemey, Yabi, Tovignan, Gantoli and Haroll Kokoye2013). This represents a significant knowledge gap in the literature on climate change adaptation strategies. As innovators, organizers, leaders, and educators, they are uniquely positioned to contribute to adaptation to the negative impacts of climate change. Economic theories of innovation adoption have generated debates about the relative importance of certain socio-demographic, economic, and agricultural institutional characteristics (Roger Reference Roger1995; Feder et al. Reference Feder, Just and Zilberman1985; Hayami and Ruttan Reference Hayami and Ruttan1985; Davis Reference Davis1989; Feder and Umali Reference Feder and Umali1993; Sunding and Zilberman Reference Sunding and Zilberman2001). Empirical studies in different countries point to different determinants of the choice of climate change adaptation strategies (age, education level, wealth, labor force, perceptions, agricultural land, input costs, social capital, financing). However, due to differences in agro-ecological and socioeconomic conditions, it is difficult to specify factors that influence farmers’ adaptation decisions in different parts of the world. Moreover, the issue of adaptation strategies raises empirical controversies about the impact of such strategies on user income and food security (Mulumeoderhwa et al. Reference Mulumeoderhwa, Mugisho, Rushigira, Biganiro, Vwima and Mushagalusa2020; Zakari et al. Reference Zakari, Ibro, Moussa and Abdoulaye2022).
This study builds on this literature by examining new evidence on factors influencing (1) women farmers’ choice of adaptation strategies to reduce the negative impacts of climate change as well as (2) the impact of these strategies on household livelihoods. The study takes place in the Center-North region of Burkina Faso. This choice is due not only to the paucity of studies in the empirical literature on climate change in relation to gender but also to the high vulnerability of women farmers to climate change and the increasing feminization of agriculture due to the out-migration of men (Da et al. Reference Da, Yacouba and Yonkeu2008; Sarr et al. Reference Sarr, Salack, Sangar, Ly and Sanda2015). The Center-North region covers an area of 19,508 km2 or 7% of the national territory. The region has a Sahelo-Sudan type of climate, and its agricultural production is chronically insufficient. Due to agro-pedoclimatic constraints, large population boom, and urbanization, it is one of the regions with the largest deficits in cereal production. The population density is high, and the pressure on the soil is significant.
The objectives of this research are twofold: (i) to examine the main adaptation strategies adopted by women farmers in the Center-North region in the face of climate change and the factors influencing adaptation decisions and (ii) to assess the impacts of these strategies on household income and food security. The results could lead to the formulation of more targeted economic policies that would strengthen the resilience of these women farmers in the face of climate change.
The remainder of this study is organized into four parts. The first part briefly reviews the empirical literature on the adoption of agricultural innovations. The second part describes the study area and the sample of women farmers interviewed. The third part presents the methods used to analyze the data collected. The fourth part presents and discusses the empirical findings.
Adoption of adaptation strategies: a brief empirical review
Adaptation can be defined as an adjustment to reduce vulnerability to change in specific communities (Intergovernemental Panel on Climate Change [IPCC] 2014 [IPCC] 2022). Adaptation to climate change means reducing vulnerability to current and future climate risks. This vulnerability is largely determined by the adaptive capacity of individuals. A given climate event does not affect all members of a community or even a family in the same way, because some people have a greater capacity to cope with a crisis than others do. Adaptation to climate change thus depends on a number of factors.
Determinants of the adoption of adaptation strategies
Many investigations mention the importance of farmers’ characteristics on the adoption of climate change adaptation measures. Deressa et al. (Reference Deressa, Hassan, Ringler, Alemu and Yesuf2009) show that age has a positive influence on farmers’ adaptation decision. Huffman (Reference Huffman2020) indicates that education level improves farmers’ ability to learn and acquire information and knowledge about climate change and adaptation technologies. Diendéré et al. (Reference Diendéré, Nguyen, Del Corso and Kephaliacos2018) conclude that better knowledge of climate phenomena as well as agricultural technologies may facilitate adaptation. Climate change adaptation opportunities are also closely related to household size. For instance, Khatri-Chhetri et al. (Reference Khatri-Chhetri, Regmi, Chanana and Aggarwal2020) find that larger household increases the likelihood that household heads adopt new agricultural technologies.
Beside these factors, the occurrence of a climate shock is a determinant of the adoption of climate change adaptation practices (Boansi et al. Reference Boansi, Tambo and Müller2017). The Intergovermental Panel on Climate Change (IPCC 2014) reveals that when the producer has already suffered a temperature-related climate shock, he is more willing to adopt the adaptation practices. Deressa et al. (Reference Deressa, Hassan, Ringler, Alemu and Yesuf2009) find that lower precipitation causes farmers to adopt soil conservation measures. In addition, some research shows that there is a positive relationship between the use of information and communication technologies (ICTs) and farmers’ adaptation measures to climate change. Behrman et al. (Reference Behrman, Bryan and Goh2014) and Diendéré (Reference Diendéré2019) point out that new ICTs allow farmers to receive real-time information without travel costs and thus make appropriate adjustments.
The adoption of coping strategies also depends on farm characteristics. Several studies indicate that the adoption of adaptation strategies is influenced by farm size (Perz Reference Perz2003). Perz (Reference Perz2003) finds that herbicide adopters had a larger area of cleared land than non-adopters. In addition, some authors suggest that economic factors also explain the likelihood of adopting different agricultural practices to cope with climate change. Perz (Reference Perz2003) highlights the cost of technology adoption when adopting water and soil conservation techniques. Other authors show that agricultural financing, including cash transfers received, relaxes this cost constraint and thus promotes the adoption of climate change adaptation practices (Barnes et al. Reference Barnes, Wang, Cinner, Graham, Guerrero, Jasny, Lau, Sutcliffe and Zamborain-Mason2020; Diendéré, Reference Diendéré2019).
Many researches indicate that in addition to economic factors and associated farmer characteristics, institutional factors are likely to play an important role in climate change adaptation. Yegbemey et al. (Reference Yegbemey, Yabi, Aïhounton and Paraïso2014) find that membership in a farmer group facilitates adaptation to climate change. This is because farmer groups are usually channels for the exchange of ideas, experiences, social support, and serve as learning centers. Moreover, Norton and Alwang (Reference Norton and Alwang2020) support that contacts with agricultural extension services increase the likelihood of adopting soil conservation strategies. Agricultural extension services provide producers with information and advice on best agricultural practices.
This synthesis of the literature highlights four categories of factors that are likely to influence the choice of climate change adaptation strategies. These are Farmer Characteristics, Agricultural Characteristics, Economic Factors, and Institutional Factors.
Impact of adaptation strategies
Several studies assess the impact of climate change adaptation strategies on household food security and income. This section presents an overview of the existing literature about the impact of coping strategies. Several studies assess the impact of climate change adaptation strategies on households’ food security and income. These empirical works are of an econometric type and are contrasted. On one hand, some studies show that the adoption of climate change adaptation practices improves food security and increases the income of adopters. On the other hand, literature shows that adaptation practices do not have a positive and significant effect on income and food insecurity.
Some studies show indeed that the adoption of climate change adaptation practices improves food security and increases the income of adopters. Using matching techniques, Zakari et al. (Reference Zakari, Ibro, Moussa and Abdoulaye2022) find that farmers’ adaptation strategies have a positive impact on household income and food security. Specifically, the authors find that farmers who adopt climate change adaptation strategies are more likely to increase their household income by 7,722 F CFA than farmers who did not adopt these strategies. Similarly, authors find that those who apply adaptation strategies are 7% to 9% more likely to be food secure than those who do not apply an adaptation strategy. Ndiaye et al. (Reference Ndiaye, Dieng and Diagne2018) conclude that adopting climate change adaptation strategies increases average annual household income by between 607,000 FCFA and 702,000 FCFA. In addition, authors find that the adoption of adaptation strategies increases the average value of household food consumption by 8–37 points. Amare and Simane (Reference Amare and Simane2018) find that farmers who adopt adaptation options have higher caloric food intake. Berhe et al. (Reference Berhe, Hoag, Tesfay, Tadesse, Oniki, Kagatsume and Keske2017) conclude that the adoption of coping strategies such as diversification of income sources, migration, and land management practices are the most important determinants affecting rural household income in Ethiopia. In general, research indicates a positive impact of the adoption of agricultural practices on yield, well-being, household income, and food security (Savadogo et al. Reference Savadogo, Somda, Seynou, Zabré and Nianogo2011; Oxfam 2018; Combary and Traore Reference Combary and Traoré2021).
On the other hand, literature shows that adaptation practices do not have a positive and significant effect on income and food insecurity. In the Minembwe highlands of South Kivu, Mulumeoderhwa et al. (Reference Mulumeoderhwa, Mugisho, Rushigira, Biganiro, Vwima and Mushagalusa2020) show that adaptation strategies implemented by rural producers are not likely to ensure household food security in the long term. Similarly, Pailler et al. (Reference Pailler, Naidoo, Burgess, Freeman and Fisher2015) report that community-based natural resource management practices have no impact on household income in Tanzania. Berhanu and Beyene (Reference Berhanu and Beyene2015) also find that the prevailing strategy of fencing land for cereal cultivation does not contribute significantly to household food security in southern Ethiopia.
In the next section, the study area and data for this research are presented.
Presentation of the study area and the data collection
After locating the research area, this section presents the approach used to collect the data and describes the sample characteristics.
The study area
The study area is the Center-North region (Figure 1). This region is subdivided into three provinces, namely Bam, Namentenga, and Sanmatenga. It has 25 rural communes and is located between the Sahel zone and the Sudano-Sahelian zone of Burkina Faso. Overall, it has a Sahelo-Sudanese type of climate characterized by two distinct seasons: a long dry season from November to May and a short rainy season from June to October (Kaboré et al. Reference Kaboré, Ouedraogo, Sanon, Yaka and Somé2017). This region, representative of regions with a Sahelo-Sudanese type climate, remains vulnerable to land degradation due to soil and climate conditions and demographic pressures. Similar to other regions of Burkina Faso, the economy of the Center-Nord region is essentially based on rain-fed agriculture. The vast majority (more than 80%) of the population depend on this activity, which is the main source of household income. The Center-North region is characterized by a rather uneven terrain. Hills with ferruginous tanks can be seen in the region (Kaboré et al. Reference Kaboré, Ouedraogo, Sanon, Yaka and Somé2017). Soils are poor, overused, and subject to erosion. Two types of bare soils are observed on agricultural lands: leached tropical ferruginous soils and eutrophic brown soils. This results in an expansion of parched soils and a degradation of natural vegetation. Sorghum, millet, rice, and cowpea are the main staple crops. Cowpea, vegetables, millet, and voandzou are the most profitable crops. Rice is grown very sporadically near dams and in the lowlands. Corn is widely consumed and sold in some parts of the area. Vegetable production is quite developed in the Bam Province, where green beans are grown extensively around Lake Bam for export to Europe. In the face of land degradation and water scarcity, the construction of dams and other water reservoirs and the establishment of irrigation areas are seen as ways to develop new and much more productive agricultural activities. Water management for irrigation purposes is an option for adaptation to climate change.
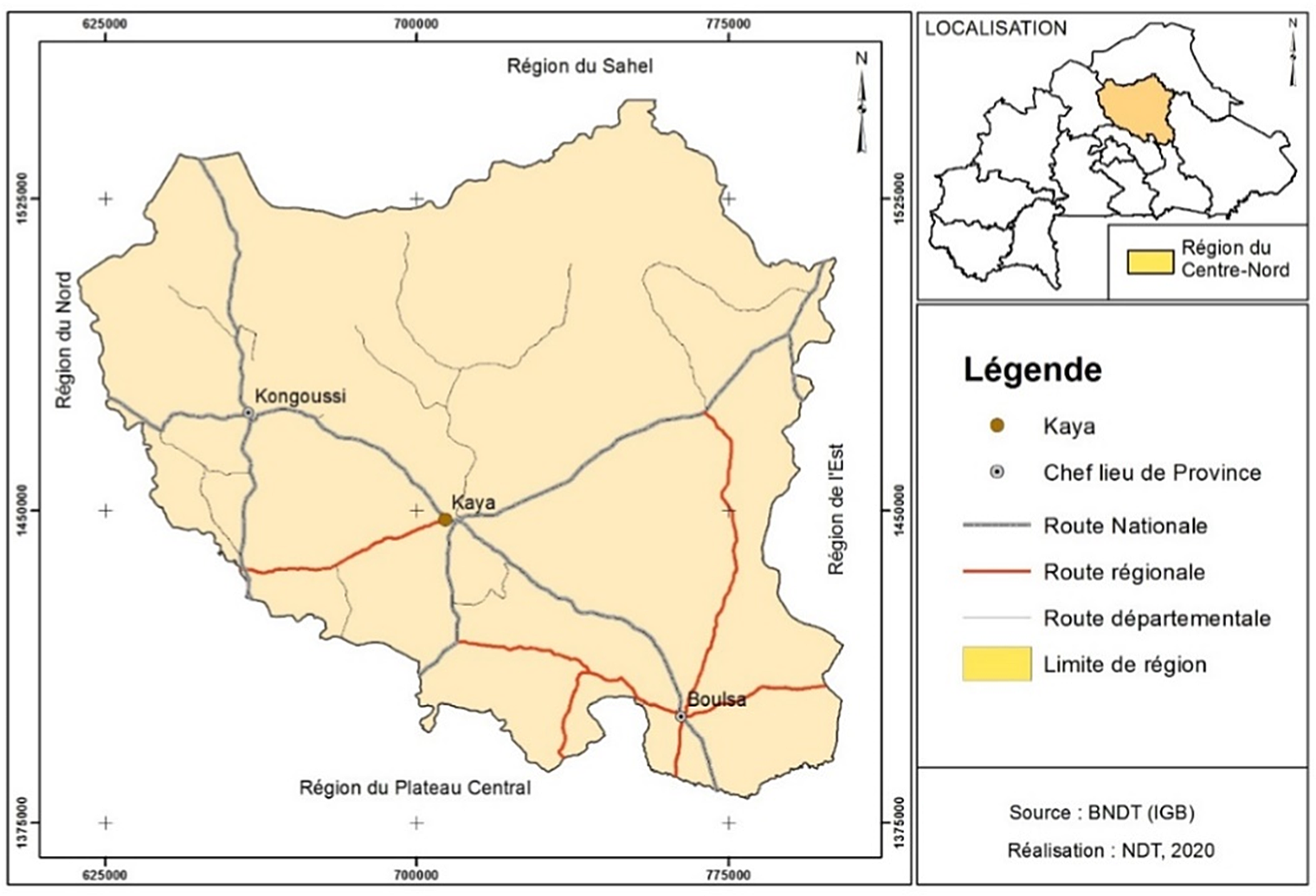
Figure 1. Study area location.
The population of the region is estimated to 1,738,831 inhabitants in 2019, with an average annual growth rate of 3% between 2009 and 2014 (Institut National de la Satistique et de la Demographique [INSD] 2021). In addition to household domestic tasks, women are involved in both production and processing of agricultural products. According to the general report of the National Institute of Statistics and Demography on household living conditions (Institut National de la Satistique et de la Demographique [INSD] 2018), the average annual income of an individual in the Center-North region is estimated to 115,135 FCFA, compared to 447,000 FCFA for the national average.
Data Collection
A survey covering the period from April to July 2021 has been carried out to collect the information. This survey was organized in three phases: (1) the choice of villages and households, (2) the exploratory survey, and (3) the large-scale survey.
Regarding the first phase, nine villages from the three provinces of the Center-North region were selected, three villages per province. The selection of the villages took into account their geographical location in the region and the possibility of access to them due to the insecurity that has prevailed in the region in recent years. The villages studied are representative of all villages in the region. The active women farmers in each village were randomly selected with the help of the representatives of each village based on the list proposed by them, taking care not to select two women from the same household. Based on these criteria, 30% of the active women farmers in each of the nine villages were interviewed, for a total of 426 women farmers. Informed consent was obtained from the participants before the survey began.
The second phase concerns the exploratory survey conducted in March 2021. This exploratory survey consisted of two steps: The first was conducted using a questionnaire and the second using a semi-structured interview. The questions were asked by trained interviewers who spoke the local dialect. The exploratory survey brought to light some important unanswered questions. It enabled the formulation of lines of inquiry. Some of the questions proved to be unclear or in need of clarification. They were revised to ensure better understanding. Some questions, particularly those related to the evaluation of a parameter by the respondents, turned out to be not very explicit or needed to be clarified. They have been reissued to ensure better understanding. Specifically, the assessment of domestic family responsibilities and climate backgrounds required providing the respondents with a diverse range of domestic works and climate backgrounds. One of the major challenges of the exploratory questionnaire is to design it in such a way that it targets the experiences of the interviewees and that the data collected are accurate and usable. Sufficient familiarity between interviewer and respondent is a prerequisite before proceeding to answer the questions.
The third phase is the administration of the questionnaire to the sampled women farmers. The low level of education of the sampled population made it difficult to understand the questions asked which required a lot of patience from the interviewers. The data collected were related to the socio-demographic characteristics of the women farmers, the characteristics of farms, the climatic disturbances observed, the coping strategies used, and the type of food consumed by the households. See Table 1 for a description of the analysis variables.
Table 1. Summary of the variables
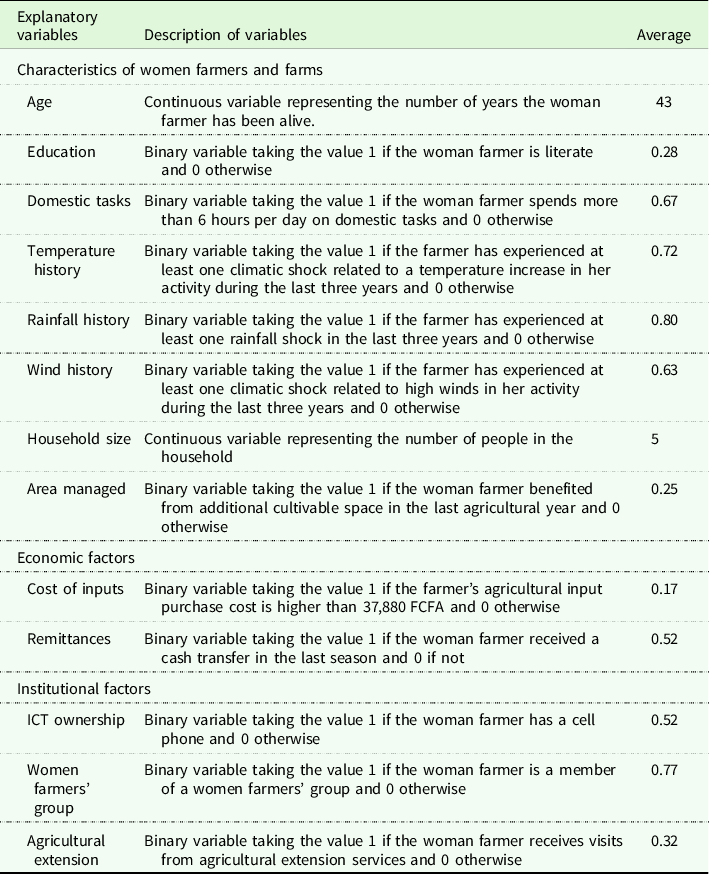
Source: Authors, based on survey data.
Sample description
The statistical analysis shows that the age of women farmers in the sample ranges from 18 to 65 years, with an average of 43 years. The educational level of all the women farmers surveyed remains low. Only 28% of these rural women had attended school, compared to 72% who had not. The majority of rural women farmers (67%) spend more than 6 hours per day on household chores. The minimum household size is five people, while the maximum size is 17, with an average of nine people per household. The data collected show that the annual cost of purchasing agricultural inputs ranges from 17,800 FCFA to 65,300 FCFA, with an average purchase cost of 37,880 FCFA (100.00 FCFA = 0.164 USD). Statistics show that the cost of purchasing agricultural inputs is higher than the average (37,880 FCFA) for 17% of women farmers. Statistical analysis also shows that 80% of the women farmers surveyed reported having experienced at least one rainfall shock in the last three years; 72% had experienced at least one temperature shock, particularly droughts; and 63% had experienced at least one shock related to high winds. Moreover, 77% of women farmers are members of a women’s group, 25% report having benefited from additional acreage in the last agricultural season, and 32% receive visits from agricultural extension services. In addition to agricultural extension services, radio, and cell phones are the sources of information for 52% of women farmers. It also shows that 59% of women farmers have an average annual income of 21,254 FCFA. The data collected show that 47% of the women farmers have an above-average income and 52% report that their income comes from remittances from other family members who have migrated. The analysis of the data collected also shows that 47% of the women are food insecure (consumption levels below 21 Kcal).
The sample reflects the overall characteristics of the population in the Center-North region. Any estimate derived from a sample is subject to sampling error, as only a portion of the population was observed. The main risk is sampling bias, which we have worked to minimize. This research indeed appears interesting insofar as the results obtained can be generalized to other areas of the country whose population has similar characteristics.
After a descriptive statistical analysis to identify the adaptation strategies of rural women farmers to climate change, an econometric model examines the various factors underlying the adoption of these adaptation strategies. An evaluation of the impacts of these strategies on household income and food security is also conducted. The next section is devoted to the methods used to analyze the data collected.
Data analysis models: the multinomial logit model and the propensity score matching (PSM) model
The specifications of the multinomial logit model and the PSM model are the focus of this section.
Specifications of the multinomial logit model
The determinants of the adoption of adaptation strategies were examined through a multinomial logit model. The advantage of this model is that it allows farmers to choose one or more strategies from a set of possible strategies. Following utility maximization theory, farmer i attempts to compare the different levels of utility associated with the various choices and then chooses the one that maximizes her utility
${U_{ij}}$
among j choices. Let us denote by i the farmer; by X = 1, 2, …, k the explanatory variables and by j = 0, 1, 2…, n the different adaptation strategies. For rural farmer i, the utility of the choice of strategy j is written as follow:

where
${\beta _j}{X_{ij}}$
represents the deterministic part of function
${U_{ij}}$
(with
${X_{ij}}$
being a vector of farmer-specific characteristics; β
j
the parameter vector to be estimated; and ϵ
ij
the random error term). If farmer i chooses strategy j,
${U_{ij}}\;$
denotes the maximum utility among the j utilities considered by this farmer. Therefore, the probability that j choice for farmer i will occur is:

To make this model operational, we assume that the error terms
${\varepsilon _{ij}}$
are statistically independently and identically distributed according to a Weibull or Gumbel distribution (McFadden 1975). The distribution function is then equal to:

Let Y ij be a variable that takes a value 1 if the rural farmer chooses strategy j and 0 otherwise. This probability of choice is thus translated into the multinomial logit model by:

By normalizing
${\beta _0}$
= 0, the probability associated with the modality 0 is:

The model’s parameters will be interpreted as deviations from the parameters of the modality 0. Specifically, our empirical model is as follows:

The parameters of the model are
${\alpha _i}$
, with i ranging from 0 to 13. The variable
$Adapt$
is the dependent variable and represents the dominant adaptation strategy chosen by each woman farmer. The explanatory variables included in the model encompass farmer and farm characteristics, economic factors, and institutional factors. For farmer-specific traits, in addition to the variables traditionally included in previous work, this research incorporates the women farmer’s domestic tasks as well as her history of rainfall and wind and temperature shocks. The description of all these explanatory variables is presented in Table 1.
The maximum likelihood method was used to estimate the model’s parameters. The likelihood associated with the m+1 independent multinomial logit model is written in terms of m parameter vectors
${\beta _j}$
, j = 1, …., m due to the normalization
${\beta _0}$
= 0. Thus, the estimation of the multinomial logit model’s parameters is done by maximizing the log-likelihood with respect to the parameter vectors (
${\beta _1}$
,
${\beta _2}$
,……,
$\;{\beta _m}$
). The log-likelihood function is as follows:

Parameter
${\beta _j}$
represents the parameters to be estimated, with j ranging from 0 to k, and related the category of strategy adopted by the women farmers. STATA 14 software was used for the estimations.
Method for assessing the impacts of adaptation strategies on food security and household income
Impact assessment can be conducted using several approaches. This study used the PSM method originally proposed by Rubin (Reference Rubin1977) and Rosenbaum and Rubin (Reference Rosenbaum and Rubin1983) for assessing impacts. PSM matches non-adapting farmers with observable characteristics comparable to those of adapting farmers on the basis of a propensity score (PS). It consists of estimating for each farmer (treated and untreated) the probability of being treated according to her initial observable characteristics X: the PS (Imbens and Angrist Reference Imbens and Angrist1994; Zakari et al. Reference Zakari, Ibro, Moussa and Abdoulaye2022). Specifically, it assesses the probability for each farmer (adapters and non-adapters alike) to have adopted a certain strategy given her observable characteristics. In our research, the method was used to estimate the average treatment effect (ATE) of adopting adaptation strategies on household income and food security. Here, it involves matching treatment farmers (farmers who have adopted adaptation strategies) with control farmers (those who have not adopted any strategy). More specifically, the technique entails matching each farmer i in the adapter subpopulation with each farmer j in the non-adapter subpopulation based on the same characteristics as farmer i. This implies that adapting farmer i, having the same characteristics as non-adapting farmer j, would have gotten the same score if she had not used an adaptation strategy.
In this way, the average difference in the outcome variable (food security and income generated) between adapters and non-adapters was measured. The advantage of this approach is that it is possible to carry out if the treatment is binary in the presence of sufficient data. Further, the evaluation can be carried out ex post, even in the absence of baseline data.
In this research, the PS is estimated as the probability of adopting a climate change adaptation’ strategy using the vector X as a conditioning factor. The PS can be calculated in two ways: logistic regressions and classification and regression trees. Logistic regression, which predicts the probability of occurrence of an event, is the most commonly used technique for estimating PSs. Here, PS estimation is performed using a logit of climate change adaptation’ strategies. The score equation is as follows:

where P(·) refers to the probability; D refers to the participation indicator; X: refers to the conditioning factor;
$1|X\;$
: refers to the farmer’s decision to adopt adaptation strategies given observable characteristics.
The inverse estimator is used to estimate the ATE and the ATE on the treated (ATT). ATE on the whole population measures the effect of the transition of the whole population from “ untreated” to “treated”. ATT “ATE on the treated” measures the effect of the entire population actually treated from “untreated” to “treated.” In this way, the ATE is the ATE given all sampled women farmers. The ATT is the ATE given only the adapting farmers.
The ATE of randomly selected farmer i in the population is defined as follows:

where E(·) is the mathematical expectation;
${Y_{1i}}$
represents the observed outcome for adapting farmer i, and
${Y_{0i}}$
denotes the observed outcome for the non-adapting farmer. The ATT determines an innovation’s average impact on a subpopulation of treated individuals. In the context of our research, it embodies the expected impact on a randomly selected adapter farmer among the farmers who used the adaptation strategies.

(Y
$|T)$
means that outcome Y is conditional on T.
The outcome variables are overall average household income generated, as well as insecurity. The treatment variable is the adoption of adaptation strategies. Overall average income generated is obtained from the list of each woman farmer’s asset categories (sheep, goats, hens, farm yield), and, for each asset, the average income generated is determined. The equation for the average income generated for each farmer is as follows:

where
${Y_i}$
is the overall average income generated by a given woman farmer and
${Y_{i,k}}\;$
is the average income per type of asset.
Food insecurity is measured by the Food Consumption Score (FCS). The FCS is a standardized World Food Program (WFP) composite score calculated to reflect the dietary diversity, frequency of consumption, and relative nutritional intake of the products and food groups consumed by a household. It is obtained by summing the frequency of consumption of each food group (capped at 7 days) multiplied by the food group weighting. According to the WFP’s calculations, a household with a food score below 21 Kcal is severely food insecure. To calculate the household FCS, WFP first grouped the different foods consumed by households into eight food categories. Then, each food category is given a weight based on the nutrient content of that category. Finally, food consumption frequencies are determined by considering the number of days each food group was consumed per household during a one-week period. The following equation is used to generate the FCS:

where (i = 1, …, n); n = total number of food categories;
${a_i}$
= number of days each food category is consumed during the week; and
${X_i}$
= weight of each food category (Table 2).
A summary of the control and treatment variables is outlined in Table 3.
Table 3. Summary of the variables
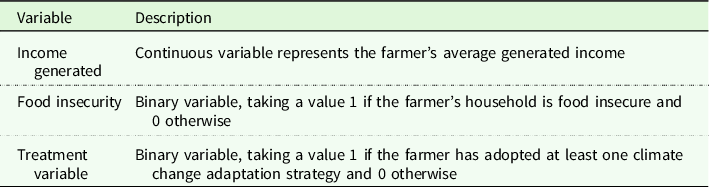
The remainder of the article is devoted to the interpretation and discussion of descriptive statistics and econometric estimations findings.
Results on strategies, determinants, and impacts
In this section, the adaptation strategies of women farmers, the determinants of these choices, and their impacts on income and food insecurity are presented.
Women Farmers’ adaptation strategies
In order to strengthen their resilience to the negative impacts of the observed climate disruptions, 72% of the women farmers surveyed have adopted at least one climate change adaptation’ measure. 28% have not applied any strategy. The most prevalent strategy in this study is the strategy adopted by woman farmer (Figure 2). An analysis of these results based on the stability of strategy adoption (the strategy most frequently adopted by the farmer) and the objective pursued in adopting each adaptation strategy allows us to identify four categories of dominant strategies among the adaptation strategies developed by women farmers. The first category includes water and soil conservation techniques such as essentially Zaï pits, stone barriers, and half-moon. Zaï pits are small holes (20–40 cm in diameter and 10–20 cm deep) filled with manure or compost. Stone barriers or contour walls: Stones wall placed along the contours of the land and where stones or rocks are available slow down water runoff and improve water collection capacity. Half-moon micro-catchments are small semicircular earthen embankments. Half-moon captures water that flows down a slope. Half-moons are especially helpful in restoring degraded areas. Changes in cultivation techniques, such as altered planting dates and crop combinations, make up the second category. The third category relates to diversification of economic activities, particularly petty trading, tontines, and agricultural food processing. Tontines are informal savings and credit practices in which an amount of money collected on a parity basis is redistributed alternately at random (drawing lots), according to need, or according to a compensatory calculation. They fulfilled two functions: With their credit function, they were close to mutual credit; with their provident function, they took the place of mutual aid societies. The diversification of activities toward non-agricultural economic activities offers different earning opportunities than those of agricultural production. It mitigates risks and reduces the negative impact of seasonality on consumption. The use of improved seeds is the subject of the fourth category. Improved seeds make it possible to adapt the seed cycle to the conditions of the ecological zones as well as to provide the producer with a product that retains the genetic qualities of the selected variety. The adoption of improved seeds contributes to increased production and agricultural crop productivity (Carter et al. Reference Carter, Laajaj and Yang2013). Crop change techniques (crop rotation and intercropping) are less expensive farming practices that require little labor and workforce compared to other farming techniques.
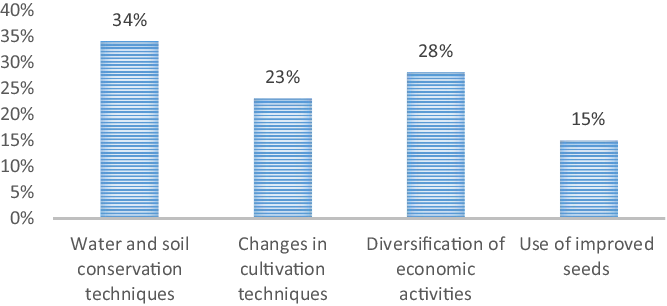
Figure 2. The dominant strategies of women farmers.
Analysis of Figure 2 shows that the highest adoption rates are the adoption of water conservation and soil fertilization techniques (34%), diversification of economic activities (28%), and modification of cultivation techniques (23%). Adoption of improved seeds (15%) is the lowest. The adoption of these categories of strategies by rural women farmers may have different determinants than those of farmers in general. The following section analyzes the determinants of women farmers’ adoption of adaptation strategies.
Determinants of women farmers’ adaptation choices
Before analyzing and discussing the findings, it is important to check the validity of the model. The results of the maximum likelihood estimation are shown in Table 4. The partial correlation test shows that there is a correlation between the dependent variable and the explanatory variables. It also indicates a correlation between the explanatory variables included in the model. Indeed, there is the problem of multicollinearity. As suggested by Deressa et al. (Reference Deressa, Hassan, Ringler, Alemu and Yesuf2009) and Negassa et al. (Reference Negassa, Hellin and Shiferaw2012), the calculation of variance inflation factors (VIF) is recommended to solve this problem. In the case of this study, the VIF values for all the coefficients associated with the explanatory variables range from 1.11 to 2.52 with an average of 1.52. These results show that for each of the coefficients associated with the explanatory variables, the VIF value is less than 10 and the mean value is also less than 2. In this case, the problem of multicollinearity does not arise in the estimation of the model. Therefore, all explanatory variables included in the model can be kept. Apart from the problem of multicollinearity, the basic assumption of the multinomial logit model is that the errors are identically and independently distributed, with the risk of extreme value distribution (McFadden Reference McFadden and Zarembka1973). To this end, Hausman-McFadden tests for Independence from Irrelevant Alternatives (IIA) assumption are performed. In these tests, the estimated coefficients of the full model are compared with those of the restricted model that excludes at least one alternative from the adaptation strategies. Four categorical outcome tests for IIA assumption are performed. In this analysis, the alternative “no adaptation” was used as the baseline category. The chi-square results and probability values (P > chi2 = 0.000) do not reject the null hypothesis of IIA, indicating that the multinomial logit specification is appropriate for this study.
Table 4. Multinomial logit model parameter estimates
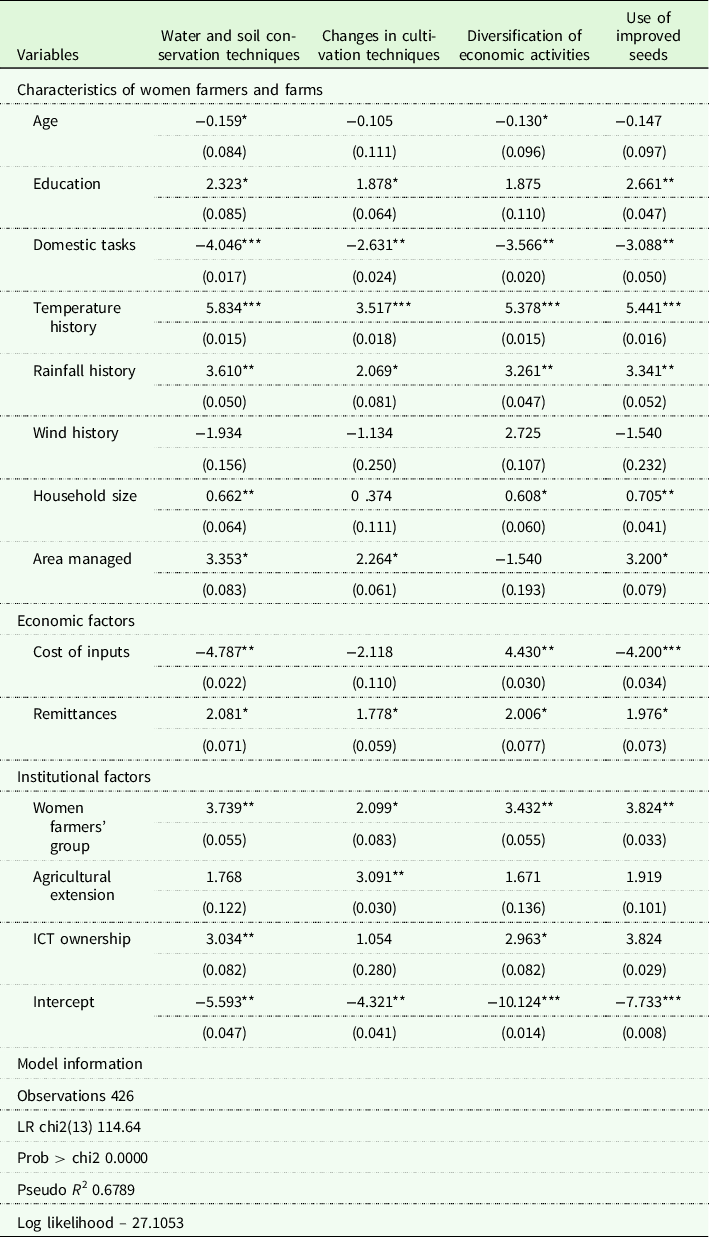
***; **; *respectively denote 1%, 5%, and 10% significance level.
The results of the logistic regression using the maximum likelihood method are reported in Table 4. This table indicates that the R2 is equal to 0.6789. Moreover, the calculated LR is 114.64 at 13 degrees of freedom; the theoretical value read from the chi2 table is 27.68 at the 1% threshold. Since the calculated value is larger than the theoretical value at the 1% threshold, there is at least one non-zero coefficient in the model. These results allow us to say that the estimated model is significant overall.
The adoption of adaptation strategies is a complex process involving several factors. The results in Tables 4 and 5 indicate that the factors determining adaptation to climate change vary according to the category of agricultural strategies adopted by women farmers. These results show that the domestic tasks on the family, temperature, and rainfall shocks experienced by women farmers, the cost of purchasing inputs, remittances, ownership of ICTs, and membership in a women’s group are important determinants. The results are discussed in terms of the explanatory variables of the model.
Table 5. Results of the marginal effects of the multinomial logit model
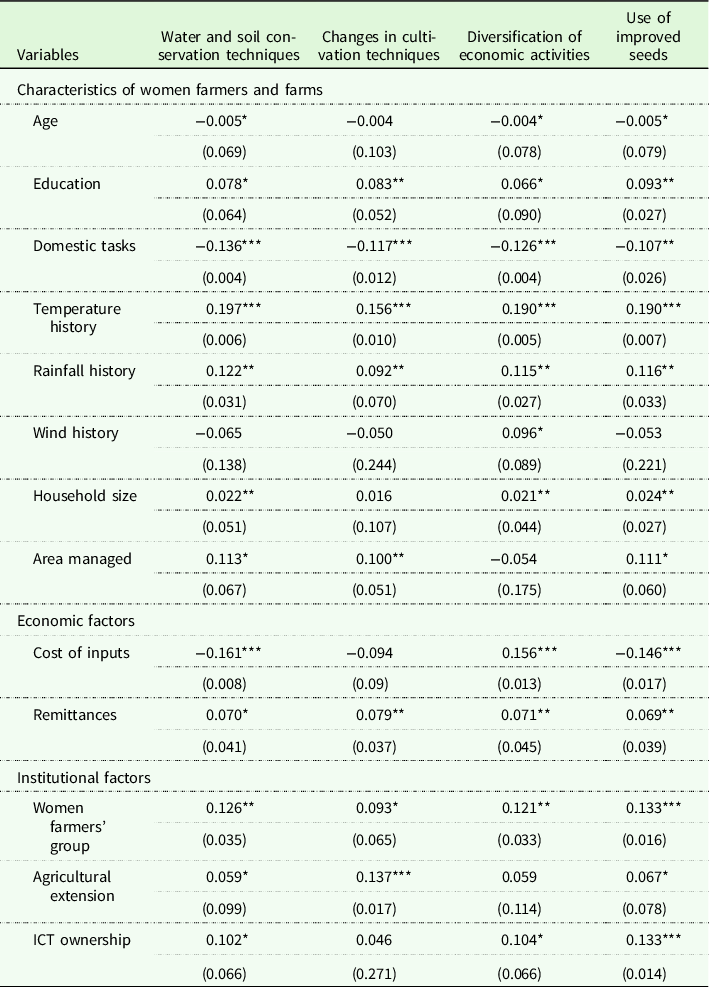
***; **; *respectively denote 1%, 5%, and 10% significance level.
Domestic tasks
The variable “domestic tasks” has a negative and significant impact on the adoption of all four categories of climate change adaptation strategies. More specifically, all other things being equal, high domestic tasks reduce the likelihood that the farmer will adopt water conservation and soil fertilization techniques, changes in cropping techniques, diversification of economic activity, or improved seeds. The results indicate that these probabilities increase by 0.136, 0.117, 0.126, and 0.107 points, respectively. In the rural areas of the Center-North region, as in many other rural areas of Burkina Faso, women farmers assume the triple responsibility of domestic, agricultural, and non-agricultural work. Domestic tasks are varied and include collecting water and firewood, processing and preparing food, traveling, transportation, and caring for sick relatives. These domestic tasks are still done in the traditional way and with all the means at their disposal. This has two consequences. First, it increases the already high poverty rate (52.41%) of women farmers in this region. This poverty limits their ability to purchase improved seeds and certain materials for water conservation and soil fertilization techniques. The tasks also reduce the time available for other economic activities. The women farmers interviewed also point out that increasing domestic workload prevents them from actively participating in community life or serving on decision-making bodies in their communities. This reinforces and perpetuates social inequalities. Domestic stresses are a major barrier to women farmers’ ability to adopt climate change adaptation’ strategies.
Membership in a women farmers’ group
This variable significantly affects the adoption of each of the four categories of adaptation strategies. Thus, membership in a women’s farming group is critical to women’s adaptation to climate change. Marginal effects results (Table 5) show that membership in a women’s group increases the likelihood that a woman will use improved seeds or adopt soil and water conservation techniques by 0.133 and 0.126 points, respectively, when all other things being equal. This finding confirms previous work conducted in the Center-North region and in Benin (Diendéré, Reference Diendéré2019), which point to the importance of clusters in adopting adaptation strategies. In our study area, rural women farmers are organized into village groups. These groups form a social network that allows women farmers to share experiences and information on effective agricultural adaptation strategies adapted to local conditions. Women farmers who are members of these groups often receive technical and material support and even agricultural inputs such as improved seeds from the many projects and NGOs in the region. Membership in the groups allows them to benefit from positive externalities through mutual aid.
The cost of purchasing agricultural inputs
The cost of purchasing agricultural inputs has a differential effect on the likelihood of adopting the different categories of adaptation strategies. The high cost of purchasing agricultural inputs, above the average cost (37,880 FCFA), reduces the likelihood that the farmer will adopt water conservation and soil fertilization techniques or choose improved seeds, all other things being equal. Agricultural inputs such as chemical fertilizers and improved seeds are not readily available to the poorest farmers. In the Center-North region, which has a poverty rate of 52.41%, women farmers have limited financial resources that make it difficult for them to access agricultural inputs. As the cost of purchasing inputs increases, women farmers are less able to adopt costly adaptation strategies. However, the high cost of purchasing agricultural inputs increases the likelihood that women farmers will pursue other non-farm economic activities. Indeed, given the high cost of purchasing farm inputs, women farmers are forced to diversify their activities into other non-farm economic activities so that this non-farm income can be used to purchase farm inputs.
Temperature antecedents
The climate shocks experienced by women farmers in relation to temperature rise have a positive and significant influence on the use of the four categories of coping strategies. The results in Table 5 show that the occurrence of a climate shock related to temperature rise increases the likelihood of women farmers adopting water conservation and soil fertilization techniques by 0.197 points, improved seeds by 0.190 points, diversification into other non-agricultural economic activities by 0.190 points, or changes in cropping techniques by 0.156 points. This result is consistent with that of Deressa et al. (Reference Deressa, Hassan, Ringler, Alemu and Yesuf2009). Indeed, the increase in temperature leads to a significant decrease in the water content of cultivated land due to evapotranspiration. In most cases, this decrease in the moisture content of the cultivated land is accompanied by a decrease in the productivity of the cultivated land, which leads to a significant decrease in the agricultural yields of women farmers. Faced with this situation, women farmers apply water conservation and soil fertilization techniques to maintain the water content of the soil, compensate for the loss of water due to the increase in temperature, and increase the fertility of the cultivated land. In addition, women farmers in the Center-North region practice crop combinations to take advantage of limited fertile soils. They also use improved seeds to avoid growing seasons that coincide with high temperatures and delays in the season. The decline in agricultural yields associated with rising temperatures is also causing women farmers to engage in other non-farm economic activities to compensate for the decline in agricultural yields.
Rainfall antecedents
Like temperature shocks, precipitation shocks have a positive and significant impact on the use of coping strategies. The results of the econometric estimates show that, all else being equal, the occurrence of a precipitation shock, such as a decrease in rainfall, increases the likelihood of adopting water and soil conservation techniques (0.122 points) or resorting to other non-agricultural economic activities (0.115 points) or making changes in cropping techniques (0.092 points) or using improved seeds. These results are consistent with those of Deressa et al. (Reference Deressa, Hassan, Ringler, Alemu and Yesuf2009) and Diendéré (Reference Diendéré2019). The decrease in rainfall and the increase in temperature lead to the deterioration of cultivated land and the destruction of crops, which reduces family production (decrease in agricultural yields). This leads some women farmers to take up other non-agricultural economic activities to meet their family needs. In addition, women farmers apply water conservation and soil fertilization techniques to maintain and increase the moisture and fertility of cultivated land.
Remittances
The results in Table 5 show that remittances are a critical factor in the adoption of adaptation strategies. Indeed, farmers’ access to finance increases the likelihood that they expand their activities to other non-agricultural economic activities (by 0.071 points), adopt water and soil conservation techniques (by 0.070 points), change their farming techniques (by 0.079 points), or use improved seeds (by 0.069 points). Perz (Reference Perz2003) found similar results. Remittances are a form of financing that increases the ability of women farmers to bear the transaction costs of adopting climate change adaptation’ strategies. Remittances help offset economic pressures and enable women farmers to purchase improved seeds and equipment such as hoes and shovels for water conservation and soil fertilization techniques. In addition, remittances enable women farmers to diversify into other economic activities outside of agriculture. Thus, profits from these activities are used to increase investment in inputs such as chemical fertilizers and improved seeds.
The level of education
The educational level of women farmers is a key determinant of the adoption of each category of climate change adaptation’ strategies. The calculated marginal effect shows that educated women farmers are more likely to adopt improved seeds (by 0.093 points), water conservation and soil fertilization techniques (by 0.078 points), changes in cropping techniques (by 0.083 points), or diversification into other non-agricultural economic activities (by 0.066 points) compared to uneducated women farmers. This result is consistent with the results of Miheretu and Yimer (Reference Miheretu and Yimer2017) and Huffman (Reference Huffman2020). This result could be explained by the fact that in the Center-North region, with the development in extension (training, awareness, extension) education favors communication between women farmers and agricultural extension services. It improves the capacity of women farmers to learn and acquire information and knowledge about climate change and adaptation technologies.
Ownership of ICTs
Ownership of ICTs has a positive effect on adoption of water conservation and soil fertilization techniques, diversification into other non-agricultural economic activities, or improved seeds. The results in Table 5 show that owning a cell phone increases the probability of the farmer adopting water conservation and soil fertilization techniques and improved seeds by 0.102 points and 0.133 points, respectively, or diversifying into other non-agricultural economic activities by 0.104 points. These results are consistent with those of Behrman et al. (Reference Behrman, Bryan and Goh2014). Such a result could be understood by the fact that in the rural areas of the Center-North region, seeking information on agricultural technologies is a problem for women farmers, not only because they do not have adequate means of travel but also because of the uncertainty, that limits the movement of agricultural extension agents. In this case, having cell phones allows women farmers to communicate in real time with agricultural extension agents at a lower cost and receives immediate information, such as water conservation, and soil fertilization techniques, which facilitates their implementation. In addition, cell phones facilitate financial (cash) transfers that enable women farmers to purchase equipment and improved seeds. In addition, cell phone ownership can facilitate collaboration among women farmers, allowing them to engage in other non-farm economic activities.
Area managed
The variable “Area managed” is positively and significantly correlated with the adoption of water conservation and soil fertilization techniques, improved seeds, and changes in cultivation techniques. The results of the econometric estimations show that an increase in the area farmed by the farmer increases the probability of adopting water conservation and soil fertilization techniques, improved seeds, or changes in cultivation techniques. The result is understandable considering that access to cultivated land is still a major problem for women farmers in the Center-North region in most cases. They state that the land available to them is insufficient and of poor quality to increase agricultural production not only for subsistence but also for sale. Therefore, they are usually forced to acquire new and additional cultivated land from their husbands or other household heads in the village to compensate for the inadequacy and poor quality of their cultivated land. The acquisition of additional cultivated land leads women farmers in the Center-North region to practice crop combinations in order to benefit from the fertility of this new land. In addition, the newly acquired land is often of poorer quality, prompting farmers to use water conservation and soil fertilization techniques or improved seeds to increase the productivity of the new land.
Contacts with agricultural extension services
The results of the econometric estimates show that rural women farmers in contact with the public extension system are more likely to adopt water conservation and soil fertilization techniques (0.059 points) or improved seeds (0.069 points) or to make changes in cultivation techniques (0.137 points). This result confirms the one found by Diendéré (Reference Diendéré2019). It could be justified by the fact that in rural areas, the agricultural extension system, based on public extension services, is the main source of information, often technical, provided free of charge to all women farmers. This enables women farmers to be equipped with the technical expertise they need to apply techniques for the conservation and management of agricultural land. The techniques for making Zaï and stone barriers, the choice of crops to combine, the time needed for crop rotation, the types of crops that can be combined, and the types of fertilizers and seeds to use are topics that are often discussed with extension agents.
After identifying the determinants of the different strategies, the following section assesses the impacts of these strategies on household income and food insecurity.
Estimating the impacts of adaptation strategies on income and food insecurity
The PSM method is used to estimate these effects. Figure 3 shows the distribution of PSs for adapters and non-adapters. It shows that the distributions of PSs overlap and the common support is very large, so that each of the adapting farmers finds at least one non-adapting farmer with a similar PS.
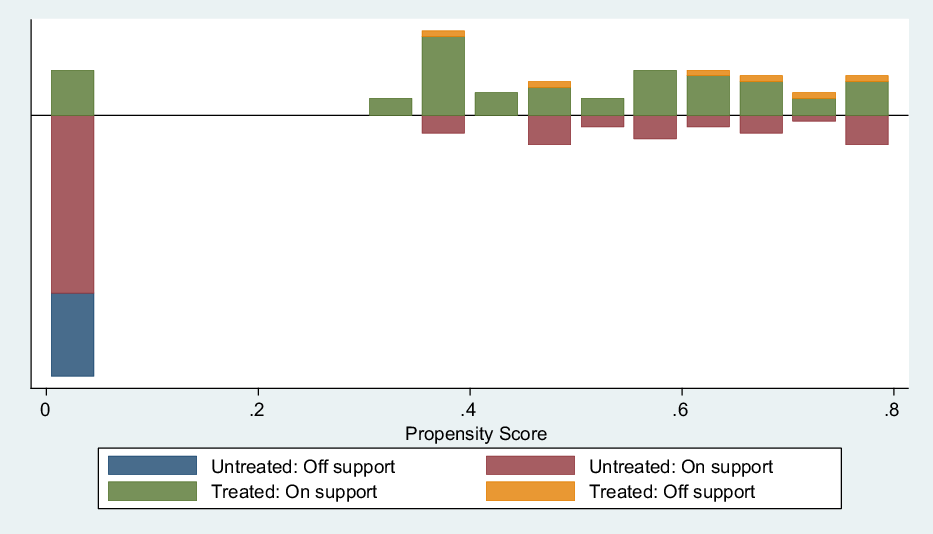
Figure 3. Propensity score distribution.
The impacts of climate change adaptation strategies on income and food security are summarized in Table 6.
Table 6. Impacts of adaptation strategies on farm income and food security
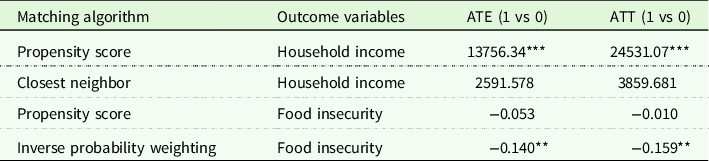
***; **; *respectively denote 1%, 5%, and 10% significance level.
The results in Table 6 indicate that the two coefficients of the ATE are all positive and significant for PS and not significant for nearest neighbor match. This result means that when all women farmers in the sample are considered, the adoption of climate change adaptation’ strategies by a woman farmer is likely to increase her household income by FCFA 13,756 compared to a woman farmer who did not adopt adaptation strategies. In addition, the results show that the coefficients of the ATE on the treated (ATT) are positive and significant for the PS and not significant for the nearest neighbor matching. This result shows that when only adapting farmers are considered, women farmers who adopt climate change adaptation’ strategies are likely to increase their household income by 24,531 FCFA above the average income of the sample. These results are consistent with those of Zakari et al. (Reference Zakari, Ibro, Moussa and Abdoulaye2022) for households in Niger.
Furthermore, analysis of the results in Table 6 shows that the use of climate change adaptation’ strategies has a negative impact on household food insecurity. This suggests that women farmers who adopt climate change adaptation’ strategies are likely to reduce food insecurity compared to those who do not adopt adaptation strategies. The results suggest that they are more likely to reduce their food insecurity than the sample average.
These results indicate that climate change adaptation’ strategies improve women farmers’ income and reduce their food insecurity.
Conclusion
The main objective of this research is to analyze the determinants of women farmers’ adaptation strategies to climate change as well as the impacts of these strategies on food security and household income. While previous work has focused on more general analyses by considering women and men as a uniform group, this research focuses specifically on rural women farmers. Statistical analysis identifies four dominant coping strategies developed by rural women farmers: water conservation and soil fertilization techniques, changes in cropping techniques, diversification into other non-agricultural economic activities, and use of improved seeds. Multinomial logit model estimates reveal that family household expenses, climatic shocks experienced by rural women farmers, the cost of purchasing agricultural inputs, and membership in a women farming group are the main determinants in the adoption of each of the four categories of adaptation strategies. The impacts of these strategies are assessed using a matching model based on PSs. The results show that climate change adaptation’ strategies improve women farmers’ income and reduce their food insecurity. The promotion of climate change adaptation’ strategies has the potential to meet SDGs. These results are generalizable to other regions of Burkina Faso and the Sahel given the agro-pedoclimatic and socioeconomic characteristics of the sampled areas.
A major finding is that domestic tasks are a determinant of each strategy adopted by the farmer. Time- and effort-saving practices and technologies in domestic activities could promote better adaptation to climate change by reducing the domestic workload and freeing up time for productive activities. In order to have a greater impact on income and food security, adaptation strategies should be promoted to mitigate the exclusion of women farmers with large domestic tasks, of which there are many. Women farmers’ domestic tasks are a serious impediment to achieving the SDGs. This is in line with target four of the fifth SDG, which calls on governments and development actors to ensure that unpaid care and domestic work is included and valued, through the provision of public services, infrastructure, and the promotion of shared responsibility in the household and family.
The educational level of the rural woman farmer increases the likelihood of adoption of certain categories of climate change adaptation’ strategies. Therefore, continued investment in the education system is essential to promote the adoption of certain adaptation strategies. Education could be formal or informal and could include all age groups. Many of the women interviewed of all ages deny climate change for a variety of reasons, including lack of education. In addition, institutional factors such as access to information through cell phones, membership in a women’s farming group, and public extension services improve the adaptive capacity of women farmers. Therefore, providing more extension services to women farmers should be supported and encouraged. Formal and informal training programs should be organized more frequently. Similarly, the market information system using the cell phone channel could be improved by public and private agencies. In the same vein, the development of formal and informal social networks can foster group discussions, increase capacity-building opportunities, and improve information flows. It is essential for government agencies to pay particular attention to this. Policies to promote adaptation could emphasize access to finance through affordable and timely credit programs to enable farmers to adapt to climate change.
The results also indicate that the cost of purchasing inputs decreases the likelihood of adopting water conservation and soil fertilization techniques and improved seeds. This cost increases when women farmers have to travel further to input markets. Governments would benefit from ensuring the development of rural road infrastructure to facilitate access to input and output markets. Governments have a responsibility to ensure the availability of appropriate agricultural inputs.
Specifically, it is important to introduce nuances into the development of climate policies. It is useful to ensure that the concessionality of public funding is passed on to women as beneficiaries. There is also a need to focus on access to energy through renewable energies in order to tackle the persistent energy poverty of many women. Approaches to increase local women groups’ access to financial resources, such as small grants approaches as part of enhanced direct access efforts, green credit lines for women entrepreneurs, or by facilitating their collaboration with accredited executing agencies as an implementing partner with local gender expertise for certain project/program components.
Burkina Faso has developed its national development strategy, and several reforms are underway. Improving farmers’ adaptation to climate change should be included in these reforms with a focus on the specificities of women farmers. This research also suggests that rural development institutions and services take into account broader priorities, going beyond agriculture and addressing the concerns of rural women farmers. The creation of a gender-sensitive climate policy could be essential to this. Such a policy could better address the specific aspirations and priorities of rural women farmers.
One of the limits that could constitute a perspective is the limited consideration of adaptation practices to climate change. Taking other practices into account could refine our analyses. Also, like any econometric estimation, the results could be sensitive to the model used. It would be important to use other econometric models in order to test the robustness of the model used.
Data availability statement
The data used in this research were collected through a field survey carried out between April and July 2021. Nine villages from the three provinces of the Center-North region were chosen. The choice of villages took into account the geographical position in the region and also the possibility of accessing it due to the insecurity that has reigned in the area in recent years. The active women farmers of each village were deliberately selected with the help of the representatives of each village and randomly according to the list proposed by the latter and so as not to choose 2 women in the same household. Based on these criteria, 20% of active women farmers from each of the 9 villages were surveyed, which represented a total of 426 women farmers. Informed consent was obtained from the participants before the interviews began. The questionnaire was tested in an exploratory survey conducted in March 2021, which allowed adjustments to be made and the questionnaire to be finalized. The finalized questionnaire was then sent to the sampled rural women farmers.
We ensure data availability. There are no data restrictions. The data sets used during the current article are available from the author on request.
Acknowledgments
None.
Conflicts of interests
Author A and Author B declare none.
Funding statement
This research received no specific grant from any funding agency, commercial, or not-for-profit sectors.