Book contents
- Frontmatter
- Contents
- Preface
- Acknowledgments
- I Introducing SAS Enterprise Guide
- II Performing Analyses and Viewing Output
- III Manipulating Data
- IV Describing Data
- V Score Distribution Assumptions
- VI Correlation and Prediction
- VII Comparing Means: The t Test
- VIII Comparing Means: ANOVA
- IX Nonparametric Procedures
- X Advanced ANOVA Techniques
- XI Analysis of Structure
- References
- Author Index
- Subject Index
- References
References
Published online by Cambridge University Press: 05 June 2012
- Frontmatter
- Contents
- Preface
- Acknowledgments
- I Introducing SAS Enterprise Guide
- II Performing Analyses and Viewing Output
- III Manipulating Data
- IV Describing Data
- V Score Distribution Assumptions
- VI Correlation and Prediction
- VII Comparing Means: The t Test
- VIII Comparing Means: ANOVA
- IX Nonparametric Procedures
- X Advanced ANOVA Techniques
- XI Analysis of Structure
- References
- Author Index
- Subject Index
- References
Summary
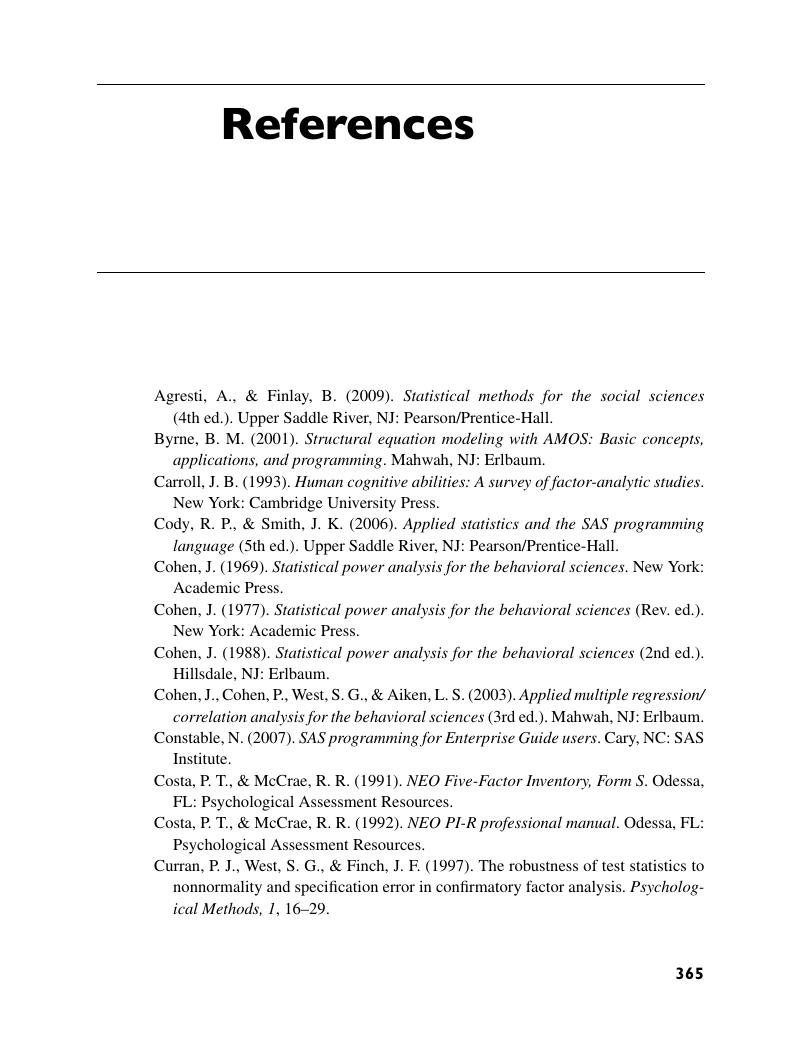
- Type
- Chapter
- Information
- Data Analysis Using SAS Enterprise Guide , pp. 365 - 370Publisher: Cambridge University PressPrint publication year: 2009