Introduction
African forest elephants Loxodonta cyclotis are under threat. According to data analysed by the CITES Elephant Trade Information System and Monitoring the Illegal Killing of Elephants programmes, the illegal trade in ivory is escalating (Milliken et al., Reference Milliken, Burn and Sangalakula2009) and offtake exceeds calculated sustainable levels (CITES, 2015). 2013 was the worst year on record for ivory seizures, with almost 50 tons seized (Vira et al., Reference Vira, Ewing and Miller2014). Central Africa remains the sub-region with the highest poaching pressure on the continent (CITES, 2015). Although forest elephants are taxonomically and functionally unique, IUCN recognizes only one species of African elephant Loxodonta africana, which is categorized as Vulnerable on the IUCN Red List (Blanc, Reference Blanc2008). However, there is significant geographical variation in the level of threat, and based on a regional assessment the Central African forest elephant is Endangered (Blanc et al., Reference Blanc, Barnes, Craig, Dublin, Thouless, Douglas-Hamilton and Hart2007).
Population status and distribution assessments are required to set goals and measure the effectiveness of management actions (Blanc et al., Reference Blanc, Barnes, Craig, Dublin, Thouless, Douglas-Hamilton and Hart2007). Several such assessments of forest elephants have been carried out (Schuttler et al., Reference Schuttler, Blake and Eggert2012; de Boer et al., Reference de Boer, Langevelde, Prins, de Ruiter, Blanc and Vis2013; Maisels et al., Reference Maisels, Strindberg, Blake, Wittemyer, Hart and Williamson2013); however, given their cryptic nature, large range within dense forest habitat, and low encounter rates, monitoring the distribution and trends of forest elephants remains a challenge (Hedges, Reference Hedges2012). Transect surveys of dung density are the most widely used method (Barnes, Reference Barnes2001; Blake et al., Reference Blake, Strindberg, Boudjan, Makombo, Bila-Isia and Ilambu2007) but this is arduous and has been applied to only a relatively small part of the species’ range (Boafo et al., Reference Boafo, Manford, Barnes, Herma, Danquah, Awo and Dubiure2009).
Given these constraints, the scale of decline of forest elephants in Central Africa has been difficult to quantify, and this lack of information is a key concern for their conservation (Karanth et al., Reference Karanth, Nichols, Seidensticker, Dinerstein, Smith and McDougal2003; Blake & Hedges, Reference Blake and Hedges2004; Sutherland et al., Reference Sutherland, Pullin, Dolman and Knight2004; Blake, Reference Blake2005; Blanc et al., Reference Blanc, Barnes, Craig, Dublin, Thouless, Douglas-Hamilton and Hart2007). With 51% of Cameroon's potential elephant range unmonitored, it is vital that the country's forests are surveyed to address this knowledge gap, resolve uncertainty and guide conservation action.
There is potential for using local ecological knowledge to assess the status and distribution of forest elephants by gathering data rapidly over areas and timescales, which would not be feasible using conventional surveys (Danielsen et al., Reference Danielsen, Burgess and Balmford2005; Jones et al., Reference Jones, Andriamarovololona, Hockley, Gibbons and Milner-Gulland2008; Mohd-Azlan et al., Reference Mohd-Azlan, Belant and Meijaard2013; Turvey et al., Reference Turvey, Risley, Moore, Barrett, Yujiang and Xiujiang2013, Reference Turvey, Trung, Quyet, Nhu, Thoai and Tuan2015; Service et al., Reference Service, Adams, Artelle, Paquet, Grant and Darimont2014). As local people often frequent areas that are relatively inaccessible (Service et al., Reference Service, Adams, Artelle, Paquet, Grant and Darimont2014), the likelihood of obtaining species encounter records can be increased substantially by questioning local people, and this is especially useful for wide-ranging and elusive species (Turvey et al., Reference Turvey, Risley, Moore, Barrett, Yujiang and Xiujiang2013, Reference Belant, Hofer and Wilting2015; Service et al., Reference Service, Adams, Artelle, Paquet, Grant and Darimont2014). Local ecological knowledge can also facilitate a better understanding of threats to species (Abram et al., Reference Abram, Meijaard, Wells, Ancrenaz, Pellier and Runting2015), resulting in faster decision-making (Danielsen et al., Reference Danielsen, Burgess, Jensen and Pirhofer-Walzl2010) through increased dialogue (Belant et al., Reference Belant, Hofer and Wilting2013; Mohd-Azlan, Reference Mohd-Azlan, Belant and Meijaard2013).
Data collected from local knowledge are comparable with those collected using conventional methods (Danielsen et al., Reference Danielsen, Burgess and Balmford2005; Jones et al., Reference Jones, Andriamarovololona, Hockley, Gibbons and Milner-Gulland2008; Meijaard et al., Reference Meijaard, Mengersen, Buchori, Nurcahyo, Ancrenaz and Wich2011; Turvey et al., Reference Turvey, Risley, Moore, Barrett, Yujiang and Xiujiang2013; Pan et al., Reference Pan, Cunningham and Milner-Gulland2015; Parry & Peres, Reference Parry and Peres2015). All methods are susceptible to biases and uncertainties, and it is important to understand these biases to control for them (Danielsen et al., Reference Danielsen, Balete, Poulsen, Enghoff, Nozawa and Jensen2000; Jones et al., Reference Jones, Andriamarovololona, Hockley, Gibbons and Milner-Gulland2008). Observer and biophysical variables are a concern for most conventional population monitoring methods (Buckland et al., Reference Buckland, Anderson, Burnham, Laake, Thomas and Borchers2001; Sethi et al., Reference Sethi, Costello, Fisher, Hanemann and Karp2005; Nuno et al., Reference Nuno, Bunnefeld and Milner-Gulland2013). Characteristics of observers, such as age (Turvey et al., Reference Turvey, Barrett, Yujiang, Lei, Xinqiao and Xianyan2010) and experience (Cerqueira et al., Reference Cerqueira, Cohn-Haft, Vargas, Nader, Andretti and Costa2013), can influence ability to detect a species accurately. Furthermore, respondent biases, for example driven by social norms, can cause deception or unconscious distortion of responses (Moller et al., Reference Moller, Berkes, O'Brian Lyver and Kislalioglu2004). Fishers may deliberately overestimate their catch (Lunn & Dearden, Reference Lunn and Dearden2006), and local people who are adept at finding a species may overestimate its population size if it is considered to be common (Moller et al., Reference Moller, Berkes, O'Brian Lyver and Kislalioglu2004).
Heterogeneous habitat type (Tracey et al., Reference Tracey, Fleming and Melville2005), survey time (Cerqueira et al., Reference Cerqueira, Cohn-Haft, Vargas, Nader, Andretti and Costa2013), seasonality (Blanc et al., Reference Blanc, Barnes, Craig, Dublin, Thouless, Douglas-Hamilton and Hart2007), or variations in animal abundance (Royle & Nichols, Reference Royle and Nichols2003) can influence the effectiveness of population survey methods by affecting species detectability along gradients that may also influence abundance (Sutherland, Reference Sutherland2006). Observer and biophysical variables must therefore be controlled to reach an unbiased estimate of species distribution and relative abundance.
Occupancy indices are widely used for large-scale monitoring programmes because they are relatively inexpensive and easy to implement compared to estimates of absolute abundance (Royle & Nichols, Reference Royle and Nichols2003; Joseph et al., Reference Joseph, Field, Wilcox and Possingham2006). Occupancy indices also benefit from being able to control for uncertainties associated with detectability, providing unbiased estimates of the likelihood of species presence in time and space (MacKenzie et al., Reference MacKenzie, Nichols, Royle, Pollock, Bailey and Hines2006). Occupancy is an estimate of the probability that a species occupies, or uses, a particular sample unit during a specified period of time during which the occupancy state is assumed to be static (Bailey et al., Reference Bailey, Simons and Pollock2004). Using the maximum likelihood occupancy model both detectability and occupancy can be estimated in a single-model framework by building a detection history (MacKenzie et al., Reference MacKenzie, Nichols, Lachman, Droege, Royle and Langtimm2002), which potentially includes covariates of occupancy and detectability within the framework (Wintle et al., Reference Wintle, Walshe, Parris and McCarthy2012).
Surveys of local people have been combined with occupancy analysis to rapidly assess the status of multiple species over time (Pillay et al., Reference Pillay, Johnsingh, Raghunath and Madhusudan2011; D'Souza et al., Reference D'Souza, Patankar, Arthur, Alcoverro and Kelkar2013) and at large spatial scales (Martinez Marti, Reference Martinez Marti2011; Puri et al., Reference Puri, Srivathsa, Karanth, Kumar and Karanth2015). Here we combine semi-structured interviews of timber industry employees across eastern Cameroon (Fig. 1) with occupancy analysis to assess large-scale distribution and trends in forest elephant populations over time. We focused on areas categorized as unknown by the IUCN African Elephant Database (IUCN/SSC, 2013), to obtain new information about the range of elephants in these areas (Fig. 1). Timber concessions are an important but under-researched habitat for elephants, comprising 60–80% of the eastern region (Bikié et al., Reference Bikié, Collomb, Djomo, Minnemeyer, Ngoufo and Nguiffo2000). We aimed to (1) assess the distribution and trends in forest elephant populations over 6 years across 30,000 km2 of eastern Cameroon using interview-based occupancy analysis, (2) assess the reliability and suitability of this method of rapid assessment in the context of forest elephants in Africa, and (3) make recommendations for conservation action in the study area.
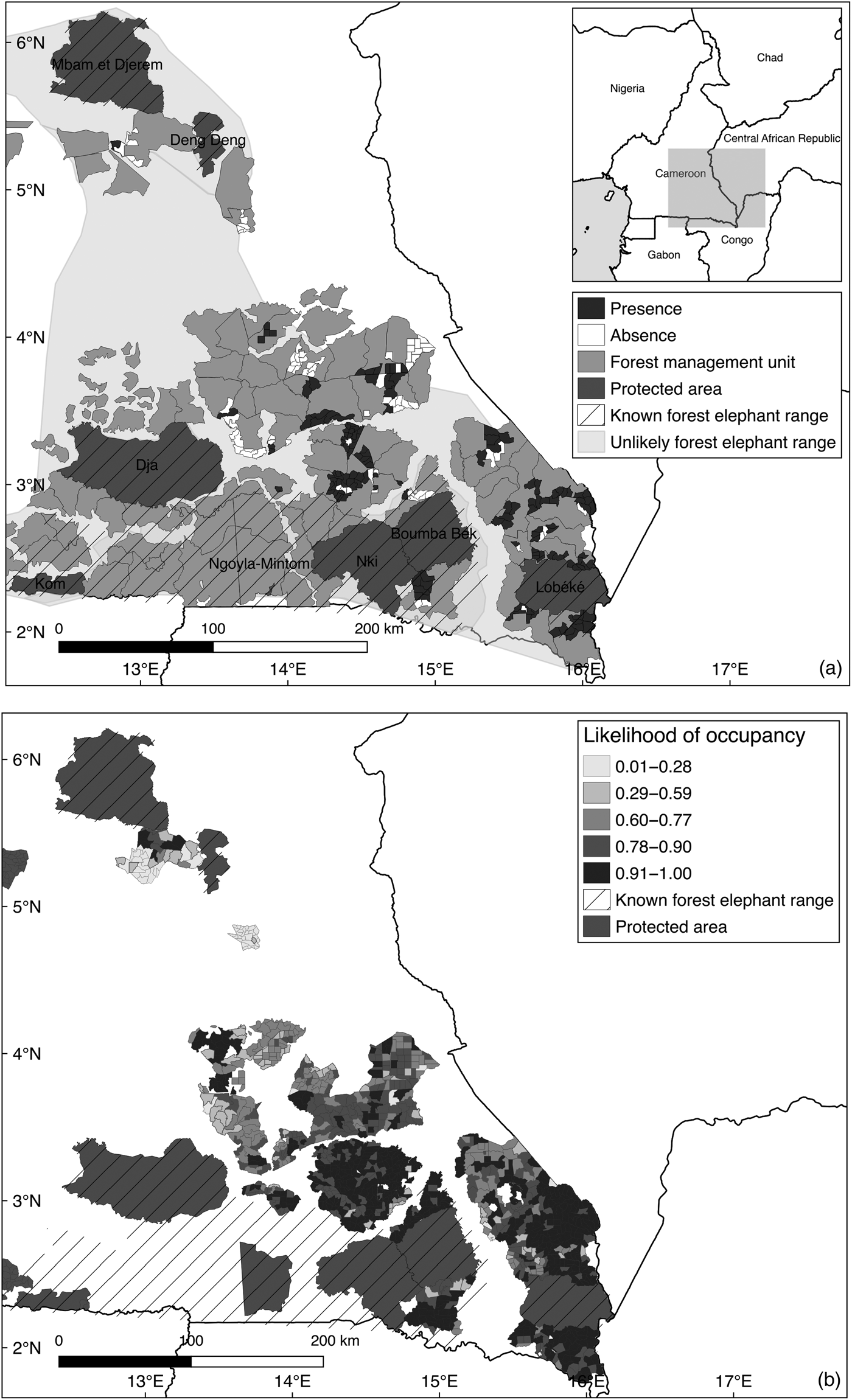
Fig. 1 (a) The study area in eastern Cameroon, including the known forest elephant range and areas deemed unlikely to be forest elephant range by IUCN/SSC (2013), and (b) likelihood of occupancy.
Methods
Timber concessions are divided into well-defined and demarcated forest management units (Cerutti et al., Reference Cerutti, Mbongo and Vandenhaute2016). Each forest management unit is divided into 30 annual allowable cuts, of which one can be exploited each year over 30 years. Sites were defined as annual allowable cuts, as these are familiar to respondents and are approximately equal in size (c. 5 km2). Maps of the forest management units were obtained from each concession manager prior to conducting interviews and each site's year of exploitation was clearly marked. Respondents stated in which site they had worked, in which year, and whether or not they had seen signs of elephants, and the maps helped them to recall fine-scale temporal and spatial data relevant to a particular site.
We conducted interviews with timber concession workers, local people and administrative authorities, using a targeted, opportunistic sampling strategy to select respondents. Although the external validity of data obtained through this strategy is low (Sapsford & Jupp, Reference Sapsford and Jupp1996), timber concession workers are a valuable source of knowledge, given the extent of concessions and their potential value as conservation land (Lamb et al., Reference Lamb, Erskine and Parrotta2005).
Interviews with administrative authorities were conducted to triangulate the data collected from timber concession workers and to obtain data on incidents of poaching. Chefs de poste of the Ministry of Forests and Wildlife, the governmental department responsible for the protection of forested areas and its biodiversity in Cameroon, should be aware of any reported poaching and can therefore give an alternative perspective on the research questions. Managers of the Department of Fauna, the managers of the eastern region departments and the chefs de poste from the Ministry of Forests and Wildlife were interviewed.
A simple questionnaire was administered to timber concession workers and authorities during 12 May–30 June 2013, to elicit their observations of elephants during 2008–2013 (Supplementary Material 1). The interviews were designed to be easily replicated and administered, whilst retaining standardization. A combination of closed and open-ended questions facilitated quantitative and qualitative analysis.
A shortened version of the semi-structured questionnaire used for timber concession workers was used to guide interviews with administrative authorities, retaining the sections on estimated abundance, distribution and threats, to collect qualitative data. Informal interviews (Newing et al., Reference Newing, Eagle, Puri and Watson2011), using open-ended questions, were conducted on several occasions to gather qualitative information on changes in the forest elephant population, and threats and attitudes towards elephants. The direction of the conversation was led by the interviewee, with the interviewer asking some questions to guide the conversation or probe an interesting point. Key details were recorded immediately after the conversation.
Efforts were made both in the design of the survey and the interview process to minimize or control for respondent bias. For each participating timber concession an initial meeting was held with the site manager, who helped us to identify which teams entered the forest on foot and did not operate machinery, and therefore had the best chance of spotting and correctly identifying signs of elephant presence. To select a subset of the most reliable workers from within the identified teams, a series of short questions were administered to focus groups to eliminate unsuitable respondents (Supplementary Fig. 2).
Selected respondents were interviewed individually to prevent audience effect bias. Questions were phrased neutrally to reduce deference effect bias (Newing et al., Reference Newing, Eagle, Puri and Watson2011) and respondents were asked to report on their own experience only. No specific reference to elephants was made at the start of the interview, to reduce order effect bias, and care was taken to use the interview funnel approach (Newing et al., Reference Newing, Eagle, Puri and Watson2011). The reliability of reported detections was validated by asking respondents to repeat both their detection and non-detection responses at the end of the interview and to describe the reported signs to ensure that the species had been identified correctly. If the respondent appeared to be unsure or gave different responses, the response was excluded from analysis.
Occupancy models were constructed, in which the response variable was whether the interviewee had observed elephants or their sign in a given annual allowable cut at any time during the study period. Given the rotational nature of exploitation within forest management units, repeat data from the same site over different years were not collected frequently enough to conduct multi-season occupancy analysis (MacKenzie et al., Reference MacKenzie, Nichols, Hines, Knutson and Franklin2003). Therefore, single-season occupancy analysis was carried out, by treating each site–year combination as a site in the detection matrix. Year could then be included as a covariate in the occupancy analysis to identify trends in detectability and occupancy over time, with a year considered to be the closure period, over which occupancy was assumed to be constant. The period 2008–2013 was chosen because the volume of reliable data was considerably less prior to 2008 (respondents were unsure when asked to repeat their responses at the end of the interview), and the number of respondents who had been employed prior to 2008 decreased. Following Martinez Marti (Reference Martinez Marti2011), individual interviewees were treated as effective repeat surveys for occupancy analysis. The number of respondents varied considerably between concessions. Although occupancy analysis accounts for missing data, sites with only one respondent were excluded from analysis and sites that did not meet the minimum of four replicates were treated with caution during analysis and discussion (MacKenzie et al., Reference MacKenzie, Nichols, Lachman, Droege, Royle and Langtimm2002).
Forest management unit group (central, south-eastern, south-western, northern; Supplementary Fig. 1) was included as a factor in analysis, facilitating comparisons of occupancy and detectability between groups. The groups are spatially distinct, separated by well-used roads and villages. Data on reported elephant tracks, broken branches, dung, carcasses and direct sightings were included in analysis to build a detection history for each site. Respondents who reported having seen a sign were asked to describe what they saw, as a means of verification. Only signs or direct sightings seen by the interviewee were included, as sightings related by others were considered to be hearsay and unreliable.
Given the easily identifiable signs of forest elephants and the controls put in place to ensure the reliability of the respondents selected, we felt false positives were unlikely, and therefore occupancy models that account for false positive detections were not deemed necessary for this study. Given the sample unit size relative to elephant home range, occupancy estimates cannot be considered to reflect the probability of long-term residence. Rather, we interpret occupancy as the proportion of area used (MacKenzie & Royle, Reference MacKenzie and Royle2005; Martinez Marti, Reference Martinez Marti2011).
Maps of site-level occupancy covariates were prepared using ArcGIS 10.0 (ESRI, Redlands, USA), and the respondent detectability covariates were obtained from each interview. Pairwise correlations were conducted to examine the independence of variables and eliminate any covariates that were too closely associated to be modelled together. Spearman's rank and Shapiro–Wilk tests were used to check for normal distribution of the continuous geographical variables. Spearman's correlation coefficient was used to test the relationship between the non-normally distributed variables. Pearson's correlation coefficient for parametric data was used for the remaining normally distributed variables. There was a strong positive correlation between age of respondent and number of trips made to the forest (ρ = 0.98) and between the number of years the respondent had worked in the concession and number of trips made to the forest (ρ = 0.91; Supplementary Table 1). The variable ‘number of trips’ was therefore not included in the models. There were no significant correlations between the covariates for the occupancy part of the model (Supplementary Table 2). Year of observation and forest management unit group were included as covariates for both occupancy and detectability to control for forest management unit-level variation in detectability (MacKenzie, Reference MacKenzie2006). Other covariates were included based on their hypothesized relationship with occupancy or detectability (Supplementary Table 3).
The Akaike Information Criterion (Burnham & Anderson, Reference Burnham and Anderson2002) was used to identify the best-fit models that account for detectability (ρ), keeping the global model for occupancy (ψ) (Supplementary Table 4). Using the best-fit model for ρ, occupancy was modelled to find the best-fit model for both ρ and ψ. The MacKenzie & Bailey (Reference MacKenzie and Bailey2004) goodness-of-fit bootstrap test was run to evaluate the best-fit model and to draw inferences to explain the effect of the covariates on ρ and ψ. All occupancy analysis was conducted in R v. 2.15.3 (R Development Core Team, 2017), using the package unmarked (Fiske & Chandler, Reference Fiske and Chandler2011).
Change in relative abundance over time was calculated based on the relationship between detection probability of individual elephants (r), site population size (N) and per-visit detection probability (P), proposed by Royle & Nichols (Reference Royle and Nichols2003):

Given detection probabilities estimated in years i = 1, 2,…6, year-specific populations are given by:

Assuming constant r, population size in year i relative to year 1 is therefore given by:

For interviews we followed the ethical guidelines of the Social Research Association (2003). The interview team (SB and MNB) spoke French to ensure accurate communication. All respondents were guaranteed confidentiality and anonymity, and free, prior, informed consent was obtained. Interviews were recorded if permission was given (>95% of respondents agreed). Otherwise, notes were taken and transcribed immediately post interview. Given the sensitive nature of the topic, no participants were asked if they had taken part in any illegal activities. We conducted a pilot study during 4–11 May 2013 to test the methodologies and sampling strategy, aiming to make any necessary adjustments to the approach and assess the reliability of the responses. The pilot study was conducted in a timber concession for which robust data on elephant presence were available.
Results
Of the 182 respondents interviewed, 161 were timber concession workers and 16 were administrative authorities; five informal interviews were conducted with researchers, poachers and hunting zone owners. Prior to analysis seven timber concession workers (4%) were deemed unreliable and their data were removed, leaving a total of 175 responses.
Survey responses suggested that interviewees were likely to be able to distinguish elephant signs in the field; 96% of respondents were raised in rural villages and 76% attributed their knowledge of animal signs to their fathers or upbringing. Respondents gave information about elephant observations at 342 sites within 34 forest management units. We interviewed 1–25 respondents per site (mean = 4.82). Fig. 1 shows the distribution of reported detections and non-detections, suggesting the forest elephant's range extends further north and east of the current known elephant range (IUCN/SSC, 2013). There is a higher proportion of sites with reported detections in the south-western and south-eastern forest management unit groups than in the central and northern groups.
The null model, assuming constant occupancy and detectability, estimated occupancy (Ψ, probability that a given site was used by elephants) to be 0.76, and detectability (P, probability that use of a forest management unit by elephants would be detected by a respondent) 0.58 (Supplementary Table 6).
The most parsimonious model with covariates that best described occupancy and detectability included, for detectability, the number of nights that the respondent camped in the forest when working, the number of years they had worked in the concession, the forest management unit group and year (Table 1). The occupancy variables included were the distance of the village from the centre of each annual allowable cut, the distance of the annual allowable cut from the nearest river and road, the elevation of the annual allowable cut, and the forest management unit group. A goodness-of-fit test found no significant lack of fit (P = 0.8).
Table 1 Summary of best fitting models with ΔAIC < 4, with Akaike Information Criterion (AIC), Akaike difference (ΔAIC), Akaike weight, standard error in probability of occupancy (Ψ) and standard error in probability of detection (p).

* p, probability of detection; C, nights camped; YW, years worked; G, forest management unit group; Y, year; Ψ, probability of occupancy; V, distance from village; Ri, distance from river; Ro, distance from road; E, elevation; S, slope; T, distance from town.
Occupancy was not strongly affected by any of the explanatory variables but, as expected (Supplementary Table 4), it was higher in areas further from villages and roads and closer to rivers. It did not vary significantly between forest management unit groups. Detectability, however, had a number of strong associations, including that those who camped for up to a week at a time in the forest were more likely to detect elephants than those who did not camp or who camped for longer, that detectability was much higher in the south-western and south-eastern forest management groups, and that there was a strong and consistent decline in detectability over time (Table 2). All sites had a similar forest environment; therefore, any spatial variation in the ability to detect the species cannot be explained by changes in habitat type or visibility. The same measures were put in place in each concession to assure the reliability and quality of respondents, and the competence of an individual to detect signs was controlled for in the model. Therefore, we do not attribute spatio-temporal changes in detectability to spatial or temporal variations in the ability of respondents to detect elephants. It is likely that variation in the detectability of forest elephants is a valid proxy for variation in abundance, rather than variation in an ability to detect elephant signs. Site-level detectability, therefore, may be a signal of the relative abundance of elephants in occupied sites, suggesting that elephants are more abundant in the south-western and south-eastern forest management unit groups than elsewhere (Supplementary Fig. 1) but that abundance may be decreasing over time in the study area as a whole (Table 2, Fig. 2).

Fig. 2 (a) Detection probability and (b) relative abundance of forest elephants Loxodonta cyclotis in the study area in eastern Cameroon during 2008–2013.
Table 2 Beta summary of best fitting model of occupancy and detectability of forest elephants Loxodonta cyclotis in timber production forests in eastern Cameroon (Fig. 1) p(C + YW + G + Y) Ψ (V + Ri + Ro + E + G), with occupancy and detectability covariates.
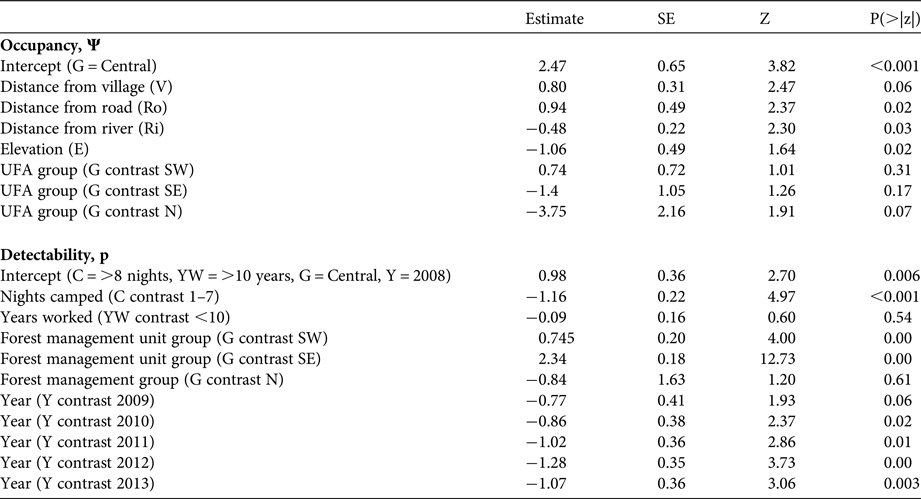
Detectability decreased by c. 30% between 2008 and 2013, which can be translated into a potential reduction in relative abundance of c. 40% (Fig. 2). Although the model shows no significant difference in occupancy related to the forest management unit groups, there is a significant difference in detectability between the groups. The confidence limits for occupancy were wide (Fig. 3), which is why the difference in occupancy is not significant. In contrast, the confidence limits for detectability were narrow for the central, south-western and south-eastern forest management unit groups, and there is a significant difference in detectability between the forest management unit groups. However, the confidence limits for detectability in the northern forest management unit group are as wide as those for occupancy. This may be because of the low number of interviews at these sites, and therefore the interpretation of data from the northern forest management unit group should be treated with caution.
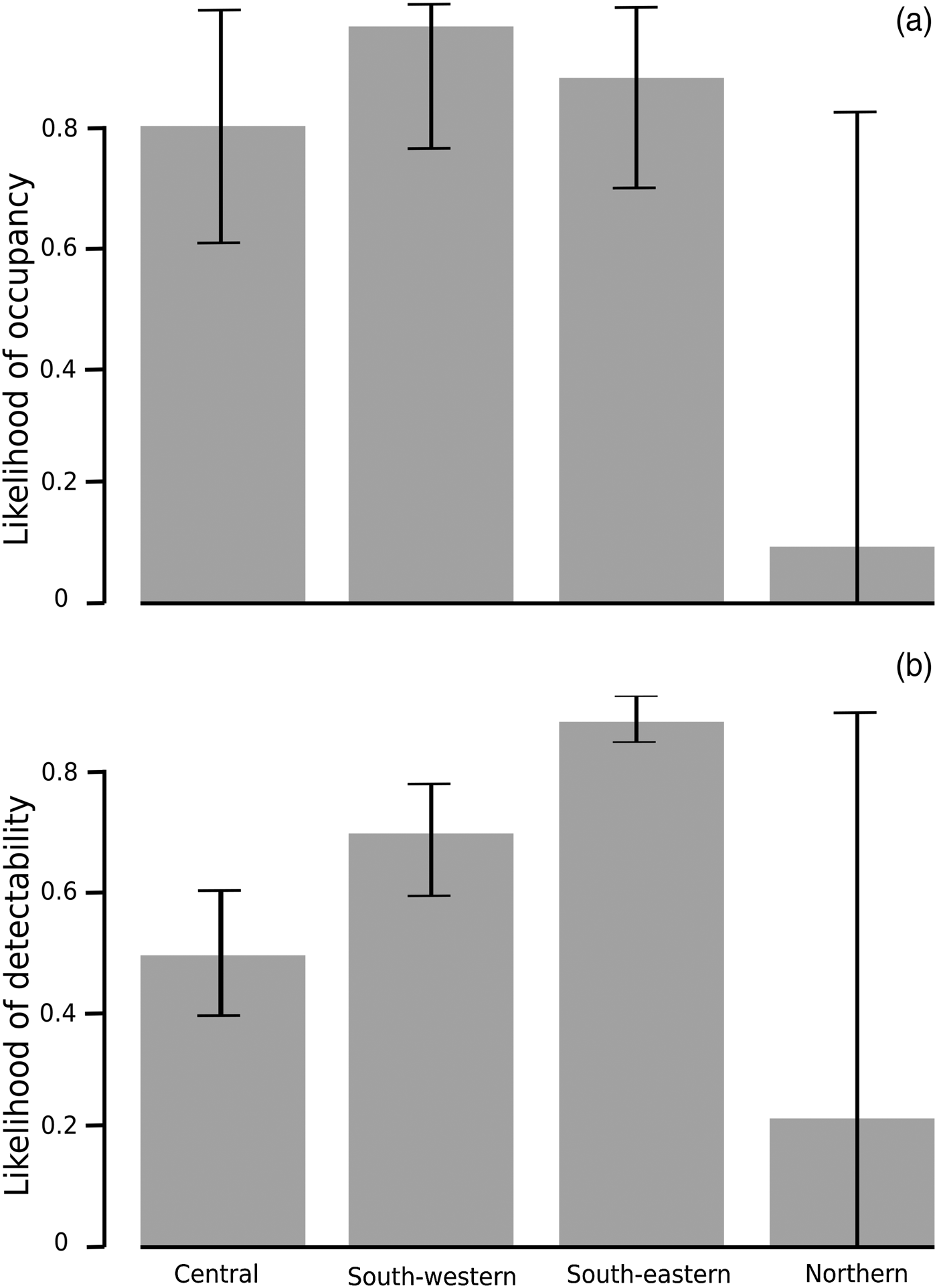
Fig. 3 Likelihood of (a) occupancy and (b) detectability (± SE) of forest elephants across the study area in eastern Cameroon during 2008–2013.
Administrative authorities and timber concession workers reported that elephants move around within the forest management units to distance themselves from villages (Buij et al., Reference Buij, McShea, Campbell, Lee, Dallmeier and Guimondou2007; de Boer et al., Reference de Boer, Langevelde, Prins, de Ruiter, Blanc and Vis2013) and the noise of logging (also reported by Bowles et al., Reference Bowles, Smultea, Wursig, Demaster and Palka1994; Richardson et al., Reference Richardson, Greene, Malme and Thomson1995). However, it was reported that elephants did not move out of the forest management units because of their proximity to villages, and major roads that separate the units, particularly in the central and northern areas, where sites of high predicted occupancy are more isolated than in the more southerly regions (Fig. 1).
Discussion
Most of the detectability covariates relate to the ability of the respondent to notice and recall signs of forest elephants; however, the forest management unit group and year provide insights into abundance in occupied sites. The data show a decline in detectability because there was a decline in detections over the 6-year study period. All sites have a similar environment, activity level and level of visibility, and observer variables are controlled for in the model.
Respondent recall must be considered when gathering data covering a long period of time; however, with the survey design and cross-checking of responses, we do not believe recall to be a significant contributor to temporal changes in detectability. It is perhaps more sensible to conclude that the declining detectability over 6 years suggests a decline in abundance, which is supported by qualitative reports of a perceived decline in elephant abundance across the whole region, and reports of increased elephant poaching. Our estimates of occupancy and detectability from the null-model (Ψ = 0.76 ± SE 0.03, P = 0.59 ± SE 0.01; Supplementary Table 6) are comparable with those of Martinez Marti, (Reference Martinez Marti2011) in neighbouring Equatorial Guinea (Ψ = 0.44 ± SE 0.03, P = 0.86 ± SE 0.01). The pattern of declining relative abundance is consistent with the findings of Maisels et al. (Reference Maisels, Strindberg, Blake, Wittemyer, Hart and Williamson2013), and also with data from the CITES Monitoring the Illegal Killing of Elephants project, which show the estimated proportion of elephants killed illegally in Central Africa remained consistently above the sustainable level over the study period (CITES, 2015). The only sites with a likelihood of occupancy of >0.6 in the northern forest management unit group are adjacent to Mbam & Djerem National Park (Fig. 1), suggesting that elephant populations living in Mbam & Djerem may be using the north of the timber concession as a corridor for access to Deng Deng National Park (Supplementary Fig. 1). The same pattern of elevated likelihood of use in sites adjacent to Boumba Bek National Park is evident for the south-western group.
We have addressed a major knowledge gap concerning elephant distribution across a region of previously unsurveyed timber production forest, finding that forest elephant range extends further north and east of the 2012 known elephant range (Fig. 1), extending into areas deemed ‘unlikely’ by IUCN. Therefore, we recommend that the IUCN known elephant range be extended, and further surveys conducted in timber concessions in which elephants have been detected. In particular, sites adjacent to protected areas are potentially of high conservation value, and therefore it is important to work closely with timber concession companies to develop sustainable logging approaches and anti-poaching activities to help protect forest elephants.
High levels of occupancy throughout the south-eastern and south-western forest management unit groups, and a high likelihood of occupancy in sites adjacent to National Parks (as also suggested by Lamb et al., Reference Lamb, Erskine and Parrotta2005) in the north, support the suggestion that well-managed timber concessions can provide refuge to forest elephants in an otherwise insecure landscape (Weinbaum et al., Reference Weinbaum, Nzooh Dongmo, Usongo and Laituri2007; Clark et al., Reference Clark, Poulsen, Malonga and Elkan2009; Kolowski, Reference Kolowski, Blake, Kock, Lee, Henderson, Honorez and Alonso2010; Stokes et al., Reference Stokes, Strindberg, Bakabana, Elkan, Iyenguet and Madzoké2010). However, the emphasis on ‘well-managed’ means that additional support should be provided to concessions with a high likelihood of occupancy, to improve their sustainability practices and ensure that they can continue to operate in a manner that minimizes the impact on remaining elephant populations.
Despite the high levels of occupancy in some sites, the detectability (and therefore relative abundance) is low in comparison to that observed elsewhere (Martinez Marti, Reference Martinez Marti2011) possibly indicating relatively low abundance in occupied sites, and further highlighting the importance of conservation action in these sites. For example, our findings suggest that it would be useful to carry out a more detailed survey in key concession units around protected areas in the north, which appear to be acting as de facto corridors. Such surveys could inform actions to develop more formal corridors to ensure the sustainability of these populations, which currently appear to be isolated and potentially unviable in the longer term.
The informal interviews and open-ended questions highlighted some key issues, in particular the high financial value of ivory, the lack of comparable alternative livelihoods in the face of increasing international demand, the lack of law enforcement, and high levels of corruption (Supplementary Material 2).
Sampling in this rapid assessment was limited to sites in which timber concession workers had been prospecting or exploiting, and consequently the sites mostly changed each year, as each site represented an area of annual exploitation, and the impacts of exploitation could not be explored, as variation in exploitation category (pre/during/post exploitation) was not available. Applying this approach in situations in which respondents’ spatial frames of reference are more spatially stable would be desirable to support multi-season occupancy modelling (Royle & Kéry, Reference Royle and Kéry2007), potentially facilitating a more sophisticated analysis of the dynamics of occupancy over time.
Occupancy estimates are generally in line with the observed detection histories and with perceived abundance. However, there are areas where the occupancy predictions do not match actual observations. Areas of under-representation within the detection/non-detection data may be an influencing factor. Alternatively, heterogeneity caused by incomplete overlap between home range and site may influence the probability of detecting an individual, as does the number of elephants within each plot. As a result, the relationship between the distribution of sampling effort and elephant home ranges may account for some variation between the naïve pattern and the predicted occupancy (Efford & Dawson, Reference Efford and Dawson2012).
Despite these potential sources of uncertainty, our findings suggest that interview-based occupancy analysis is a reliable method for the rapid assessment of forest elephant occupancy at large spatio-temporal scales and in challenging forest habitat, as a complement to, or first stage in, a monitoring process (Meijaard et al., Reference Meijaard, Mengersen, Buchori, Nurcahyo, Ancrenaz and Wich2011). Using this method we were able to gain new insights into the distribution and trends in forest elephant populations at a large scale, effectively surveying c. 30,000 km2 in 10 weeks on a budget of GBP 2,000. We also produced contextual qualitative insight data, providing a socio-demographic context that can inform subsequent conservation planning.
This approach is best suited to surveying large, remote areas that potential informants visit on a regular basis, for poorly known but easily recognizable species (Meijaard et al., Reference Meijaard, Mengersen, Buchori, Nurcahyo, Ancrenaz and Wich2011). Several authors (e.g. Pan et al., Reference Pan, Cunningham and Milner-Gulland2015; Nash et al., Reference Nash, Wong and Turvey2016) have used sightings by local people to infer changes in presence or abundance of species with these characteristics. However, with the addition of an occupancy modelling framework to structure and analyse observational datasets, more robust inferences may be drawn, specifically about detection-corrected occupancy, its covariates (such as geographical factors) and, through spatio-temporal changes in detectability, trends in relative abundance. The additional requirements for using an occupancy approach are not onerous: they include collecting information about potential observer effects (such as time spent in the forest), and biophysical variables, ensuring at least four repeat observations per sampling unit, and choosing sample units that are familiar to the respondents, to collect spatially accurate data.
Acknowledgements
We thank ZSL Cameroon for their assistance during data collection, and Imperial College London for financial support. The work was supported by a NERC-CASE studentship to SB. Datasets are available on request from the corresponding author.
Author contributions
SB and MNB collected the data. SB designed the study and conducted analysis, with guidance from MR and PDO. SB led the publication of the article, with support and editing from EJMG and MR and input from the whole team.
Biographical sketches
Stephanie Brittain's research interests lie in the use of local knowledge for wildlife population monitoring. Madeleine Ngo Bata monitors threatened species in timber forest concessions in Cameroon. Paul De Ornellas focuses on the illegal wildlife trade, and improvement of monitoring within reserves across Africa. E.J. Milner-Gulland has a broad range of interests in interdisciplinary conservation science (her research group page is at http://www.iccs.org.uk). Marcus Rowcliffe is a conservation scientist interested in improving methods for the monitoring of elusive and threatened species.