Traditional dietary assessment techniques
For more than 100 years, nutritional research has played an important role for our understanding of diet–health relations in the human body. Nutrition researchers have contributed substantially to meaningful ways of improving health of individuals as well as populations. Valid and reproducible dietary assessment has been the cornerstone of this work. Food diaries, 24 h recalls and FFQ are commonly used self-report dietary assessment methods in human studies of nutrition in relation to health( Reference Willet 1 ). Multiple days, i.e. 7 d, weighed food records, the so-called gold standard dietary assessment method, are often used in controlled dietary studies( Reference Cameron and van Staveren 2 ). However, dietary records are very time consuming and labour intensive for both participants and researchers, and they are not very accurate( Reference Nes, Frost Andersen and Solvoll 3 , Reference Yuan, Spiegelman and Rimm 4 ). Therefore, they are not commonly used in large studies. As an alternative, 24 h recalls, i.e. a structured interview aiming to collect detailed information about all food items consumed during the preceding 24 h, are more frequently used in research settings. A major drawback of 24 h recalls is that 2–3 recalls are often not sufficient to capture the day-to-day variation of a variety of nutrients and foods such as vitamin A, vitamin C, cholesterol and fish( Reference Willet 1 ). Therefore, the use of 24 h recalls is often considered to be too time- and labour-intensive for use in large studies( Reference Beaton, Milner and McGuire 5 ). For larger studies, self-administered FFQ are most often the method of choice and are generally used to evaluate food consumption during the past 1–12 months. In contrast to food records and recalls, FFQ require an intensive preparation before the method is applied in actual studies, but the administration and processing of a validated FFQ can be done rather efficiently for several thousand participants at once. However, the large supply of available foods, inaccurate estimation of portion sizes, socially desirable answers and errors in food composition tables also make FFQ prone to measurement error( Reference Cade, Thompson and Burley 6 – Reference Johansson, Solvoll and Bjorneboe 9 ). A common concern with self-reported dietary intake is the objectivity and accuracy of self-reported data.
Nutritional biomarkers
The limitations of the present dietary measurement tools have motivated many researchers to search for nutritional biomarkers as a complementary or alternative measure of dietary intake. Objective biological food intake and nutrient intake biomarkers may provide valuable information beyond self-reported food intake data and are particularly valuable when food composition data are not available or limited. Unfortunately, there are still very few well-validated nutritional intake biomarkers, with the exception of urinary nitrogen for protein, potassium and sodium( Reference Jenab, Slimani and Bictash 10 ); carotenoids for vegetables and fruits( Reference Brevik, Andersen and Karlsen 11 , Reference Al-Delaimy, Ferrari and Slimani 12 ); and n-3 fatty acids for oily fish( Reference Saadatian-Elahi, Slimani and Chajes 13 ). These examples of the well-validated food intake biomarkers are all natural food constituents. Researchers also explore the value of nutritional biomarkers reflecting food additives, nutritional biomarkers that are the result of the digestion or absorption of foods, and endogenous metabolites that are affected by the consumption of certain foods and as such may act as nutritional biomarkers. Previous research has for instance highlighted significant associations between fish consumption and biomarkers of mercury, arsenic and polychlorinated biphenyl( Reference Miklavcic, Casetta and Snoj Tratnik 14 , Reference Philibert, Schwartz and Mergler 15 ). Moreover, the Dutch National Institute for Public Health and the Environment and the University of Ghent are presently focusing part of their research on mycotoxins, a food contaminant where dietary exposure represents the main exposure( Reference Marin, Ramos and Cano-Sancho 16 ). During the past 3 years, two draft calculators have been developed to study associations between dietary mycotoxin intakes and urinary mycotoxin concentrations. Furthermore, these two research centres are conducting an intervention study in which volunteers are exposed to a single dose of a rapidly eliminated mycotoxin (i.e. deoxynivalenol), after which urine is collected quantitatively at different time intervals up to 24 h after administration.
New metabolomics techniques
Recent developments in analytical chemistry suggest that much more useful food intake biomarkers can and should be identified. New metabolomics techniques provide a unique opportunity. Using metabolomics techniques, it is possible to measure up to thousands of metabolites at once providing valuable information on the food metabolome (i.e. essential and non-essential chemicals derived from foods after digestion and subsequent metabolism by the tissues and the microbiota)( Reference Scalbert, Brennan and Manach 17 ), using plasma( Reference Lawton, Berger and Mitchell 18 ), serum( Reference Psychogios, Hau and Peng 19 ), erythrocytes and leucocytes( Reference Norheim, Gjelstad and Hjorth 20 ), urine( Reference Bouatra, Aziat and Mandal 21 ), saliva( Reference Dame, Aziat and Mandal 22 ), faeces( Reference Deda, Chatziioannou and Fasoula 23 ), cerebrospinal fluid( Reference Wishart, Lewis and Morrissey 24 ), as well as hair( Reference Sulek, Han and Villas-Boas 25 ). Metabolomics techniques do not only allow identification of numerous biomarkers at once, but also to conduct studies where we may account more precisely for food–food interactions. Moreover, these new techniques provide the opportunity to more easily use combinations of biomarkers to predict food intakes( Reference Souverein, de Vries and Freese 26 ). Commonly used platforms for food metabolomics analyses are NMR spectroscopy( Reference Pan and Raftery 27 ), liquid chromatography–MS and GC–MS( Reference Kanani, Chrysanthopoulos and Klapa 28 , Reference Egert, Weinert and Kulling 29 ). Advantages of NMR include its high reproducibility, short sample preparation time and small interlaboratory variability( Reference Dettmer, Aronov and Hammock 30 , Reference Laghi, Picone and Capozzi 31 ). Conversely, NMR only allows identification of a limited number of metabolites as compared to liquid chromatography–MS and GC–MS( Reference Dettmer, Aronov and Hammock 30 , Reference Martin, Dumas and Wang 32 ). MS techniques allow identification of many more metabolites, but tissue samples require more preparation and the overall throughput is lower( Reference Dettmer, Aronov and Hammock 30 ). Given the pros and cons of the different platforms, a multiplatform approach is preferred to identify a broad range of metabolites( Reference Bub, Kriebel and Dorr 33 , Reference Scalbert, Brennan and Fiehn 34 ).
Between- and within-person variability in food intake biomarkers
During identification of novel food intake biomarkers, one needs to be aware that metabolite concentrations may be influenced by genetic factors, different rates of digestion, absorption, metabolism, and excretion( Reference Brown, Ferruzzi and Nguyen 35 ), smoking( Reference Brude, Finstad and Seljeflot 36 ), body composition( Reference Haugen and Drevon 37 ), lifestyle( Reference Vinknes, Elshorbagy and Nurk 38 ), drug use( Reference van Orten-Luiten, Janse and Dhonukshe-Rutten 39 ) and geographical location( Reference Walsh, McLoughlin and Roche 40 ). To illustrate, random plasma 13C concentrations have been shown to be highly correlated with cane sugar/high fructose maize syrup consumption of the preceding meal (R 2 = 0·90)( Reference Cook, Alvig and Liu 41 ). However, these concentrations only reflect very recent intakes that are probably not detected when studying fasting concentrations. Additionally, although red meat consumption has been significantly associated with creatinine( Reference Cross, Major and Sinha 42 ), creatinine is also a product of muscle catabolism. Red wine consumption has been associated with various resveratrol metabolites( Reference Rotches-Ribalta, Andres-Lacueva and Estruch 43 ). However, also in this example, between-person variability should be considered due to for instance the production of resveratrol metabolites by colonic microflora( Reference Manach, Scalbert and Morand 44 ). Ideally, a robust food intake biomarker is not markedly influenced by such factors, but in any case such aspects need to be taken into account in the validation process of food intake biomarkers. However, a general validation system for food intake biomarkers is still lacking and better guidance for biomarker validation is needed. During the past years, a system to score food intake biomarker quality and validity has been developed, allowing researchers to evaluate potentially interesting food intake biomarkers for standard analytical quality control along with criteria related to biomarker kinetics (dose–response, time–response), metabolic and other host factor effects, food matrices, and specificity for the actual foods (Q Gao, G Praticò, A Scalbert et al., unpublished results). In addition to the aforementioned effort, the University Medical Centre Groningen and Dutch National Institute for Public Health and the Environment are investigating to what extent food intake biomarkers are biased by within-person variation, and if so, whether correction for within-person variation improves the estimate of the proportion of people with less than adequate or excessive intakes.
The Food Biomarkers Alliance
It is clear that food metabolomics is a complex discipline requiring the expertise and facilities in nutrition, metabolomics, epidemiology, clinical science, analytical chemistry, molecular biology, food sciences, bioinformatics and statistics. Therefore, a large group of experts in food metabolomics decided to join forces in the Food Biomarkers Alliance (FoodBAll), which is a Joint Project Initiative ‘A Healthy Diet for a Healthy Life’ (JPI-HDHL). This is a nationally funded collaboration between twenty-four partners from thirteen countries (Belgium, Canada, Denmark, Finland, France, Germany, Ireland, Italy, Norway, Spain, Sweden, Switzerland and the Netherlands), aiming to identify and validate food intake biomarkers of commonly consumed foods in Europe that can improve food intake assessments. FoodBAll started its funded activities in December 2014 and will continue for 3 years up to mid-2018 (http://foodmetabolome.org/)( 45 ). FoodBAll aims to systematically explore and validate food intake biomarkers to provide a better assessment of the food intake in different European regions. In order to identify and validate new food intake biomarkers, the FoodBAll team decided to focus on the following aspects of food intake biomarker research: (1) discover novel food intake biomarker by means of extensive systematic literature reviews, observational studies and acute intervention studies; (2) develop a validation scoring system for food intake biomarkers and its application to new biomarkers; (3) explore and validate alternative/less-invasive food intake biomarker sampling techniques; (4) develop tools for identification of food intake biomarkers and online resources to facilitate sharing of food intake biomarker data and resources with the (scientific) community; (5) explore biological health effects of food intake biomarkers; (6) describe the value of food intake biomarkers for authorities and stakeholders. In order to align all the work from many different disciplines, a food intake biomarker was defined as being a specific measurement in a biological specimen accurately reflecting the intake, selective and dose-dependent, of a food constituent or food. In contrast to a food intake biomarker, a nutritional biomarker relates to nutrients and can be any biological indicator of nutritional status linked either to intake, metabolism or effect of a nutrient.
Present actions on novel food intake biomarker discovery
Present scientific literature
During the past decades already numerous studies have been conducted that used dietary biomarkers or studied potentially new dietary biomarkers( Reference Norheim, Gjelstad and Hjorth 20 ). A French-Canadian research collaboration extracted and organised the available data for all the nutritional biomarkers used so far in scientific studies in the Exposome-Explorer database( Reference Neveu, Moussy and Rouaix 46 ). Compounds and nutrients included in the database are for instance kaempferol, isorhamnetin, m-coumaric acid and phloretin for apples; naringenin for grapefruit; carotenoids, lycopene and lutein for tomato; 5-heneicosylresorcinol, 5-tricosylresorcinol and alkylresorcinols for whole-grain wheat; daidzein, genistein, isoflavones and O-desmethylangolensin for soya products; and iodine, margaric acid, pentadecylic acid and phytanic acid for dairy products( Reference Scalbert, Brennan and Manach 17 , Reference Neveu, Moussy and Rouaix 46 ). For a full overview of the identified metabolites presented in the Exposome-Explorer database, please visit the website (http://exposome-explorer.iarc.fr)( Reference Neveu, Moussy and Rouaix 46 ). The FoodBAll research team is also presently reviewing the state of the art of the present food intake markers to expand the Exposome-Explorer database. All major food groups are addressed, including alcoholic beverages, food of animal origin, fruit and vegetables, cereals and wholegrain, fats and oils, legumes, non-alcoholic beverages, confectionary, and spices and herbs. Most of the single foods within these groups are also reviewed.
Application of new food metabolomics techniques in observational studies
Future studies to expand the present scientific literature are warranted. Observational studies collecting information on dietary intake and biological samples studies may provide valuable data to identify new (long-term) food intake biomarkers( Reference Scalbert, Brennan and Manach 17 ). A commonly used approach in these types of studies is to rank participants according to their food intakes( Reference Pujos-Guillot, Hubert and Martin 47 , Reference Madrid-Gambin, Llorach and Vazquez-Fresno 48 ) and explore associations with metabolites identified by the metabolomics platform of choice. As an example, Madrid-Gambin et al. recently applied urinary NMR in an observational dataset of the PREDIMED study to describe the fingerprinting of dietary pulses, where dietary pulse intakes were assessed with an FFQ and classified as non-pulse and habitual pulse consumers( Reference Madrid-Gambin, Llorach and Vazquez-Fresno 48 ). This approach revealed differences for sixteen metabolites coming from choline pathways, protein-related compounds and energy metabolism, and hence provided leads for potentially novel food intake biomarkers for pulse intake( Reference Madrid-Gambin, Llorach and Vazquez-Fresno 48 ). An extensive overview of similar studies available in the present literature can be found in a recent review by Manach et al. ( Reference Manach, Brennan and Dragsted 49 ). The FoodBAll consortium researchers aim to expand the literature on this matter by conducting similar studies using plasma and urine biobanks of cross-sectional and longitudinal cohorts with extensive dietary intake data. Altogether these databases include 197 000 individuals, men and women of all ages, from the general population to selected populations such as obese subjects and former cancer patients. A limitation of this approach is that high correlations may exist between the various foods consumed. Hence, associations may appear that are not the result of the food analysed, but of a highly correlated other food( Reference Scalbert, Brennan and Manach 17 ).
Application of new food metabolomics techniques in (acute) intervention studies
Controlled acute dietary intervention studies are assumed to be less sensitive to confounding by correlated foods and therefore provide an important additional source of information in the field of food intake biomarker discovery. Gibbons and Brennan recently published an extensive overview of intervention studies using a metabolomics approach( Reference Gibbons and Brennan 50 ). In addition, the same research group recently successfully predicted citrus intakes in the Irish National Adult Nutrition Survey by urinary proline betaine using calibration curves obtained from an acute intervention study( Reference Gibbons, Michielsen and Rundle 51 ). A German research group also just published a randomised crossover intervention study on the effect of dietary non-fermentable and fermentable fibre (i.e. cellulose and inulin), and propionate (i.e. major product of the fermentation of fibres) on odd-chain fatty acid concentrations, showing significant effects of inulin and propionate, but not cellulose( Reference Weitkunat, Schumann and Nickel 52 ). To contribute to this research area, seven study centres are presently conducting well-defined standardised short-term intervention studies. All study centres follow the same design, exploring potential food intake biomarkers of fourteen foods, including sugar-sweetened beverage, apple, tomato, banana, milk, cheese, bread, meat/meat products, red meat and white meat, potato, carrot, peas, lentils, beans and chickpeas (Fig. 1). Participants are exposed to a test meal after which urine and blood samples are collected over a time-course up to 24 h with the option to continue to 48 h. Collected samples are analysed by a multiplatform approach, including untargeted liquid chromatography–MS, GC–MS and NMR. The first results of these studies have just been published, which pointed towards lactose, galactose, and galactonate as potential biomarkers of milk intake, urinary 3-phenyllactic acid of cheese intake, and pinitol and trigonelline of soya drinks( Reference Munger, Trimigno and Picone 53 ). Moreover, FoodBAll researchers are conducting metabolomics analyses using previously collected data from intervention studies. FoodBAll has access to fifty-four human intervention studies with biobanks of more than 14 000 men and women from age 8 to 95 years, including up to twenty different foods (e.g. dairy, meat, apples, cocoa, Nordic diet) and more than fifteen different nutrients or compounds (e.g. vitamin C, vitamin B12 and polyphenols). Although intervention studies are assumed to be less sensitive to detect food–metabolite associations confounded by other correlated foods, the highly controlled character of these studies may result in identification of metabolites that are not specific enough for the food under investigation when explored in free-living human subjects (i.e. population-based studies)( Reference Scalbert, Brennan and Manach 17 ).
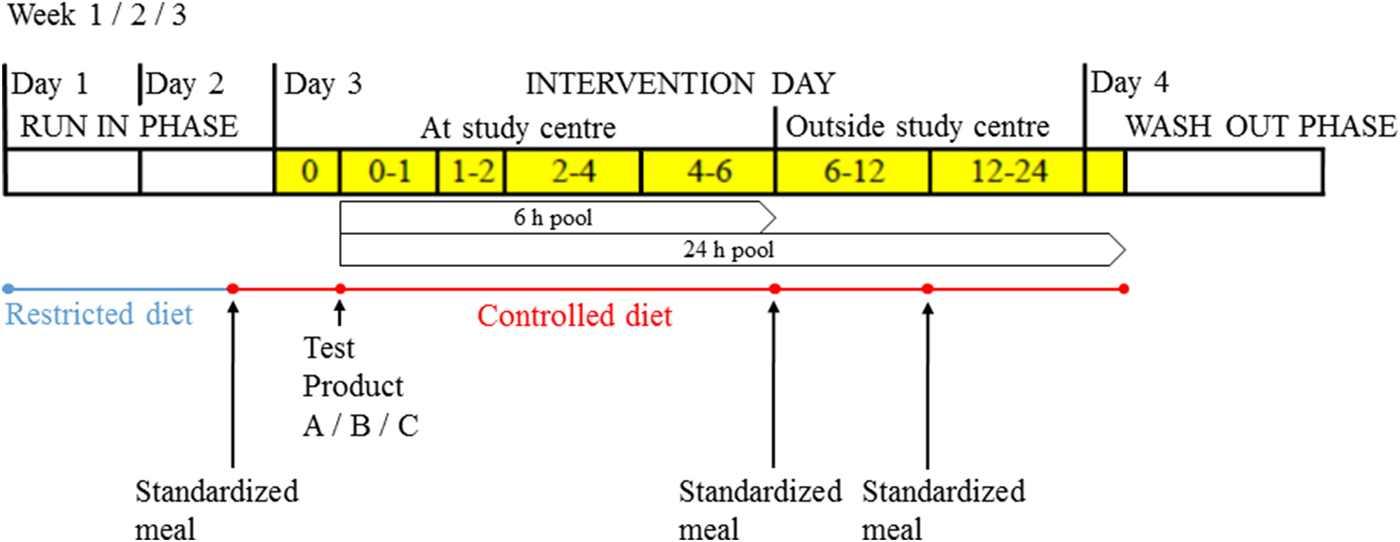
Fig. 1. (Colour online) Design of the randomised, controlled, crossover studies as conducted as part of the Food Biomarkers Alliance (FoodBAll) project (adapted from Munger et al. ( Reference Munger, Trimigno and Picone 53 )). Test products are administered in random order and urine samples are collected before and after ingestion of test product during defined intervals (yellow blocks) up to 24 h; 6 and 24 h pools are later prepared in the laboratory.
Novel alternative/less-invasive food intake biomarker sampling techniques
Despite many potential advantages of food intake biomarkers, they may also be costly and more invasive than self-reported dietary assessment methods. To date, venous blood sampling is the most commonly used approach to collect biological samples from human volunteers. This is a time-consuming process that requires well-trained personnel. Recent technological developments allow us to conduct food intake biomarker analyses with high sensitivity requiring very small blood volumes or tissue samples( Reference Lakshmy, Tarik and Abraham 54 – 56 ). Combining these novel and sensitive techniques with non-professional biological sampling (e.g. blood collection by finger-pricking or filters/sticks) offers the opportunity to collect biological data without the employment of health professionals. Recently, an extensive literature review has been performed summarizing the present evidence with respect to food intake biomarkers from different body fluids, e.g. blood, leucocytes, plasma, serum, urine, stool, saliva, sweat, hair, nails, skin, adipose tissue and skeletal muscle( Reference Holen, Norheim and Gundersen 57 ). It is feasible to collect and submit dried blood spot samples via regular mail in large epidemiological settings with more than 70 % response rates similar to saliva collection( Reference Sakhi, Bastani and Ellingjord-Dale 58 ). Documentation of dried blood spot application on biomarkers is available for fatty acids( Reference Albani, Celis-Morales and O'Donovan 59 ), 25-hydroxyvitamin D( Reference Avagyan, Neupane and Gundersen 60 ) and 8-epi-Prostaglandin F2α ( Reference Bastani, Gundersen and Blomhoff 61 ). Presently, the FoodBAll consortium continues the work on alternative food intake biomarker sampling techniques by conducting validation studies on the use of dried blood spot and Mitra sticks for the analyses of lipid biomarkers as well as different nutrients, including sodium, magnesium, potassium, calcium, iron, cupper, zinc, selenium and iodine.
Bridging dietary biomarkers to health pathways
In order to strengthen evidence for the application of intake/health efficacy biomarkers, future research should aim to relate food intake biomarkers to diet-related disease risk by (1) taking a pathway- and network-based bioinformatics approach and (2) building on existing transcriptomic and metabolomics data( Reference Afman, Milenkovic and Roche 62 ). O'Gorman et al. recently applied this approach in the Metabolic Challenge Study and identified twenty-two lipid biomarkers that were associated with total dietary fat intake( Reference O'Gorman, Gibbons and Ryan 63 ). Five of these twenty-two lipid biomarkers were significantly elevated in participants with increased risk of the metabolic syndrome( Reference O'Gorman, Gibbons and Ryan 63 ). Wittenbecher et al. also applied a metabolomics approach and identified six out of twenty-one potential biomarkers for red meat consumption to be associated with type 2 diabetes( Reference Wittenbecher, Muhlenbruch and Kroger 64 ). A Spanish group observed urolithin A glucuronide as the most discriminant marker of nuts consumption, which in turn showed a significant inverse correlation with abdominal overweight and glycaemia( Reference Mora-Cubillos, Tulipani and Garcia-Aloy 65 ). The FoodBAll consortium is presently conducting additional studies on the relevance and value of transcriptomics and metabolomics data patterns relating dietary intake with health.
Online (food) metabolomics databases
Although many food compounds can be found in human bio specimens and detected in metabolomic profiles, the interest in food metabolomics is relatively new, and annotation of the food metabolome is still very limited as compared to annotation of the endogenous metabolome. Thus, identification and validation of potentially new food intake biomarkers using metabolomics techniques requires knowledge of presently available electronic resources, such as food composition, compound and biomarker databases, libraries of spectra, software tools and various online tools for food metabolome profiles annotation and interpretation. A new public web portal developed by the FoodBAll consortium has been launched in 2017 that provides descriptions, links and tutorials for more than fifty selected databases, spectral libraries, software programmes, and online tools useful for food metabolome profiles annotation and food intake biomarker discovery( 45 ). One of the major online resources is the human metabolome database, which contains detailed data about small molecule metabolites identified in the human tissues( Reference Wishart, Jewison and Guo 66 ). The most comprehensive metabolome database specifically focusing on the food compounds is FooDB (http://foodb.ca/), covering more than 25 000 native food compounds, food additives and man-made compounds also formed during food processing with their dietary sources (>900 foods)( Reference Wishart 67 ). However, online databases are still incomplete, especially regarding the human metabolites of all non-nutrients present in foods. Initiatives are ongoing to manually collect information available in the literature and to add in silico predicted metabolites of all food components. PhytoHub is a compound database focused on food phytochemicals and their human metabolites manually extracted from the literature, initially built by Institut national de la recherche agronomique for nutrimetabolomics studies( 68 ). Besides databases useful for annotation in metabolomics, International Agency for Research on Cancer (France) and University of Alberta developed the Exposome-Explorer database on biomarkers of environmental exposure (http://exposome-explorer.iarc.fr/) in order to compare the performance between biomarkers and their fields of application( Reference Neveu, Moussy and Rouaix 46 ). Exposome-Explorer contains data on many attributes related to 142 dietary biomarkers extracted, such as type of exposure, biomarker concentrations in various human body fluids or tissue and associated analytical techniques, biomarker reproducibility over time, population characteristics, study design and with references to publications. FooDB, PhytoHub and Exposome-Explorer are being further developed within the FoodBall project to better meet all needs for the discovery and validation of new nutritional biomarkers. The FoodComEx ‘Food Compound Exchange’ may be another valuable online source for metabolomics researchers. FoodComEx is a chemical library recently developed by FoodBAll partners that aims to facilitate the sharing of standards of food compounds, their metabolites and other reference materials( 69 ). These standards are essential for the validation or identification of candidate biomarkers in metabolomics studies. An interface has been developed that facilitates the exchange between researchers interested in using a specific compound and researchers owning that specific compound. Presently, about forty rare food-derived metabolites and 1005 commercial compounds are included in this database. The database will be further extended by attracting more contributors and as such more compounds. Furthermore, additional compounds will be produced by using in vitro (microbial fermentation, liver microsomes and isolated enzymes) and in vivo (rodents) systems to ‘metabolise’ food chemicals.
Conclusion
Food intake biomarkers provide a valuable alternative/addition to traditional self-reported dietary intake assessment methods. However, although many biomarkers have been described, only relatively few are sufficiently validated and accepted as food intake biomarkers. In the identification of new potential biomarkers of food intake, many factors need to be considered, such as metabolism and lifestyle. Additionally, the specificity of the metabolite for the food under study needs to be evaluated, where also the increasing use of fortified foods and supplements should be considered. By joining international multidisciplinary expertise, the FoodBAll consortium intends to contribute to the discovery of new food intake biomarkers, using novel metabolomics techniques. Ultimately this effort aims to facilitate three main applications, such as (1) validation/adjustment of self-reported dietary assessment tools, (2) serve as markers of compliance in intervention studies and (3) improve the value of many observational studies investigating nutrition and health associations by providing less biased intake estimates.
Acknowledgements
All FoodBAll collaborators and the EU Joint Programming Initiative ‘A Healthy Diet for a Healthy Life’ are gratefully acknowledged.
Financial support
This work was financially support by the EU Joint Programming Initiative ‘A Healthy Diet for a Healthy Life’ on Biomarkers BioNH FOODBALL (grant number 529051002); the German Federal Ministry of Food and Agriculture through the Federal Office for Agriculture and Food (grant numbers 2814ERA01E, 2814ERA02E, 2814ERA03E); the Canadian Institutes of Health Research; the Spanish National Grants from the Ministry of the Economy and Competitiveness (PCIN-2014-133-MINECO Spain), the Generalitat de Catalunya's Agency AGAUR (2014SGR1566), the CIBERFES (co-funded by the FEDER Program from EU); the Research Foundation Flanders (grant number G0D4615N); the Agence Nationale de la Recherche (#ANR-14-HDHL-0002-02); the Italian Ministry of Agriculture, Food and Forestry Policies (MiPAAF) within the JPI-HDHL (MIUR D.M. 115/2013); the Swiss National Science Foundation (40HD40_160618) in the frame of the national research programme ‘Healthy nutrition and sustainable food protection’ (NRP69)’; the Danish Innovation Foundation (4203-00002B); the Science Foundation Ireland (SFI 14/JPI-HDHL/B3076 & SFI 11/PI/1119); and the Norwegian Research Council (246413 JPI-HDHL: The FoodBAll).
Conflict of interest
C. A. D. is a cofounder, board member, stock-owner and consultant for Vitas AS (http://vitas.no) performing the dried blood spot analyses.
Authorship
E. J. M. F., L. B., C. A. D., L. O. D., C. M., H. M. R. and H. van K. are the work-package leaders of the FoodBAll, and responsible for the design and conduct of the project. C. A.-L., S. J. L. B., J. B., L. B., F. C., S. D. S., L. O. D., C. A. D., T. E. G., H. van K., M. K., R. L., J. L., C. M., F. M., R. P. M., H. M. R., A. S., S. K., C. S., T. S., I. T., G. V., D. W. and E. J. M. F. are principal investigators at the partaking institutes. E. M. B.-B. and E. J. M. F. drafted the manuscript. All authors provide feedback on the draft manuscript and approved the final manuscript.