No CrossRef data available.
Article contents
NeXL: A Platform for Innovation in Microanalysis
Published online by Cambridge University Press: 30 July 2021
Abstract
An abstract is not available for this content so a preview has been provided. As you have access to this content, a full PDF is available via the ‘Save PDF’ action button.
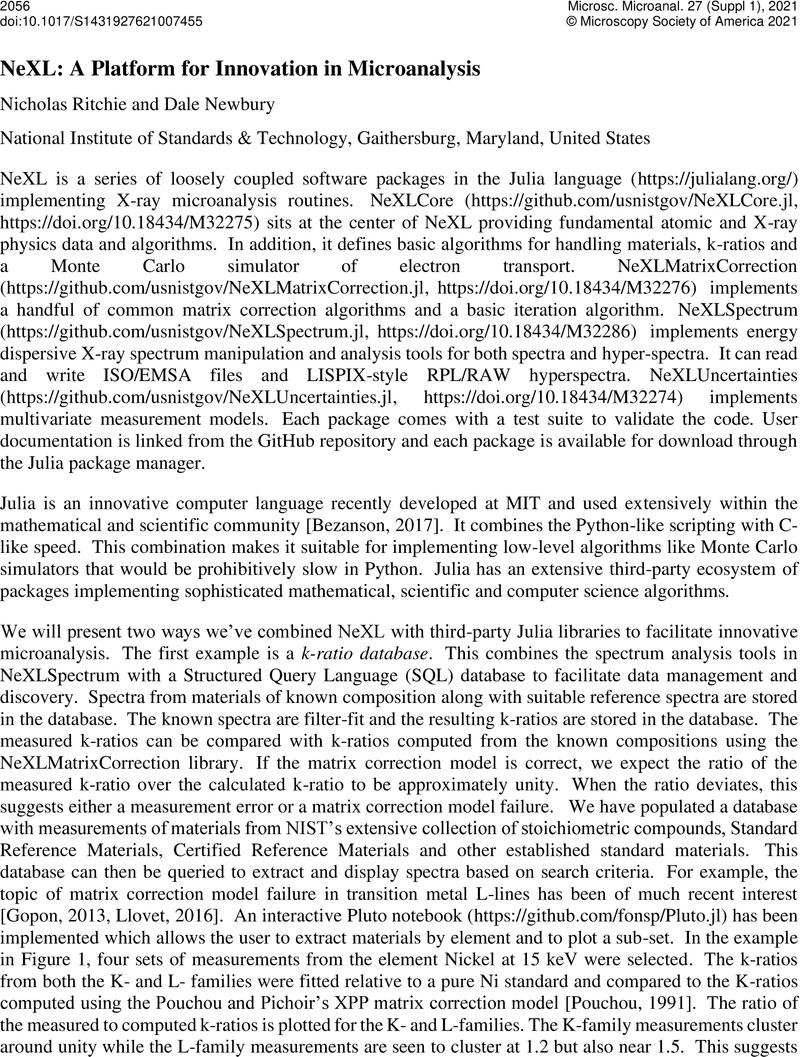
- Type
- Unresolved Challenges in Quantitative X-ray Microanalysis
- Information
- Copyright
- Copyright © The Author(s), 2021. Published by Cambridge University Press on behalf of the Microscopy Society of America
References
Bezanson, J., Edelman, A., Karpinski, S., & Shah, V. B. (2017). Julia: A fresh approach to numerical computing. SIAM review, 59(1), 65-98.Google Scholar
Hornik, Stinchcombe & White, (1989) Multilayer Feedforward Networks are Universal Approximators Neural Networks, Vol. 2, pp. 359-366.Google Scholar
Innes, M. (2018). Flux: Elegant machine learning with Julia. Journal of Open Source Software, 3(25), 602.Google Scholar
Gopon, P., Fournelle, J., Sobol, P. E., & Llovet, X. (2013). Low-voltage electron-probe microanalysis of Fe-Si compounds using soft X-rays. Microscopy and Microanalysis, 19(6), 1698.Google ScholarPubMed
Llovet, X., Pinard, P. T., Heikinheimo, E., Louhenkilpi, S., & Richter, S. (2016). Electron probe microanalysis of Ni silicides using Ni-L X-ray lines. Microscopy and Microanalysis, 22(6), 1233-1243.CrossRefGoogle ScholarPubMed
Pouchou, J. L., & Pichoir, F. (1991). Quantitative analysis of homogeneous or stratified microvolumes applying the model “PAP”. In Electron probe quantitation (pp. 31-75). Springer, Boston, MA.CrossRefGoogle Scholar