Introduction
Birth weight is widely recognized as a fundamental determinant of health status. Notably, low birth weight (LBW, birth weight < 2,500 g) is associated with worsened short- and long-term prognoses in some diseases. In this regard, LBW is associated with poor mortality and neurodevelopmental outcomes Reference Walker and Marlow1 as well as the risk of developing non-communicable diseases (NCDs), such as cardiovascular disease, diabetes, and hypertension in adulthood. Reference Eriksson, Forsén, Tuomilehto, Osmond and Barker2–Reference Kanda, Murai-Takeda, Kawabe and Itoh6 The average birth weight in the Japanese population is decreasing, resulting in increasing prevalence of LBW infants, Reference Takemoto, Ota, Yoneoka, Mori and Takeda7–Reference Yorifuji, Naruse and Kashima9 which represents a significant concern in Japan and worldwide. The reproductive outcome of LBW in female individuals has gained attention and emerging evidence indicates that LBW is associated with pregnancy complications. Maternal LBW reportedly carried an increased risk of hypertensive disorders of pregnancy (HDP), gestational diabetes mellitus (GDM), Reference Wagata, Ishikuro and Obara10–Reference Seghieri, Anichini and De Bellis12 and preterm delivery (PTD, the most common pregnancy complication). Reference Selling, Carstensen, Finnström and Sydsjö13
PTD (childbirth before 37 weeks of gestation) occurs in approximately 10% of women during pregnancy, and its number (currently estimated to be 15 million) is increasing worldwide. Reference Beck, Wojdyla and Say14,Reference Chawanpaiboon, Vogel and Moller15 Children born preterm have higher risks of neonatal mortality, morbidity, and long-term adverse consequences, including neurodevelopmental and respiratory diseases. Reference Huddy16–18 As medical and educational costs and lost productivity associated with preterm birth are estimated to be >26.2 billion USD in the United States of America alone, Reference Petrou19–Reference Kim, Andronis and Seppänen21 reducing PTD is an urgent priority. Although some PTD risk factors, such as gestational hypertension, intrauterine infections, uterine tumors, morphological abnormalities of the uterus, and assisted reproduction technologies (ART), have been identified, Reference Tamura, Hanaoka and Ito22–Reference Ohmi, Hirooka and Mochizuki24 other potential determinants of PTD remain unexplored.
The associations between maternal birth weight (MBW), including LBW and small for gestational age (SGA), and PTD have been explored; however, the outcomes are controversial. Reference Selling, Carstensen, Finnström and Sydsjö13,Reference Klebanoff, Schulsinger, Mednick and Secher25–Reference Emanuel, Leisenring and Williams27 These studies were conducted in Western countries, and thus, the Asian population was not included. Recently, a Japanese single-center cohort study revealed that MBW was inversely associated with birth delivery to smaller babies (LBW and SGA) but not with PTD. Reference Shibata, Ogawa and Kanazawa28 Here, we aimed to investigate the association between MBW and PTD using a nationwide prospective birth cohort study. We hypothesized that maternal LBW represents a higher risk of PTD, even after adjusting for numerous variables.
Method
Study design and participants
This study was a cross-sectional study within the Japan Environment and Children’s Study (JECS), an ongoing prospective birth cohort study, this investigation utilized data of approximately 100,000 pairs of pregnant women and their children from 15 Regional Centres across Japan since 2011. The main objective of the JECS is to identify environmental factors that influence children’s growth and development from the fetal period through childhood. The JECS protocol was reviewed and approved by the Ministry of the Environment’s Institutional Review Board for epidemiological studies and by the Ethics Committees of all participating institutions. The JECS was also conducted in accordance with the Declaration of Helsinki. Details of the study protocol of the JECS were previously reported. Reference Kawamoto, Nitta and Murata29,Reference Michikawa, Nitta and Nakayama30 Pregnant women were recruited at 15 Regional Centres between January 2011 and March 2014 in the JECS.
As shown in the study design paper, to ensure that the results of the JECS could be considered relevant for the general Japanese population, 15 Regional Centres from which mothers were recruited were selected from each region to cover the whole of Japan Reference Kawamoto, Nitta and Murata29 Each Regional Centre consisted of one or more study areas. The study sites varied from urban to rural areas. They also included areas with a variety of characteristics, including agricultural, fishing, commercial, and industrial lands. The Regional Centres were selected after each university or research institute was asked to propose their target areas, populations, methods for recruiting research participants, organizational structure, regional contacts, and resources, and after evaluating these proposals. The goal was to cover half of all births in the target region, with the aim of recruiting at least half of all eligible mothers for the study. The coverage rate (the number of births registered in JECS divided by the total number of births in the study area) for the participating regions in 2013 was approximately 45%. Reference Michikawa, Nitta and Nakayama30 Recruitment was by one or both of the following two methods.
1. Recruitment at the time of the first prenatal examination at medical institutions cooperating in the survey.
2. Recruitment at municipalities that publish Mother-Child Health Handbooks (an official booklet distributed free of charge to all expectant mothers in Japan and necessary to receive municipal services related to pregnancy, childbirth, and childcare).
Consent to participate in the study was obtained in writing from the mothers and their partners. Even once the consent was provided, participants could withdraw it at any time. Study exclusions were those who did not consent to the study protocol and could not be contacted during pregnancy. It is common for mothers to return to their parents’ homes to give birth in Japan. Mothers who planned to return to their parents’ homes to deliver were excluded from the study, except for those who could be contacted by the Regional Centres. Although every effort was made to contact as many mothers as possible in the study area and the study participants were carefully selected, the JECS survey was not a completely random survey. After written informed consent was obtained from all participants, questionnaires were administered twice to collect maternal information during the first trimester (MT1) and the second or third trimester (MT2) of pregnancy. Additionally, the questionnaire was administered 6 months after delivery (C6m). The study analyzed the “jecs-ta-20190930” dataset, which was released by the Programme Office in October 2019.
MBW
Information on MBW in this study was collected from the question “How many grams were you born with?” in the questionnaire C6m. However, data on the mother’s delivery weeks were not collected in the JECS. With reference to previous studies, MBW was classified into five categories as follows: < 2,500 g, 2,500–2,999 g, 3,000–3,499 g, 3,500–3,999 g, and ≥ 4,000 g. Reference Shibata, Ogawa and Kanazawa28,Reference Andraweera, Dekker and Leemaqz31 MBWs of < 500 g or > 6,000 g were treated as improbable data in this study. Reference Wagata, Ishikuro and Obara10,32
Definition of PTD, early PTD, and late PTD
Information on delivery week was transcribed from medical records in this study. Expected date of confinement, a basis of gestational age, was determined based on last menstrual period, crown rump length measured ultrasonography, or date of transfer of embryo in the case of ART pregnancies. PTD was defined as delivery from 22 to < 37 weeks of gestation. Early and late PTD were defined as deliveries from 22 to < 34 weeks of gestation and from 34 to < 37 weeks of gestation, respectively. Reference Tsuji, Shibata and Morokuma33–Reference Machado, Passini Júnior and Rodrigues Machado Rosa35
Data collection for other variables
Maternal height, pre-pregnancy body weight, parity, conception method, and fetal number were transcribed from medical records. Pre-pregnancy body mass index (BMI) was calculated from maternal height and pre-pregnancy body weight. Parity was categorized into primipara or multipara. The conception method was classified into spontaneous pregnancy, non-ART, and ART. Non-ART included ovulatory induction and artificial insemination by husband. ART included in vitro fertilization and embryo transfer (IVF-ET) and intracytoplasmic sperm injection. The number of fetuses was classified into singleton and multiple pregnancies. Medical history was obtained from the MT1 questionnaire for the following conditions (type 1 diabetes, type 2 diabetes, other types of diabetes, mental illness, polycystic ovarian syndrome [PCOS], endometriosis, adenomyosis, uterine malformation, hypertension, hyperthyroidism, hypothyroidism, kidney disorder, systemic lupus erythematosus [SLE]). Mental illness was defined as depression, anxiety disorder, schizophrenia, or dysautonomia. Kidney disorder was defined as immunoglobulin A nephropathy and/or nephrotic syndrome. History of antiphospholipid syndrome (APS) was transcribed from medical records. Maternal smoking history, secondhand smoking status, alcohol consumption, and marital status were obtained from the MT1 questionnaire. Maternal smoking history was categorized as never; previously did, but quit before realizing current pregnancy; previously did, but quit after realizing current pregnancy; currently smoking. Secondhand smoking status was categorized as almost never, never, and 1–7 days a week. Maternal alcohol consumption was categorized as never, quit drinking, and continue drinking. Marital status was categorized into married, never married, divorced, and widowed. The maternal education and household income were collected from the MT2 questionnaire. The maternal education was categorized as junior high school, high school, technical junior college, technical/vocational college, associate degree, bachelor’s degree, and graduate school (master’s and doctor’s). Annual household income was categorized as follows: < 2, 2.00–3.99, 4.00–5.99, 6.00–7.99, 8.00–9.99, 10.00–11.99, 12.00–14.99, 15.00–19.99, and ≥ 20.00 million yen. The geographical areas in which the Regional Centres exist were also provided in the dataset, and categorized into Hokkaido, Tohoku, Kanto, Chubu, Kinki, Chugoku, Shikoku, and Kyushu/Okinawa.
Statistical analysis
Characteristics of study participants were shown as mean ± standard deviation for continuous variables and number (percentage) for categorical variables. Differences in characteristics between study participants whose data were analyzed and those who were excluded were examined using Student’s t-test for continuous variables and Chi-squared test for categorical variables.
To investigate the association of MBW with PTD, we applied a multiple logistic regression model. Regarding the associations of MBW with early and late PTD, a multinomial logistic regression model was applied. When MBW, as a categorical variable (i.e., <2,500 g, 2,500–2,999 g, 3,000–3,499 g, 3,500–3,999 g, and ≥4,000 g), was included in a multinomial logistic regression model, MBW of 3,000–3,499 g was set as a reference category in each model according another study on Japanese pregnant women. Reference Shibata, Ogawa and Kanazawa28 A linear-trend test for the associations of MBW with PTD, early and late PTD were also conducted. In addition, the associations of MBW, as a continuous variable per 500 g decrease, with PTD, early and late PTD were also investigated. Model 1 was a crude model. Model 2 was adjusted for areas where Regional Centres exist and maternal age Reference Fuchs, Monet, Ducruet, Chaillet and Audibert36 at the MT1 questionnaire. Model 3 was created by adjusting maternal height, pre-pregnancy BMI, Reference Han, Mulla, Beyene, Liao and McDonald37 conception method, parity (primipara or not), Reference Koullali, van Zijl and Kazemier38 history of mental illness, Reference Männistö, Mendola and Kiely39 history of kidney disease, Reference Zhang, Ma and etal40 history of hyperthyroidism, history of hypothyroidism, Reference Tao, Adurty, D’Angelo and DeSancho41 history of SLE and/or APS, Reference Korevaar, Schalekamp-Timmermans and de Rijke42 history of gynecologic disease (PCOS, endometriosis, adenomyosis, uterine malformation), smoking history, Reference Soneji and Beltrán-Sánchez43 secondhand smoking status, and alcohol consumption, Reference Patra, Bakker, Irving, Jaddoe, Malini and Rehm44 socioeconomic factors, including marital status, Reference Merklinger-Gruchala and Kapiszewska45 maternal education level, Reference Granés, Torà-Rocamora, Palacio, De la Torre and Llupià46 and annual income Reference DeFranco, Lian, Muglia and Schootman47 in addition to model 2. Maternal height is influenced not only by their birth weight but also by other factors such as genetic factors, nutrition, exercise, childhood diseases, and socioeconomic factors such as parental education, so we did not exclude it from the adjustments. Reference Bozzoli, Deaton and Quintana-Domeque48–Reference Lakshman, Zhang and Zhang51 Because a previous study based on the JECS reported the non-linear association of pre-pregnancy BMI with PTD, pre-pregnancy BMI as a categorical variable, instead of a continuous variable, was included in model 2. Reference Nakanishi, Saijo and Yoshioka52 Pre-pregnancy BMI was categorized as follows: <18.5 kg/m2, 18.5–24.9 kg/m2, 25.0–29.9 kg/m2, and ≥30.0 kg/m2. With reference to previous studies, model 4 was created by adjusting for both HDP and glucose metabolism disorders (GMD) (i.e., GDM, type 1 diabetes, and type 2 diabetes) Reference Li, Xing and Wang53 as potential intermediate variables in addition to model 3. Reference Rewers and Ludvigsson54–Reference Pettitt and Jovanovic56 Figure 1 illustrates the causal relationship between MBW and PTD. As shown in Figure 1, the covariates adjusted for in our study may be intermediate variables, except for regions where the Regional Centres exist and maternal age, rather than confounding factors. Regions where the Regional Centres exist and maternal age may be confounding factors.
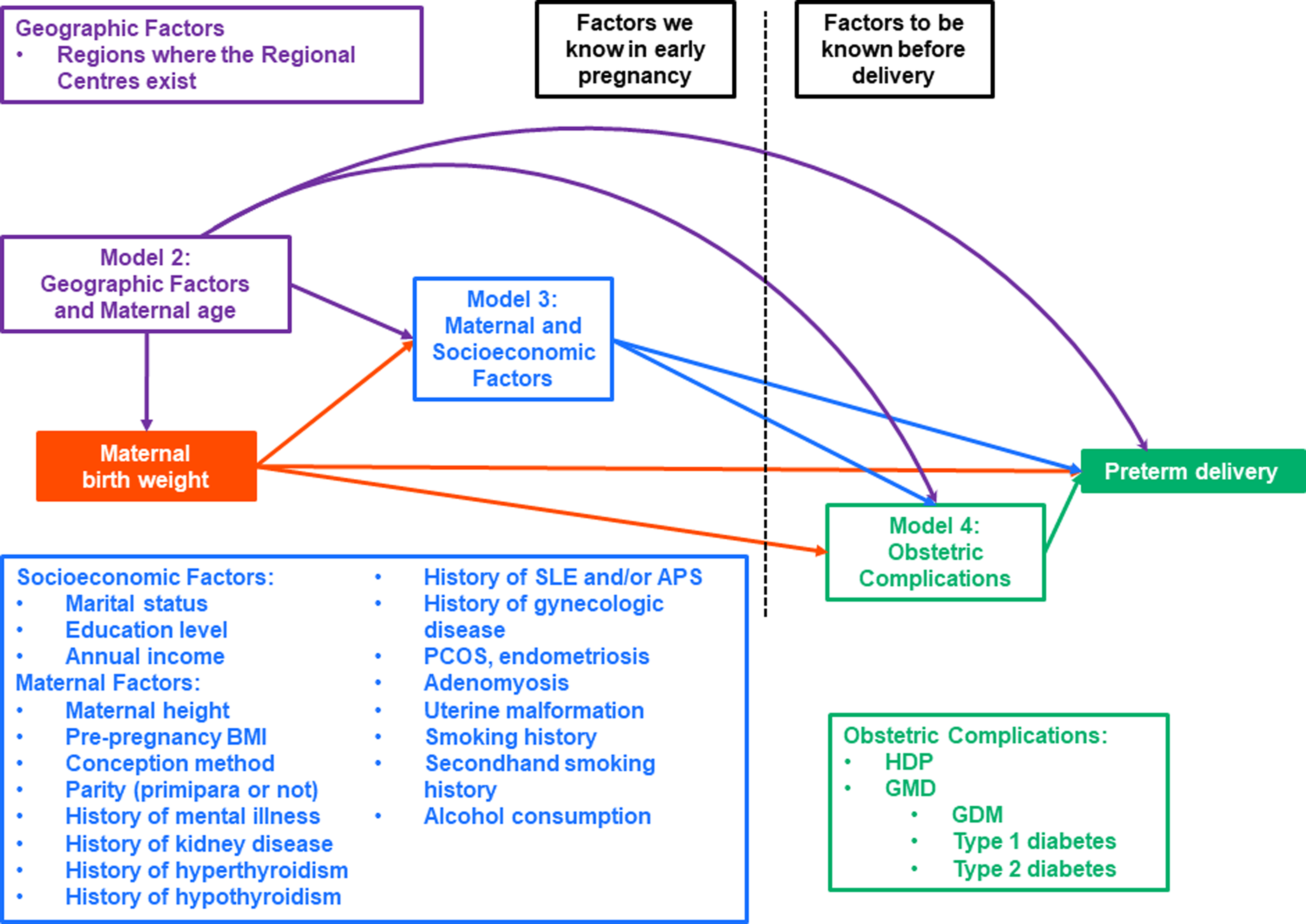
Figure 1. Causal diagram of maternal birthweight and preterm delivery.
Several independent variables with strong multicollinearity were merged as described below. The categories of maternal smoking status “Never,” “Previously did but quit before realizing current pregnancy,” and “Previously did but quit after realizing current pregnancy” were merged into one category. Never married, divorced, and widowed were reclassified into one category. Regarding the highest level of education, junior high school and high school were reclassified into one category. Bachelor’s degrees and graduate degrees (master’s/doctor’s) were reclassified into one category as well. Annual household income was reclassified into the following categories: < 12.00, 12.00–14.99, and ≥ 15.00 million Japanese yen. The regions where the Regional Centres exist had no strong multicollinearity.
We used a multiple imputation with Markov chain Monte Carlo simulation to analyze several independent variables with missing data. Reference Woodward57 The missing pattern of covariates was non-monotone. Imputation model was constructed using the dependent variable and independent variables from model 4. After 10 datasets were created using multiple imputation, the same analysis in each dataset was conducted. Each result was then combined based on Rubin’s rule and reported in this manuscript. A two-sided p < 0.05 was considered statistically significant.
Characteristics of study participants were summarized using a gtsummary package of R, version 4.1.1. Reference Daniel, Michael and Margie58,59 The gtsummary package provides presentation-ready data summary and analytic result tables. We also performed statistical analysis using the SAS software, version 9.4 (SAS Institute Inc., Cary, North Carolina, USA).
Results
Characteristics of study participants
Among 104,062 maternal and fetal records, participants with multiple participations in the JECS (N = 5,689), participants with abortion or stillbirth (N = 1,531), participants with multiple pregnancies (N = 1,809), and those without Japanese nationality (N = 436) or with missing data on nationality (N = 9,584) were excluded. Participants who consented to termination (N = 2,225), participants with missing (N = 3,620) or improbable (N = 28) MBW data, and those with missing data on weeks of delivery (N = 168) were also excluded. Finally, 78,972 participants were included in the analysis. The details of the participant selection process are shown in Figure 2.

Figure 2. Flowchart of the study participants selection.
Maternal and neonatal characteristics of the study participants are summarized in Table 1. The numbers (percentages) of participants with MBWs < 2,500 g and ≥ 4,000 g were 3,881 (4.9%) and 1,781 (2.3%), respectively. The number (percentage) of PTD (delivery from 22 to <37 weeks of gestation) was 3,555 (4.5%). The numbers (percentages) of early PTD (delivery from 22 to <34 weeks of gestation), and late PTD (delivery from 34 to <37 weeks of gestation) were 704 (0.9%) and 2,851 (3.6%), respectively.
Table 1. Characteristics of study participants

APS, antiphospholipid antibody syndrome; ART, assisted reproductive technology; BMI, body mass index; DVT, deep venous thrombosis; GDM, gestational diabetes mellitus; HDP, hypertensive disorders of pregnancy; PCOS, polycystic ovary syndrome; PTD, preterm delivery; SLE, systemic lupus erythematosus.
Continuous variables and categorical variables were expressed as mean ± standard deviation and number (%), respectively.
MBW, the main study exposure, was categorized into five groups as previously described, Reference Shibata, Ogawa and Kanazawa28 with detailed characteristics shown in Table 2. The proportions of PTD, early PTD, and late PTD were higher among participants with an MBW < 2,500 g than among those with an MBW of 3,000–3,499 g. As MBW decreased, there were higher proportions of underweight participants and lower proportions of obese participants. In addition, as MBW decreased, infants tended to have lower birth and placental weights. The proportions of HDP in participants with MBWs < 2,500 g and 2,500–2,999 g were higher than that in participants with an MBW of 3,000–3,499 g. The proportions of GDM in participants with MBWs of < 2,500 g, 2,500–2,999 g, and ≥ 4,000 g were higher than those in participants with MBWs of 3,000–3,499 g and 3,500–3,999 g. A comparison of the characteristics of the included participants and those who were excluded due to missing data was described in the Supplementary Material (Supplementary Table S1).
Table 2. Characteristics of study participants according to categories of maternal birth weight
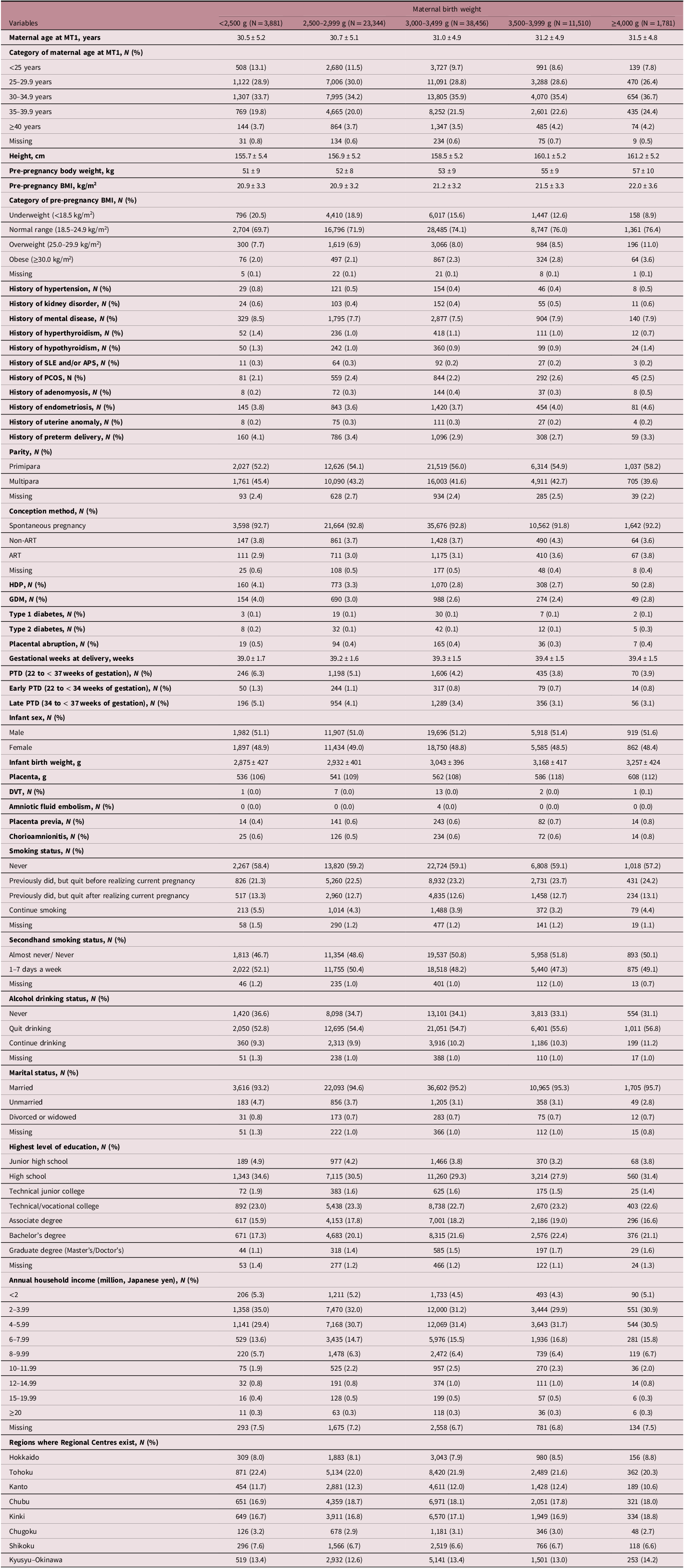
APS, antiphospholipid antibody syndrome; ART, assisted reproductive technology; BMI, body mass index; DVT, deep venous thrombosis; GDM, gestational diabetes mellitus; HDP, hypertensive disorders of pregnancy; PCOS, polycystic ovary syndrome; PTD, preterm delivery; SLE, systemic lupus erythematosus.
Continuous variables and categorical variables were expressed as mean ± standard deviation and number (%), respectively.
Association of MBW with PTD
Multiple logistic regression analysis was performed. Figure 3 shows the association of MBW with PTD to investigate the association of MBW with PTD. After adjustment for the geographical areas of the Regional Centres and maternal age (model 2) with participants having an MBW of 3,000–3,499 g as a reference category, the adjusted odds ratios [ORs] of PTD were 1.574 (95% confidence interval [CI]: 1.370–1.808), 1.255 (95% CI: 1.162–1.355), 0.891 (95% CI: 0.800–0.993), and 0.918 (95% CI: 0.718–1.172) in participants with MBWs < 2,500 g, 2,500–2,999 g, 3,500–3,999 g, and ≥ 4,000 g, respectively. Lower MBW was associated with an increased risk of PTD (p-for-trend < 0.0001). With each 500 g of MBW decrease, the adjusted OR for PTD was 1.206 (95% CI: 1.158–1.256). When we added maternal factors and socioeconomic factors to model 2 to generate model 3, the adjusted ORs of PTD were 1.514 (95% CI: 1.316–1.742), 1.233 (95% CI: 1.140–1.332), 0.903 (95% CI: 0.809–1.007), and 0.922 (95% CI: 0.721–1.179) in participants with MBWs < 2,500 g, 2,500–2,999 g, 3,500–3,999 g, and ≥ 4,000 g, respectively. Lower MBW was associated with an increased risk of PTD (p-for-trend < 0.0001). With each 500 g of MBW decrease, the adjusted OR for PTD was 1.189 (95% CI: 1.140–1.240). Similar results were obtained in Model 4, which added obstetric complications to the adjustment. In all models, lower MBW was associated with higher odds of PTD. The results of the sensitivity analysis of the association between MBW and PTD without excluding multiple participation data, using a generalized estimating equation logistic regression model are shown in the Supplementary Material (Supplementary Table S2).
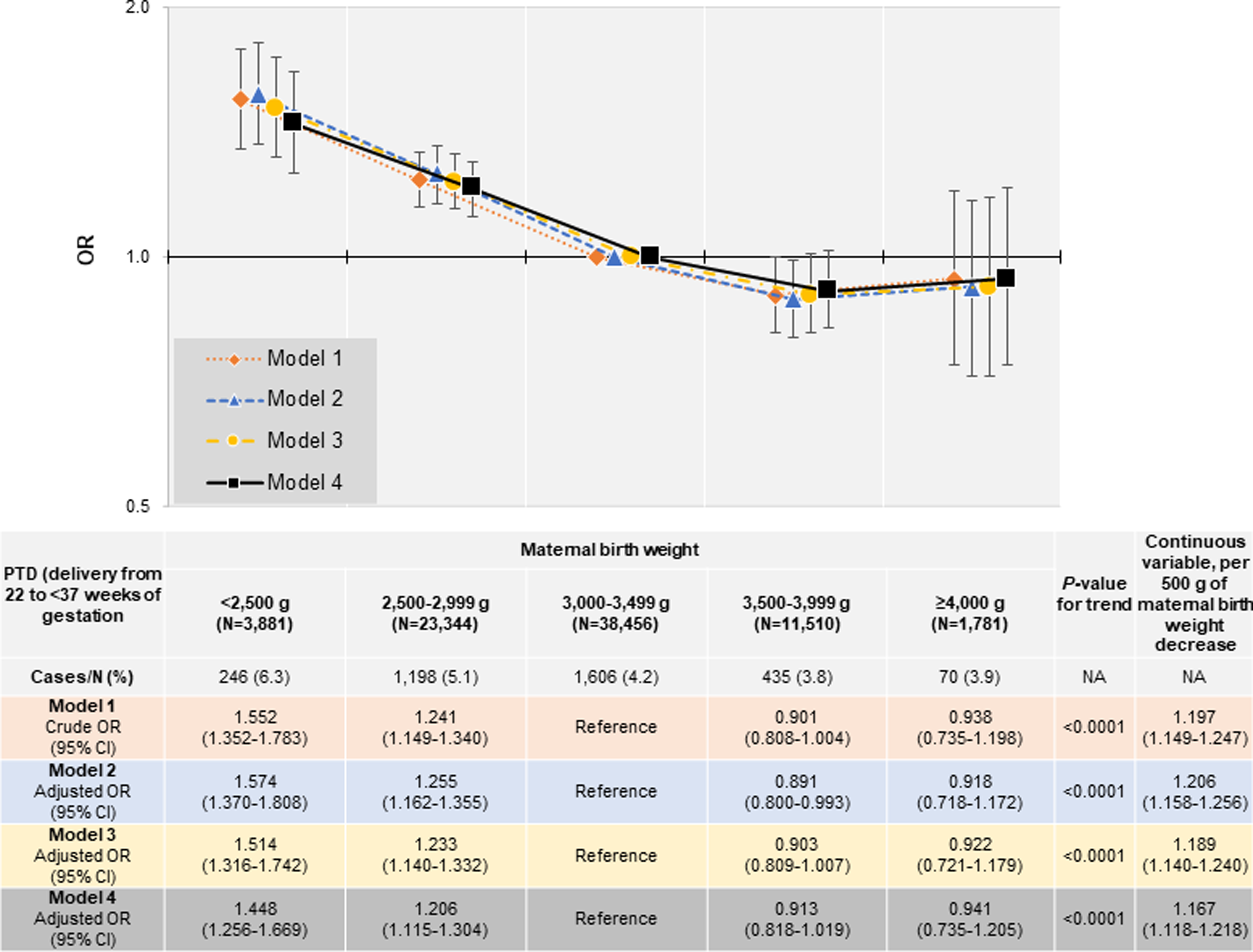
Figure 3. Association of maternal birth weight with PTD (delivery from 22 to < 37 weeks of gestation). Model 1: Crude model. Model 2: Adjusting for regions where the Regional Centres exist and maternal age. Model 3: Adjusting for maternal height, pre-pregnancy BMI, conception method, parity (primipara or not), history of mental illness, history of kidney disease, history of hyperthyroidism, history of hypothyroidism, history of SLE and/or APS, history of gynecologic disease (PCOS, endometriosis, adenomyosis, uterine malformation), smoking history, secondhand smoking history, alcohol consumption, socioeconomic factors, including marital status, education level, and annual income in addition to model 2. Model 4: Adjusting for both HDP and GMD (i.e., GDM, type 1 diabetes, and type 2 diabetes) in addition to model 3. Categorical variables: regions where the Regional Centres exist, maternal age, pre-pregnancy BMI, conception method, parity, history of mental illness, history of kidney disease, history of hyperthyroidism, history of hypothyroidism, history of SLE and/or APS, history of gynecologic disease (PCOS, endometriosis, adenomyosis, uterine malformation), smoking history, secondhand smoking history, alcohol consumption, marital status, education level, annual income, HDP, GDM, type 1 diabetes, and type 2 diabetes. Continuous variables: maternal age, and height. APS, antiphospholipid antibody syndrome; BMI, body mass index; CI, confidence interval; GDM, gestational diabetes mellitus; GMD, glucose metabolism disorders; HDP, hypertensive disorders of pregnancy; OR, odds ratio; PCOS, polycystic ovary syndrome; PTD, preterm delivery; SLE, systemic lupus erythematosus.
Associations of MBW with early and late PTD
We further investigated whether the associations of MBW with PTD differed between the two PTD subtypes (early PTD, delivery from 22 to < 34 weeks of gestation; late PTD, delivery from 34 to <37 weeks of gestation) using multinominal logistic regression analysis. Term delivery (≥37 weeks of gestation) was set as a reference. Adjustments for each model were the same as for the PTD analysis, with participants having an MBW of 3,000–3,499 g as a reference category.
Table 3 shows the association of MBW with early PTD. In model 2, the adjusted ORs of early PTD were 1.568 (95% CI: 1.159–2.121), 1.277 (95% CI: 1.079–1.512), 0.823 (95% CI: 0.642–1.055), and 0.900 (95% CI: 0.525–1.544) in participants with MBWs <2,500 g, 2,500–2,999 g, 3,500–3,999 g, ≥ 4,000 g, respectively. Lower MBW was associated with an increased risk of early PTD (p-for-trend<0.0001). With each 500 g of MBW decrease, the adjusted OR for early PTD was 1.237 (95% CI: 1.132–1.353). In model 3, the adjusted ORs of early PTD were 1.436 (95% CI: 1.058–1.950), 1.230 (95% CI: 1.037–1.459), 0.823 (95% CI: 0.641–1.056), and 0.887 (95% CI: 0.515–1.526) in participants with MBWs < 2,500 g, 2,500–2,999 g, 3,500–3,999 g, ≥ 4,000 g, respectively. Lower MBW was associated with an increased risk of early PTD (p-for-trend: 0.0002). With each 500 g of MBW decrease, the adjusted OR for early PTD was 1.207 (95% CI: 1.101–1.323). Almost similar results were obtained for model 4.
Table 3. Association of MBW with early PTD and late PTD

APS, antiphospholipid antibody syndrome; BMI, body mass index; CI, confidence interval; GDM, gestational diabetes mellitus; GMD, glucose metabolism disorders; HDP, hypertensive disorders of pregnancy; OR, odds ratio; PCOS, polycystic ovary syndrome; PTD, preterm delivery; SLE, systemic lupus erythematosus; NA, not applicable.
Model 1: Crude model. Model 2: Adjusting for regions where the Regional Centres exist and maternal age. Model 3: Adjusting for maternal height, pre-pregnancy BMI, conception method, parity, history of mental illness, history of kidney disease, history of hyperthyroidism, history of hypothyroidism, history of SLE and/or APS, history of gynecologic disease (PCOS, endometriosis, adenomyosis, uterine malformation), smoking history, secondhand smoking history, alcohol consumption, socioeconomic factors, including marital status, education level, and annual income in addition to model 2. Model 4: Adjusting for both HDP and GMD (i.e., GDM, type 1 diabetes, and type 2 diabetes) in addition to model 3.
Categorical variables: regions where the Regional Centres exist, maternal age, pre-pregnancy BMI, conception method, parity, history of mental illness, history of kidney disease, history of hyperthyroidism, history of hypothyroidism, history of SLE and/or APS, history of gynecologic disease (PCOS, endometriosis, adenomyosis, uterine malformation), smoking history, secondhand smoking history, alcohol consumption, marital status, education level, annual income, HDP, GDM, type 1 diabetes, and type 2 diabetes.
Continuous variables: maternal age, and height.
The association of MBW with late PTD is also shown in Table 3. In model 2, the adjusted ORs of late PTD were 1.524 (95% CI: 1.305–1.780), 1.227 (95% CI: 1.126–1.337), 0.917 (95% CI: 0.814–1.034), and 0.908 (95% CI: 0.691–1.194) in participants with MBWs <2,500 g, 2,500–2,999 g, 3,500–3,999 g, ≥4,000 g, respectively. Lower MBW was associated with an increased risk of late PTD (p-for-trend < 0.0001). With each 500 g of MBW decrease, the adjusted OR for late PTD was 1.181 (95% CI: 1.129–1.236). In model 3, the adjusted ORs of late PTD were 1.470 (95% CI: 1.257–1.720), 1.208 (95% CI: 1.107–1.318), 0.922 (95% CI: 0.817–1.040), and 0.916 (95% CI: 0.696–1.205) in participants with MBWs <2,500 g, 2,500–2,999 g, 3,500–3,999 g, ≥ 4,000 g, respectively. Lower MBW was associated with an increased risk of late PTD (p-for-trend <0.0001). With each 500 g of MBW decrease, the adjusted OR for late PTD was 1.168 (95% CI: 1.114–1.224). Almost similar results were obtained for model 4. In all models, lower MBW was associated with higher odds of both early and late PTD.
Discussion
To our best knowledge, the current study is the first to demonstrate an inverse association between MBW and PTD in the Japanese population. This association was also observed when PTD was categorized into two subtypes, early and late PTD. Our results confirmed findings from previous studies that maternal LBW was a risk factor of PTD. Reference De, Lin, Lohsoonthorn and Williams26,Reference Emanuel, Leisenring and Williams27 There were no major differences between the basic characteristics of the study group in this study and those in other Japanese birth cohort studies. Therefore, we believe that participant selection did not influence the results.
In a study from the United States of America with Caucasian, Native American, African American, and Hispanic participants, the OR of PTD for maternal LBW (<2,500 g) was 1.51 (95% CI: 0.96–2.38) and the adjusted OR (adjusted for maternal age, parity, ethnicity, and pre-pregnancy BMI) was 1.54 (95% CI: 0.97–2.44). Although similar trends were observed in each of the four ethnic groups, an Asian population was not included in this study. Another study showed that the ORs of PTD with maternal LBW (<2,500 g) among African Americans and Caucasians were 1.6 (95% CI: 1.3–1.9) and 1.3 (95% CI: 1.0–1.6), respectively. Reference Simon, Vyas, Prachand, David and Collins60 The baseline characteristics of the cohort in our study were similar to those in other birth cohort studies in Japan (i.e., The Hokkaido Birth Cohort Study on Environment and Children’s Health, Tohoku Medical Megabank Project Birth and Three-Generation Cohort Study, and the Kyushu Okinawa Maternal and Child Health Study). Reference Kuriyama, Metoki and Kikuya61–Reference Miyake, Tanaka and Arakawa63 Specifically, maternal age, BMI, gestational weeks at delivery, PTD, birth weight, smoking status, alcohol consumption, parity, highest level of education, and annual household income were similar. Therefore, the generalizability of our results would not be low. Our result in a Japanese population is consistent with these aforementioned findings, which indicated that maternal LBW was a PTD risk factor with different degrees of association among the races. Further, MBW was an indicator of PTD, regardless of ethnicity. However, our results were inconsistent with those shown in a previous Japanese study. Reference Shibata, Ogawa and Kanazawa28 Although pregnant women with MBW < 2,500 g also tended to have higher odds ratios for PTD than those with 3,000–3,499 g in the previous study, Reference Shibata, Ogawa and Kanazawa28 statistical significance was not noted. Our study used a large-scale cohort from multiple facilities (N = 78,972), while the previous study was conducted at a single facility with a relatively small number of participants (N = 944). The small number of cases of PTD in the previous study might be the reason there was no statistical significance.
Regarding the association of MBW with PTD subtypes, the effect size of the association of MBW with early PTD were similar to that with late PTD. In contrast, De et al. reported that the association of MBW with early PTD was stronger than that with late PTD. Reference De, Lin, Lohsoonthorn and Williams26 The ORs of early and late PTD in participants with an MBW < 2,500 g were 1.94 (95% Cl: 0.73–5.20) and 1.46 (95% Cl: 0.88–2.43), respectively, in the study of De et al., whereas in our study, the corresponding ORs were 1.347 (95% Cl: 0.989–1.835) and 1.418 (1.210–1.660), respectively. Differences in the number of participants, independent variables in the statistical analysis, and ethnicity might account for this discrepancy.
Numerous risk factors for PTD, including gestational hypertension, intrauterine infections, uterine tumors, morphological abnormalities of the uterus, and ART, have been reported. Reference Tamura, Hanaoka and Ito22–Reference Ohmi, Hirooka and Mochizuki24 Even after adjusting for several variables, the association of maternal LBW with PTD persisted. Maternal height might be an intermediate variable. Therefore, we performed an analysis excluding maternal height from the adjustments, but the results did not change significantly. The results of Model 4, created to account for the possibility that maternal complications such as HDP and GMD were intermediate variables, were similar to those of Model 3, which did not adjust for these variables. Therefore, we considered that the relationship between MBW and PTD could not be fully explained by HDP and GMD. Furthermore, as an additional sensitivity analysis, we performed statistical analysis in pregnant women with neither HDP nor GMD, but the results did not change significantly. Thus, we believe that the relationship between MBW and PTD cannot be fully explained by HDP and GMD. As an epidemiological study, our research could not explore the underlying mechanism of this phenomenon, which might require further investigations with animal or human models to discover related molecular components.
Maternal LBW includes cases of preterm birth and SGA, which were not considered in our study. Therefore, it was not possible to determine whether the maternal LBW was caused by a preterm delivery or SGA. Thus, maternal LBW would include both SGA and PTD in our study. Previous studies Reference Selling, Carstensen, Finnström and Sydsjö13,Reference Klebanoff, Schulsinger, Mednick and Secher25 demonstrated that either SGA or preterm birth was a PTD risk factor.
This study has several strengths. First, the study participants were recruited from diverse geographical areas in Japan. The PTD rate in Japan was 5.9% in 2010, one of the lowest rates in the world. 64 Similar to that provided in the World Health Organization report, the PTD rate was 4.5% in the current study. Therefore, the external validity of our results is expected to be high. Second, this study considered many variables in the statistical analysis, including maternal and socioeconomic factors.
However, some limitations of this study should also be considered. First, the causes of PTD (the main outcome in our study), such as artificial and spontaneous PTD, were not collected in the JECS. As information regarding maternal birth’s gestational age was not collected in the JECS, we were not able to determine whether the cause of maternal LBW was SGA or PTD. Second, MBW information was obtained from the self-reported questionnaire at 6 months after delivery, rather from the MT1 or MT2 questionnaires, which is the study design of the JECS. However, birth weights transcribed from medical records and self-report were known to be comparable. Reference Little65 Additionally, the actual MBW obtained from the Maternal and Child Health Handbook was similar to that in the self-report in Japan. Reference Katsuragi, Okamura, Kokubo, Ikeda and Miyamoto66 Some previous studies, using the JECS, showed an association between low MBW and perinatal outcomes, including HDP or GDM, similar to the case using MBW collected from the birth weight stated in the maternal birth certificate. Reference Tagami, Iwama and Hamada67–Reference Tagami, Iwama and Hamada69 Therefore, this limitation would not significantly affect our findings. Furthermore, JECS did not collect also did not collect factors that led to a pregnant woman’s own birth as a LBW baby. Kasuga et al. found that low pre-pregnancy maternal weight, inadequate gestational weight gain, birth at 37 gestational weeks, HDP, anemia during pregnancy, female sex, and neonatal congenital anomalies were risk factors for LBW in Japanese full-term infants. Reference Kasuga, Ikenoue and Tamagawa70 It is necessary to examine the relationship between these risk factors for giving birth to LBW babies and PTD in the next generation. A presumed confounding factor would be a family history of preterm delivery and socioeconomic variables at maternal delivery. Because our study did not collect family history of PTD and socioeconomic variables at maternal delivery, which are possible confounding factors, residual confounding would exist. Reference Rolnik, Wright and Poon72 The clinical significance of our study is that the use of information on MBW would contribute to detect pregnant women at high risk of PTD.
Decreasing the number of LBW girls might reduce the PTD risk in future pregnancies. Treatment of preeclampsia, a risk factor of LBW, could presumably reduce the number of LBW girls. Low-dose aspirin for high-risk cases might play a role in reducing preterm preeclampsia, Reference Rolnik, Wright and Poon72 and thus, reducing the LBW risk.
In conclusion, the current study showed an inverse association between MBW and PTD. Obtaining the birth weights of the pregnant women as a part of the prenatal medical history examinations could play an important role in PTD prediction. Future studies are expected to elucidate the mechanism by which MBW influences gestation length.
Supplementary material
The supplementary material for this article can be found at https://doi.org/10.1017/S2040174424000126.
Acknowledgments
We would like to thank all JECS participants and staff and the members of the 2022 JECS Group listed in the appendix of the supplementary materials. We also gratefully acknowledge Editage (https://www.editage.com/) for their help in revising the syntax and grammar of the manuscript.
Financial support
This study was funded by the Ministry of Environment, Japan. The findings and conclusions of this article are solely the responsibility of the authors and do not represent the official views of the above government.
Competing interests
None.