1 Introduction
Many engineering researchers consider decision-making a fundamental activity to the engineering design process (Tribus Reference Tribus1969; Mistree et al. Reference Mistree, Smith, Bras, Allen and Muster1990; Hazelrigg Reference Hazelrigg1998; Lewis & Chen Reference Lewis, Chen and Schmidt2006). Designers make a variety of decisions that impact, directly or indirectly, the final design definition. Decisions directly impacting the design definition include, among other things, selecting a system architecture, selecting materials, and determining physical dimensions of parts. Decisions indirectly impacting the final design include, among other things, selecting metrics for design evaluation, selecting from among various design process alternatives (e.g., whether to commit to a solution or gather more information), and choosing an overall product or system development strategy (e.g., whether to use a product platform to develop a product family, whether to outsource portions of a systems design problem).
One can categorize decision-making research according to three main types (Bell et al. Reference Bell, Raiffa and Tversky1988):
-
(i) Normative research: how ideal, rational agents should make decisions that are consistent with some set of predetermined rules for rationality, e.g., the von Neumann–Morgenstern axioms of rationality,
-
(ii) Descriptive or behavioral research: how real people actually make decisions,
-
(iii) Prescriptive research: how real people can make decisions.
Individual studies may involve more than one of these categories. For example, investigators might arrive at a prescription for decision-making based on conclusions drawn from normative theory or observations of decision makers. Examples of all three types of research exist in the context of engineering and systems design, but not in equal proportions. Although behavioral studies are common in design creativity and idea generation research (Paulus & Yang Reference Paulus and Yang2000; Cross Reference Cross, Eastman, Newstatter and McCracken2001; Gero Reference Gero2010; Gero & Maher Reference Gero and Maher2013; Sauder & Jin Reference Sauder and Jin2016), relatively little behavioral research exists on individual decision-making in engineering and systems design. The field of psychology has made many advances in understanding human judgement and decision-making (Angie et al. Reference Angie, Connelly, Waples and Kligyte2011; Gigerenzer & Gaissmaier Reference Gigerenzer and Gaissmaier2011; Strough et al. Reference Strough, Karns and Schlosnagle2011; Newell & Shanks Reference Newell and Shanks2014). However, these results often times pertain to a certain context, e.g., consumer decisions or health-related decisions (Teare et al. Reference Teare, Mazanec, Crawford-Welch and Calver1994; Shiv & Fedorikhin Reference Shiv and Fedorikhin1999; Riva et al. Reference Riva, Antonietti, Iannello and Pravettoni2015; Kraus & Marco Reference Kraus and Marco2016), and it is not immediately clear how well these results translate to engineering contexts. For example, one study found that engineers react differently to gain–loss framing effects in an engineering context than was observed in general populations in other studies (Vermillion et al. Reference Vermillion, Malak, Smallman and Linsey2015). A deeper understanding of how individuals make decisions in an engineering and systems design context would support prescriptive decision-making research by yielding greater insight into undesirable biases and the prescriptions that would promote rational decision-making.
When conducting descriptive and behavioral decision-making research, single decision-making scenarios can be embodied in a number of ways, just as we can embody a conceptual design in a number of ways. Written narratives and surveys constitute one general way to embody decision-making scenarios where decision makers are given a written summary of the scenario with explicit decision alternatives. However, the use of written narratives and surveys intuitively has its limitations when studying more complex scenarios. In this paper, we investigate a game-based approach for embodying decision-making scenarios in engineering contexts. Games used for purposes beyond entertainment are called serious games, and began primarily as educational and training tools (Abt Reference Abt1987; Zyda Reference Zyda2005). Games offer an alternative approach that can be more open-ended in the sense that the decision maker experiences the scenario, as opposed to reading about it, and can discover decision-related information through game play.
The aims of this paper are twofold: (1) to demonstrate the use of serious gaming for decision-making research; and (2) to demonstrate how game design can impact results when compared to a written narrative embodiment of the same decision-making scenario. In two separate experiments (one with a Mars mission narrative and one with an oil drilling narrative), we compare behavior observed in a game-based context with that of a standard written narrative design. The primary hypothesis is that appropriately designed games will yield the same results as a standard written narrative design. An appropriately designed game is one that does not inadvertently introduce confounding factors that distort the standard written narrative. In the next section, we provide background on the written narrative approach, serious gaming and discuss the use of serious gaming in human subjects research. Subsequent sections are devoted to the experiments used to demonstrate and evaluate the proposed game-based framework.
2 Background
2.1 Written narratives
The written narrative approach for communicating decision-making scenarios is a widely used and accepted technique for decision-making research. Typically, this approach involves providing a participant with a written description of a situation or decision-making scenario, after which they make scenario-related decisions. Studies examining decision-making biases such as hindsight bias (Christensen-Szalanski & Willham Reference Christensen-Szalanski and Willham1991), counterfactual thinking (Sanna & Turley Reference Sanna and Turley1996), and sunk cost effect (Arkes & Blumer Reference Arkes and Blumer1985) have traditionally relied on this approach. Psychologists often use this approach because it allows for more experimental control than some other methods would provide. This allows researchers to be more confident in any causal inferences drawn from the study. However, this type of methodology has been criticized for its artificiality (Visser et al. Reference Visser, Krosnick, Lavrakas, Reis and Judd2000).
While researchers commonly use written narratives to study human behavior, research has shown that the presentation of materials may alter participants’ decision-making processes. For example, research on affective forecasting has found significant differences in people’s predictions about their future feelings (based on a narrative description of a future event) and their emotional response to the actual event (Wilson & Gilbert Reference Wilson and Gilbert2003). Other research has shown that videos (versus text descriptions) can significantly increase hindsight bias, breed overconfidence, and that other phenomenon, such as the propensity effect, may only be triggered by interactive or video stimuli (Roese et al. Reference Roese, Fessel, Summerville, Kruger and Dilich2006; Fessel & Roese Reference Fessel and Roese2011). This may be because interactive stimuli increase processing fluency (Fessel & Roese Reference Fessel and Roese2011) and are more likely to activate deeper meaning than text stimuli (Spruyt et al. Reference Spruyt, Hermans, Houwer and Eelen2002) or that more realistic stimuli lead to more genuine responses (Blascovich et al. Reference Blascovich, Loomis, Beall, Swinth, Hoyt and Bailenson2002). Therefore, it is important to examine both written narrative and interactive game approaches when examining behavioral decision-making.
2.2 Serious gaming
To date, educational and training contexts dominate serious gaming research. Researchers in education and training recognize (1) those born since the 1980s have been exposed to video games their entire lives (Zyda Reference Zyda2005), and (2) well-designed games motivate immersion (Yee Reference Yee2006; Greitzer et al. Reference Greitzer, Kuchar and Huston2007; Bostan & Kaplancali Reference Bostan and Kaplancali2009). Educators have taken advantage of these factors to increase engagement and motivation in the learning environment (Lloyd & Poel Reference Lloyd and Poel2008; Doucet & Srinivasan Reference Doucet and Srinivasan2010; Froschauer et al. Reference Froschauer, Arends, Goldfarb and Merkl2011; Huang Ling Reference Huang Ling2011). Similarly, serious gaming has expanded into other application areas such as crowd sourcing (Van ’t Woud et al. Reference Van ’t Woud, Sandberg and Wielinga2011; Franco Reference Franco2012; Kawrykow et al. Reference Kawrykow, Roumanis, Kam, Kwak, Leung, Wu and Phylo2012) and physical therapy (Rego et al. Reference Rego, Moreira and Reis2010; Geurts et al. Reference Geurts, Vanden Abeele, Husson, Windey, Van Overveldt, Annema and Desmet2011).
In terms of education and training, serious gaming has already been utilized in a number of contexts across a diverse range of topic areas such as engineering ethics (Lloyd & Poel Reference Lloyd and Poel2008), energy sustainability (Doucet & Srinivasan Reference Doucet and Srinivasan2010), politics (Huang Ling Reference Huang Ling2011), and art history (Froschauer et al. Reference Froschauer, Arends, Goldfarb and Merkl2011). Furthermore, serious games have been used in medical settings to help enact behavioral change related to better treatment outcomes in patients with a variety of illnesses such as asthma (Bussey-Smith & Rossen Reference Bussey-Smith and Rossen2007) and cancer (Beale et al. Reference Beale, Kato, Marin-Bowling, Guthrie and Cole2007). In terms of training, serious gaming has demonstrated value as a training tool in fields such as oil drilling (Brasil et al. Reference Brasil, Neto, Chagas, Monteiro, Souza, Bonates and Dantas2011), inventory management (van der Zee et al. Reference van der Zee, Holkenborg and Robinson2012), network security (Greitzer et al. Reference Greitzer, Kuchar and Huston2007), and medical triage (Heinrichs et al. Reference Heinrichs, Youngblood, Harter and Dev2008).
Aside from training and educational purposes, serious gaming can also be useful for intervention and research. Serious games have been shown to be useful as intervention tools for health promotion, including physical therapy, healthy eating, sexual health and mental health treatment and prevention to name a few (Desmet et al. Reference DeSmet, Van Ryckeghem, Compernolle, Baranowski, Thompson, Crombez and Van Cleemput2014; Fleming et al. Reference Fleming, Cheek, Merry, Thabrew, Bridgman, Stasiak and Hetrick2014; Desmet et al. Reference DeSmet, Shegog, Van Ryckeghem, Crombez and De Bourdeaudhuij2015; Shegog et al. Reference Shegog, Brown, Bull, Christensen, Hieftje, Jozkowski and Ybarra2015; Thompson et al. Reference Thompson, Bhatt, Vazquez, Cullen, Baranowski, Baranowski and Liu2015). Economists have suggested that virtual environments in video games may serve as adequate and less expensive substitutes for laboratory settings in the conduct of economics research, which normally require examining real-world situations (Castronova Reference Castronova2008; Chesney et al. Reference Chesney, Chuah and Hoffmann2009).
2.3 Serious gaming in engineering
Engineering researchers have used serious gaming in a number of applications ranging from engineering education to facilitating the design process. We classify games found in the engineering literature using the G/P/S model (Djaouti et al. Reference Djaouti, Alvarez and Jessel2011). This model has three dimensions: Game play, Purpose, and Scope. Along the Game-play dimension, games are either play-based (PB), i.e., lacking in defined objectives and rules for players, or game-based (GB), i.e., featuring defined objectives and rules for players. The Purpose dimension categorizes games based on their intended function. The following are the three, broad serious game functions:
-
(i) Message broadcasting (MB) – the game is intended to deliver some type of educative, informative, or persuasive message.
-
(ii) Training (T) – the game is intended to improve cognitive or physical performance.
-
(iii) Data exchange (DE) – the game is intended to facilitate the exchange of data between players or from the player to the game facilitators.
The final dimension, Scope, captures the context of the game as well as its intended player base.
Table 1 classifies games used in engineering with the G/P/S model. As shown in this table, games are not only used in engineering educative message broadcasting and training but also to facilitate data exchange between both collaborating designers and between study participants and researchers. However, games listed that facilitate data exchange are classified as play-based (PB) as they do not supply objectives to players but rather allow players to formulate their own objectives; the game acts merely as a mechanism for data exchange. For example, Habraken & Gross (Reference Habraken and Gross1988) formulated the silent game wherein two players take turns modifying an arrangement of game pieces; the game itself offers no objective to the players but rather allows the players to formulate their own objectives when arranging game pieces. The play-based games listed in Table 1 are referred to as games by their authors.
Table 1. Gaming with engineering applications

The purpose of the current research is to design a gaming application that measures human decision-making within an engineering context. As a research tool, the purpose of our gaming application is data exchange (DE), and we define objectives for the player base such that game play is game-based (GB) rather than play-based. Defining objectives for the player base allows us to observe how different players approach meeting the same objective, and therefore, we can measure the frequency and impact of decision-making strategies. This approach is similar to prior uses of serious gaming that seek to use human-derived strategies for computational algorithm improvement, see Cooper et al. (Reference Cooper, Khatib, Treuille, Barbero, Lee, Beenen and Popović2010) and Ren et al. (Reference Ren, Bayrak and Papalambros2016). However, our motivation is describing engineers’ decision-making biases and tendencies on a foundational level that we can then use to improve engineers’ decision-making capabilities in future work.
2.4 Serious game design
Serious game design is similar to the design of games for entertainment, with the ultimate goal of education, research, and/or training (Zyda Reference Zyda2005). Serious games designed with the context of our proposed usage can be viewed as human-in-the-loop simulations (Greenblat Reference Greenblat1988) offers the following five stages for simulation-based serious game development:
-
(1) setting objectives and parameters – as in identifying the game’s purpose, context of use, and resources available for development;
-
(2) model development – as in identifying the major referent system characteristics;
-
(3) decisions about representation – as in linking model elements with game elements;
-
(4) construction and modification – as in coding software;
-
(5) preparation for use by others – as in producing operating material and opening distribution channels.
As mentioned in the previous section, the objective for our serious game application is to collect data (purpose) concerning cognitive and social biases in engineering scenarios (context).
The model development stage can be expanded using van der Zee’s framework for creating conceptual models for simulation games, which itself is an adaptation of Robinson’s framework for developing conceptual models for simulation (Robinson Reference Robinson2008a ,Reference Robinson b ; van der Zee et al. Reference van der Zee, Holkenborg and Robinson2012). This framework largely consists of identifying model outputs, inputs, and content. For applications in decision-making research, the content of the conceptual model should address all of the elements of a decision, which include decision alternatives, decision outcomes, perceptions about how alternatives relate to outcomes, and preferences over decision outcomes. By properly controlling these decision elements, researchers can observe how participants’ real-world decisions may differ from predictions, i.e., from normative decision theory.
A key motivation for the use of serious gaming is that games – through their entertainment value – promote immersion into a study scenario (Koster Reference Koster2005). Entertainment results from positive experiences (Ritterfeld et al. Reference Ritterfeld, Cody and Vorderer2009), and Greitzer et al. (Reference Greitzer, Kuchar and Huston2007) identify keeping players in a flow zone facilitates immersion. Difficult games or games that introduce mechanics too quickly can frustrate players such that immersion and interest in game play is broken. Similarly, easy games and games that introduce mechanics too slowly can bore players with the same consequence. A best practice for game design is to introduce game mechanics systematically to keep players in a flow zone. Introducing game mechanics systematically affords players the opportunity to learn how they can affect the state of a game. However, it is unclear if a game’s graphical representation and mechanics create confounding factors when trying to isolate a particular cognitive bias. Since confounding factors could lead to difficulty interpreting the data, the current research is concerned with gaining insight into how a graphical interface and sequential introduction of game mechanics might influence the study results.
3 Study overview
There are many open questions about the effectiveness of using games to study decision-making in engineering design and best practices for applying it. In this investigation, we use this framework to investigate a relevant systems engineering phenomenon: the sunk cost effect, i.e., the propensity of decision makers to favor alternatives based on prior investment of resources or effort rather than actual future value (Arkes & Blumer Reference Arkes and Blumer1985). The sunk cost effect is widely studied in the psychology community, which allows us to construct scenarios based on those found in prior sunk cost research. We chose to investigate the sunk cost effect because it is conceivable that game play itself could constitute a prior investment of effort as opposed to, or in addition to, any prior investment stated in a narrative that participants are simply told had been expended. Therefore, game play could magnify the sunk cost effect in a game embodiment of a sunk cost decision-making scenario relative to a written narrative embodiment of the same scenario.
3.1 The sunk cost effect
In principle, prior investments of resources or effort – i.e., sunk costs – should have no bearing on a decision. Rather, rational decision makers should rank alternatives based only on their future outcomes. For example, an investor purchasing shares of a stock should base their decision on beliefs about the future stock price and not whether he or she had lost money investing in the same stock previously.
Arkes & Blumer (Reference Arkes and Blumer1985) conducted a series of experiment that highlight the deviation from rational decision theories in the context of sunk cost narratives. In one experiment, they presented participants the following scenario:
Assume that you have spent $100 on a ticket for a weekend ski trip to Michigan. Several weeks later you buy a $50 ticket for a weekend ski trip to Wisconsin. You think you will enjoy the Wisconsin ski trip more than the Michigan ski trip. As you are putting your just-purchased Wisconsin ski trip ticket in your wallet, you notice that the Michigan ski trip and the Wisconsin ski trip are for the same weekend! It is too late to sell either ticket, and you cannot return either one. You must use one ticket and not the other. Which ski trip will you go on?
The problem is reduced to the following: you have spent $150 for two ski trip tickets to separate locations, you can only use one, and you like one of the destinations better. We would expect a rational individual to go on the Wisconsin ski trip since the $150 cost is common to both alternatives but the Wisconsin trip will be more enjoyable. However, Arkes and Blumer observed that when given this scenario, 33 out of 61 respondents chose the Michigan trip, presumably because a larger amount was spent on its ticket. Additional research supports the idea that sunk cost impacts decision-making and extends these findings by showing that its impact is magnified with greater levels of prior investment and proportion of project completion (Garland Reference Garland1990; Garland et al. Reference Garland, Sandefur and Rogers1990; Keil et al. Reference Keil, Truex and Mixon1995; Mann Reference Mann1996; Viswanathan & Linsey Reference Viswanathan and Linsey2013).
The sunk cost effect has been shown in more than just lab-based contexts. For example, sunk cost has been identified as a potential reason for setbacks or failures in a number of systems engineering projects including the Concorde airliner (Arkes & Ayton Reference Arkes and Ayton1999), a mobile drilling rig (Paté-Cornell Reference Paté-Cornell1990), and the Mars Climate Orbiter (Shore Reference Shore2008). However, this has not been a consistent finding. For example, researchers do not find evidence for the sunk cost effect in the context of oil exploration (Garland et al. Reference Garland, Sandefur and Rogers1990). While these findings appear inconsistent, research has shown that several factors may mitigate the sunk cost effect, e.g., when an explicit alternative is available (Keil et al. Reference Keil, Truex and Mixon1995; Vermillion et al. Reference Vermillion, Malak, Smallman and Fields2014), when sunk costs are in terms of time (Soman & Cheema Reference Soman and Cheema2001; Navarro & Fantino Reference Navarro and Fantino2009), and when sunk costs are stated absolutely as opposed to as a proportion of a budget (Garland & Newport Reference Garland and Newport1991). Furthermore, the sunk cost effect seems to be sensitive to the context of decisions, e.g., medical decisions versus nonmedical decisions (Bornstein et al. Reference Bornstein, Christine Emler and Chapman1999).
3.2 Experimental design and implementation
Experiment 1 involves a manned Mars mission narrative, and Experiment 2 involves an oil drilling narrative. Test conditions in both experiments are defined using a combination of: (a) whether the problem given to subjects involves a sunk cost, and (b) whether subjects play an interactive game or are given a text-based narrative of the problem. Thus, both experiments have a general 2 (sunk cost versus no sunk cost)
$\times 2$
(game versus written narrative) design. Experiment 1 has additional conditions that are discussed in Section 4.1.
One of the implementation challenges is to embody the desired narrative in game play. Elements in the narrative can be explicitly stated or, in the case of the game, learned or inferred through game play. Therefore, there can be many different manifestations of the same narrative in a game. Table 2 summarizes the high-level implementation strategy for the Mars and oil drilling games. These implementations are elaborated in Sections 4 and 5, respectively.
Table 2. Narrative implementation by condition

4 Experiment 1: manned Mars mission
4.1 Experiment 1 design
To test if introductory learning levels (i.e., game play intended to systematically introduce game mechanics to the participant) influence the decisions of a participant, we tested two control conditions (i.e., no sunk cost) in the game-based implementation: one where participants make a design decision at the beginning of the game (control-beginning) and one where participants make a design decision after they play learning levels (control-middle). In addition, we tested two sunk cost conditions for the game-based implementation: one in which action outcomes are stated explicitly (sunk cost-explicit) and one in which outcomes are left implicit (sunk cost-implicit). These two sunk cost conditions are implemented with learning levels. This allows us to examine the best mechanism for translating the sunk cost effect from a written narrative into an interactive game format. Figure 1 summarizes the experimental design. All written narrative and game-based conditions are described in further detail below.
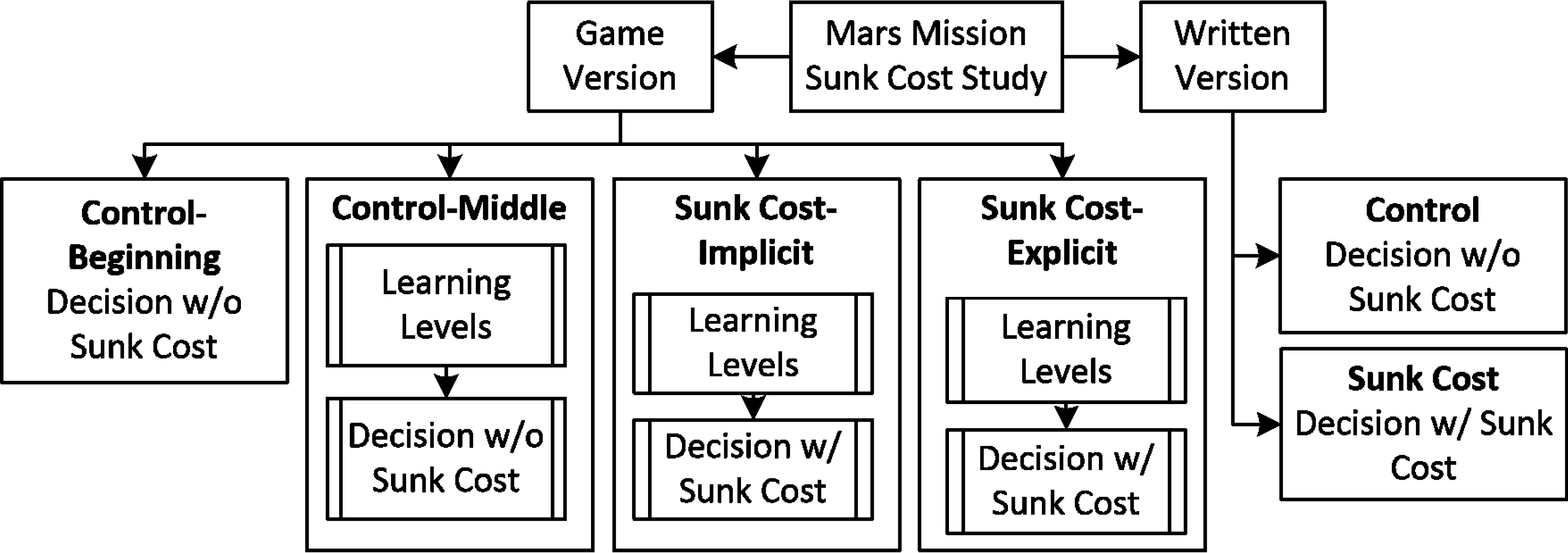
Figure 1. Summary of Experiment 1 conditions.
4.2 Participants
Participants consisted of undergraduate students (
$N=271$
) from a large, U.S. public university who received credit toward a psychology course as compensation. Participants were between the ages of 17 and 25 (
$M_{\text{age}}=18.79$
). The majority of participants were Caucasian, non-Hispanic (
$N=202$
, 74.5%). Participants were randomly assigned to one of six conditions (game – control-beginning, control-middle, sunk cost-implicit, sunk cost-explicit; written narrative – control, sunk cost) and completed their tasks in a supervised, laboratory environment where they were not allowed to collaborate. Participants in the Manned Mars Mission experiment did not participate in the Oil Drilling experiment. Participants had 30 min to complete their assigned study tasks.
4.3 Experiment 1 scenario
Participants are placed in a scenario in which they assume the role of a project leader designing a crew habitation module for a manned mission to Mars. In this role, participants must make a decision to either (a) design and develop a crew habitation module in-house or (b) purchase another spaceflight organization’s crew habitation module. This scenario is highly abstracted as to not require specific engineering domain knowledge. The written narrative and game implementations of this scenario are described below.
4.3.1 Written narrative implementation
There are two variants of this scenario in a written narrative format, a control version and a sunk cost version. In the control variant, participants are informed prior to designing an in-house module that another space flight organization has made their module available for purchase. The control version of the written narrative is as follows:
You are the head of a space flight project whose goal is to safely send six astronauts to Mars. As part of this project you plan to use the last 50 million dollars of your research and development budget to create a crew habitat module, a critical component of the mission to Mars.
However, another spaceflight organization has just begun marketing their own crew habitat module. Also, it is apparent that their habitat module is more robust and more likely to succeed during the mission.
The question is: should you invest the last 50 million dollars to build your own habitat module?
The available responses are (1) Yes – invest the funds in building an in-house module or (2) No – do not invest the funds on the in-house module with the implicit understanding that the alternative module will be purchased.
The scenario’s sunk cost variant is largely the same except the information about the alternative crew habitation module is provided after an investment into an in-house module has been made (90% of budget has been used). The sunk cost version of the written narrative is the following:
You are the head of a space flight project whose goal is to safely send six astronauts to Mars. So far, you have invested 450 million dollars of the organization’s money into this project. As part of this project you plan to create a crew habitat module, a critical component of the mission to Mars. To finish this part of the project would cost the last 50 million dollars of your research and development budget.
When the project is 90% completed, another spaceflight organization begins marketing their own crew habitat module. Also, it is apparent that their habitat module is more robust and more likely to succeed during the mission.
The question is: should you invest the last 50 million dollars to finish building your own habitat module?
Similarly to the control variant, the available options are (1) Yes – invest the funds in building an in-house module or (2) No – do not invest the funds. The written narrative is formulated similarly to the sunk cost narratives of Arkes & Blumer (Reference Arkes and Blumer1985).
4.3.2 Game implementation
In the game, rather than being told their investment, they invest their budget in actually designing a crew habitation module. This module is given a robustness attribute (a fictional figure of merit signifying the module’s potential success in the mission). Increasing the module’s robustness score increases its probability of success. Participants influence the module’s robustness by arranging red, yellow, and blue blocks; each color representing a different subsystem. Blocks closer to a specified target location contribute to a higher robustness score. The block-arrangement interface is shown in Figure 2.

Figure 2. Top left: example of the block-arrangement interface. Top right: game level hierarchy. Bottom left: decision prompt from the sunk cost-implicit condition. Bottom right: decision prompt from control condition.
Furthermore, participants are informed that another space organization has developed a crew habitation module with the implication that this alternate module is more robust than anything the participant can create. Given this information, participants must make the decision whether to continue with their own in-house crew habitation module design or not, similar to the decision posed in the written narrative. In the sunk cost conditions, participants are given a budget of $450 million, and each time they analyze a design candidate, i.e., click on an analyze button to evaluate an arrangement’s robustness, $50 million is deducted from the budget. The decision to continue with their design or not is presented after participants have invested 90% of their allotted budget (in parallel with the written narrative). After participants decide on a final design, the Mars mission is simulated so the participants can see if their craft arrived to Mars or not. Because of the ambiguity of what the outcome of a ‘No’ response would be, a second sunk cost condition was developed in which participants are told explicitly that choosing ‘No’ will lead to the purchase of the alternate module; this condition is termed sunk cost-explicit. The original sunk cost condition that follows more closely with the written narrative version is termed sunk cost-implicit. Figure 1 summarizes the game conditions.
Since we were interested in what they inferred would happen if they made a ‘No’ response, we asked participants in the game’s nonexplicit conditions (control-beginning, control-midgame, and sunk cost-implicit) what they thought was going to happen if they chose ‘No’, i.e., to not continue with their own crew module. Available responses were (1) the alternative will be bought, (2) the mission will be scrapped, or (3) other. We assumed those in the sunk cost-explicit would know that the outcome of choosing ‘No’ is to buy the alternative crew module since it is explicitly stated.
To familiarize participants with the mechanics of the game, learning levels precede the crew habitation module level. These levels are intended to give participants experience with the block-arrangement interface and the relationship between the robustness score and probability of mission success. However, the time spent playing these learning levels could be considered sunk cost as the players have invested effort into playing the game. Thus, players may exhibit sunk cost behavior even without investing budget in developing the crew habitat module (the standard sunk cost manipulation). Therefore, we included two control conditions: one in which participants make a decision whether or not to create their own crew habitat module design at the beginning of game play (control-beginning) and one in which they make the decision after the learning levels but before beginning of the crew habitation module level (control-midgame).
The game interface is built using web languages – such as HTML, CSS, and JavaScript – and runs through a web browser. The telemetry back end is also web-based such that participants’ responses are collected and stored in a database in real time. Time measures were only recorded for the final game level containing the decision to continue with their current design or not. On average, participants spent 5.5 min on this level. Extrapolating across all levels, we expect an average of 16.5 min of play time with approximately 11 min of ‘sunk cost’ time in the learning levels.
5 Experiment 2: oil drilling scenario
5.1 Experiment 2 design
With Experiment 1 providing a better understanding of how sunk cost may function in a gaming environment, Experiment 2 involved a more streamlined design to test for differences in the sunk cost effect in a visual, interactive environment compared to a written narrative. In particular, we designed the game for Experiment 2 to be less of a puzzle game, as the Manned Mars Mission, and more of an interactive narrative game. By implementing a different game genre and representation, we can better generalize our findings beyond the original game scenario. Figure 3 summarizes the design, and all conditions are described in further detail below. We consider the mechanics of the Oil Drilling game to be less cumbersome than those of the Manned Mars Mission game, and thus do not include learning levels in the Oil Drilling game.

Figure 3. Summary of Experiment 2 conditions.
5.2 Participants
Participants consisted of undergraduate students (
$N=212$
) from a large, U.S. public university who received credit toward a psychology course as compensation. Participants were between the ages of 18 and 30 (
$M_{\text{age}}=19.44$
). The majority of participants were Caucasian, non-Hispanic (
$N=170$
, 80.2%). Participants were randomly assigned to one of four conditions (game-control, sunk cost; written narrative-control, sunk cost) and completed their tasks in a supervised, laboratory environment where they were not allowed to collaborate. Participants in the Oil Drilling experiment did not participate in the Manned Mars Mission experiment. Participants had 30 min to complete their assigned study tasks.
5.3 Experiment 2 scenario
Participants are presented with an oil drilling scenario. Consistent with the Manned Mars Mission scenario, this scenario is devised to be generic in that it does not rely on specific knowledge from any particular engineering domain. Participants assume the role of drilling operations manager. They are presented with information that a pocket of granite has been discovered in their current drilling location and must make a choice between (a) drilling in the current drilling location or (b) moving the drilling rig to a new location to avoid the granite pocket.
5.3.1 Written narrative implementation
There are two variants of this written narrative: a control condition and a sunk cost condition. In the control condition, participants are informed that a seismic scan shows a dense pocket of granite directly under the intended drilling location; this information comes before any amount of the drilling budget has been invested, thus no sunk costs are present. The control version of this written narrative is given below:
You are the drilling manager for an oil drilling operation. Your goal is to maximize net profits. We plan to invest the last 10 million dollars to drill in the current location. However, seismic tests revealed there is a pocket of granite in our drilling location. It is unlikely that our drill head will be able to penetrate it. Another drilling company has another type of drill head that they can sell us. It is a stronger drill head, which has a better chance of penetrating the granite.
The question is: Should we invest the last 10 million dollars of our budget to continue drilling with the current drill head in the present location?
The sunk cost condition is substantially the same scenario, but differs in that the participant is not informed of the granite pocket until after an investment has been incurred. The sunk cost version of the written narrative is given below:
You are the drilling manager for an oil drilling operation. Your goal is to maximize net profits. So far, we have invested 5 million dollars in setting up to drill in this location. However, seismic tests revealed there is a pocket of granite in our drilling location. It is unlikely that our drill head will be able to penetrate it. Another drilling company has another type of drill head that they can sell us. It is a stronger drill head, which has a better chance of penetrating the granite.
The question is: Should we invest the last 10% of our budget to finish drilling with the current drill head in the present location?
In both cases, the options available to participants are ‘Yes’ (continue drilling with current drill head) or ‘No’ (do not continue), and we would expect a rational decision maker to choose ‘No’. The written narrative is formulated similarly to the sunk cost narratives of Arkes & Blumer (Reference Arkes and Blumer1985).
5.3.2 Game implementation
The game is designed to parallel the written narrative in terms of story line and numerical facts. However, the game has added interactive characteristics. This game is built using the same open source frameworks as the manned Mars mission game. The game begins by familiarizing the participant with the user interface and describing the game objective (to maximize net profit). The user interface is comprised of three components: a view port showing the drilling operation graphically, a text box indicator of net profit, and a text box indicator that conveys what is happening during the drilling operation. Figure 4 shows examples of this interface.

Figure 4. Oil game interface examples. Top: selection between Sites A and B with annotation highlighting the two sites. Bottom: decision prompt for the sunk cost condition.
An important distinction between the written narrative and the game is that there are added decisions in the game. After participants are familiarized with their role and the user interface, they choose a drill site. There are two drill sites from which to choose, and each has associated with it an estimated crude value. Site A has a potential value of $115M
$\pm$
$1M and Site B has a potential value of $125M
$\pm$
$5M. Site B is the rational selection as the worst possible crude value at Site B is still greater than the best possible crude value at Site A. This decision is added to determine whether or not participants are making decisions based on the in-game objective (maximize net profit).
Once participants choose a site, they use the keyboard’s arrow keys to move their drilling rig to their desired drilling location and initiate drilling. In the control condition, participants are told immediately (before any drilling has begun) about the pocket of granite that will likely prevent reaching any crude. In the sunk cost condition, the drill initiates and begins its descent into the ground; as the drill gets deeper, money is subtracted from the participant’s net profit. When the participant has spent $5 million, they are informed about the pocket of granite. In both conditions, participants are asked the same question as in the written narrative described above with the same options: ‘Yes’ (continue drilling with current drill head) or ‘No’ (do not continue); if a participant chooses ‘No’, a stronger drill head is attached to the drilling rig. Following this decision, participants play the rest of the game which entails installing a pump and pumping crude to the surface, and finally discovering how much net profit their decisions produced.
6 Results
6.1 Experiment 1 results
Experiment 1 game and written narrative responses are summarized in Tables 3 and 4, respectively, and Figure 5 graphically shows proportion distributions for each condition. Statistical comparisons using Pearson’s chi square tests between game and written narrative results are summarized in Table 5. The control conditions of the game are compared to the control condition of the written narrative, and likewise, the sunk cost-implicit condition of the game is compared to the sunk cost condition of the written narrative. The sunk cost-explicit condition of the game is intentionally excluded as it has no equivalent in the written narrative. For all of the comparisons in Table 5,
$p>0.05$
. Therefore, independence between response and method of presentation, i.e., game or written narrative, cannot be rejected with 95% confidence, suggesting that there is no significant difference between performance in the game and performance in the written narrative. A limitation of this study is the disparity between the numbers of participants in each game condition and the number of participants in each written narrative condition. Roughly, the number of participants in a given game condition was twice the number in a written narrative condition. This disparity leads to low statistical power when comparing game and written narrative results.

Figure 5. Sample proportions showing the difference between game and written narrative response distributions.
Table 3. Response distribution in Experiment 1 game conditions

Table 4. Response distribution in Experiment 1 written narrative conditions

Table 5. Summary of Experiment 1 game versus written narrative comparisons

Now let us compare the control and sunk cost responses for the written narrative and game to see if there are significant differences in responses. Table 6 summarizes using Pearson’s chi square tests between the control and sunk cost conditions of the written narrative and game, respectively. Comparing game conditions, no significant difference in response is detected. The statistical
$p$
-value between the responses in the written narrative’s control and sunk cost conditions is significant. As mentioned in the previous paragraph, the number of participants that used the written narrative method is about half of the number of participants that played the game. It is unclear if more participants in the written narrative would intensify or mitigate statistical difference between responses in the control and sunk cost conditions. However, we see qualitatively that detecting statistically significant results in the sunk cost study might depend on the method in which we embody the study. This observation is verified in Experiment 2.
Table 6. Summary of Experiment 1 control versus sunk cost comparisons

Let us turn focus to comparing the two game control conditions and the two game sunk cost conditions. For the game’s two control conditions, i.e., control-beginning versus control-midgame, response is not dependent on condition,
$\unicode[STIX]{x1D712}^{2}\;(1,N=108)=0.49$
,
$p=0.49$
. Therefore, playing the learning levels did not elicit an effect that differs from not playing the learning levels. Similarly, response is not dependent on game sunk cost condition, i.e., sunk cost-implicit versus sunk cost-explicit,
$\unicode[STIX]{x1D712}^{2}\;(1,N=111)=0.03$
,
$p=0.86$
. Therefore, the added information clarifying that a ‘No’ response leads to the purchase of an alternative module did not affect response. Interestingly, as shown in Figure 6, the perceived outcomes to a ‘No’ response across the control-beginning, control-midgame, and sunk cost-implicit conditions are fairly homogeneous; most believed buying the alternative was the associated outcome but some believed the Mars mission would be scrapped by choosing ‘No’. Aggregating the perceived outcomes, participants who believed that the associated outcome was buying the alternative were generally split between choosing ‘Yes’, continue, and ‘No’, do not continue. However, those who believed that the associated outcome was scrapping the mission overwhelmingly choose ‘Yes’, as shown in Figure 7. We can infer from this trend, within the game context, that these participants would rather risk an unsuccessful mission than one that was canceled. This trend could imply at a higher level that these participants chose to continue either out of a desire to continue playing the game or a concern about their mission failing. The former interpretation lends support to the hypothesis that, at least in this game, a portion of the participant base values game play over the objectives set by the game scenario, i.e., to work toward a successful Mars mission.

Figure 6. Distributions of perceived outcome to a ‘No’ response.

Figure 7. Response by perceived outcome.
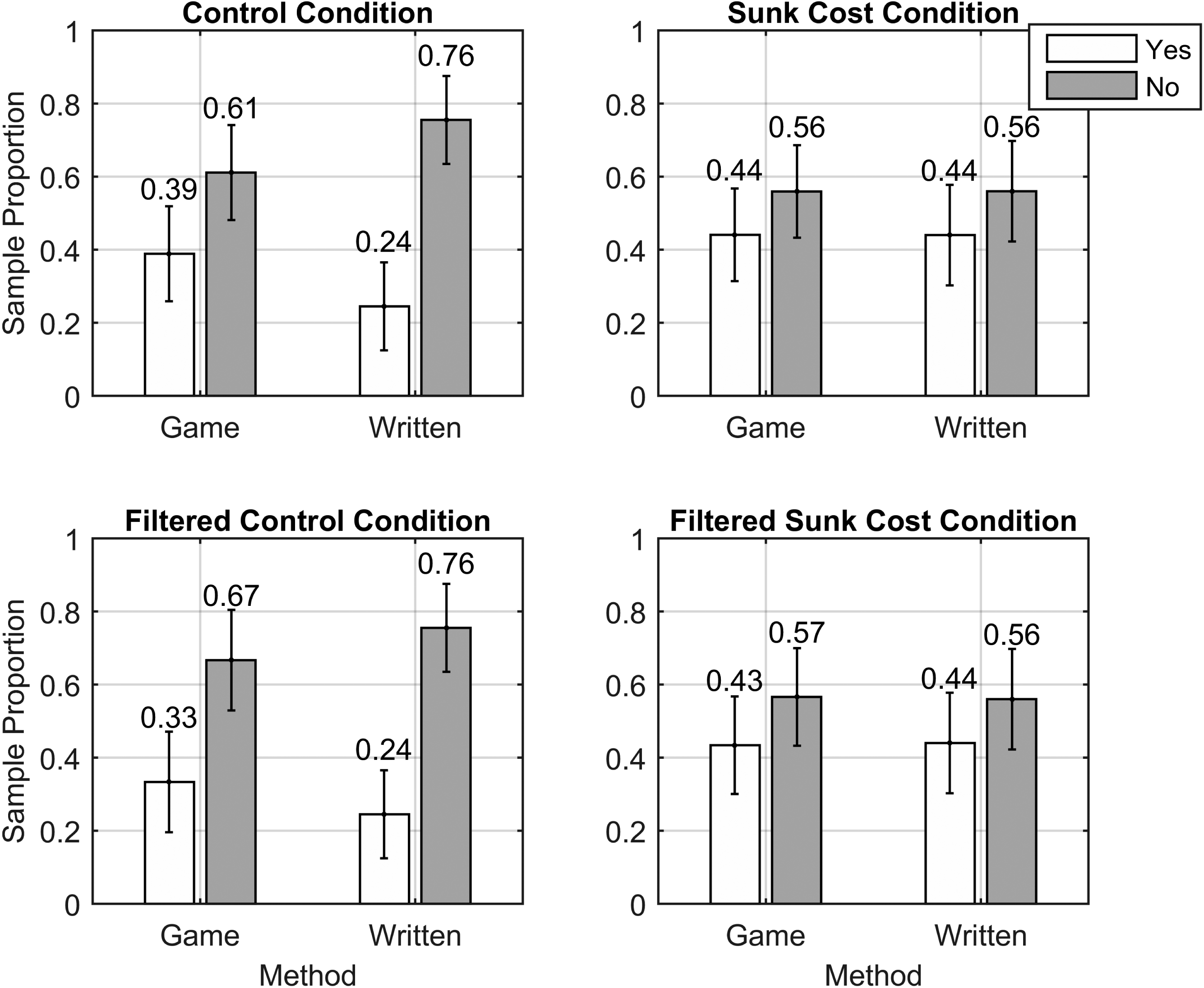
Figure 8. Graphical comparison of game and written narrative response distributions. Top row shows unfiltered game response distributions while the bottom row shows filtered game response distributions (i.e., those who chose Site B).
6.2 Experiment 2 results
Game and written narrative responses are summarized in Tables 7 and 8, respectively. However, the drilling site decision embedded into the game (see Section 5.3.2) affords the ability to filter responses based on rationality in this decision. Because this decision is presented simply, a nonrational response, i.e., selecting Site A, could be viewed as the participant not fully reading or understanding the information given to them. In choosing which site to drill, 98 game participants, approximately 87%, chose rationally, i.e., chose Site B. Of the 15 game participants that chose irrationally, 9 were in the control condition (6 chose ‘Yes’ and 3 chose ‘No’) and 6 were in the sunk cost condition (3 chose ‘Yes’ and 3 chose ‘No’); the choices of these participants suggest that they did not fully understand the goal of the game. If we use site selection as a filter and remove the data of those that chose irrationally, the game response distributions are those in Table 9. Figure 8 shows response proportions for each condition.
Table 7. Response distribution in Experiment 2 game conditions

Table 8. Response distribution in Experiment 2 written narrative conditions

Table 9. Response distribution in game filtered by site selection choice (i.e., those who chose Site B)

Table 10. Summary of Experiment 2 game versus written narrative comparisons
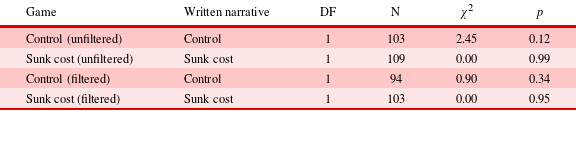
Table 11. Summary of Experiment 2 control versus sunk cost comparisons

Table 10 summarizes statistical comparisons using Pearson’s chi square test between game conditions and written narrative conditions and shows that for all comparisons,
$p>0.05$
. This means we do not observe a statistically significant difference between game and written narrative responses despite whether game results have been filtered (selecting only those players who chose the rational Site B) or not. This result is consistent with Experiment 1 wherein the gaming environment elicits statistically similar behavioral effects as the written narrative version.
We now statistically compare the control and sunk cost conditions for each study embodiment method using Pearson’s chi square test. Table 11 summarizes these statistical comparisons. We see that there is a statistically significant effect detected between the control and sunk cost responses from the written narrative. However, there is no significant effect detected using the game regardless of whether we filter the responses by the site selection decision. Therefore, significant effect detection is dependent on the study embodiment method.
7 Conclusions
The following conclusions are supported by statistical analyses of the data presented in the previous sections:
-
(i) In both experiments, there is no statistically significant difference in participant responses between written narrative and game sunk cost study embodiment methods.
-
(ii) In both experiments, there is a statistically significant difference in participant responses between the control and sunk cost conditions of the written narrative, but no such difference between the control and sunk cost conditions in the game embodiment.
-
(iii) In Experiment 1, game participants who believed the Mars mission would be aborted if they selected ‘No’ more frequently chose ‘Yes’ (to invest the resources in their in-house design).
-
(iv) In Experiment 1, there is no statistically significant difference between the game-control condition that uses learning levels and the game-control condition that does not.
The first and second conclusions seemingly contradict each other. What we can infer from these two conclusions that the game does not induce completely different or contradictory trends from the written narrative, but there is greater noise in the game response distributions that is large enough to preclude detecting significant differences. Greater noise in data collected from game embodiments suggests a large participant sample size is needed to detect any significant effects. Greater noise might be a symptom of a sunk cost effect induced by game play itself. The third conclusion might support this speculation.
The third conclusion suggests players in Experiment 1 who believed that selecting ‘No’ would scrap the mission were more likely to continue with the mission even though that option is irrational. What is unclear is the context in which participants were making this decision. Participants could have been making this decision (1) within the context of the scenario presented to them, in which case participants would therefore prefer to attempt the mission rather than scrapping it, or (2) within the context of the experiment, in which case participants would prefer to continue game play rather than facing the possibility of stopping game play. If the latter is true, then the in-game narrative objectives may be in conflict with the meta-level objectives of game players: to actively play a game. Therefore, it is no surprise that the game embodiment would have noisier response distributions than the written narrative embodiment. Aldrich (Reference Aldrich2009) notes that playing a game is inherently an active exercise and some mechanics, such as doing nothing, can be counterintuitive for game players. As with other game mechanics, counterintuitive mechanics should be introduced through learning levels to familiarize players with the concept and how it influences the state of the game. However, if participants made their decision within the context of the game narrative, the fact that players formulated different outcomes based on not investing resources in their in-house design can motivate future research into the inferences made by engineers during decision-making tasks. For example, do engineering decision makers generally formulate decisions as a ‘continue with project A or do not continue with project A’ type decision or as a ‘continue with project A or switch to project B’ type decision? Keil et al. (Reference Keil, Truex and Mixon1995) suggest formulating decisions in these two different ways can produce different trends in what is ultimately chosen.
The fourth conclusion suggests that systematic introduction of game mechanics through learning levels is not the primary or sole factor that contributes to greater noise in game data. While the games presented in this paper may be simple enough that learning levels are not required, the use of learning levels is best practice in game design, and they are likely needed when game designs are more complex to keep players in a flow zone, as discussed in Section 2.4.
Given the experimental results and our experiences using games for decision-making research, we recommend the following research paths for future work on game-based embodiments of decision-making scenarios:
-
(i) Best practices for game design in decision-making research contexts: This paper used game design strategies from prior work on serious gaming. However, game design specifically for decision-making research can conceivably be streamlined to give decision-making researchers a clear framework from which to design and construct games-based embodiments of their conceptual studies. An important consideration with any human subjects study is the individual differences among participants. For example, prior research suggests there are differences between expert and novice engineers in some areas such as how they generate, represent, and evaluate ideas (Ahmed et al. Reference Ahmed, Wallace and Blessing2003; Cross Reference Cross2004; Dixon & Johnson Reference Dixon and Johnson2011). Additionally, age differences between expert and novice engineers may impact how they view and play games. Conceivably, the optimal game design strategy to introduce mechanics and keep players in a flow zone may differ with a study’s targeted player base.
-
(ii) Best practices for game-based decision-making studies: The studies presented in this paper were conducted in a supervised laboratory setting, and participants played the game in a single session. However, the impact of allowing participants to play the games in an unsupervised setting and/or allowing participants to play a game over several sessions is unclear. Additionally, the conclusions in this paper suggest games induce noisy data such that a greater sample size might be needed to detect any statistically significant results. Establishing best practices for using games in a decision-making research context should resolve these issues for future uses of gaming in research contexts.
8 Summary
Engineering design research can benefit from an efficient mechanism to supplement decision-theoretic work with engineers’ decision-making characteristics. Serious gaming is an alternative approach for operationalizing decision-making scenarios that can facilitate study distribution and data collection. Given the novelty of gaming as a human decision-making research tool, we set out to understand the behavioral effects of using games. The experiments described in this paper represent largely exploratory activities toward evaluating the use of games, or gaming-inspired computer programs, as a means to build behavior profiles of engineers and ultimately to evaluate design and systems engineering methods and theories. The results lend support that gaming environments do not necessarily induce significantly unexpected behavior when compared to behavior captured through a written narrative, but do suggest that games induce noisier data when compared to a written narrative equivalent.
Acknowledgments
This material is based upon the work supported by the National Science Foundation under grant no. CMMI-1346553. Opinions expressed in this paper are of the authors and do not necessarily reflect the views of the National Science Foundation.