INTRODUCTION
Working memory (WM) is related to language processing (e.g., Juffs & Harrington, Reference Juffs and Harrington2011), for example, when a reader holds earlier parts of incoming information in memory until integrating them with latter parts during reading (e.g., Cowan, Reference Cowan2005). WM has a limited capacity, leading to trade-off between processing and storage of information (Daneman & Merikle, Reference Daneman and Merikle1996): Cognitive resources for maintenance are reduced if processing is not automatic or requires longer time. Thus, slow readers may have difficulty maintaining what they have read, lose memory traces while reading, and may then fail in overall comprehension. The relationship between WM and reading has been examined in many second-language (L2) studies, with mixed findings and various moderator variables seemingly coming into play (e.g., Juffs & Harrington, Reference Juffs and Harrington2011; Sagarra, Reference Sagarra2017), some of which have not been examined in detail. In this article, we approach these issues using meta-analysis.
LITERATURE REVIEW
WORKING MEMORY
WM is a memory system conceptualized in various ways. Baddeley and Hitch’s (Reference Baddeley and Hitch1974) model considered both temporal storage of information and processing and manipulation of stored information while engaged in a task; in their model, WM plays an important role in not only remembering information temporarily but also controlling, selecting, and maintaining information concurrently with other information.
In Baddeley and Hitch (Reference Baddeley and Hitch1974), WM consists of three parts. The central executive is in charge of directing attention to relevant information, inhibiting irrelevant information, and coordinating information between the phonological loop and visuo-spatial sketchpad. The phonological loop briefly holds auditory information by rehearsing it subvocally. The visuo-spatial sketchpad briefly holds visual and spatial information about things. These three components also appear in Baddeley (Reference Baddeley2012), which includes a fourth component—the episodic buffer, which holds integrated information or chunks from a variety of sources.
Many researchers have investigated WM’s role in second language acquisition (SLA) using Baddeley and Hitch (Reference Baddeley and Hitch1974). The phonological loop and central executive have been most studied and considered the most relevant (Wen, Reference Wen2016). Sound-based information is held in the phonological loop over a brief period through articulatory rehearsal, by which one subvocally repeats the information to prevent rapid forgetting. Information is then processed in the central executive, whose functions include updating information, switching/shifting attention between tasks, and inhibiting/rejecting irrelevant information (Miyake et al., Reference Miyake, Friedman, Emerson, Witzki, Howerter and Wager2000). According to Wen (Reference Wen2016), the phonological and executive components of WM can be measured using span tasks: the former through simple memory span tasks, such as digit span and word span tasks, and both through complex memory span tasks, such as reading span and operation span tasks. Wen (Reference Wen2016) claims that the phonological component is more important for beginners and the executive component for advanced learners.
WORKING MEMORY AND L2 READING
Reading is a complicated process (e.g., Grabe & Stoller, Reference Grabe and Stoller2020; Koda, Reference Koda2007), involving word recognition, syntactic parsing, sentence and discourse processing, and inference generation. Although a passage is composed of sentences, discourse processing is not the combined result of sentence processing (e.g., Sanford & Garrod, Reference Sanford and Garrod1981). Writers try to avoid redundancy, and readers need to establish coherent understanding of the text by generating inferences using the information provided (e.g., Sanford & Emmott, Reference Sanford and Emmott2012). Thereby, readers with greater WM capacity might more efficiently connect current and previous reading content. Effects of WM capacity have been examined at various levels in first-language (L1) processing, such as those of inference generation (e.g., Currie & Cain, Reference Currie and Cain2015) and syntactic processing (e.g., King & Just, Reference King and Just1991), and a positive WM–L1 reading relationship reported, with WM capacity assessed using WM span tasks. Attempts have been made to synthesize the WM–reading comprehension relationship using meta-analysis. Three L1 meta-analyses examined the usefulness of WM for predicting students’ reading skills (Carretti et al., Reference Carretti, Borella, Cornoldi and De Beni2009; Daneman & Merikle, Reference Daneman and Merikle1996; Peng et al., Reference Peng, Barnes, Wang, Wang, Li, Swanson, Dardick and Tao2018), finding that complex WM tasks, highly demanding of attentional resources, better predicted reading comprehension performance than simple span tasks.
Studies on the WM–L2 reading relationship have revealed mixed findings. As the studies also vary, in research design, scoring methods, WM span tasks, and reading comprehension tests, what factors are responsible for differences in results remain unclear. This difficulty can be partly addressed by classifying studies according to their features in a meta-analysis, to examine how WM–L2 reading relationships in particular studies are a function of study features. As our study is a meta-analysis, three previous meta-analyses are particularly relevant. Jeon and Yamashita (Reference Jeon and Yamashita2014) meta-analyzed correlations between discourse-level L2 reading and 10 reading variables, including WM span. The average correlations from 58 studies showed that L2 reading was highly correlated with L2 grammar (r = .85), vocabulary (r = .79), and decoding (r = .56) but only moderately with WM span (r = .42 [95% confidence interval = .29, .53]). Linck et al. (Reference Linck, Osthus, Koeth and Bunting2014) found small correlations between WM and L2 proficiency (r = .255 [.219, .291], 79 studies) and between WM and L2 comprehension (r = .242 [.191, .292], 43 studies). They investigated whether these relationships were moderated by variables such as participant characteristics and WM measurement features, concluding that WM capacity has a robust positive relationship with L2 proficiency. Shin (Reference Shin2020) focused on reading span tasks and meta-analyzed correlations between discourse-level L2 reading and WM. A small relationship was found between L2 reading comprehension and WM (r = .30 [.24, .35], 25 studies). Methodological features, such as the stimuli language used in reading span measures and text type in reading comprehension tasks, moderated the WM–L2 reading relationship.
In Jeon and Yamashita (Reference Jeon and Yamashita2014), WM span received a minor focus, with only 10 studies meta-analyzed and without moderator analyses. In Linck et al. (Reference Linck, Osthus, Koeth and Bunting2014), this relationship was not examined, as L2 reading was lumped into L2 comprehension category alongside grammar and vocabulary. Shin (Reference Shin2020) focused on reading span tasks and some moderators. The scope needs to be expanded to other WM task types and moderators, including learner’s characteristics, reviewed in the following text.
REVIEW OF MODERATOR VARIABLES
Variables moderating the WM–L2 reading relationship can be classified into task variables (e.g., WM and L2 reading measures/tasks) and learner variables (e.g., proficiency levels).
TASK VARIABLES
Working memory task content
WM tasks can be classified by whether they include verbal (i.e., recalling words or letters) or nonverbal (e.g., recalling numbers) activities. Verbal tasks include word span, reading span, and listening span tasks, whereas nonverbal tasks include nonword span and digit span tasks. Task content classification is associated with a domain-specific (as against a domain-general) argument: that WM functions using domain-specific knowledge (e.g., Wen, Reference Wen2016) based on one’s domain-specific proficiency; if so, language-related WM tasks (i.e., verbal tasks) would correlate more strongly with reading comprehension measures than nonverbal WM tasks. In contrast, the domain-general argument is that WM functions independently from the domain being measured; if so, language-unrelated WM tasks (i.e., nonverbal tasks) and language-related WM would relate to reading comprehension tasks similarly.
Working memory task language
Language-related WM tasks (verbal tasks) have been criticized because it is not clear whether reading span tasks or listening span tasks measure pure WM span or comprehension skills (Daneman & Merikle, Reference Daneman and Merikle1996). Critics argue that if WM tasks are presented in L1, WM span would be estimated higher than in L2. Between L1- and L2-based WM tasks, various correlations have been reported (e.g., r = .72 and .84, Osaka & Osaka, Reference Osaka and Osaka1992; r = .39, Harrington & Sawyer, Reference Harrington and Sawyer1992). For the WM–L2 reading relationship, Linck et al. (Reference Linck, Osthus, Koeth and Bunting2014) showed no moderating effect across L1 and L2 (r = .228 and .229), whereas Shin (Reference Shin2020) reported that the relationship was stronger in L2 WM tasks (r = .35) than in L1 WM tasks (r = .17). Therefore, it remains unclear whether and to what extent stimulus language moderates the WM–L2 reading relationship.
Further, Alptekin and Erçetin (Reference Alptekin and Erçetin2010) suggested that differences between WM span measured in L1 WM tasks and L2 WM tasks would decrease as L2 proficiency increases. If so, as L2 proficiency increases, the relationship between WM (measured in L2 WM tasks) and L2 reading would become similar in size to the one between WM (measured in L1 WM tasks) and L2 reading. This possibility merits examination.
Working memory task complexity
WM span tasks have been classified as simple or complex based on the construct they measure (e.g., Wen, Reference Wen2016). Simple span tasks measure information storage in the phonological component of WM and include word span tasks (recall of several words shown right beforehand), nonword span tasks, and digit span tasks. In contrast, complex span tasks measure storage and processing of information in the phonological and central executive components of WM simultaneously, and include reading span, operation span, and N-back span tasks. In complex span tasks, participants memorize words and recall them afterward, while completing an additional processing task to measure processing of information, for example, judging grammaticality/semantic acceptability of sentences or solving mathematical operations. In addition, reaction times of correct responses in judgment tasks can be analyzed.
Previous studies suggest that WM assessed using complex span tasks is more strongly correlated than WM assessed using simple span tasks with L1 reading (Daneman & Merickle, Reference Daneman and Merikle1996; e.g., r = .41 vs. .28 in complex and simple tasks, respectively) and with L2 comprehension and production combined (Linck et al., Reference Linck, Osthus, Koeth and Bunting2014; r = .272 vs. .175). These findings merit further examination regarding reliability and proficiency levels. First, different strengths of WM–L2 reading relationships across simple versus complex span tasks could be partly because complex span tasks have higher reliability than simple span tasks, better discriminating participants and yielding larger correlations between WM and reading. For example, Waters and Caplan (Reference Waters and Caplan2003) found that reliability for complex span tasks was typically within .80–.90 in previous studies. Reliability of simple span tasks is less established, but Carpenter and Alloway (Reference Carpenter and Alloway2018) reported a range of .69–.89—slightly lower than that of complex span tasks, which could partly explain the stronger relationship for WM using complex tasks than using simple tasks with reading. Second, studies suggest differential relationships across simple and complex span tasks as L2 proficiency advances (Wen, Reference Wen2016), detailed in the “Proficiency Level” section.
Mode of working memory and reading comprehension
WM has been measured using paper-and-pencil and computer-based tests. Although mode effects have been discussed for L2 reading tests (Sawaki, Reference Sawaki2001), it is unclear whether these modalities yield equivalent scores for WM tests. An exception is Carpenter and Alloway (Reference Carpenter and Alloway2018), which found better performance in paper-and-pencil verbal phonological tests and WM tests than on computer. Modality effects on in WM research merit investigation.
Reading comprehension test standardization
Standardized tasks include large-scale proficiency tests developed by teams of experts and administered widely (e.g., the TOEFL test), designed for specific purposes (e.g., screening university applicants), and producing comparable scores across different versions of the same test. However, these tasks may not measure proficiency well in a local context where participants are homogenous in ability. In contrast, nonstandardized tasks include textbook-based and author-made reading comprehension tests, small-scale and locally developed to meet needs of researchers conducting a study. These tasks can be finer-grained measures discriminating among participants of similar proficiency or can be developed ad-hoc with few items and low reliability. Shin (Reference Shin2020) reported that the WM–L2 reading relationship was slightly stronger when standardized reading tasks were used (r = .28) than nonstandardized reading tasks (r = .24). Whether the results are replicable merits examination.
Scoring methods for complex span tasks
Scoring methods for complex span varies across studies, but comparative analyses are scarce (e.g., Conway et al., Reference Conway, Kane, Bunting, Hambrick, Wilhelm and Engle2005; Juffs & Harrington, Reference Juffs and Harrington2011). Research is warranted particularly as, with more widespread use of computers in classroom and research settings, reaction times of correctly judged sentences in judgment tasks have been included in WM measures, and many scoring methods are possible. Waters and Caplan (Reference Waters and Caplan1996) argue that (a) correct responses in judgment tasks (storage) and (b) reaction times of correctly judged sentences can mode how participants allocate limited cognitive resources—to judging sentences or remembering sentence-final words. Including (a) and (b) allows for considering trade-offs across recalling and processing to better capture WM span. Shin (Reference Shin2020) showed that WM was more strongly related to L2 reading comprehension when storage and processing task scores were used (r = .33) than when these task scores and reaction time (r = .30) or only storage scores (r = .20) were used.
Leeser and Sunderman (Reference Leeser, Sunderman, Granena, Jackson and Yilmaz2016) compared complex span task scoring methods using responses in a sentence processing task: “Recall: the number of sentence-final words recalled correctly” and “Set Size: the highest set size number at which participants recalled all of the items correctly for at least two-thirds of the sets” (Leeser & Sunderman, Reference Leeser, Sunderman, Granena, Jackson and Yilmaz2016, pp. 92–93). Correlations between WM (measured using reading span tasks) and L2 sentence interpretation varied across scoring methods (r = .028 to .276, all nonsignificant). Except for Leeser and Sunderman (Reference Leeser, Sunderman, Granena, Jackson and Yilmaz2016), comparative analyses of scoring methods for complex span have not been conducted in primary studies, partly because investigation into their potential impact on the WM–L2 reading relationship was not the focus. Yet, comparative analyses of scoring methods for complex span can be done in meta-analysis by classifying studies by scoring method, to expand meta-analysis targeting reading span tasks.
Reliability of working memory span and comprehension tasks
For research into the WM–L2 reading comprehension relationship using reading tasks, measurement precision is of paramount importance; when psychometric properties of measures are not reported, it is unclear whether small correlations between WM span and L2 reading comprehension are due to low quality of measures, real (weak) status of the relationship, or both. In L1 research, Waters and Caplan (Reference Waters and Caplan2003) reported reading span task reliability of .73 and .76 (test-retest) and backward digit span reliability of .65 (test-retest), .813, and .825 (Cronbach’s alpha), suggesting that WM span measures have sufficient reliability (Conway et al., Reference Conway, Kane, Bunting, Hambrick, Wilhelm and Engle2005, had similar results).
In L2 studies, referring to WM tasks, Juffs and Harrington (Reference Juffs and Harrington2011) argue that “L2 articles rarely report reliability values” (p. 145) and that “to obtain reliability scores … is a promising way forward” (p. 158). Shin (Reference Shin2020) showed that only 9 and 12 out of 37 studies (24.32% and 32.43%) reported reliability of reading span tasks and L2 reading tests, respectively. The WM–L2 reading relationship was stronger when reading span task reliability was reported (r = .30) than when not (r = .25). The relationship was similar in size across studies that did and did not report the reading comprehension task reliability (r = .27 in both cases). Overall, WM span task and comprehension task reliability have not been widely examined: only 6%–64% of studies in syntheses for L2 subdomains reported reliability (Plonsky & Derrick, Reference Plonsky and Derrick2016). Thus, besides WM span task and L2 reading comprehension measures, the percentage of studies reporting reliability of these measures needs examination.
LEARNER VARIABLE
Proficiency levels
Learner proficiency levels have been examined in relation to the WM–reading relationship (e.g., Wen, Reference Wen2016). Linck et al.’s (Reference Linck, Osthus, Koeth and Bunting2014) meta-analysis showed that WM was related to L2 reading to a similar degree across proficiency levels (comprehension [including reading] and production combined; r = .255 for less proficient learners, and r = .259 for highly proficient learners), suggesting that less and highly proficient learners equally benefit from WM capacity.
Regarding specific relationships between WM components, L2 skills, and L2 proficiency levels, Kormos and Sáfár (Reference Kormos and Sáfár2008) measured secondary school students’ phonological components of WM and L2 proficiency and found different relationships between memory subcomponents, L2 skills, and L2 proficiency. For beginners, phonological memory was not related to any subcomponent of performance; for preintermediate learners, phonological memory was moderately related to grammar and vocabulary combined, writing, and overall proficiency (r = .49, .48, and .47, respectively). This suggests that preintermediate learners benefit more from phonological memory than beginners, as they receive more explicit instruction, including memorization of many grammar and vocabulary learning rules (see Williams, Reference Williams, Gass and Mackey2012), while preintermediate learners undergo more implicit learning of new words, influenced by phonological memory (Masoura & Gathercole, Reference Masoura and Gathercole2005). Thus, Wen (Reference Wen2016) argued that phonological memory plays different roles by proficiency.
Summarizing previous studies, Wen (Reference Wen2016) describes how phonological and central executive components of WM relate to language learning across proficiency levels. With low L2 proficiency, the phonological component is postulated to have a greater association with development of vocabulary and grammar than the central executive component because remembering sound-based information through articulatory rehearsal in phonological memory contributes to these areas at early learning stages, in L1 and L2. As learners progress, the central executive component is hypothesized to become more important, to address more cognitively demanding tasks requiring processing information while remembering it temporarily, which involves the central executive component. Thus, Wen (Reference Wen2016) suggests the importance of examining how components of WM operate while considering learners’ proficiency levels. To better understand the WM–L2 reading relationship, with proficiency level as a potential moderator variable, meta-analytic investigation seems useful.
RESEARCH QUESTIONS
The current meta-analysis examined the WM–L2 reading relationship across studies and the impact of different moderator variables on it. Mode of WM and L2 reading comprehension measures were not investigated in previous meta-analyses.
Two research questions were addressed: What is the WM–L2 reading relationship, and how is it moderated by task and learner variables?
METHOD
LITERATURE SEARCH
We conducted a search in October 2020 in three ways (Figure 1).

FIGURE 1. Flow diagram for literature search and inclusion of studies.
Search was conducted first using databases (Educational Resources Information Center [ERIC], Linguistics and Language Behavior Abstracts [LLBA], PsycINFO, ScienceDirect, and Web of Science) and Google Scholar and second using journals with search functionality on their websites: broad-scope journals (Annual Review of Applied Linguistics, Applied Linguistics, Foreign Language Annals, Language Learning, Language Testing, Modern Language Journal, RELC Journal, Studies in Second Language Acquisition, System, and TESOL Quarterly); and reading and psycholinguistics journals (Applied Psycholinguistics, Discourse Processes, Journal of Research in Reading, Reading in a Foreign Language, and Transactions of the Philological Society). We used keywords—L2, second language, foreign language, bilingual, reading span, phonological, short term, executive, reading, and working memory—derived from keywords and synonyms retrieved from thesauruses supplied in databases, books and articles reviewed, authors’ experience, and feedback from colleagues and reviewers. Abstract, title, and keyword searches were conducted, with no restrictions on publication date or language. Third, recent relevant resources were inspected, including Wen’s list of studies on WM (Reference Wen2016, Table 5.2), its updated version (Wen, Reference Wen2018), previous meta-analyses of the WM–L2 comprehension relationship (Jeon & Yamashita, Reference Jeon and Yamashita2014; Linck et al., Reference Linck, Osthus, Koeth and Bunting2014), and meta-analyses of the relationship between aptitude and learning/grammar acquisition (Li, Reference Li2015, Reference Li2016) because WM has been discussed in relation to aptitude. Across these three ways of searching, the reference list of each paper or chapter, both published and unpublished, was scrutinized for additional materials.
STUDY INCLUSION CRITERIA
To be included, a study had to (a) examine the WM–L2 reading relationship using correlations (Pearson or Spearman) or other statistics that could be converted to correlation coefficients and (b) target nonclinical L2 learners. Spearman correlations included one from Bailer et al. (Reference Bailer, Tomitch and D’Ely2013) and four from Chun and Payne (Reference Chun and Payne2004). Statistics converted to correlations were two standardized regression beta coefficients (Rai et al., Reference Rai, Loschky, Harris, Peck and Cook2011), converted into correlations based on Peterson and Brown (Reference Peterson and Brown2005). Six correlations in Alptekin and Erçetin (Reference Alptekin and Erçetin2010), two in Hummel (Reference Hummel1999), and one in Sagarra (Reference Sagarra2017) were reported nonsignificant, with no corresponding values; they were replaced with zero. This method to handle missing data is known to underestimate an average effect size across studies (see Lipsey & Wilson, Reference Lipsey and Wilson2001). Linck et al. (Reference Linck, Osthus, Koeth and Bunting2014) conducted sensitivity analysis of this method’s impact on their results by entering versus removing such correlations from their analysis, as do we.
As Figure 1 shows, 548 studies were identified initially. They were examined for all previously mentioned criteria by the first author, leaving 74 studies (see Appendix A).
CODING
The 74 selected studies (228 correlations) were coded in terms of correlations and moderators as follows. Other potentially interesting variables were often found unreported and were not included (e.g., topic familiarity; group vs. individual administration of WM measures; experimenter-paced vs. self-paced mode of WM measures; allowability of looking back at text when answering reading comprehension questions; time limit vs. no time limit for reading comprehension measures; strategy use while answering WM tasks; reading subskills [e.g., literal vs. inferential items]).
Task variables
Following Linck et al. (Reference Linck, Osthus, Koeth and Bunting2014), task variables coded were (a) WM task content (verbal, nonverbal, or both); (b) WM task language (L1 or L2); and (c) WM task complexity (simple or complex). Further, we coded (d) mode of WM and comprehension tasks (paper-based or computer-based); (e) reading comprehension test standardization (standardized or nonstandardized); (f) scoring methods for complex span; and (g) reliability of WM span and L2 reading comprehension tasks. We explain some of these variables below (see also Appendix B, Table 1 coding sheet).
TABLE 1. WM and L2 reading: overall and moderator variable analysis
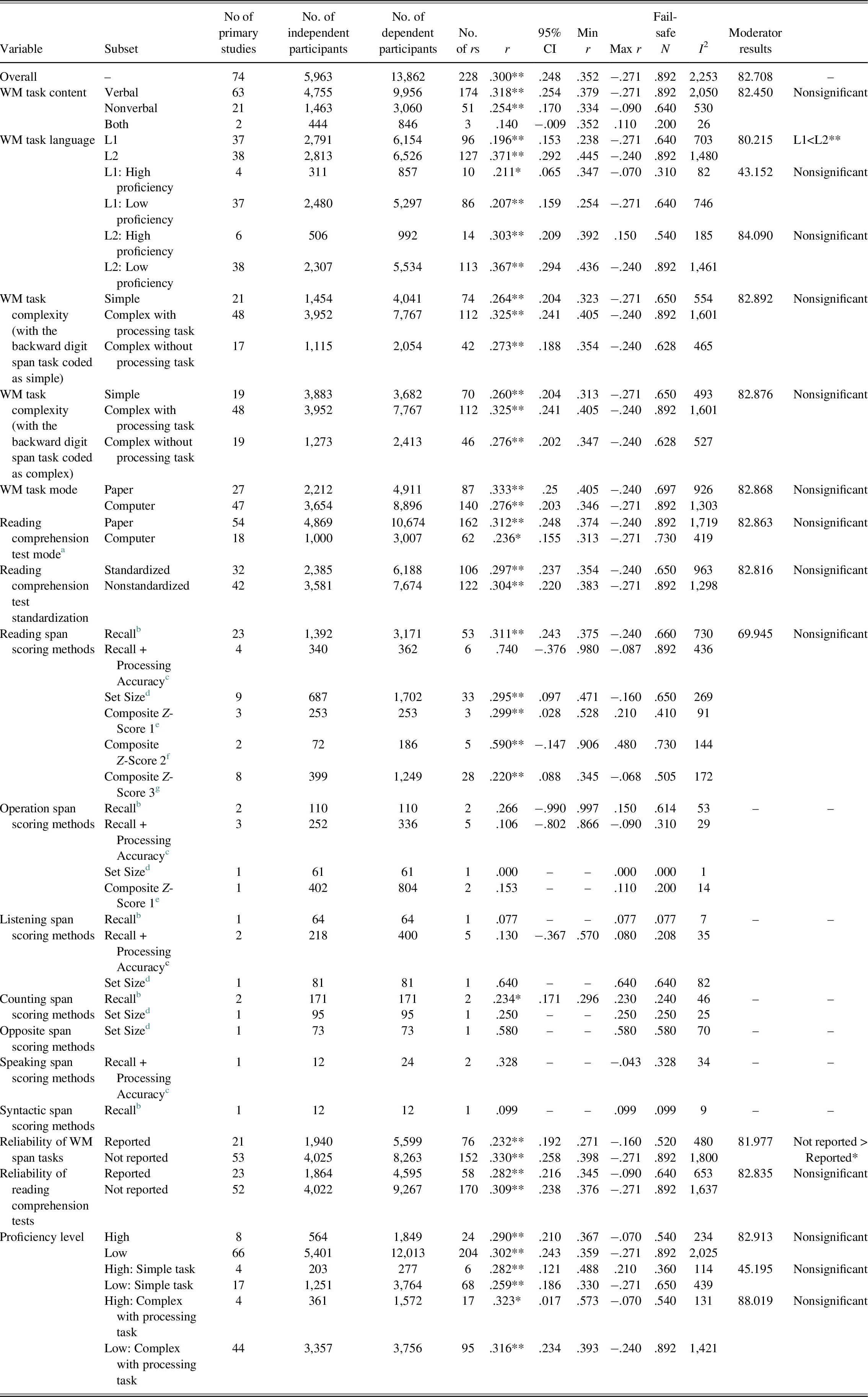
* p < .05; ** p < .01. CI = confidence interval.
a The “both” condition was removed from analysis, for the following reason. Three correlations from one study (Walter, Reference Walter2007) were coded as measuring reading comprehension in both paper and computer formats. However, when this “both” condition was analyzed along with (a) paper condition and (b) computer condition, a warning message appeared stating “In sqrt(diag(VR.r)): NaNs produced.” This indicated that negative values were produced that should have been positive. This problem occurs because, for example, there is not much information to extract from the data (Troubleshooting with glmmTMB, 2020), causing difficulty in computing the standard error of the synthesized correlation for the “both” condition or the significance of the synthesized correlation.
b Recall (the total number of words recalled correctly).
c Recall + Processing Accuracy (the number of words recalled for sentences or mathematical operations judged accurately or generated).
d Set Size (the highest set size number [at which participants recalled all items correctly for at least two-thirds of sets]).
e Composite Z-Score 1 (an average of z-scores for reaction times of correctly judged sentences/mathematical operations, number of correctly judged sentences/mathematical operations, and total number of items recalled).
f Composite Z-Score 2 (an average of z-scores for reaction times of correctly judged sentences/mathematical operations, percentage of correctly judged sentences/mathematical operations, and set size).
g Composite Z-Score 3 (an average of z-scores for the number of correctly judged sentences/mathematical operations and the total number of items recalled).
For (c), simple span tasks included backward digit span, digit span, nonword span, pseudoword repetition, sentence repetition, and word span. Complex span tasks included counting span, listening span, operation span, opposite span, reading span, speaking span, and syntactic span. Based on Waters and Caplan (Reference Waters and Caplan1996), complex tasks were further coded as to whether to include a processing task (e.g., sentence acceptability/verification judgment tasks in reading span tasks, in which participants’ responses were recorded) or not (e.g., include read-aloud tasks, in which participants’ responses did not seem to be recorded). Because backward digit span tasks were considered either simple (Linck et al., Reference Linck, Osthus, Koeth and Bunting2014) or complex (Kormos & Sáfár, Reference Kormos and Sáfár2008), we coded them accordingly. These tasks require participants to memorize numbers presented sequentially and to recall them backward. Conceptualizing them as simple or complex seems to depend on whether researchers consider that recalling numbers backward requires only storage in the phonological component or both storage and processing of information in the phonological and central executive components.
Regarding (f), based on Leeser and Sunderman (Reference Leeser, Sunderman, Granena, Jackson and Yilmaz2016), we included six scoring methods: Recall, Recall + Processing Accuracy, Set Size, Composite Z-Scores 1, 2, and 3. Definitions of the first four methods were as in Leeser and Sunderman. See the note for Table 1.
Concerning (g), reliability, or internal consistency (i.e., Cronbach’s alpha and Kuder–Richardson Formula 20), was coded for each measure. If multiple reliability indices were reported for a single measure (e.g., reliability for WM span task 1 and a different reliability for WM span task 2) in multiple measures of the same construct, they were averaged. For 13 correlations across four studies, reliability estimates were reported separately for storage and processing tasks and averaged for each correlation.
Learner variable
We categorized participants as low or high proficiency, following Linck et al. (Reference Linck, Osthus, Koeth and Bunting2014). High learners were those enrolled in an academic program conducted entirely in their L2, majoring in language at a graduate school, or working in a foreign-language environment; others were low proficiency. Note that proficiency levels have been defined differently across studies, and that no universally agreed-upon definition of this construct is available (Thomas, Reference Thomas1994).
Other issues
For longitudinal studies reporting multiple-timepoint data, we coded Time 1 data, as Time 2 or later data could be influenced by time-varying extraneous variables. We found one longitudinal study, Speciale et al. (Reference Speciale, Ellis and Bywater2004), which provided four correlations in Time 1 and two in Time 2. The four correlations in Time 1 were coded.
There were two cross-sectional studies that included multiple correlations. We coded correlations considered most representative of the study. Walter (Reference Walter2004) presented correlations for low-proficiency groups, high-proficiency groups, and two overall groups; we coded only two correlations from the overall groups. Shin et al. (Reference Shin, Dronjic and Park2019) provided correlations for a treatment A group, a treatment B group, and an overall group; we coded only one correlation from the overall group.
To examine interrater reliability, 24 of the 74 studies were coded by the first and second authors; agreement ranged from 87% to 100% across all coded variables. Disagreement was resolved through discussion. The remaining studies were coded by the first author.
ANALYSES
Following a typical meta-analysis methodology (e.g., Plonsky & Oswald, Reference Plonsky, Oswald and Plonsky2015), we conducted a two-stage analysis. First, a correlation coefficient from each study was used as an effect size estimate. Because most studies did not report reliability of WM span or L2 reading tasks (only 27.03% [20/74] did so for WM span tasks and 29.73% [22/74] for reading tasks), we did not correct correlations for attenuation. Second, retrieved correlation coefficients were converted to Fisher’s z-scale and aggregated to estimate an average sample-size-weighted effect size; the result was converted back to correlations for interpretation. This procedure was repeated for correlations between WM and reading with/without grouping by moderator variables.
Sample-size-weighted effect size aggregation was conducted using a random-effect model, addressing dependent effect sizes (e.g., two or more effect sizes from a single study) using robust variance estimator with the robumeta package in R (Fisher & Tipton, Reference Fisher and Tipton2015). This allows modeling of within-study dependencies and is considered a more appropriate way to address them than averaging dependent effect sizes per study (Tanner-Smith et al., Reference Tanner-Smith, Tipton and Polanin2016).
For moderator variable analysis, significance was specified as p < .05. Categorical moderator variables were dummy-coded. Subset differences in categorical moderator variables were examined by changing reference levels in turn, following the Econometric Computing Learning Resource (2015). I 2 statistics were calculated to examine what proportion of variance observed reflected true variance rather than sampling error. The minimum number of correlations for moderator analyses was three, following Li (Reference Li2016). Correlations for speaking, syntactic, listening, operation, counting, and opposite span scoring methods were mostly below three in number but were analyzed for any overall trend.
Publication bias (file drawer problem) was examined using Orwin’s (Reference Orwin1983) failsafe N, with a criterion effect size of .01 (r = .01) and an effect size of 0 for missing values (r = .00). The obtained failsafe N suggests the number of studies needed to reduce the summary effect to the criterion effect. Failsafe Ns that reached or exceeded 5k + 10, where k was the number of studies combined, were considered to indicate a trivial effect of publication bias and to support the interpretation of the summary effect (Rosenthal, Reference Rosenthal1979). Failsafe Ns were calculated in Microsoft Excel because the current robumeta package does not conduct publication bias analyses. Averaged correlations of .25, .40, and .60 were interpreted as small, moderate, and large correlations, respectively (Plonsky & Oswald, Reference Plonsky and Oswald2014).
RESULTS
WORKING MEMORY AND L2 READING
As Table 1 shows, the sample-size-weighted synthesized correlation between WM and L2 reading was small in size (r = .300 [.248, .352], p < .001). The I 2 indicates that 83% of variance observed reflects true variance in the overall correlation between WM and moderator variables rather than sampling error. This suggests the need for moderator analyses to explain the variability. The results showed no evidence of a file drawer problem. For the overall reading data, the failsafe N was 2,253, indicating that 2,253 studies were needed to reduce the summary effect (r = .300) to the criterion effect (r = .01). This number exceeded Rosenthal’s (Reference Rosenthal1979) criterion (5 × 74 + 10 = 380) and indicates a trivial impact of publication bias in the current data. Results from other analyses in terms of file drawer problem were similar, suggesting a trivial impact of publication bias. When the nine correlations reported as nonsignificant and replaced with zero were removed, the synthesized effect size was .307 (.254, 357), p < .001 (not reported in Table 1), almost identical to when these correlations were included, suggesting little impact of coding method. We report results including all these nine correlations.
TASK AND LEARNER VARIABLES IN WORKING MEMORY SPAN AND L2 READING
The correlation between WM and L2 reading was similar when WM was measured using verbal task (r = .318 [.254, .379]), nonverbal task (r = .254 [.170, .334]), and both (r = .140 [−.009, .352]). These correlations were not significantly different, suggesting that WM task content does not seem to moderate the WM–L2 reading relationship.
Of the remaining variables, most were not significant either. For example, as for WM task complexity, using simple or complex tasks to measure WM capacity was likely to produce similar degrees of correlations between WM and reading comprehension. Results were the same regardless of whether the backward digit span task was coded as a simple or complex span task. Nonsignificant findings were also found for WM task mode: WM measures in paper or computer format yielded similar correlations between WM and reading comprehension.
However, we found two variables significantly moderating the WM–L2 reading correlation: WM task language and reporting of reliability of WM span tasks. First, the correlation was significantly higher when WM task language was in L2 (r = .371 [.292, .445]) than in L1 (r = .196 [.153, .238]), which aligned with Shin (Reference Shin2020). This suggests the impact of the language used in WM tasks, with larger WM–L2 reading correlations likely when WM is measured in the L2. Second, the correlation between WM and L2 reading was significantly larger for datasets where WM span task reliability was not reported (r = .330 [.258, .398]) than where it was (r = .232 [.192, .271]).
DISCUSSION
This meta-analysis examined two research questions. First, concerning the WM–L2 reading relationship (research question 1), the synthesized correlation was small (r = .300 [.248, .352]). This suggests a weak relationship of WM with L2 reading and concurs with previous meta-analyses examining the WM–L2 reading relationship (Jeon & Yamashita, Reference Jeon and Yamashita2014, r = .42 [.29, .53]; Shin, Reference Shin2020, r = .30 [.24, .35]) and that between WM and L2 comprehension, including reading (Linck et al., Reference Linck, Osthus, Koeth and Bunting2014, r = .242 [.191, .292]). The confidence intervals of the correlations substantially overlap, suggesting that the WM–L2 reading relationship is similar in size across meta-analyses. Repeatedly obtaining relatively weak relationships across meta-analyses suggests that the impact of WM on L2 reading is small but consistent. Second, concerning moderators affecting this relationship (research question 2), the results—both statistically significant and nonsignificant—are discussed in the following text.
WORKING MEMORY TASK CONTENT
WM measured using verbal tasks, nonverbal tasks, and both tasks correlated with reading to a similar degree. Thus, choice of verbal, nonverbal, or combined tasks does not moderate the WM–reading comprehension relationship, supporting the domain-general argument, that WM is involved in maintaining and processing relevant information requiring complex cognition, such as language comprehension across domains, and is not limited to processing particular linguistic stimuli. The results also answer for a lack of criteria for selecting domain-specific (e.g., verbal tasks) or domain-general tasks (e.g., nonverbal tasks) raised by Wen (Reference Wen2016). As WM task content was not a significant moderator, it would not influence the results which task content type was selected for use. Note that these results could be a function of learners’ L2 proficiency levels and should be interpreted with caution.
WORKING MEMORY TASK LANGUAGE
The average correlation between WM and reading was significantly larger when WM task language was L2 (r = .371 [.292, .445]) than L1 (r = .196 [.153, .238]). This suggest the impact of the language in which WM is measured. Although the results did not concur with Linck et al. (Reference Linck, Osthus, Koeth and Bunting2014), who reported no difference between WM measured in L1 and L2 (r = .228 vs. .299), they were consistent with Shin (Reference Shin2020; r = 35 [.34, .36] in L2 vs. r = .17 [.16, .17] in L1). However, as mentioned, Linck et al. (Reference Linck, Osthus, Koeth and Bunting2014) did not meta-analyze correlations between WM and L2 reading alone: L2 reading was lumped into the comprehension category along with grammar and vocabulary. Shin’s (Reference Shin2020) and our meta-analyses suggest that in understanding WM–L2 reading relationships, differences in WM task language do matter: WM–L2 reading correlations would be expected to be larger for WM tasks presented in L2 than in L1. Thus, to the extent that stimuli in WM measures require the use of L2 and increase the cognitive workload of WM tasks compared with stimuli presented in L1, the measured construct represents a mixture of WM and L2 (Linck et al., Reference Linck, Osthus, Koeth and Bunting2014). Although using well-planned L1 and L2 in WM tasks may produce similar, correlated scores on WM span (Osaka & Osaka, Reference Osaka and Osaka1992), it is advisable to use L1 WM measures to minimize interference of L2 proficiency with WM capacity.
Furthermore, the results suggest that when WM tasks presented in L1 and in L2 are used, we should not average scores to obtain a more representative WM span of L2 learners. Wen (Reference Wen2016) emphasized the need for empirical verification of the average method before use. Given the current results, averaging scores from L1 and L2 WM tasks could mask what the scores represent; we suggest treating L1 and L2 WM task scores separately and correlating either with reading comprehension measures.
Finally, the correlation of L2 reading with L1 and L2 WM measures was of similar size across high- and low-proficiency learners. The results were not consistent with a prediction based on Alptekin and Erçetin (Reference Alptekin and Erçetin2010) that as L2 proficiency increases, the relationship between WM (measured in L2 WM tasks) and L2 reading would be similar to the one between WM (measured in L1 WM tasks) and L2 reading. One explanation is that differences between L2 proficiency levels in the current meta-analysis (low and high) might not have been sensitive enough to examine this prediction. Further, the number of primary studies included in the current meta-analysis was much larger for the low-proficiency group than the high-proficiency group (i.e., n = 38 vs. 6 for L2 WM tasks), which may have led to wide variability in correlations among the low-proficiency group (−.240 to .892 for L2 WM tasks). Future meta-analysis should use more finely classified groups consisting of a more equal number of primary studies across proficiency levels. This might clarify how the moderating effect of WM task language on the WM–L2 reading relationship varies across proficiency levels.
WORKING MEMORY TASK COMPLEXITY
WM assessed using both simple and complex span tasks, with or without a processing task, was related to L2 reading to a similar degree, suggesting that adding a processing task in a complex span task to measure information processing (e.g., through judgment of grammaticality/semantic acceptability of sentences in reading span tasks) does not produce larger WM–L2 reading correlations. The results did not concur with previous findings that complex span tasks were more strongly correlated than simple span tasks with L1 reading (Daneman & Merickle, Reference Daneman and Merikle1996) and L2 comprehension and production combined (Linck et al., Reference Linck, Osthus, Koeth and Bunting2014), perhaps partly because of differences in constructs measured in comprehension tests: L1 reading, L2 comprehension (including reading, vocabulary and grammar) and production combined, and L2 reading.
Another explanation for these different results may relate to different levels of reliability of tasks. As reviewed in the preceding text, reliable instruments likely better discriminate participants and yield larger correlations. To explore this possibility, we calculated median reliability of WM and reading tasks (see Table 2). We found that reporting reliability was not very high (8.11%–50.00%), and simple span tasks were as reliable as complex span tasks with processing tasks (.800 vs. .808); complex span tasks without processing tasks had slightly lower reliability (.600). The reliability results of L2 reading tasks were similar. Thus, it seems differential reliability values are unlikely to explain nonsignificant results across tasks with different WM complexity.
TABLE 2. Descriptive statistics of reliability of WM and reading comprehension tasks a
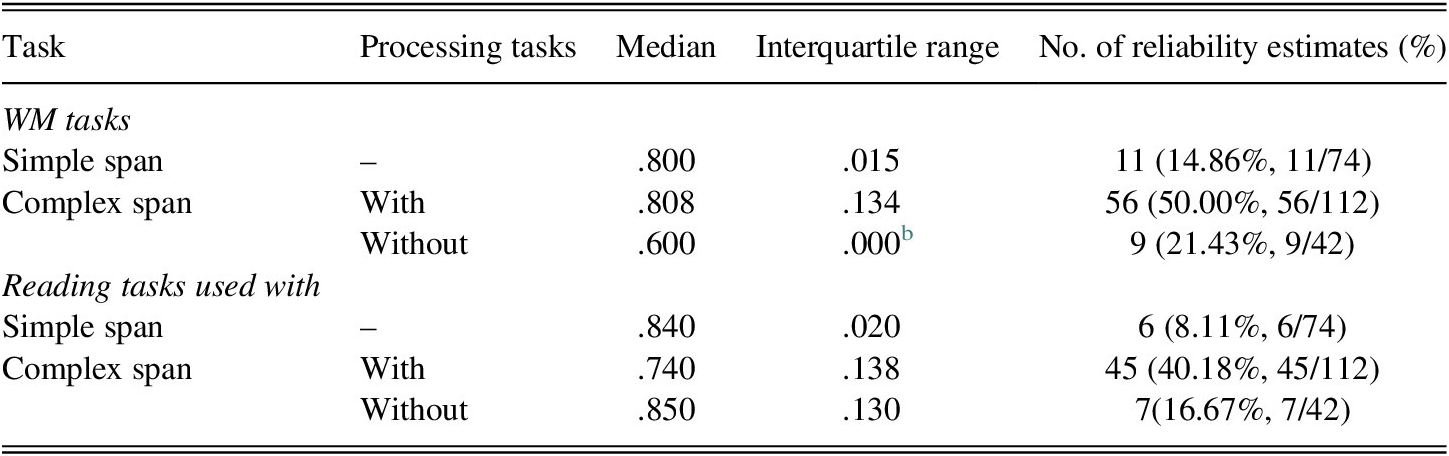
Note: % = Percentage of correlations that were reported with reliability. With/without = with or without processing task.
a When the backward digit span task was coded as a simple span task. The results were essentially the same when it was coded as a complex span task.
b Reliability was .600 in all nine datasets.
MODE OF WORKING MEMORY AND READING COMPREHENSION TASKS
No difference was observed in the average correlation between WM and L2 reading in terms of WM task mode (paper-based or computer-based); thus, WM tasks administered on paper or by computer were associated with L2 reading to a similar degree. The results suggest that delivery mode effects of WM tasks do not moderate the WM–L2 reading relationship.
For comprehension tasks, paper-based reading measures were as strongly correlated with WM measures as computer-based reading measures were. The results were the same as the WM task mode effects in the preceding text, suggesting that mode of L2 reading comprehension tasks—paper or computer—does not moderate the WM–L2 reading relationship. Note that this only goes for reading comprehension; learners’ processing may differ across modes and may influence the construct measured in each format. Mayes et al. (Reference Mayes, Sims and Koonce2001), for example, found that participants reading from a monitor, spent more time reading and comprehended less information than those reading on paper. Further, participants exhibited more time variation to finish reading from a computer than from paper (see Sawaki, Reference Sawaki2001 for summary of potential variables impacting reading). This could apply to tasks testing learners’ ability to understand long passages spanning pages on computers, going back and forth between pages while memorizing the question being asked, and looking for the answer in the text. These results suggest that computer-based reading tasks likely produce lower scores and elicit processes different from paper-based ones, less relevant to comprehension. However, these effects could be too subtle to be reflected in WM–L2 reading correlations, which might be similar in size but represent different processing of reading.
READING COMPREHENSION TASK STANDARDIZATION
L2 reading measured in standardized reading tasks correlated similarly with WM as did L2 reading measured in nonstandardized reading tasks. Thus, L2 reading comprehension task type did not moderate the WM–L2 reading relationship—consistent with Shin (Reference Shin2020). Additionally, this finding was not affected by reliability: Median reliability values were .725 (Interquartile range = .153, reliability reported = 32.08% [34/106]) for standardized reading tests and .760 (Interquartile range = .110, reliability reported = 18.85% [23/122]) for nonstandardized reading tests, respectively, suggesting that both tasks were equally precise indicators of reading comprehension. Nevertheless, reliability is merely one source of evidence for the validity of score interpretation. To better understand the degree to which reading comprehension was measured appropriately, other evidence regarding such as content and construct should be examined as well.
SCORING METHODS FOR COMPLEX SPAN
WM was similarly correlated with reading across scoring methods for reading span, suggesting an overall similar impact of scoring methods for WM complex span tasks on the WM–L2 reading relationship. For complex span tasks other than reading span, datasets were sparse. Recall + Processing Accuracy for listening span and operation span was the only scoring method with three or more correlations, and the results showed wide confidence intervals (e.g., −.802, .866 for operation span). Remaining methods had only one or two correlations—too few to compare scoring methods.
Although reading span scoring methods were not a significant moderator, the correlation between WM and L2 reading was larger when Recall + Processing Accuracy was used (r = .740) than others (r = .220 to .590). The correlation for Recall + Processing Accuracy was nevertheless nonsignificant, probably because of the wide range of the six correlations, including a negative one (r = −.087 to .892). The large correlation for Recall + Processing Accuracy was overall consistent with previous studies on the contribution of storage and processing components of WM to predicting reading comprehension (Daneman & Merikle, Reference Daneman and Merikle1996; Shin, Reference Shin2020), supporting the view of WM as limited resources shared between information processing and storage. Such trade-offs are likely well represented with a composite of storage and processing scores (Leeser, Reference Leeser2007). This also indicates that rigorously measuring WM may require a combination of (a) complex tasks with processing tasks and (b) the scoring method of Recall + Processing Accuracy.
Shin (Reference Shin2020) also compared three scoring methods for reading span and found that the WM–L2 reading relationship was the highest using (x) the scoring method including storage and processing accuracy (r = .33), followed by (y) the one including storage, processing accuracy, and processing speed (r = .30) and (z) the one including storage only (r = .20). In the current meta-analysis, the first method (i.e., [x]) corresponded to Recall + Processing Accuracy, Set Size, and Composite Z-Score 3 (r = .740, .295, and .220, respectively), the second (i.e., [y]) to Composite Z-Scores 1 and 2 (r = .299, .590, respectively), and the third (i.e., [z]) to Recall (r = .311). Comparison of the current results with Shin’s shows varied correlations across scoring methods for the same construct, indicating the importance of using an appropriate scoring method that fits research purposes.
RELIABILITY OF WORKING MEMORY SPAN TASKS AND L2 READING COMPREHENSION TASKS
When reliability of WM span tasks was not reported (r = .330 [.258, .398]), the synthesized WM–L2 reading correlation was larger than when it was (r = .232 [.192, .271]). This was unexpected; we had assumed that nonreporting of reliability of WM span tasks was likely due to their low reliability, leading to small WM–L2 reading correlations, as in Shin (Reference Shin2020; r = .30, with reliability reported, vs. r = .25, without). However, nonreporting of reliability values does not necessarily mean low reliability (Plonsky et al., Reference Plonsky, Marsden, Crowther, Gass and Spinner2020; Plonsky & Derrick, Reference Plonsky and Derrick2016). Studies not reporting reliability have been published in major journals such as Bilingualism: Language and Cognition, Developmental Psychology, Memory, and Reading and Writing. Thus, nonreporting can be inferred to be due to a field convention; these studies may have had high reliability and reflected the WM–L2 reading relationship well. In contrast, whether to report reliability of reading comprehension tasks was not related to the WM–L2 reading relationship; the synthesized correlations were both around .300 and numerically very similar (r = .282 and .309).
Although not reported so far, equally important are the reliability values of WM and reading tasks when these values are reported. Median reliability values were .798 (Interquartile range = .146, reliability reported = 33.33% [76/228]) and .742 (Interquartile range = .148, reliability reported = 25.00% [57/228]), respectively. Reported reliability estimates of WM span tasks in primary studies were satisfactory overall and varied little, suggesting that WM span tasks functioned just as well in L2 studies here as in the L1 studies in Waters and Caplan (Reference Waters and Caplan2003; e.g., .813 to .825). For reading tasks, reported reliability was satisfactory as well, suggesting that they functioned precisely as intended overall. For both WM and L2 reading tasks, median reliability values in our meta-analysis (.798 and .742) resembled those for instruments used in L2 research (.82 [Plonsky & Derrick, Reference Plonsky and Derrick2016]) and for grammatical judgment tasks in particular (.80 [Plonsky et al., Reference Plonsky, Marsden, Crowther, Gass and Spinner2020]). WM and reading tasks seem to work as reliably as other tasks in L2 studies, as shown by their reported reliability in the current meta-analysis.
An additional area requiring particular attention is how reliability estimates are reported for WM span tasks. Even when storage and processing tasks are separately correlated to comprehension tasks, separate reporting of reliability is uncommon. So far, reliability estimates have been reported separately for storage and processing tasks for only 14 correlations (12.50% [14/112]) from five studies (10.42% [5/48]) using complex tasks that included processing tasks. Of the five studies, three were conducted by the same authors. In the other studies, reliability was reported for storage and processing tasks combined. Note that among the abovementioned 14 correlations, the median reliability of storage tasks (.773, Interquartile range = .073) was higher than that of processing tasks (.663, Interquartile range = .108). Combined reporting could mask whether both tasks functioned precisely or if learners performed well in one task and poorly in the other. To minimize such impacts, researchers should report reliability separately for storage and processing tasks.
Finally, reliability of WM tasks and reading tasks was reported in one-quarter of studies―18 studies (27.03% [20/74]) and 20 studies (29.73% [22/74])—respectively, with 15 studies (20.27% [15/74]) reporting both. These percentages were similar to Shin’s (Reference Shin2020; 24.32% and 32.43% for WM and reading tasks) and within the extent of reported reliability, 6%–64%, in L2 subdomains in Plonsky and Derrick (Reference Plonsky and Derrick2016). They were favorable results, given that Juffs and Harrington (Reference Juffs and Harrington2011) stated that reliability has been rarely reported in L2 studies overall, but they still suggest that reporting reliability is uncommon in L2 WM studies. This is worrisome, as the correlation between two measures changes depending on their reliability and there is no assurance that reliability is sufficient when it is not reported. Specifically, the correlation cannot be large when two measures have low reliability (Conway et al., Reference Conway, Kane, Bunting, Hambrick, Wilhelm and Engle2005). Thus, small correlations may be due to weakly correlated constructs or low reliability of measures and do not necessarily mean constructs are not strongly related. Authors are encouraged to report the reliability of their measures. Using a formula of correction for attenuation or using structural equation modeling (SEM) are two viable options for considering measurement error, which will result in larger correlations. For example, Babayigit (Reference Babayigit2015) reported that the correlation between oral language (vocabulary, sentence repetition, and verbal WM) and word reading (text reading accuracy and single word reading) was large (r = .75) in SEM analysis, whereas simple correlations between observed variables of oral language and word reading were lower (r = .51 to .65). Reporting reliability and considering measurement error can help researchers better understand the WM–L2 reading relationship. This resonates with the call for methodological transparency and rigor by Plonsky et al. (Reference Plonsky, Marsden, Crowther, Gass and Spinner2020).
PROFICIENCY LEVELS
For low- and high-proficiency learners the WM–L2 reading relationship was similar, consistent with Linck et al. (Reference Linck, Osthus, Koeth and Bunting2014), suggesting an equally important role played by WM vis-à-vis comprehension across proficiency levels.
We analyzed whether and how WM task complexity (i.e., simple and complex tasks) interacted with proficiency levels when moderating the WM–L2 reading relationship. As Table 1 shows, we found no significant differences in correlations in simple tasks or complex tasks across proficiency levels. These results did not support Wen’s (Reference Wen2016) synthesis of previous findings, suggesting instead that both phonological and central executive components may be similarly important at early and at later stages of L2 development. This can be explained by articulatory rehearsal, a mechanism to which both components are related, which involves subvocally rehearsing incoming information to temporarily hold it in storage. Articulatory rehearsal is important for learners to recognize and decode written words automatically, so that text comprehension is enhanced and more resources can be directed to the central executive component to update, switch, and inhibit the information (e.g., Wen, Reference Wen2016). Regardless of passage/sentence length and difficulty and cognitive demands of the task on learners, they need to rehearse and remember passage/sentence content while engaging in the task. Thus, both phonological and central executive components may play important roles in reading for both lower and higher proficiency learners. WM task complexity does not seem to interact with proficiency levels when moderating the WM–L2 reading relationship.
However, the caveat discussed in the preceding text also applies here: The current meta-analysis employed a previously used but rough classification of L2 proficiency (low and high)—language of instruction, majors, and work environment. Moreover, we included fewer high-proficiency than low-proficiency learners. Thus, the impact of L2 proficiency and WM task complexity on WM–L2 reading relationships should be reexamined after more primary studies are conducted.
CONCLUSION
This study examined the WM–L2 reading comprehension relationship using meta-analysis. For the first research question, regarding the WM–L2 reading relationship, we found a significant, small relationship. For the second research question, how this relationship was moderated by task and learner variables, WM task language (L1 or L2) and reporting of the reliability of WM span tasks moderated the WM–L2 reading relationship. Methodological features of reading comprehension measures or learner proficiency did not moderate the relationship, suggesting that WM measurement practices may be more important than L2 reading measures or learner characteristics in understanding the WM–L2 reading relationship.
While we searched for relevant studies, both published and unpublished, and obtained 74, more primary studies are needed before conducting comprehensive moderator variable analyses. We originally intended to include other potentially interesting variables, but they were often not reported and could not be included. For example, Leeser (Reference Leeser2007) examined how the WM–L2 reading relationship was moderated by topic familiarity and found that in L2 reading recall tasks, high- and medium-WM groups that read familiar texts outperformed high-, medium-, and low-WM groups that read unfamiliar texts, suggesting that WM facilitates reading comprehension only when one is familiar with the text topic. Thus, the WM–L2 reading relationship seems to be moderated by topic familiarity. Such relationships can be meta-analyzed if reported in primary studies.
Our meta-analysis has confirmed findings of previous meta-analyses (Jeon & Yamashita, Reference Jeon and Yamashita2014; Linck et al., Reference Linck, Osthus, Koeth and Bunting2014; Shin, Reference Shin2020) and showed how moderator variables affect the WM–L2 reading relationship. Our research has expanded on previous studies by carefully examining moderator variables. It merits further examination and replication, especially for moderator variables represented in relatively few studies. As discussed in Plonsky and Oswald (Reference Plonsky, Oswald and Plonsky2015) and In’nami et al. (Reference In’nami, Koizumi, Tomita, McKinley and Rose2020), such an iterative process would lead to greater accumulated knowledge. Such a process is necessary for further research into the WM–L2 reading relationship.