Introduction
The use of patient electronic health record (EHR) data for clinical trial data collection has been helpful in streamlining parts of the research process [Reference Laird-Maddox, Mitchell and Hoffman1–Reference Nordo, Levaux and Becnel4]. However, EHR data and data for clinical trials are collected for fundamentally different purposes. EHR data are collected primarily to inform clinical care and support hospital billing with little consideration for secondary research use. Clinical trials, on the other hand, require rigorous protocol-specific data collection with precise clinical context and timing parameters to enable controlled comparisons. While not all EHR data are beneficial for clinical studies, some, such as clinical lab results and vital signs collected in usual care clinical settings, can be repurposed for research by entering them into case report forms (CRFs) from the EHR. Reusing EHR data reduces participant burden by avoiding the duplication of patient testing and conserves valuable study resources by reducing study costs.
Historically, the process for using EHR data in study CRFs has relied upon research coordinators performing chart reviews on research participants, then manually transcribing data from the EHR into participants’ CRFs in an electronic data capture (EDC) system such as REDCap (Research Electronic Data Capture) [Reference Harris, Taylor, Thielke, Payne, Gonzalez and Conde5]. The process of chart review and transcribing data from the EHR to an EDC by research coordinators is labor intensive and prone to error [Reference Mays and Mathias6]. Automated CRF completion using EHR data has the potential to improve the efficiency and accuracy of study data collection [Reference Marsolo7]. Researchers have shown that automatically transferring data from EHRs to CRFs can decrease data latency, transcription errors, database queries, monitoring activity, and staff time and effort [Reference Buckley, Vattikola, Maniar and Dai8,Reference Nordo, Eisenstein and Hawley9]. Others have found that the added value for EHR-to-CRF transfer is limited by data missingness and a lack of contextual detail that is needed for research [Reference Bots, Groenwold and Dekkers10,Reference Zopf, Abolafia and Reddy11]. Nevertheless, guidance from the U.S. Food & Drug Administration (FDA) has encouraged the secondary use of EHR clinical data for research, emphasizing that the interchange between EHR and EDC systems should leverage interoperable standards [Reference Rocca, Asare, Esserman, Dubman and Gordon12,13].
This study assesses the potential benefit of automated EHR-to-CRF data transfer to augment coordinator chart review for an actual clinical trial. We measure coverage (the number of fields that automation could complete), concordance (the degree of agreement between human and machine-extracted data), and efficiency (the amount of coordinator time potentially saved by automating data transfer). We also outline a process for setting up EHR-to-CRF mapping to maximize concordance and coverage using this methodology for other clinical trials.
Materials and Methods
We used the Accelerating COVID-19 Therapeutic Interventions and Vaccines (ACTIV) Host Tissue (A4-HT) platform as a test case for the EHR-to-CRF data transfer. A4-HT seeks to test various therapeutic medications for critically ill patients with COVID-19 [Reference Moskowitz, Shotwell and Gibbs14]. A4-HT is a multisite trial platform with over 50 recruitment sites across the USA. In this study, we focused on data collected and managed by the study team at VUMC. A4-HT research coordinators assess outcomes based on EHR data collected for routine care and enter them into a REDCap study database daily. There are 28 CRFs in the A4-HT REDCap project. Most, such as eligibility criteria, medical history, and demographics, are only collected at baseline. Three CRFs – the daily inpatient form, clinical labs, and vital signs – must be completed for each day during which the participant is an inpatient at the hospital for up to 28 days during the study. The primary REDCap study database supporting A4-HT at VUMC is not currently configured for EHR-to-CRF data transfer. Thus, it serves as a reference database for comparing traditional CRF data entry and automated CRF extraction using REDCap’s Clinical Data Interoperability Services module (CDIS). CDIS gives REDCap the ability to extract data from EHR Application Programming Interfaces (APIs) that comply with the HL7 Fast Healthcare Interoperability Resources (FHIR) standard [Reference Cheng, Duda, Taylor, Delacqua, Lewis, Bosler, Johnson and Harris15].
We obtained permission to conduct this evaluation from the A4-HT principal investigator, the VUMC site principal investigator, and the VUMC Institutional Review Board (study #220069). First, we assessed which A4-HT CRF instruments contained fields where data are available from the EHR. Next, we invoked a ‘copy project’ procedure in REDCap to clone the original A4-HT REDCap project, thereby creating a new REDCap study database with identical CRFs, data fields, and events, but no study data. We then used REDCap’s researcher-facing CDIS mapping tools to map data from the EHR to each of the CRF fields where mapping was feasible.
Once our new FHIR-enabled REDCap database was established and all data mappings were configured by our study team, we began collecting and comparing the automated EHR data. We chose the first 10 of the 40 A4-HT participants who had completed the study at VUMC by January 24, 2022 for comprehensive data comparison. For these 10 participants, we extracted medical record numbers (MRN) and randomization date and time from the original REDCap study database and inserted them into the FHIR study database. The REDCap CDIS module then extracted all mapped EHR data into participants’ CRFs.
Since the FHIR REDCap project was a clone of the original A4-HT REDCap project with the same variable and event names, statisticians were able to use R to query the REDCap API and compare the values in the two projects. For the 10-participant subset, a A4-HT study coordinator and REDCap analyst reviewed and discussed data discrepancies between the two projects and modified REDCap CDIS mappings to better align with the original data wherever appropriate and possible. We iteratively reapplied the mapping rules, refreshed the EHR data, and compared the data between the two projects until we could no longer make any additional improvements. As part of our assessment of efficiency, we documented personnel efforts for the coordinator and analyst to conduct these mapping tasks.
Finally, using data from the remaining 30 A4-HT participants (total of 40 participants), we tested whether our findings could be extrapolated to a larger data set. For this set of data, we did not perform an in-depth comparison with a research coordinator but did characterize both coverage and high-level (exact match only) concordance. We also estimated the amount of time spent entering data using results from Nordo et al., which timed the abstraction of demographic data from the EHR into REDCap by research personnel [Reference Nordo, Eisenstein and Hawley9]. That study found that coordinators spent an average of 15 seconds filling out each demographic field in REDCap from the EHR. Estimating 15 seconds per field provides a conservative estimate of time spent completing CRFs since demographic information is easier to find and transcribe than clinical information and because the 15 seconds does not include initiation tasks such as opening the EHR browser and CRFs.
Results
Coverage
In assessing which instruments and fields were most appropriate for automated EHR data exchange, we eliminated instruments related to randomization, blinding, and compliance. We considered EHR data for adverse events but deemed that creating phenotypes for adverse events from EHR codes was outside the scope of this work. Medical history and concomitant medications seemed feasible by scanning for condition and medication names in the problem list and medication lists, respectively. However, our testing with the first few patients proved difficult to generate a complete list of conditions and medications that fit into the various categories. Additionally, these data only have one status (i.e., active, or inactive) per condition or medication, which made it difficult to determine retroactively which conditions and medications started before the trial started.
We utilized six CRFs for which to test FHIR data pull: Demographics, Eligibility Criteria, COVID-19 Testing/Vaccination, Daily Inpatient Form, Clinical Labs, and Vital Signs. The Demographics and COVID-19 Testing/Vaccinations CRFs had FHIR data types, known as resources, that addressed those instruments well (the Patient and the Immunizations FHIR resources, respectively). The Daily Inpatient Form, Clinical Labs, and Vital Signs instruments all relied primarily on the Observation FHIR resource. These three forms presented the greatest opportunity to address coordinator burden since they had to be completed on each day of participants’ inpatient stays during the study. In total, we mapped data for 85 CRF fields out of 100 possible fields on these six forms.
At the time we collected data for this study, 40 participants had enrolled in and completed the A4-HT study at VUMC. Coordinators entered 11,952 values in six CRFs for these 40 participants. The data from FHIR were able to populate 10,081 (84%) values. Table 1 summarizes the coverage results. Our analysis found that the study could have saved 42 hours of personnel time by using only the automated EHR extraction for the six CRFs of these 40 participants. The study coordinator and analyst expended approximately 42 hours to review and optimize the mapping rules.
Table 1. Coverage of FHIR to complete data filled by coordinator by CRF for 40 participants

* Based on Nordo et al. mean of 15 seconds per value entered by coordinator [Reference Nordo, Eisenstein and Hawley9].
CRF, case report form; FHIR, Fast Healthcare Interoperability Resources; COVID-19, coronavirus disease of 2019.
Concordance: Detailed Evaluation of 10 Participants
Our detailed assessment of concordance was performed on data from 10 participants (Fig. 1). Of the 2659 values entered by the coordinator with data from the automation, 2463 (93%) matched exactly. The remaining 196 instances were discrepancies that could not be resolved by modifying the mapping or REDCap calculations. There were 152 instances in which the automated data from the EHR was correct and the data hand-entered by the coordinator was incorrect as mutually agreed by the coordinator and analyst. Data entry errors included recording the first value after 8:00 am instead of the value closest to 8:00 am, entering data for the wrong day (i.e., 1 day ahead of or behind the actual study day), entering values into the wrong field adjacent to the correct field, and other simple typos. In 20 discrepancies, FHIR lacked mapping to some source fields in the EHR that the coordinators used. For example, we were able to map “pulse” in the EHR feed, but the coordinators typically selected “heart rate” from another tab in the EHR. For these 20 discrepancies, neither human nor machine were technically incorrect. Finally, 24 of the discrepancies were due to other limitations in the EHR data. For example, fever was not consistently documented in patients’ problem lists and therefore was not always available for determining eligibility criteria.
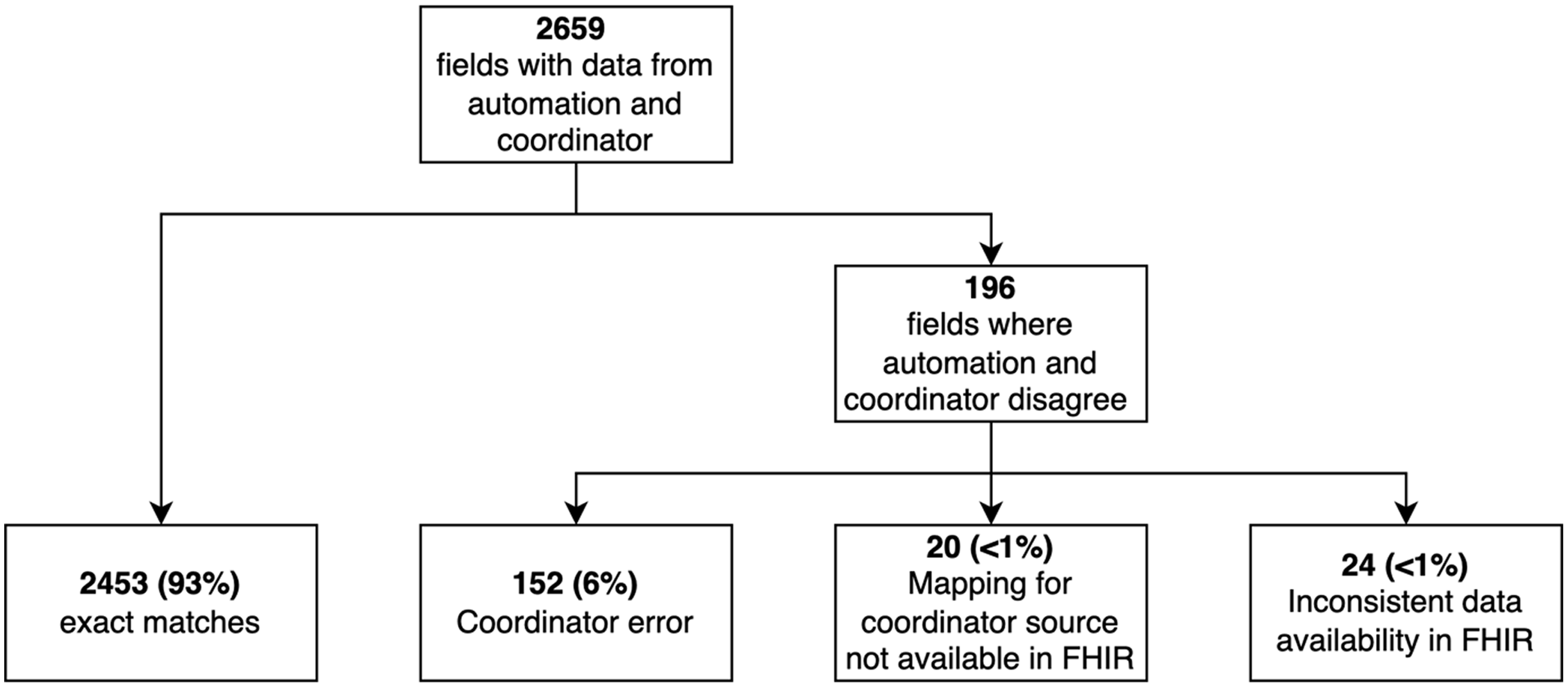
Fig. 1. Summary of data concordance for the first 10 participants in the trial at Vanderbilt University Medical Center. FHIR, Fast Healthcare Interoperability Resources.
Concordance: Larger Participant Sample (40 Participants)
In examining all 40 records, we found that over 90% of values with both automation and coordinator values matched exactly except in the vital signs form which had 79% concordance (Table 2). As we discovered in our detailed analysis of the first 10 records, many of the vital signs data had several sources and multiple measurements to choose from on any given study day, which may have led to these discrepancies.
Table 2. Concordance results by form

CRF, case report form; EHR, electronic health record; COVID-19, coronavirus disease of 2019.
Discussion
While superiority of EHR-to-CRF data transfer over manual methods in accuracy and efficiency has been assumed, the only prototype or proof-of-concept studies that have attempted to map real-world EHR data to CRFs using FHIR resources have met with mixed success [Reference Zopf, Abolafia and Reddy11,Reference Hume, Abolafia and Low16–Reference Garza, Rutherford and Myneni19]. A major limitation of these attempts has been the limited array of data elements that can be extracted from EHR to CRF via FHIR, because of fields that are semantically nonequivalent or lack coverage in FHIR resources. Garza et al. [Reference Garza, Rutherford and Myneni19], for example, performed mapping from EHR to CRF using FHIR resources in three diverse multisite clinical trials to evaluate FHIR coverage in support of data collection and transformation across a wide range of study data elements and found 45–80% of elements were covered in FHIR resources.
Previous efforts to “eSource” data from the EHR for clinical trials used a standard called Retrieve Form Data Capture (RFD) which allowed systems such as REDCap to extract EHR data [Reference Nordo, Eisenstein and Hawley9]. RFD has since been supplanted by FHIR [20] as the de facto standard for exchanging healthcare data between systems. Although adoption of the FHIR standard has accelerated progress in automating data pipelines for randomized clinical trials and mitigating the need for manual data transcription, the semantic interoperability across systems has continued to impede efforts at more complete automation [Reference Nordo, Levaux and Becnel4].
Another limitation in EHR-to-CRF automation has been the lack of accessibility for researchers without significant IT budgets or available informatics experts. Leveraging REDCap, however, enables researchers at over 6000 institutions in 147 countries to access EHR FHIR API data and to seamlessly collect data for clinical and translational research [Reference Harris, Taylor and Minor21]. Currently, 40 institutions in the United States and Canada have integrated REDCap with either Epic or Cerner EHR systems. Local use of CDIS is strong at Vanderbilt University Medical Center (VUMC) and is offered at no cost to VUMC research teams for projects meeting IRB and Privacy Office requirements.
This study provides strong evidence that automated CRF completion using EHR FHIR API data has the potential to improve the accuracy, consistency, and efficiency of clinical trial data collection. This could also translate into significant resource savings in a clinical trial by not requiring the coordinator to manually extract and enter these data. These benefits are particularly true for data where there is just one value for a given patient, such as demographics, and where a single value needs to be extracted at a particular time from the EHR, such as labs and vitals. Results where the data collector needs to search through EHR records over a time period, such as concomitant medications, medical history, or eligibility criteria, are more challenging to automate with EHR data, but still feasible with text searches and calculated fields.
After project setup, the automated EHR data extraction can be initiated in less than 5 minutes of personnel effort. Therefore, the A4-HT study team might have saved 42 hours of personnel time if they had automated EHR data extraction for the first 40 participants in the study. The combined 40 hours that the coordinator and analyst spent design mapping and validating the data coming from the EHR must be taken into account when designing future studies using this automation. The amount of time it takes to set up the project may vary depending on the clinical and informatics expertise of the team members. We therefore recommend that both a study coordinator, with knowledge of the research, and an analyst, with knowledge of the EHR and REDCap be involved in the mapping and testing. While this setup time is considerable, we believe project-level implementation mapping and data validation exercises will decrease over time as study personnel become more versed with the data availability in the EHR and the mapping process. Moreover, the value of automated EHR extraction will be maximized for large trials with many participants and many EHR data points. Smaller trials with few participants and data points are unlikely to benefit from automation.
Future EHR-to-CRF Work with Additional A4-HT Sites
A4-HT was an ideal trial to test the EHR-to-CRF interface because the study team had already planned to use REDCap for collecting and managing abstracted EHR data. REDCap has existing functionality allowing rapid export and reuse of study data dictionaries and mapping files for sharing with other sites. Going forward, we are working to find additional A4-HT sites to externally test our coverage, concordance, and setup time results. Additional sites would receive a REDCap XML project setup file, a mapping file, and an API query and analysis R script to recreate the calculations we did at VUMC. Since EHR data structure is highly variable at different institutions, some modifications to the EHR mapping and associated calculations may be necessary. Characterizing this congruence or noncongruence will be a secondary finding. Our primary analysis will demonstrate that the automated CRF completion works at a diverse group of health systems with a variety of EHR vendors. We also plan to demonstrate that there is minimal effort needed from the adopting sites once the study mapping has been performed at one institution (e.g. data coordinating center) and shared with other site institutions. We anticipate, based on anecdotal work, that meaningful sharing of implementation-ready field mappings will be straightforward for sites where standardized codes (e.g. Logical Observation Identifiers Names and Codes LOINC) are well characterized in the local EHR system. In other cases, individual sites would have to perform their own mapping with local codes to adopt EHR-to-CRF automation. Smaller domestic sites with older EHR systems and international sites with diverse coding standards would likely be more difficult to onboard.
Guidance for Use of REDCap CDIS Services in Single or MultiSite Trials
This study and other pragmatic clinical trials demonstrate that researchers can confidently use EHR data embedded in CRFs to augment or streamline several clinical trial processes. For example, a screening form could extract a patient’s problem list and medication list from the EHR so that the coordinator could review them quickly for eligibility criteria without having to open the EHR. EHR-to-CRF methods could also be used to assist with data monitoring. In our in-depth review of 10 participants’ data to compare the coordinator-entered and automation-entered data, our results showed that most of the discrepancies were a result of human error. These data had already been audited by a study monitor. Therefore, the EHR was able to identify many cases of incorrectly entered data that two humans had previously reviewed. This suggests we could use EHR-to-CRF integration to make risk-based monitoring more efficient. Instead of asking monitors to check all or a sample of CRF entries with the EHR, they could run a discordance report of all instances where the automated EHR data and the coordinator-entered data disagree and focus efforts on those entries.
Based on our experience in this study and working with investigator teams at VUMC implementing EHR data mapping and transfer services using REDCap CDIS, we have developed a set of recommendations that should generalize across institutions and studies. Future studies that will use EHR data should consider what data can be automated from the EHR during the study design phase. After defining the study goals, EHR analysts, statisticians, and coordinators should work together to ensure that the EHR data obtained meets the intended purpose for the study. When mapping EHR data, study personnel should identify a few real patients that would qualify for the study as examples of what data is available using the CDIS mapping helper feature. Fields that are automated with EHR data should be segregated into forms separate from fields that are coordinator-entered. Table 3 outlines the process for designing and running a study with automated EHR-to-CRF data collection.
Table 3. Process for planning and executing a trial with automated EHR-to-CRF data collection
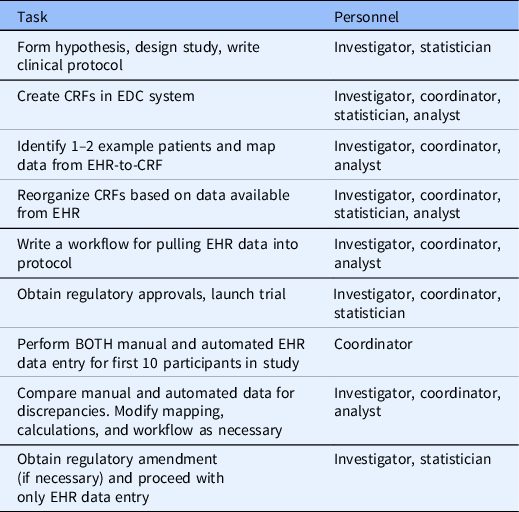
CRF, case report form; EDC, electronic data capture; EHR, electronic health record.
Conclusions
Based on our assessment in this study, we believe automated CRF completion with EHR data has a strong potential for increasing timeliness, accuracy, and efficiency of data-related clinical trial tasks, including participant screening, data collection, and data monitoring. For the A4-HT study, we found that the majority of coordinator data entry burden for demographics, eligibility criteria, vaccine, and daily labs and vital signs could be reduced through automation. The automation would have also reduced the number of data abstraction errors. Future work evaluating resource investment for study start-up versus downstream benefit is needed to inform the total value proposition for diverse single and multisite studies.
Acknowledgments
The authors would like to thank our funders at NHLBI 1OT2HL162110 (JDJ, MMJ, AJB, SC, WHS, MSS, CJL), 1OT2HL156812 (ACC, MKB, CJL, PAH) and NCATS 2UL1TR002243 (ACC, MKB, LS, NK, FD, AL, SC, WHS, SC, PAH), 5U24TR001608 (PAH), 4U24TR001579 (ACC, NK, PAH).
Disclosures
SC has served as a consultant for Vir Biotechnology. CJL has received research funding to institution from Endpoint Health for projects related to automated EHR data extraction; stock options in Bioscape Digital unrelated to the current work; contracts to institution for research services from bioMerieux, AbbVie, AstraZeneca, and Entegrion (unrelated to the current work); patents for risk stratification in sepsis and septic shock held by Cincinnati Children’s Hospital Medical Center, unrelated to the current work.
Disclaimer
The views and conclusions contained in this document are those of the authors and should not be interpreted as representing the official policies, either expressed or implied, of the NIH.