Introduction
Currently, the most widely used methods for diagnosis of intestinal parasites in both field and laboratory settings are fecal egg count (FEC) techniques, based on the microscopic identification and count of parasitic structures (eggs, larvae, oocysts and cysts) in fecal specimens, e.g. McMaster, Kato-Katz, FLOTAC, FECPAK, Mini-FLOTAC (MAFF, 1986; Cringoli et al., Reference Cringoli, Rinaldi, Maurelli and Utzinger2010, Reference Cringoli, Maurelli, Levecke, Bosco, Vercruysse, Utzinger and Rinaldi2017; Levecke et al., Reference Levecke, Behnke, Ajjampur, Albonico, Ame, Charlier, Geiger, Hoa, Kamwa Ngassam, Kotze, McCarthy, Montresor, Periago, Roy, Tchuem Tchuenté, Thach and Vercruysse2011, Reference Levecke, Dobson, Speybroeck, Vercruysse and Charlier2012; WHO, 2019). Also, the availability of a reliable, low-cost, easy-to-use and quantitative test to perform the fecal egg count reduction test (FECRT) is of pivotal importance to facilitate the monitoring of deworming programmes in endemic countries (Cools et al., Reference Cools, Vlaminck, Albonico, Ame, Ayana, José Antonio, Cringoli, Dana, Keiser, Maurelli, Maya, Matoso, Montresor, Mekonnen, Mirams, Corrêa-Oliveira, Pinto, Rinaldi, Sayasone, Thomas, Verweij, Vercruysse and Levecke2019) and to determine anthelmintic efficacy/resistance in consideration of the growing concern of the emergence of anthelmintic resistance in humans (Vlaminck et al., Reference Vlaminck, Cools, Albonico, Ame, Ayana, Cringoli, Dana, Keiser, Maurelli, Matoso, Montresor, Mekonnen, Mirams, Corrêa-Oliveira, Pinto, Rinaldi, Sayasone, Thomas, Vercruysse, Verweij and Levecke2019), livestock (Vercruysse et al., Reference Vercruysse, Charlier, Van Dijk, Morgan, Geary, von Samson-Himmelstjerna and Claerebout2018; Kaplan, Reference Kaplan2020) and pet animals (Jimenez Castro et al., Reference Jimenez Castro, Howell, Schaefer, Avramenko, Gilleard and Kaplan2019; Kitchen et al., Reference Kitchen, Ratnappan, Han, Leasure, Grill, Iqbal, Granger, O'Halloran and Hawdon2019).
Among the above mentioned techniques, Mini-FLOTAC, in particular, is considered a good candidate for a standardized FEC/FECRT either in lab or in field setting (Nikolay et al., Reference Nikolay, Brooker and Pullan2014; Dias de Castro et al., Reference Dias de Castro, Abrahao, Buzatti, Molento, Bastianetto, Rodrigues, Lopes, Silva, Freitas, Conde and de Almeida Borgese2017; George et al., Reference George, Paras, Howell and Kaplan2017; Paras et al., Reference Paras, George, Vidyashankar and Kaplan2018; Cools et al., Reference Cools, Vlaminck, Albonico, Ame, Ayana, José Antonio, Cringoli, Dana, Keiser, Maurelli, Maya, Matoso, Montresor, Mekonnen, Mirams, Corrêa-Oliveira, Pinto, Rinaldi, Sayasone, Thomas, Verweij, Vercruysse and Levecke2019; Rinaldi et al., Reference Rinaldi, Amadesi, Dufourd, Bosco, Gadanho, Lehebel, Paola Maurelli, Chauvin, Charlier, Cringoli, Ravinet and Chartier2019; Amadesi et al., Reference Amadesi, Bosco, Rinaldi, Cringoli, Claerebout and Maurelli2020). However, the Mini-FLOTAC, as all the other FEC techniques, requires specialized personnel and is time-consuming, especially when a large number of specimens are examined like in case of large epidemiological surveys (Cringoli et al., Reference Cringoli, Maurelli, Levecke, Bosco, Vercruysse, Utzinger and Rinaldi2017; Vercruysse et al., Reference Vercruysse, Charlier, Van Dijk, Morgan, Geary, von Samson-Himmelstjerna and Claerebout2018; Sukas et al., Reference Sukas, Van Dorst, Kryj, Lagatie, De Malsche and Stuyver2019).
Recently, the use of new technologies is beginning to offer potential solutions to overcome gaps and limitations of FEC techniques (i.e. human errors and time for analysis). Recent studies have evaluated different smartphone-based technologies to magnify objects, to capture images or to perform an automated identification of endoparasites (i.e. protozoa and helminths), showing a good potential for wider application. Saeed and Jabbar (Reference Saeed and Jabbar2018) reviewed the applications of various smartphone-based methods and devices developed from 1990 to 2017 for the diagnosis of different parasites of public health relevance (e.g. soil-transmitted helminths, Schistosoma spp., protozoa, etc.). Moreover, several studies, reported in Table 1, focused on the development of semi-automated and automated systems for assessing FEC in the veterinary field. However, these systems have shown difficulties in commercialization, mainly due to their low sensitivity and accuracy, high costs and/or the limited data on validations in the lab and/or in the field. For these reasons, we consider that there is still the need for a reliable automated system that improves the efficiency of parasitological diagnosis in veterinary medicine and public health at an affordable cost.
Table 1. Semi-automated and automated systems for parasite detection in humans and animals (principle, the hosts from which fecal samples were collected, the parasites detected, the advantages, limits and references)

In this paper, we present the characteristics of the Kubic FLOTAC microscope (KFM), a new compact, cubic in shape (20 × 20 × 20 cm), low-cost (~600 euros), versatile and portable digital microscope capable of scanning fecal specimens prepared with Mini-FLOTAC or FLOTAC (Mini-FLOTAC/FLOTAC) (Fig. 1A and B). The KFM can be used also directly in field, because it does not need any additional special requirement (e.g. centrifuge or any electricity source), being provided of a lithium battery with an autonomy up to 20 h. Moreover, we report the findings of the first validation of the KFM to perform FEC of gastrointestinal nematodes (GINs) in cattle using the Mini-FLOTAC technique and compare the results obtained by KFM with those obtained by a traditional optical microscope (OM).

Fig. 1. KFM used with the Mini-FLOTAC (A) and FLOTAC devices (B).
Materials and methods
The Kubic FLOTAC microscope (KFM) description
The KFM is composed of an electromechanical part that allows a three-dimensional (3D) scan of the Mini-FLOTAC/FLOTAC (reading discs) and software that allows remote interactions and digital image processing supported by artificial intelligence (AI) for the recognition of helminth eggs and other parasitic structures. FreeCAD (open source, LGPL license) and Design Spark Mechanical (RS-components, Corby, UK) software were used to perform the 3D mechanical of the KFM (Fig. 2A and B). Once opportunely prepared, you can insert the Mini-FLOTAC or FLOTAC devices into a specific slide-out tray of the KFM (similarly to inserting a DVD into a player). Then, the tray is withdrawn inside and specific 3D landmarks corresponding to the corners of the two flotation chambers of the Mini-FLOTAC/FLOTAC are automatically located. The KFM scanning device is equivalent to a XYZ motorized stage for microscopy.

Fig. 2. (A) FreeCAD and Design Spark Mechanical of the KFM (external view); (B) FreeCAD and Design Spark Mechanical of the KFM (internal view); (C) schematic diagram showing mechanical, electronic and optical systems of the KFM and (D) a particular of the handling motor based on the no-standard Cartesian system.
The 3D positioning system of the motorized stage is based on a simple, non-standard Cartesian motor system solution and is provided with open-loop stepper motors coupled with precision translation stages to achieve accurate 3D motion control (Fig. 2C and D). A standard, low-cost stepper motor driver based on Arduino nano-board 105 (Arduino AG, Ivrea, Italy) and a free Grbl firmware were adopted to remote control of the KFM.
The KFM optical part is mainly composed of an LED light source with a condenser, which provides brightness adjustment, and a digital camera, which permits adjustable magnification at 100×, 200×, 300×, with a maximum resolution of 8 MPixel (3264 × 2448 pixel) and the size of image of 1024 × 768 (0.8 Mp) (Fig. 3). A Raspberry PI (Raspberry Pi Foundation, Caldecote, UK) permits the remote control of the optical part. The motorized stage can automatically move stepwise to entirely scan the two flotation chambers of the Mini-FLOTAC/FLOTAC. For each step, the KFM camera captures a picture (or a Z-stack). Eventually, you can photograph the whole surface of the flotation chambers (each 18 mm by 18 mm wide) and the software stitches the multiple photos to obtain only one picture to perform the visual recognizing and the count of parasitic structures.

Fig. 3. Digital imaging of GIN eggs (green arrow) and air bubbles (red square) using the KFM with a digital zoom 100× (A), 200× (B) and 300× (C).
The KFM system has various options for external connectivity: (i) two USB ports to easily transfer the captured images; (ii) an Ethernet cable connector, a Bluetooth and a Wi-Fi wireless connection, which permit all users to easily transfer, share and backup the captured images to other devices. In addition, you can fully control the KFM using software by an external device, i.e. a smartphone, a tablet or a PC. A web interface permits the remote control of the KFM (Fig. 4); the internal software works on Linux operating system. Such an interface can be activated using any web browser. The remote interface supports Android 4.1, iOS 8.0, Windows 7/8/10 and MacOS X 10.8. We developed a specific app that allows the remote control of the KFM directly from mobile phones.
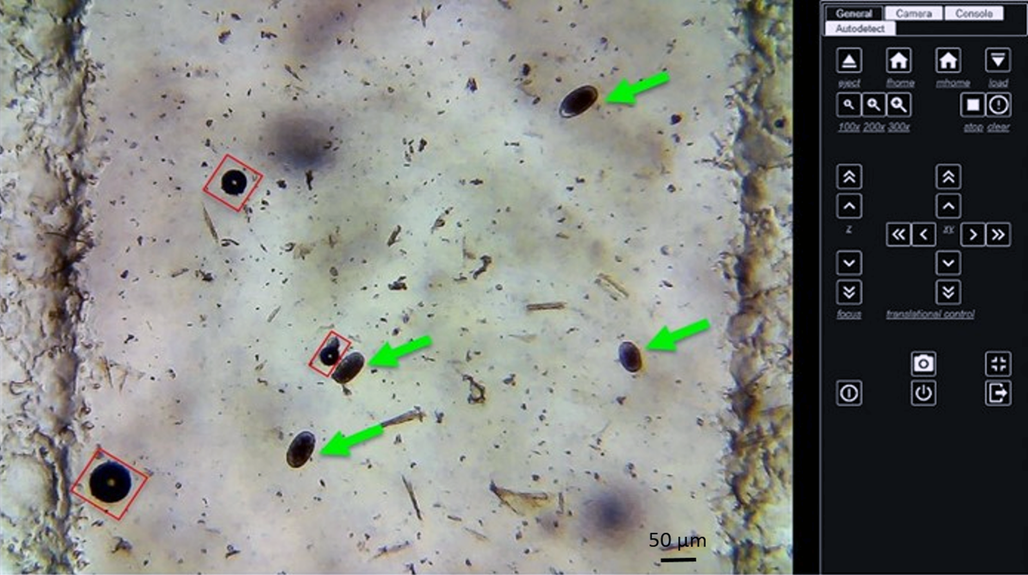
Fig. 4. Image of a part of a Mini-FLOTAC chamber, captured by technological devices (smartphone, tablet or a PC) connected with the microscope that shows GIN eggs (green arrows) and air bubbles (red squares).
The software has two modalities: ‘Administrator’, password-protected, for the management of settings and mechanical movements and ‘Viewer’ to allow remote viewing. The images captured by the KFM can be stored in an Internet cloud and/or transmitted to a diagnostic hub for telediagnosis or parasitological consultation.
At the moment, for these characteristics, you can use the KFM as a digital microscope, instead of an OM, remotely controlled to perform the visual identification and counting of parasitic structures, as validated in this paper. Moreover, further developments are ongoing to update a fully automated version of KFM, as reported in the ‘Discussion’ section.
Study sampling and laboratory activities
From September to December 2019, 30 fecal samples were collected from Belgian Blue and Holstein cattle (6 months old) experimentally infected with 50 000 third stage larvae (L3) of Cooperia oncophora (n = 15 calves) or Ostertagia ostertagi (n = 15 calves) stabled at the experimental farm of the Faculty of Veterinary Medicine, Ghent University (Belgium). Then, we transferred the collected samples to the Department of Virology, Parasitology and Immunology of Ghent University. In the lab, an operator analysed six sub-samples for each fecal sample, filling six Fill-FLOTACs with 2 g of feces and homogenizing them with 38 mL (dilution ratio 1:20) of a saturated sodium chloride flotation solution (specific gravity = 1200). Then, six Mini-FLOTAC devices were filled (one per each sub-sample), following the SOPs described in Cringoli et al. (Reference Cringoli, Maurelli, Levecke, Bosco, Vercruysse, Utzinger and Rinaldi2017). To ensure the quality of parasitological examination, one operator read the Mini-FLOTACs with an OM (Leica Microsystems, Wetzlar, Germany), then a second operator randomized the Mini-FLOTACs, before the reading with the KFM to obtain blinded results. Finally, the total time was evaluated to perform the Mini-FLOTAC technique either with the reading under the KFM or under a traditional OM.
Statistical analysis
A Mann–Whitney test was used to compare the readings by both microscopes. Moreover, we calculated the Lin's concordance correlation coefficient (CCC) and the corresponding 95% confidence interval (CI) to quantify the agreement between the analysis using the OM and the KFM. The agreement was classified as poor, moderate, substantial or almost perfect for CCC values <0.9, 0.90–0.95, 0.95–0.99 or >0.99, respectively (McBride, Reference McBride2005). Finally, the level of agreement was analysed using the Bland–Altman plot. All the statistical analyses were performed using GraphPad Prism v.8 (Graph Pad Software, San Diego, CA, USA) and SPSS Statistics v.23 (IBM, Armonk, NY, USA). All tests were considered statistically significant at P < 0.05.
Results
In total, 180 counts were performed using the OM and 180 counts using the KFM. A range of GIN eggs from 1 to 62 was found for each sample analysed, as depicted in Fig. 5. Based on the counted eggs, each sample was assigned to one of the three egg count levels: low (<10), medium (10–25) and high (>25). Table 2 shows the sum and mean egg counts detected by the OM and the KFM at the different count levels. The correlation and the agreement between counted eggs by both microscopes for each level, and total counts are reported in Figs 6 and 7.

Fig. 5. Comparison of the mean of counted eggs between the readings by the OM and the KFM for each sample.

Fig. 6. Correlation between the number of counted eggs based on the examination of Mini-FLOTAC using the OM and KFM for each level of egg counts and total counts.

Fig. 7. Bland–Altman plot of number of counted GIN eggs based on the examination of Mini-FLOTAC using OM and KFM.
Table 2. Number of counted eggs (sum and mean) for the analysis performed by the Mini-FLOTAC using a traditional OM and the KFM at low (<10), medium (10–25), high (>25) egg count levels and total counts

The results analysed by the Mann–Whitney test showed that there was not a statistically significant difference (P > 0.05) between medians of counted eggs obtained with the two microscopes, for each egg count level. The CCC between the reading with OM and KFM was substantial for low (CCC = 0.984; 95% CI = 0.973–0.990), medium (CCC = 0.981; 95% CI = 0.968–0.988) and high (CCC = 0.998; 95% CI = 0.996–0.999) egg count levels; similar findings were found when considering total egg counts (CCC = 0.999; 95% CI = 0.998–0.999) (Fig. 6). Furthermore, the Bland–Altman plot (Fig. 7) demonstrated an excellent agreement between the two reading approaches (bias = −0.425 ± 7.370, with 95% limits of agreement from −14.872 to 14.021). Finally, the time required to perform the Mini-FLOTAC technique with the KFM and the traditional OM is reported in Table 3.
Table 3. Time of analysis to perform the Mini-FLOTAC technique with the traditional OM and the KFM for each step of the protocol used

Discussion
The KFM presented for the first time in this paper has proven a promising system for an accurate assessment of GIN egg counts in cattle. The results showed that there were not statistically significant differences between medians of counted eggs obtained with OM and KFM. Moreover, a substantial agreement (CCC = 0.999) and a low (−0.425 ± 7.370) discrepancy were found between the two microscopes. These findings were confirmed also by the Bland–Altman plot that was very useful to compare the egg counts obtained with the two microscopes. The Bland–Altman plot showed a very low bias (−0.425) and the absence of a systematic error, in fact points of the graph corresponding to the differences between the readings with the two systems are all around the line zero, showing a dispersion of the points only for the low egg count level (<10 eggs), without outliers.
The time taken to scan and count the GIN eggs in the Mini-FLOTAC chambers under the KFM was ~3–8 min compared to ~1–5 min taken to read the Mini-FLOTAC under the traditional OM by the same skilled operator. However, the KFM system used in this comparison study was only a prototype, but we are developing an App that will allow us to improve the speed of scanning of the Mini-FLOTAC apparatus, thus reducing the time for analysis.
There are many advantages of the KFM compared to the OM, e.g.: (i) it can be used without requiring electricity in both laboratory and field settings; (ii) it is cheap (~€600) and (iii) it is able to transfer via internet the captured pictures to other laboratories or can be used by remote from another country. Therefore, it could be very useful to create a network of laboratories or to support operators directly in the field as expected by Tele-Medicine and Tele-Parasitology (Di Cerbo et al., Reference Di Cerbo, Morales-Medina, Palmieri and Ianniti2015; Scheild et al., Reference Scheild, Lam, Thömmes and Zöller2007; Zaffarano et al., Reference Zaffarano, Morandi, Menegotto, Ostanello and Poglayen2018).
Moreover, this system is very easy to be performed by any operator without a specific training (it will be possible to use the KFM just following the manufacturer's instructions).
One of the future improvements of the KFM, actually under development, is the tuning of a predictive model, for the automated identification and counting of helminth eggs, based on AI (machine learning) using a single deep neural network, named Single Shot Multibox Detector (SSD) (Liu et al., Reference Liu, Anguelov, Erhan, Szegedy, Reed, Fu, Berg, Liebe, Matas, Sebe and Welling2016). For this aim, the use of high-quality images, as well as those captured by the KFM in our study, is fundamental. To date, to perform the first ground-truth phase of the training software a dataset with 11 136 images of GIN eggs (confirmed by experts) from large and small ruminant feces was used. The acquired images are first elaborated to separate the parasitic structures from the background, which can be either impurities or pseudo-parasites, through the use of ImageJ (National Institute of Health) with the main goal of being able to characterize the parasite species and to automatically count eggs (Grishagin, Reference Grishagin2015).
In future, this software will be available also for other parasitic structures of veterinary and public health importance.
Therefore, KFM permits the combination of a sensitive, accurate, precise and standardized FEC techniques, as the Mini-FLOTAC/FLOTAC (Cringoli et al., Reference Cringoli, Rinaldi, Maurelli and Utzinger2010, Reference Cringoli, Maurelli, Levecke, Bosco, Vercruysse, Utzinger and Rinaldi2017), with a reliable system to capture and analyse pictures. Moreover, thanks to the development of AI software for automated identification and counts, this system will allow a reduction in human errors and time of reading, increasing its diagnostic efficiency.
Conclusions
In conclusion, the KFM is a promising system that may, upon successful validation studies performed in different settings and laboratories of medical and veterinary parasitology, allow progression in overcoming the numerous limitations of traditional egg counting techniques.
The development of the AI predictive model will be very useful for an easy-to-use, low cost and precise automated system for identification and counting of parasitic structures allowing a rapid assessment of FEC/FECR to assist a new generation of operators (i.e. technicians, physicians, veterinarians and farmers) in veterinary and human parasitology and diagnostics. In principle, the KFM could also be adapted to read other fecal preparation slides such as the Kato-Katz or McMaster.
Data
All data generated or analysed during this study are included in this published paper. The datasets used and/or analysed during the current study are available from the corresponding author upon reasonable request.
Acknowledgements
The authors acknowledge M.E. Morgoglione, M. Santaniello, P. Pepe, D. Ianniello, S. Pennacchio and V. Musella for their participation in the development of the Kubic FLOTAC microscope. Moreover, they would like to express sincere appreciation to Prof. E. Claerebout, Prof. B. Levecke, N. De Wilde, S. Casaert and all the Department of Virology, Parasitology and Immunology of the Faculty of Veterinary Medicine, Ghent University, Belgium, for their collaboration.
Author contribution
Invented the KFM: GC; all the authors participated in the KFM development. Conceived, designed and coordinated the validation study of the KFM to perform FEC of GINs in cattle: GC, LR and MPM. Performed sampling and laboratory analyses: AA. Performed the first ground-truth phase of training software: GP and AA. All authors contributed to data analysis and interpretation, and preparation of the manuscript. All authors read and approved the final manuscript.
Financial support
This research did not receive any specific grant from funding agencies in the public, commercial or not-for-profit sectors.
Conflict of interest
The Mini-FLOTAC technique was developed and is patented by GC, but the patent has been handed over to the University of Naples Federico II. The fact that GC is the current patent holder of the Mini-FLOTAC and Fill-FLOTAC had no role in the preparation and submission of the protocols reported or the design and implementation of ongoing and future studies. To obtain Mini-FLOTAC or Fill-FLOTAC devices, a contribution is required that is used only to cover costs of production and packaging, and to contribute to the ongoing FLOTAC research. The remaining authors declare that they have no competing interests.