Impact Statement
Injury to the low back is common among workers, painful to individuals, and costly to society as a whole. Two risk factors associated with low-back injury are the cumulative low-back load (CLBL), and the peak low-back load (PLBL). In this work, we use simulation and a two-factor block study design to examine how much the risk of low-back injury is affected by an exoskeleton and improvements to lifting technique. Our simulations indicate that training alone can substantially reduce CLBL, but only modestly reduce PLBL. When both training and an assistive exoskeleton are used, our simulations indicate that both risk factors can be substantially reduced.
Introduction
Low-back pain (LBP) accounts for approximately 15.5% of worker absenteeism in industry across Europe and North America (Wynne-Jones et al., Reference Wynne-Jones, Cowen, Jordan, Uthman, Main, Glozier and Van Der Windt2014), while being globally the first ranked disorder for years lived with disability (Buchbinder et al., Reference Buchbinder, Blyth, March, Brooks, Woolf and Hoy2013). Assistive exoskeletons, and more specifically, back-support exoskeletons, are being developed for the prevention of LBP by alleviating low-back loads. There exists a variety of passive exoskeletons such as Laevo (Laevo, Reference Laevo2018; Hensel and Keil, Reference Hensel and Keil2019), Personal Lift Assist Device (PLAD) (Abdoli-E et al., Reference Abdoli-E, Agnew and Stevenson2006), SuitX (Kazerooni et al., Reference Kazerooni, Tung and Pillai2019), Moment Restoring Device (Wehner et al., Reference Wehner, Rempel and Kazerooni2010), and Bending Non-Demand Return (BNDR) (Ulrey and Fathallah, Reference Ulrey and Fathallah2013). There are a similar number of active exoskeletons such as the Robomate (Huysamen et al., Reference Huysamen, de Looze, Bosch, Ortiz, Toxiri and O’Sullivan2018; Toxiri et al., Reference Toxiri, Koopman, Lazzaroni, Ortiz, Power, de Looze, O’Sullivan and Caldwell2018), Wearable Stop-Assist Device (WSAD) (Luo and Yu, Reference Luo and Yu2013), and the Muscle Suit (Muramatsu and Kobayashi, Reference Muramatsu and Kobayashi2014). In many cases, these devices manage to lower joint torques and muscle activity (de Looze et al., Reference de Looze, Bosch, Krause, Stadler and O’Sullivan2016). Although many exoskeletons are focused at reducing the risk of low-back injury, it is not clear how effective these devices are in comparison to simply improving lifting technique.
Repetitive lifting and bending tasks contribute most to LBP (Coenen et al., Reference Coenen, Kingma, Boot, Twisk, Bongers and Van Dieën2013). Repetitive lifting causes the accumulation of microdamage to the tissue through cumulative low-back loads (CLBLs; Brereton and McGill, Reference Brereton and McGill1999). Apart from CLBL, instantaneous damage can be caused to the lower back by peak low-back loads (PLBLs). Both of these quantities are typically highest at the L5/S1 lumbosacral joint (Coenen et al., Reference Coenen, Kingma, Boot, Bongers and Van Dieën2014). Risk factors based on the L5/S1 extension moment (such as CLBL and PLBL) are both easy to calculate and capture the risk associated with many different specific injuries, since the loads applied to the ligaments, disks, vertebrae, and muscles of the back, scale with the L5/S1 extension moment (van Dieën, Reference van Dieën2005).
Even though there has been a lot of modeling and simulation work done to learn more about back injury (McGill and Norman, Reference McGill and Norman1987; de Zee et al., Reference de Zee, Hansen, Wong, Rasmussen and Simonsen2007; Christophy et al., Reference Christophy, Senan, Lotz and O’Reilly2012), much of this work is based on inverse-dynamics data taken from real people lifting, and therefore cannot predict how someone might use a novel exoskeleton. The few optimal control studies that have been done to predict new lifting motions (Xiang and Arefeen, Reference Xiang and Arefeen2020) do not combine human and exoskeleton models. The limited amount of work that includes an exoskeleton (Millard et al., Reference Millard, Sreenivasa and Mombaur2017; Harant et al., Reference Harant, Millard, Šarabon and Mombaur2019), however, does not consider the effects of training, nor risk factors related to low-back injury. In addition, no simulation work has been found that examines the effect of lifting technique on the risk of low-back injury.
In this work, we employ optimal control and multibody dynamics to model, simulate, and predict the movements and forces needed for a person to lift a 10-kg box from the floor. Using motion capture data of an experimental participant lifting a 10-kg box from the floor, we recreate the motion using a least-squares (LSQ) fitting approach. We then add the exoskeleton model and re-evaluate the LSQ problem in order to quantify the effect of adding an exoskeleton without changing the original motion. Next, we examine how much CLBL and PLBL can be reduced through improved lifting technique, with the use of an exoskeleton, and lastly with the use of an exoskeleton plus improved lifting technique. In this study, we use a movement between a stoop and a squat (stoop–squat), since this technique is most often used when picking up objects from the ground (Burgess-Limerick et al., Reference Burgess-Limerick, Abernethy, Neal and Kippers1995). We extend our previous work (Marinou and Mombaur, Reference Marinou, Mombaur, Moreno, Masood, Schneider, Maufroy and Pons2020) by simulating the effects of two different conditions in our simulations: lifting using experimentally measured technique versus lifting with optimal lifting technique; and lifting without any aids versus lifting with the assistance of an exoskeleton. We evaluate three different optimal lifting techniques that minimize three different risk factors for lifting: (a) CLBL, (b) PLBL, and (c) a weighted sum of both CLBL and PLBL (HYB). We evaluate the effects of assistance by simulating the lift using two different models: a model of our human participant, and a model of our human participant wearing the SPEXOR exoskeleton. We hypothesize that both the lifting technique alone and with the exoskeleton will reduce CLBL and PLBL, but that the exoskeleton will provide the greatest reduction in injury risk.
Methods
We simulate eight different stoop–squat lifts of a 10-kg box in the sagittal plane using a planar multibody model. We create a reference solution using an LSQ fitting problem (Figure 1b), where we track the motion of one participant from the recorded motion capture data. To examine how much an exoskeleton reduces the risk of injury using the same lifting technique, we solve the same LSQ fitting problem but using a model that includes the exoskeleton. The remaining six of the lifts are completely synthesized and rely on no experimental data (Figure 1a). To examine the effect of lifting technique, we create three different cost functions: one for minimizing CLBL, one for minimizing PLBL, and lastly a hybrid function minimizing both CLBL and PLBL. The following sections describe our modeling procedure, the optimal control problem (OCP) formulation, and the evaluation of the cost functions employed.

Figure 1. Two optimization methods: (a) prediction through optimization of human-only (HO) and human-with-exoskeleton (HwE) stoop–squat lifts and (b) dynamic reconstruction of human-recorded stoop–squat lifts for both HO and HwE optimal control problems based only on human capture data.
Experimental Data
A male participant of 76.5-kg mass and height of 1.69 m performed a stoop–squat lift of a 10-kg box. Kinematics of the body segments and the box were recorded by recording the position of markers on the participant and the box, using an OptoTrack system (Northern Digital Inc., Waterloo, Ontario, Canada). Ground reaction forces were collected using Kistler force plates (model 9260AA6 from Kistler, Winterthur, Switzerland). The experiment was conducted at the University of Primorska in Slovenia and approved by the national medical ethics committee of the Republic of Slovenia (0120-199/2016-2, KME 93/04/16) with written and informed consent from the participant. The data were collected for the human alone, without the aid of the exoskeleton.
Dynamics
We have modeled the human body in the sagittal plane using an 11-segment model with 13 degrees-of-freedom (DOFs), the exoskeleton with 6 segments and 8 DOFs, and the box with 1 segment and 3 DOFs. Accordingly, the generalized position vector contains 3 entries for the box, and 13 entries for the human model. When the exoskeleton is included, eight additional entries are added for the exoskeleton model. The geometry, masses, and inertias of the segments in the human model have been scaled using de Leva’s (Reference de Leva1996) anthropomorphic tables and the participant’s height and mass. The mass and geometry properties of the box and the exoskeleton have been set to match the physical box used in the experiment and the SPEXOR exoskeleton (Näf et al., Reference Näf, Koopman, Baltrusch, Rodriguez-Guerrero, Vanderborght and Lefeber2018).
The system is modeled as a constrained multibody system,

where
$ G $
is the Jacobian of the kinematic constraint equations

and
$ \mathbf{q} $
,
$ \dot{\mathbf{q}} $
, and
$ \ddot{\mathbf{q}} $
are the generalized vectors for position, velocity, and acceleration of the model’s segments.
$ M\left(\mathbf{q}\right) $
is the mass matrix of the system, and
$ c\Big(\mathbf{q} $
,
$ \dot{\mathbf{q}}\Big) $
is the vector of Coriolis and centripetal forces. Equation (2) describes the kinematic constraints, which consists of the coupling equations for the lumbar spine (Christophy et al., Reference Christophy, Senan, Lotz and O’Reilly2012), the contact constraints between the foot and the ground, the contact constraints between the hands and the box, and the constraints that attach the exoskeleton to the human body model. The entries contained in
$ G{\left(\mathbf{q}\right)}^T\lambda $
are the generalized forces imposed by these constraints, where
$ G\left(\mathbf{q}\right) $
is the Jacobian of the constraint equations
$ g\left(\mathbf{q}\right) $
with respect to
$ \mathbf{q} $
, and
$ \lambda $
is a vector of Lagrange multipliers. Finally,
$ \tau $
is the vector of generalized force applied to the system, which consists of the joint torques developed at the internal joints of the human model and the hip actuator of the exoskeleton (Figure 2).
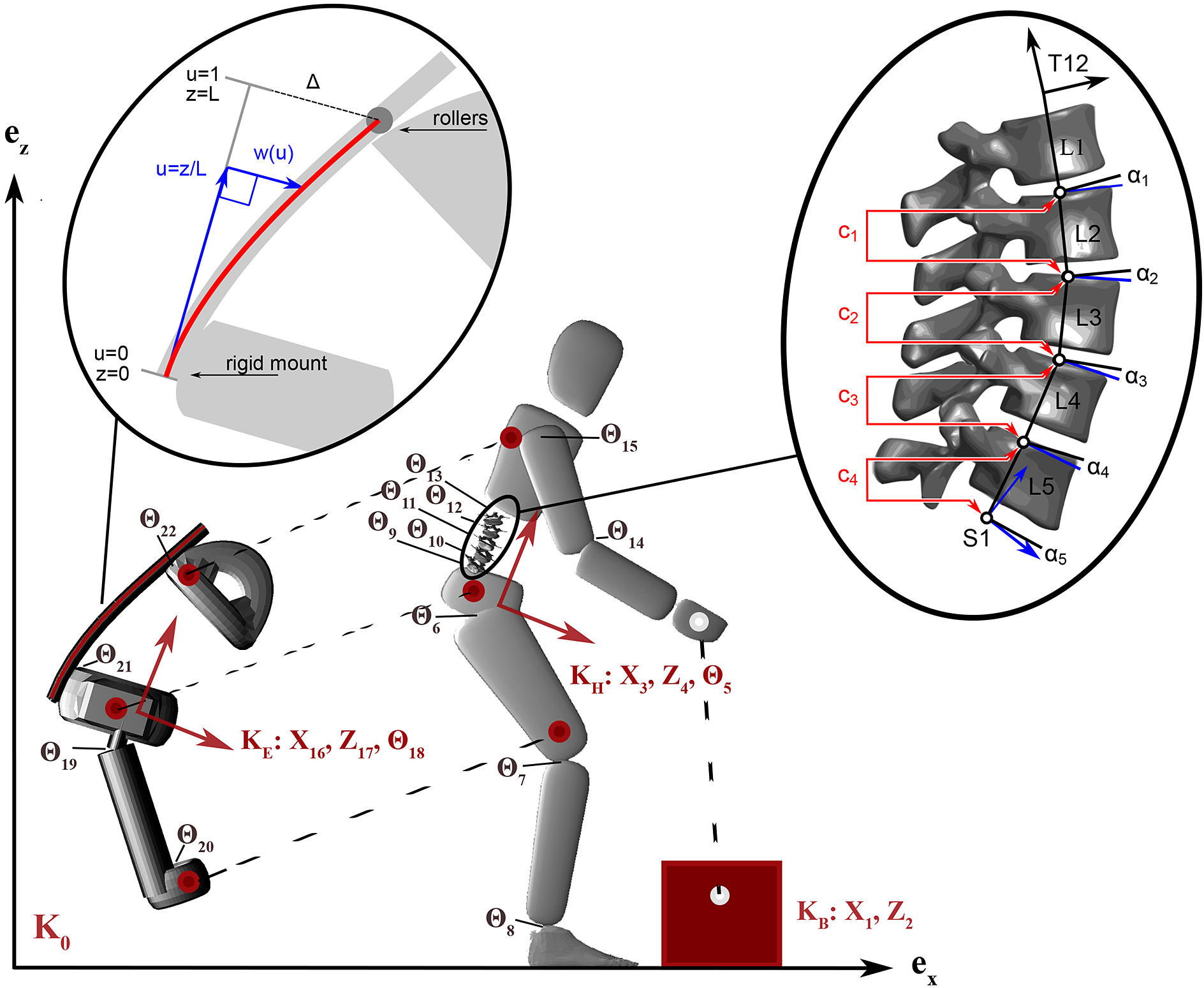
Figure 2. The human as an 11-segment, 13-DOF model and the attachment points to the 6-segment, 8-DOF exoskeleton. Dashed lines indicate the kinematic constraints between the exoskeleton and the human, as well as the human and the box. The feet are constrained to the ground throughout the motion, whereas the box is constrained to the ground until lifted by the human. The letter
$ K $
denotes a coordinate system where the subscripts
$ B $
,
$ H $
,
$ E $
, and
$ 0 $
correspond to the coordinate systems of the box, human, exoskeleton, and global reference frames, respectively. The planar positions are indicated with
$ x $
and
$ z $
and angles by
$ \Theta $
. A close-up of the lumbar spine model depicts the L1–L5 lumbar vertebrae and the four constraint equations that couple the movements of the joints. Each disk is approximated as a spherical joint located at the center of rotation identified by Pearcy and Bogduk (Reference Pearcy and Bogduk1988) from radiographic data. We have scaled the center of rotation of each vertebrae to fit the high-resolution meshes of the lumbar vertebrae of Mitsuhashi et al. (Reference Mitsuhashi, Fujieda, Tamura, Kawamoto, Takagi and Okubo2009).
In describing the interaction between the human and the exoskeleton, we simulate the exoskeleton as an external rigid body which is attached to the human through eight kinematic constraints defined at three attachment points: the thigh, pelvis, and upper trunk. The thigh and upper trunk modules of the exoskeleton are attached to the human model using weld constraints. A point constraint is used to attach the pelvis module of the exoskeleton to the pelvis, which permits rotation between these two bodies. Weld constraints are applied between the human feet and the ground, as well as the hands and the box during the contact and lifting phases. To simulate this constrained system forward in time, we use the Rigid Body Dynamics Library of Felis (Reference Felis2017).
Lumbar Spine Model
We have included an articulated and coupled model of the lumbar spine similar to Christophy et al. (Reference Christophy, Senan, Lotz and O’Reilly2012) to ensure that the bending movements of the model are as accurate as possible. In this model, the lumbar spine is described as five vertebrae attached serially using revolute joints. The revolute joints of the lumbar back are located at the average center of rotation of each vertebra as reported by Pearcy and Bogduk (Reference Pearcy and Bogduk1988). We have fit the data of Pearcy and Bogduk (Reference Pearcy and Bogduk1988) to the high-resolution vertebral meshes of Mitsuhashi et al. (Reference Mitsuhashi, Fujieda, Tamura, Kawamoto, Takagi and Okubo2009), and scaled the model to fit our participant. All internal joints of the human model are torque-driven.
Although the lumbar spine has five joints, we have coupled those joints with four constraint equations, so that the entire lumbar spine has only one DOF in the sagittal plane. The constraint equations have been formulated, so that the resulting coordinated motion is consistent with the coordinated bending of the lower back as measured by Wong et al. (Reference Wong, Luk, Leong, Wong and Wong2006). Wong et al. (Reference Wong, Luk, Leong, Wong and Wong2006) observed that the flexion of each joint,
$ {\alpha}_i $
, scales linearly with the total lumbar flexion angle,
$ {\alpha}_L\hskip2pt =\hskip2pt {\sum}_1^{i\hskip2pt =\hskip2pt 5}{\alpha}_i $
, such that
$ {\alpha}_i\hskip2pt =\hskip2pt {n}_i{\alpha}_L $
, where
$ {\alpha}_5 $
corresponds to the angle from S1 to L5, and
$ {\alpha}_4 $
corresponds to the angle from L5 to L4, and so forth. The coefficients
$ {n}_5,\dots, {n}_1 $
that best fit the 30 participants in Wong et al.’s (Reference Wong, Luk, Leong, Wong and Wong2006) study are
$ 0.255 $
,
$ 0.231 $
,
$ 0.204 $
,
$ 0.185 $
, and
$ 0.125 $
. Similar to Christophy et al. (Reference Christophy, Senan, Lotz and O’Reilly2012), we use the linear relationship between
$ {\alpha}_i $
and
$ {\alpha}_L $
to form the velocity-level constraint

between neighboring pairs of joints (Figure 2). Prior to simulation, each lumbar joint angle is biased, so that a lumbar flexion angle of zero,
$ {\alpha}_L\hskip2pt =\hskip2pt 0 $
, poses the lumbar spine to match the resting position (as shown in Figure 2) of the participant from Mitsuhashi et al. (Reference Mitsuhashi, Fujieda, Tamura, Kawamoto, Takagi and Okubo2009). In this case, the bias angles are
$ {2.1}^{\circ } $
,
$ {8.8}^{\circ } $
,
$ {10.6}^{\circ } $
,
$ {12.9}^{\circ } $
, and
$ {11.3}^{\circ } $
of extension for the joints from the L5/S1 joint to the L1/L2 joint, respectively. As with other kinematic constraints in this model, index reduction is used to transform the original set of differential algebraic equations of Index 3 to a system of differential algebraic equations of Index 1. During the simulation, the constraint error is reduced using the stabilization of Baumgarte (Reference Baumgarte1972).
Exoskeleton Model
We model the SPEXOR exoskeleton using an eight-DOF mechanism composed of six segments and a total mass of 9.12 kg. Unique to this exoskeleton are three carbon fiber rods (4.7 mm in diameter, with a Young’s modulus of 166 GPa) producing counter torques about the lumbar in order to support lifting motions. In addition, a hydraulic actuator (with a maximum output torque of 25 Nm) is placed at the exoskeleton hip joints, which feature a misalignment compensation mechanism (Figure 2). The exoskeleton further includes a trunk and pelvis module that are connected by carbon fiber beams, and two thigh modules that are connected to the pelvis interface by a rigid metal rod on each side. The beams are rigidly fixed to the pelvis module and pass through the torso module via a series of rollers.
We model the path traced by the slender beams using a cubic spline. As has been shown by Holladay (Reference Holladay1957), a cubic spline traces a path that minimizes total curvature, which is the same path traced by a slender elastic beam. At every instant in time, a cubic spline
$ w(u) $
is fitted to match the end conditions imposed by the rigid pelvis mount and the rollers (Figure 2). We describe the spline in normalized coordinates

along the undeformed path of the beam, and by deflections

perpendicular to
$ u $
. Here,
$ L $
is the distance between the pelvis mount and the rollers projected onto the beam’s axis that is fixed to the pelvis module. The coefficients in Equation (5) are evaluated using the boundary conditions imposed by the rigid pelvis mount

and the boundary conditions imposed by the rollers on the torso module where the beam must deflect by
$ \Delta $

and

since the rollers cannot apply a reaction moment. The moments and shear forces the slender bent beam applies to the pelvis (
$ z\hskip2pt =\hskip2pt 0 $
) and torso (
$ z\hskip2pt =\hskip2pt L $
) modules are evaluated using the spline
$ w(u) $
and an Euler–Bernoulli beam model where

and

OCP Formulation
We divide the task of lifting a box from the floor into three phases for both human-only (HO) and human-with-exoskeleton (HwE) OCPs (Figure 3): first, the model moved from a standing phase to touching the box; second, the model applies force to the box until the full weight of the box is supported; finally, the model lifts the box and stands back up again. We use a series of constraints to ensure that contact forces are physically realistic and the beginning and end poses are comparable. The forces in the global
$ {\mathbf{e}}_{\mathrm{Z}} $
direction are constrained to be positive, while friction forces must be within the friction cone. Similarly, tangential forces between the hands and the box are also constrained to be within the friction cone, as would be case if the lift is being performed with an open grip. Finally, we constrain the model to begin and end the lift at rest and in the same starting and ending pose as the human participant.

Figure 3. We formulate lifting as a three-phase problem: standing to touching the box, touching the box to lifting the box, and finally lifting the box to standing back up again with the box. Image sequence taken from the LSQ HwE optimal control problem.
For the simulations that include an exoskeleton, we have additional constraints to limit the interaction forces between the human and the exoskeleton. We solve both problems using a direct multiple-shooting algorithm implemented in MUSCOD-II (Bock and Plitt, Reference Bock and Plitt1985; Leineweber et al., Reference Leineweber, Bauer, Bock and Schlöder2003).
In this work, we formulate a multiphase OCP that minimizes the Lagrange term

which includes the control vector
$ u(t) $
which is composed of the joint torques of the human model and the actuator control signal of the exoskeleton, and the state variables vector
$ x(t) $
which contains the positions and velocities of the multibody system segments. The vector
$ p $
stands for physical parameters, such as exoskeleton, and
$ i $
iterates through the multiple phases of the problem through time t from
$ {t}_o $
to
$ {t}_N $
. The dynamics of the systems (Equations (1) and (2)) take the form of ordinary differential equations

that are limited by equality and inequality constraints


at specific time points throughout the separate phases. The time vector is broken up into N consecutive time intervals

LSQ quadratic fitting
To find solutions that mimic the human participant’s lifting technique, we solve an LSQ problem. For both HO and HwE formulations, the LSQ problem has two terms: a tracking term

and a term

to ensure that the exoskeleton is used during the HwE simulations. We include a small regularization on the exoskeleton’s motor torque, so that the exoskeleton is not unnecessarily used.
$ M $
denotes the number of shooting nodes for the given phase
$ i $
, and
$ {q}^H $
and
$ {q}^{REF} $
are the computed and tracked positional coordinates, respectively. The vector
$ {q}^{REF} $
is a function of time that comes from using inverse kinematics to pose the model, so that its virtual markers minimize the squared distance to the recorded positions of the markers on the participant. The vector
$ {\overline{\mathbf{u}}}^{\mathbf{ALL}} $
is the normalized human joint torques vector, and
$ {u}^{motor} $
is the control signal of the motor which is used as a regularization term.
$ {w}_1 $
and
$ {w}_2 $
have the values of
$ 0.02 $
and
$ 1x{10}^{-9} $
, respectively.
Synthesizing lifting techniques with a lower risk of injury
We synthesize three lifting motions that minimize the risk factors associated with low-back injury: CLBL, PLBL, and a weighted sum of both CLBL and PLBL. When analyzing experimental data, the CLBL is calculated as

as described by Coenen et al. (Reference Coenen, Kingma, Boot, Twisk, Bongers and Van Dieën2013). Although Equation (18) is perfectly suited for analysis, it is ill-suited as a cost function for an OCP, because it is signed: it is possible for the model to produce a flexion torque and thus reducing the CLBL after lifting. Instead, we integrate the normalized torque of the lower back squared

Squaring the lumbar torques has been suggested by Coenen et al. (Reference Coenen, Kingma, Boot, Bongers and Van Dieën2012) to put a bit more emphasis of the CLBL result on higher loads. We have also normalized these values according to the maximum torque output of these joints (
$ {\tau}_{max} $
), so that their value remains between 0 and 1, to avoid numerical scaling problems that occur with either very big or very small numbers. Since the motion of the five vertebrae are coupled by four constraint equations, it is possible to drive the lumbar spine using only a subset of the joints, but this is physiologically unrealistic. By summing across the moments developed by each joint, we ensure that the final load distribution does not favor one vertebra at the expense of the other joints.
When analyzing experimental data, the PLBL is evaluated as

over a time duration of interest (Coenen et al., Reference Coenen, Kingma, Boot, Bongers and Van Dieën2012). Both Coenen et al. and Jäger et al. (Reference Jäger, Jordan, Theilmeier, Wortmann, Kuhn, Nienhaus and Luttmann2013) have suggested instead to evaluate PLBL risk by integrating the L5/S1 moment over time but raised to a higher power to further penalize peak values. To find motions that reduce PLBL risk, we minimize the sum of normalized lumbar torques

raised to the power of 4.
Finally, we consider a third cost function which is simply the sum of these two cost functions

in hopes of finding a motion that is able to reduce both risk factors simultaneously. In all cost functions, we include a small regularization term that includes all joint torques

to ensure that the result is a minima (Nagarajan and Kolter, Reference Nagarajan and Kolter2017). The weight factor
$ w $
takes the value of
$ {10}^{-3} $
.
Evaluation
We use the following procedure to evaluate our results:
-
• We compare the peak lumbar load and maximum lumbar flexion angles of our participant to the values reported in the literature (Kingma et al., Reference Kingma, Bosch, Bruins and van Dieën2004) where 10 participants performed a stoop–squat lift with a 10.5-kg box.
-
• We report CLBL and PLBL which we have calculated according to Coenen et al. (Reference Coenen, Kingma, Boot, Twisk, Bongers and Van Dieën2013), for each of the prediction simulations and dynamic reconstruction problems.
-
• We report the results for the four study blocks: HO with tracking (LSQ); HO with improved lifting technique; HwE with tracking; and HwE with improved lifting technique.
Results
Simulated improvements to lifting technique were able to reduce the CLBL and PLBL risk factors for the unassisted lifts (Table 1 and Figures 4, 5a, and 6a). The experimental participant performed a stoop–squat lift with a peak torque of 210 Nm and a cumulative torque of 243 Nms (Table 1 and Figure 5) according to the dynamic reconstruction, close to the peak torque range reported by Kingma et al. (Reference Kingma, Bosch, Bruins and van Dieën2004) of 199 ± 12 Nm. These simulation results indicate that training can produce modest reductions in PLBL, and larger reductions in CLBL (Table 1 and the gray bars in Figure 4).
Table 1. Cumulative low-back load (CLBL) and peak low-back load (PLBL) torques for both human-only and human-with-exoskeleton simulations for the four different optimal control problems (OCPs). The least-squares (LSQ) entry is the reference used for comparison purposes, by tracking the motions of the experimental participant

Note. CLBL and PLBL have different units, as CLBL is the torque as a function of time, whereas PLBL is the torque at a specific point in time. All values rounded to three significant figures.

Figure 4. Bar plot representing normalized lumbar torques about the L5/S1 joint from simulations of (a) cumulative low-back loads and (b) peak low-back loads. Shaded bars refer to HO and white bars to human-with-exoskeleton optimal control problems (OCPs). The torques are normalized according to the L5/S1 joint torque from the result of the HO tracking (LSQ) OCP. Numbers on top of bars indicate the values of the normalized torque, relative to the HO LSQ of value 1.

Figure 5. Models of (a) human only and (b) human with exoskeleton at the moment of lifting the box for all objective functions. The shaded regions in the background resemble a stacked bar plot, indicating the time (seconds) of box liftoff with the respective peak torques (Newton meters) and lumbar flexion angles (degrees) achieved at the point of lifting the box, for every model separately, with all the values indicated in the respective boxes.

Figure 6. L5/S1 torques for (a) human only (HO) and (b) human with exoskeleton as calculated for the biomechanic metrics of cumulative low-back load and peak low-back load. The shaded region in the HO plot reports the values measured in literature (Kingma et al., Reference Kingma, Bosch, Bruins and van Dieën2004) for net lumbar torque. The phases of the minimization problems were scaled according to the experimental phases for easier graphical comparison.
Larger reductions in both CLBL and PLBL can be observed in the HwE OCPs. While it was not possible to reduce the PLBL in the HO simulation by more than 16.2%, the exoskeleton was able to achieve a 34.3% reduction (Table 1 and the white bars in Figure 4) when looking at the PLBLcost metric. In addition, the exoskeleton was able to reduce further both CLBL and PLBL in all cases when compared with the HO simulations (Figure 6). While the overall trend in torques for the various solutions appears to be similar in both unaided and aided motions, Phase 2 does not follow this pattern. The drop in torque during the HO simulations at the end of Phase 2 (Figure 6) happens as a result of a rapid counter movement: just prior to lifting the box, the model relaxes and drops the hips. During the exoskeleton assisted lifts, the model does not exploit this technique presumably, because the exoskeleton’s support makes this counter movement less effective.
The lumbar flexion angle closely tracked the participant data in the LSQ solution (Figure B1 in Appendix B) and was substantially reduced when the model was free to move. The dominant factor for the peak lumbar flexion angle appears to be the cost function, as there is little difference between the lumbar flexion angles of the HO and HwE lifts. Curiously, all the minimization cost functions for the HO condition result in a lower lumbar flexion angle in Phases 1 and 2 (Figure 7), whereas for the same phases in the HwE condition, the exoskeleton seems to be increasing the lumbar flexion angle of the human. In addition, we can see that the CLBLcost results in the fastest lifts (Table 2), since cumulative load accumulates over time.

Figure 7. Lumbar flexion angles for (a) human only (HO) and (b) human with exoskeleton as resulted from the tracked and optimized stoop–squat motion. The shaded region in the HO plot reports the values measured in literature (Kingma et al., Reference Kingma, Bosch, Bruins and van Dieën2004) for net flexion angle. The phases of the minimization problems were scaled according to the experimental phases for easier graphical comparison.
Table 2. Phase durations (in seconds) for all three phases for all optimal control problem (OCP) formulations

Abbreviations. CLBL, cumulative low-back load; LSQ, least squares; PLBL, peak low-back load.
Discussion
The risk of low-back injury can be reduced by improving lifting technique and by using an exoskeleton (Toxiri et al., Reference Toxiri, Näf, Lazzaroni, Fernández, Sposito, Poliero, Monica, Anastasi, Caldwell and Ortiz2019). Two of the biomechanic metrics that have been associated with the risk of injury to the lower back are CLBL and PLBL. In this study, we used simulation to examine how effective training, and an exoskeleton, are at reducing the CLBL and PLBL during a stoop–squat lift of a 10-kg box. We then compare the contributions of the improved technique alone, and with the exoskeleton assistance, to the HO reference motion that we have reconstructed from motion capture data. The cost functions we use do not only change the motion of the human in order to decrease the risk of injury, but they affect the way the exoskeleton supports the human as well, in order to help decrease the risk of injury.
We have had to make simplifications to our model, because the human body, the exoskeleton, and the interaction of the two are complex. We have ignored the effects of muscles and muscle dynamics. This simplification is reasonable under the assumption that the human is performing a submaximal lift and is not at the limits of the participant’s flexibility, force, or speed. By creating three hypothetical lifting techniques using CLBLcost , PLBLcost , and HYBcost , we assume that humans can actually be trained to minimize these risk factors. We have also included limits on the interaction forces (Figure A1 in Appendix A) under the assumption that the exoskeleton is comfortable to use if these limits are met. While we have imposed human–exoskeleton interaction force limits, it remains unclear how interaction forces will affect the motion of the human.
The HO L5/S1 peak moments compare well (176–210 Nm) to the ones reported by Kingma et al. (Reference Kingma, Bosch, Bruins and van Dieën2004; 187–211 Nm; Figure 6a), as well as the peak lumbar flexion angles (Figure 7). In a study by Lavender et al. (Reference Lorenz, Lavender and Andersson2002), 265 participants were trained to perform a lift of a 12–13-kg box to improve their lifting technique. The peak moment varied from 225 to 178 Nm throughout the training period. In relation to our HO simulation of lowering the PLBL, we can see that this reduction is similar to the reduction in PLBL in our PLBLcost HO simulations, as the HO reference motion has a PLBL of 210 Nm and PLBLcost HO OCP minimizes this to 176 Nm. De Looze et al. (Reference de Looze, Bosch, Krause, Stadler and O’Sullivan2016) report similar peak moment reductions of 19.5 and 15% when participants use the PLAD (Abdoli-E et al., Reference Abdoli-E, Agnew and Stevenson2006) and BNDR (Wehner et al., Reference Wehner, Rempel and Kazerooni2010) exoskeletons. In contrast, Koopman et al. (Reference Koopman, Kingma, de Looze and van Dieën2020a) report a 5–10% reduction in peak moments when using the Laevo exoskeleton. In the context of active back-support exoskeletons, the XoTrunk exoskeleton (Lazzaroni et al., Reference Lazzaroni, Tabasi, Toxiri, Caldwell, De Momi, van Dijk, de Looze, Kingma, van Dieën and Ortiz2020) achieved a peak moment reduction of 17%. The passive SPEXOR exoskeleton produced a maximum of 23 ± 3% reduction in the peak L5/S1 extension moment (Koopman et al., Reference Koopman, Näf, Baltrusch, Kingma, Rodriguez-Guerrero, Babič, de Looze and van Dieën2020b) for 10 subjects using different lifting techniques, including exoskeleton assistance. Using our simulations based on the active SPEXOR exoskeleton, we predict a reduction of 21.4% (according to the CLBL cost function) to 34.3% (according to the PLBL cost function).
Conclusion
Our simulations indicate that improving the lifting technique alone can reduce the CLBL from 243 to 130 Nms, but only modestly reduces the PLBL from 210 to 176 Nm. When the SPEXOR exoskeleton is used without any alteration to the person’s lifting technique, the CLBL is reduced from 243 to 217 Nms, while the PLBL is reduced from 210 to 177 Nm. The biggest reductions in both CLBL and PLBL are realized when both the SPEXOR exoskeleton and improved lifting technique are used together where the CLBL is reduced to 121 Nms and the PLBL to 138 Nm. While all of the three cost functions we examined reduced the risk factors for back injury, the HYB cost function offered the most balanced reduction of both the CLBL (38.3%) and the PLBL (28.6%). Thus, improvements to lifting technique alone may be a suitable intervention for people who infrequently lift light loads. However, in more demanding tasks, an exoskeleton should be considered, since it is more effective than the technique alone at reducing both the cumulative and peak loads experienced by the low back.
Acknowledgments
We would like to thank Žiga Kozinc and Borut Fonda at the University of Primorska for their help collecting the experimental data used in this work. We also thank the Simulation and Optimization Research Group of the IWR at Heidelberg University for allowing us to work with the optimal control code MUSCOD-II.
Ethical Standards
The authors assert that all procedures contributing to this work comply with the ethical standards of the relevant national and institutional committees on human experimentation and with the Helsinki Declaration of 1975, as revised in 2008. The authors assert that the experimental recordings were approved by the national medical ethics committee of the Republic of Slovenia (0120-199/2016-2, KME 93/04/16).
Data Availability Statement
The data that support the findings of this study are available from the corresponding author, G.M., upon reasonable request.
Competing Interests
The authors declare no competing interests exist.
Funding Statement
Financial support provided by the European Commission within the H2020 project SPEXOR (Project ID 687662), from the project HeiAge funded by the Carl Zeiss Foundation (Germany), and from the Canada Excellence Research Chair Tri-Agency program is gratefully acknowledged.
Authorship Contributions
G.M. and M.M. undertook the research and the manuscript preparation. N.S. designed and undertook the experimental work. K.M. conceived of the research idea and plan, obtained funding for the project, and assisted in the work, and in the preparation of the manuscript.
A. Interaction Forces

Figure A1. Interaction forces for human-with-exoskeleton prediction optimal control problems. Values shown correspond to normal and shear forces, and for moments about the three attachment points: pelvis, torso, and thigh. Interaction force limits are also reported.
B. LSQ Validation

Figure B1. Lumbar flexion angles for the inverse-kinematics (IK) solution obtained from motion capture versus human-only (HO) least-squares optimal control problem (OCP) and human-with-exoskeleton (HwE) solutions from our OCPs. This serves as a validation of our dynamic reconstruction procedure, so as to show the close correlation between our solution and the IK data from the motion capture.