Policy Significance Statement
In managing the COVID-19 pandemic, governments and policymakers have become heavily reliant on the use of data to inform policy and decision-making. However, their ability to do so depends on individuals being willing to provide personal information. Thus, it is important to examine whether the pandemic has resulted in shifts in individuals’ trust in how their data is used, and their attitudes on data privacy. This article provides researchers and policymakers with a greater understanding of how data trust and attitudes on data privacy have changed throughout the pandemic, and which demographic groups have lower levels of trust. These insights can help governments to develop strategies to improve the level of trust in their communities.
1. Introduction and Overview
A key feature of the COVID-19 pandemic has been the constant reporting of data, almost in real time. There is daily reporting of epidemiological data on the number of new cases of COVID-19, number of deaths, number of tests and the reproductive rate of the virus. More recently, reporting on vaccine take-up has taken on a greater focus. Alongside the availability of epidemiological data, there has been continued reporting of broader economic and social outcomes, with an early focus on the income and employment effects of the pandemic, and more recent data focusing on an apparent increase in inflation and resignation rates. Both sets of data have been compared and contrasted between and within countries to debate the trade-off or lack thereof between economic and public health outcomes. Such a great shift in how data is collected and reported on could have impacts on views of data trust and data privacy by individuals.
This article uses longitudinal data from a country that has been relatively less affected by COVID-19, Australia, to examine how these attitudes may be changing during the COVID-19 period.
Aggregate data collected during the COVID-19 period ultimately comes from individuals or individual businesses. This includes individuals who have been infected, individuals who have been tested and found to be negative, individuals who have lost their job, and businesses that have experienced significant economic harm. Data about individuals is collected by both government and nongovernment organizations and increasingly we are generating electronic data about our lives that is being held by businesses. For example, the shift toward electronic payments, which has accelerated post COVID-19 (De Vito and Gomez, Reference De Vito and Gomez2020), has created a wealth of data for those institutions that administer the electronic payments system, some of which have been utilized for public policy purposes (Baker et al., Reference Baker, Farrokhnia, Meyer, Pagel and Yannelis2020). People are utilizing social media more often, partly because of an increase in demand for information about COVID-19, but also because of restrictions on other forms of social interaction (Cinelli et al., Reference Cinelli, Quattrociocchi, Galeazzi, Valensise, Brugnoli, Schmidt, Zola, Zollo and Scala2020). People’s movements have also been tracked, with that information used to predict the spread of the Coronavirus, as well as the effectiveness of physical distancing policies (Zhou et al., Reference Zhou, Su, Pei, Zhang, Du, Luo, Cao, Wang, Yuan, Zhu and Song2020).
There have also been a number of direct demands for our data in order to help with the tracking and tracing of contact with those who are suspected of having been infected (Whitelaw et al., Reference Whitelaw, Mamas, Topol and Van Spall2020). At various points throughout the pandemic individuals have been asked to give their name and telephone number and/or e-mail address when visiting commercial establishments, either through analogue technologies (pen and paper) or through Quick Response (QR) technologies (Chen and Thio, Reference Chen and Thio2021; Grekousis and Liu, Reference Grekousis and Liu2021; Shahroz et al., Reference Shahroz, Ahmad, Younis, Ahmad, Boulos, Vinuesa and Qadi2021). At a national level, a number of countries including Australia have designed or endorsed tracking applications on mobile phones that record people who an individual has come into close proximity with, using a variety of technologies and methods.
While there are still significant limits on accessing data from outside of government, there are examples of data being shared across organizations for both commercial, research, and policy purposes. Witness, for example, the use of payroll tax data by the Australian Bureau of Statistics to monitor the changing labor market during the COVID-19 pandemic (Australian Bureau of Statistics, 2020), or the use of credit card data by the Commonwealth Bank to track changes in consumption patterns.Footnote 1 While this data analysis and data sharing has potential benefits for policy development and public debate, any increase in the collection or sharing of data will all else being equal lead to an increased privacy risk. There are a range of mechanisms that can be put in place to minimize this risk and collectively society may see these risks as being outweighed by the benefits. Risks nonetheless remain.
For all this data collection and aggregation to be effective, it requires individuals to willingly provide their personal information, consenting explicitly or implicitly. The data environment during the COVID-19 pandemic has the potential to be affected by the public’s views on the safety of their own data, as well as shape these views. Gerdon et al. (Reference Gerdon, Nissenbaum, Bach, Kreuter and Zins2021) made use of a pre-existing vignette study looking at the use of data in April 2020 compared to July 2019, finding that “Public acceptance of the use of individual health data to combat an infectious disease outbreak increased notably between the two measurements, while acceptance of data use in several other scenarios barely changed over time.”
The aim of this article is to capture such relationships using high-quality longitudinal survey data.
This article seeks to better understand to what extent have attitudes toward data privacy and data security changed during the COVID-19 period, and the factors associated with this change.
This article will firstly outline the methodology and data used, before going on to outline the results, and finally provide some concluding remarks.
2. Methodology
2.1. Survey methodology
The article is primarily based on the May 2020 ANUpoll (the 34th ANUpoll) which collected data from a representative sample of the Australian population from Life in Australia, Australia’s only probabilistic, longitudinal panel.Footnote 2 Most of the panel members who completed the May 2020 ANUpoll (the 38th Wave of data collection on Life in Australia) had also completed the April 2020 ANUpoll (Wave 37) and the February survey (Wave 35). That is, they are the same individuals. The longitudinal nature of our data allows us to look at the changes in outcomes at the individual level.Footnote 3 The May 2020 ANUpoll collected information from 3,249 respondents aged 18 years and over across all eight States/Territories in Australia, and is weighted to have a similar distribution to the Australian population across key demographic and geographic variables.Footnote 4
The vast majority of respondents completed the surveys online, with a small proportion of respondents enumerated over the phone. For the May 2020 ANUpoll, about half of respondents (1,555) completed the survey on May 12 or 13, with the remaining respondents interviewed between May 14 and 24.Footnote 5
Of those individuals who completed the May 2020 ANUpoll, 91.6% or 2,976 individuals had completed the February 2020 survey (Table A1). The linkage rate was slightly higher with the April 2020 ANUpoll with 2,984 individuals or 91.8% of the May respondents having completed the survey in the previous month. Data for this survey is available through the Australian Data Archive (doi: 10.26193/GNEHCQ).
We also use information from individuals surveyed in October 2018 (Wave 21, Data Governance ANUpoll) and October 2019 (Wave 31, Crime and Justice ANUpoll). Of those individuals who completed the May 2020 ANUpoll, 55.4% or 1,800 individuals had completed the October 2018 survey. The linkage rate was slightly lower with the October 2019 ANUpoll with 1,651 individuals or 50.8% of the May respondents having completed that survey. The proportion of respondents to the May 2020 ANUpoll who responded to the 2018 and 2019 surveys is much lower because between October–December 2019, the panel was refreshed with 347 panelists being retired from the panel and 1,810 new panelists being recruited. Neither the retired nor new panelists are available in the linked sample.Footnote 6
2.2. Data items and analysis approach
There were a number of questions asked on the May 2020 and October 2018/2019 ANUpolls that were used to examine attitudes toward data trust and data privacy. The first of the questions outlined below was developed specifically for the October 2018 survey and designed to be consistent with a general trust question that had been asked on previous ANUpolls. The second and third questions were adapted from existing international surveys for cross-national comparisons. They were kept consistent through time to facilitate longitudinal analysis, and there was therefore very little scope for changes for the May 2020 survey.
In the May 2020 survey, respondents were first asked: “On a scale of 1–10, where 1 is no trust at all and 10 is trust completely, how much would you trust the following types of organizations to maintain the privacy of your data?” We asked about eight types of organizations, with the order randomized. These were:
-
a) The Commonwealth Government in general,
-
b) The State/Territory Government where you live,
-
c) Banks and other financial institutions,
-
d) Social media companies (e.g., Facebook, Twitter, and Google),
-
e) Universities and other academic institutions,
-
f) Telecommunications companies,
-
g) Companies that you use to make purchases online, and
-
h) The Australian Bureau of Statistics.
An index was constructed using principal components analysisFootnote 7 and standardized to have a mean of zero and standard deviation of one across the sample. Principal components analysis is an appropriate technique to use given that the index is used in this article as a point in time measure. A higher value of the index indicates that the individual has a higher level of trust in the overall ability of the different types of organizations to maintain data privacy.
Secondly, respondents were asked: “Please indicate how much you agree or disagree with the following statements.” The statements were randomized, with response options of “totally agree; tend to agree; tend to disagree; and totally disagree.” Statements included:
-
a) You are concerned that your online personal information is not kept secure by websites,
-
b) You are concerned that your online personal information is not kept secure by public authorities,
-
c) You avoid disclosing personal information online,
-
d) You believe the risk of becoming a victim of cybercrime is increasing, and
-
e) You are able to protect yourself sufficiently against cybercrime, for example, by using antivirus software on.
Finally, respondents were asked: “Cybercrimes can include many different types of criminal activity. How concerned are you personally about experiencing or being a victim of the following situations…?” Response options were “Very concerned; Fairly concerned; Not very concerned; and Not at all concerned” and the two specific situations that were asked about (with random ordering) were:
-
a) Identity theft (somebody stealing your personal data and impersonating you) and
-
b) Receiving fraudulent emails or phone calls asking for your personal details (including access to your computer, logins, banking, or payment information).
In order to measure the overall level of concern about the security of personal data, an index was produced which combined the seven questions on level of concern for data security and personal information. The index varies from a value of 7 for those who were least concerned about personal information and data to 28 for those who were most concerned.
A second index was then constructed using only the May 2020 survey data based on a principal components analysis.Footnote 8 This index is scaled to have a mean of zero and standard deviation of one, with higher values for those who have greater levels of concern.
Descriptive statistics were produced using each of these measures, with the factors associated with data trust and attitudes toward data privacy examined. While the above questions were exactly the same in the two surveys, it should be noted that the questions leading up to them were quite different. In particular, the May 2020 survey had a range of questions focused on COVID-19 impacts, substance use, and relationship status, with the module immediately preceding the data trust questions being on gambling behavior over the previous 12 months. There may therefore be some priming effects and thus some caution in interpreting changes over time is warranted, especially if the changes in responses are only small. Fortunately, one specific data-relevant question on contact tracing app usage was asked after the questions analyzed in this article. Furthermore, as will be seen in Section 3, changes through time are quite large, and not universal across the sample, suggesting that the differences are real rather than question-order driven. In the absence of an experimental design that randomizes the question ordering, however, it is not possible to discount priming effects.
3. Results
3.1. Trust in organizations to maintain data privacy
This section reports data on Australian’s level of trust in different types of organizations to maintain the privacy of their personal data and how this has changed since October 2018. There has been a statistically significant increase in the extent to which Australian’s say that they trust all eight types of organizations asked about to maintain data privacy (Figure 1). The largest improvements, of just under 30%, was in the level of trust in for companies that are used to make purchases online, banks and other financial institutions, and telecommunications companies.
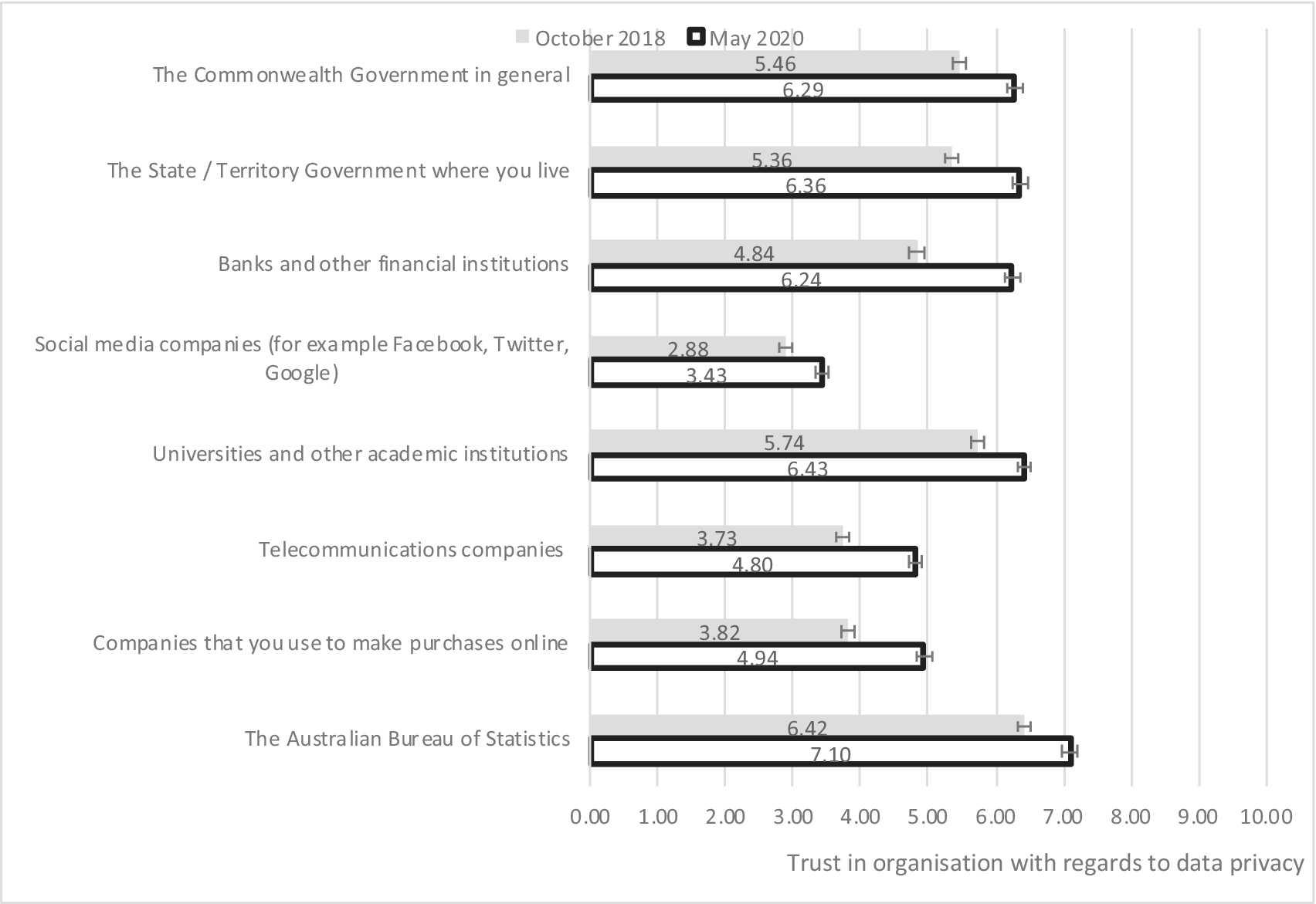
Figure 1. Average trust in types of organizations to maintain privacy of data, October 2018 and May 2020. The “whiskers” on the bars indicate the 95% confidence intervals for the estimate.
Source: ANUpoll, October 2018 and May 2020.
Cross-sectionally, the highest level of trust is in the Australian Bureau of Statistics, followed by universities and other academic institutions, state and territory governments and the Commonwealth Government. On the other hand, very low levels of trust social media companies maintaining data privacy are reported.
The changes observed between October 2018 and May 2020 in trust in institutions are comparable in size to changes in confidence in key institutions over the COVID-19 period (a relationship we will return to in Section 3.1.2). For example, between January 2020 and April 2020 there was an increase in the per cent of Australians who were confident or very confident in the Federal Government from 27.3 to 56.6%.
3.1.1. Factors associated with trust in organizations to maintain data privacy
There is a very strong correlation between trust in one type of organization and trust in many of the other types of organizations. The strongest correlation (Table A2) is between trust in the Commonwealth Government and trust in State/Territory governments whereas the weakest correlation is between trust in social media companies and trust in the Australian Bureau of Statistics.
This high correlation means that the responses to the question about trust in the ability of each type of organization to maintain data privacy can be used to construct an overall index of trust, described in Section 2, which has a mean of zero and a standard deviation of one, where a higher value of the index indicates a higher level of trust.
Table 1 reports estimates from a regression model of the associations between individual level demographic, socioeconomic and geographic variables, and overall level of trust in organizations to maintain data privacy. The covariates in the model are included in order to describe variation across key, policy-relevant groups in Australia.
Table 1. Factors associated (a) with overall trust in organizations to maintain privacy of data, May 2020
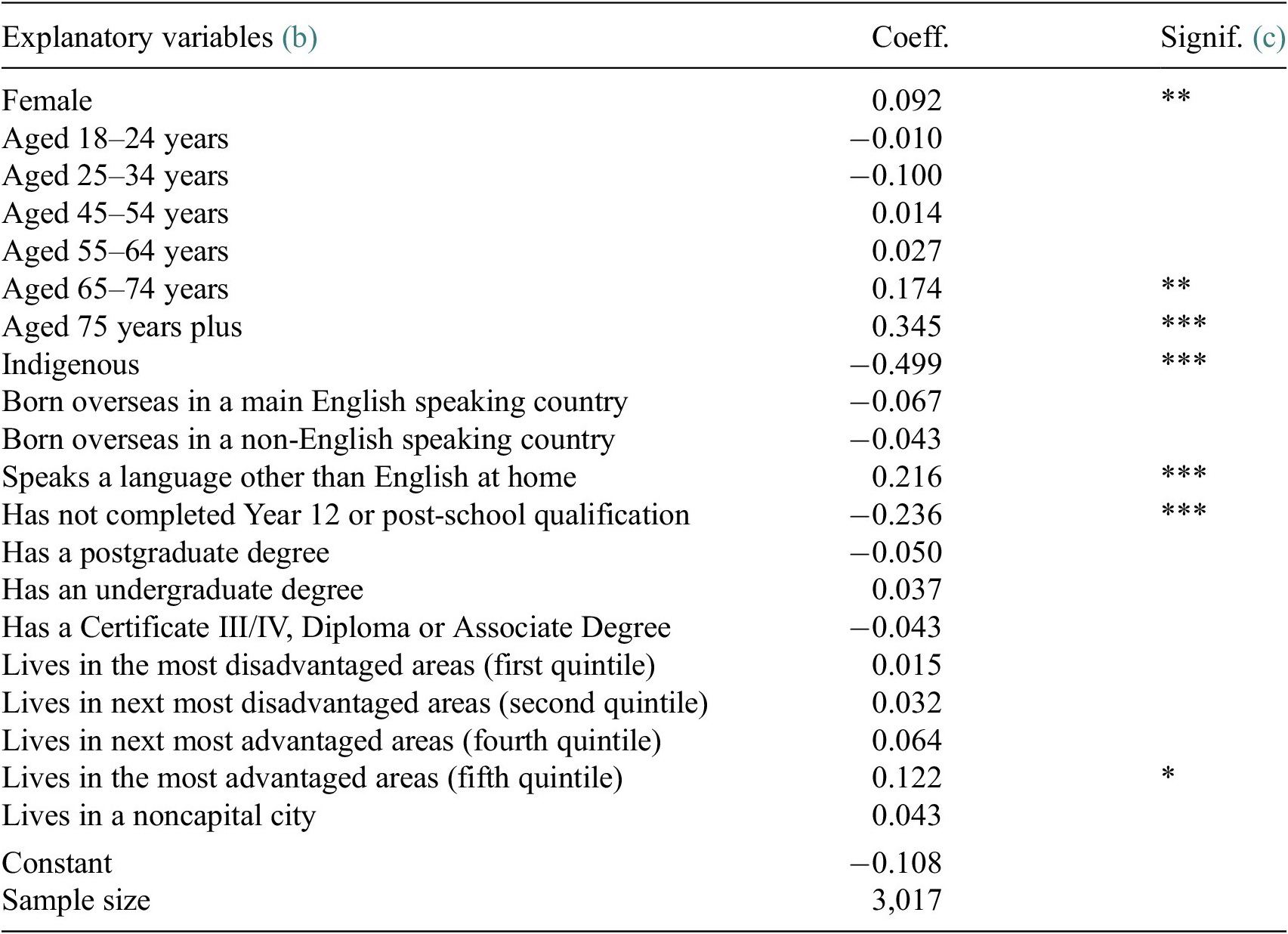
a Linear Regression Model.
b The base case individual is female; aged 35–44; non-Indigenous; born in Australia; does not speak a language other than English at home; has completed Year 12 but does not have a postgraduate degree; lives in neither an advantaged or disadvantaged suburb (third quintile); and lives in a capital city.
c Coefficients that are statistically significant at the 1% level of significance are labeled ***, those significant at the 5% level of significance are labeled **, and those significant at the 10% level of significance are labeled *.
Source: ANUpoll, May 2020.
Females are more likely to trust organizations to maintain the privacy of their data (relative to males), as are those aged 65 years and over (compared to those aged 35–44 years). Indigenous Australians are significantly and substantially less likely to trust organizations with their data, with the difference almost exactly equal to one-half of a standard deviation. This potentially reflects a past, negative experience with government for Indigenous Australians, and is a potential reason for the strong recent push for data sovereignty amongst Indigenous peoples in Australia and internationally (Kukutai and Taylor, Reference Kukutai and Taylor2016). Those who speak a language other than English at home are more trusting, whereas those who have not completed Year 12 are less trusting than those who have completed Year 12, with the difference equal to around one-quarter of a standard deviation. Similar associations were the October 2018 data was analyzed as a cross-sectional dataset (Biddle et al., Reference Biddle, Edwards, Gray and McEachern2018).
3.1.2. Factors associated with change in trust over time
By examining the data longitudinally, we are able to measure the individual level factors associated with changes in trust in organizations to maintain data privacy between 2018 and 2020. Change in trust of organization to maintain data privacy is measured using the change between 2018 and 2020 in the average level of trust across the eight types of organizations asked about. The average is used rather than an index generated from principal components analysis because the principal components-based index would, by construction, have the same average in both years and therefore not be useful for measuring change. The average overall level of trust in organizations to maintain data privacy increased from 4.78 to 5.70 between 2018 and 2020 based on the full samples for each year and by 0.81 on average for the sample that responded to both the 2018 and 2020 surveys (i.e., the longitudinal sample).
In order to estimate the factors associated with changes between 2018 and 2020 in level of trust in organization to maintain data privacy a regression model is used. Two models are estimated. The first model includes the same covariates as in Table 1 in order to test whether the demographic, socioeconomic and geographic variables have the same association with change through time in trust as they do with the cross-sectional measure.
The second model includes additional variables derived from the January, February, April and May 2020 surveys that relate to people’s potential determinants of trust. These variables are included to test whether changes in broader trust measures are associated with changes in trust with regards to data privacy. There are a number of potential reasons why people’s trust measures might improve, including their general view on the organizations involved (i.e., not specifically related to data), specific changes in policy or practice related to data, and the individual’s own experience. While the data we use in our analysis was not designed to answer these questions directly, there are a number of variables that do capture these potential determinants of change.
Specifically, the first set of variables measures change in average confidence in three institutions—the Federal Government in Canberra; the public service; and the State/Territory Government in which the person lives. Values range from 1 (none at all) to 4 (a great deal of confidence). We include baseline measures of confidence in January to control for any potential changes between October 2018 and just prior to the spread of COVID-19, as well as the change between January 2020 and April 2020, with the average change for the linked sample being 0.209. Unfortunately, we do not have any measures of confidence in other types of organizations included in the trust in data privacy measure
The second set of variables measure social cohesion at a more individual level. In both February 2020 and April 2020, participants were asked whether most people can be trusted; whether people are fair (as opposed to taking advantage of others); and whether people are helpful. The responses are given on a scale from 0 to 10 where 0 is the most negative assessment and 10 the most positive. We include the average value across these three measures in February 2020, as well as the change in the average between February and April 2020 (with the average change in the average being 0.297).
The final two variables relate to whether the respondent had sought help for a list of issues and had no difficulties accessing services, whether they had sought help but had difficult accessing services or whether they had not sought help (either because they did not have any issues or they had issues but had not sought help) the omitted category in the regression model).Footnote 9
Three key sets of findings emerge from the analysis presented in Table 2. First, there are a number of demographic variables that are associated with changes in the overall level of trust in organizations to maintain privacy of data. Without controlling for the other government-related variables (i.e., focusing on Model 1), females, those aged 45 years and over, and those who live in relatively advantaged areas have increased their trust with regards to data privacy between October 2018 and May 2020. This cannot be directly attributable to COVID-19 as there have been other changes in Australia over the period. However, these results do show that improvements in trust during COVID-19 have been concentrated in certain groups, rather than being consistent across the population.
Table 2. Factors associated (a) with change in trust in types of organizations to maintain privacy of data (change between October 2018 and May 2020)
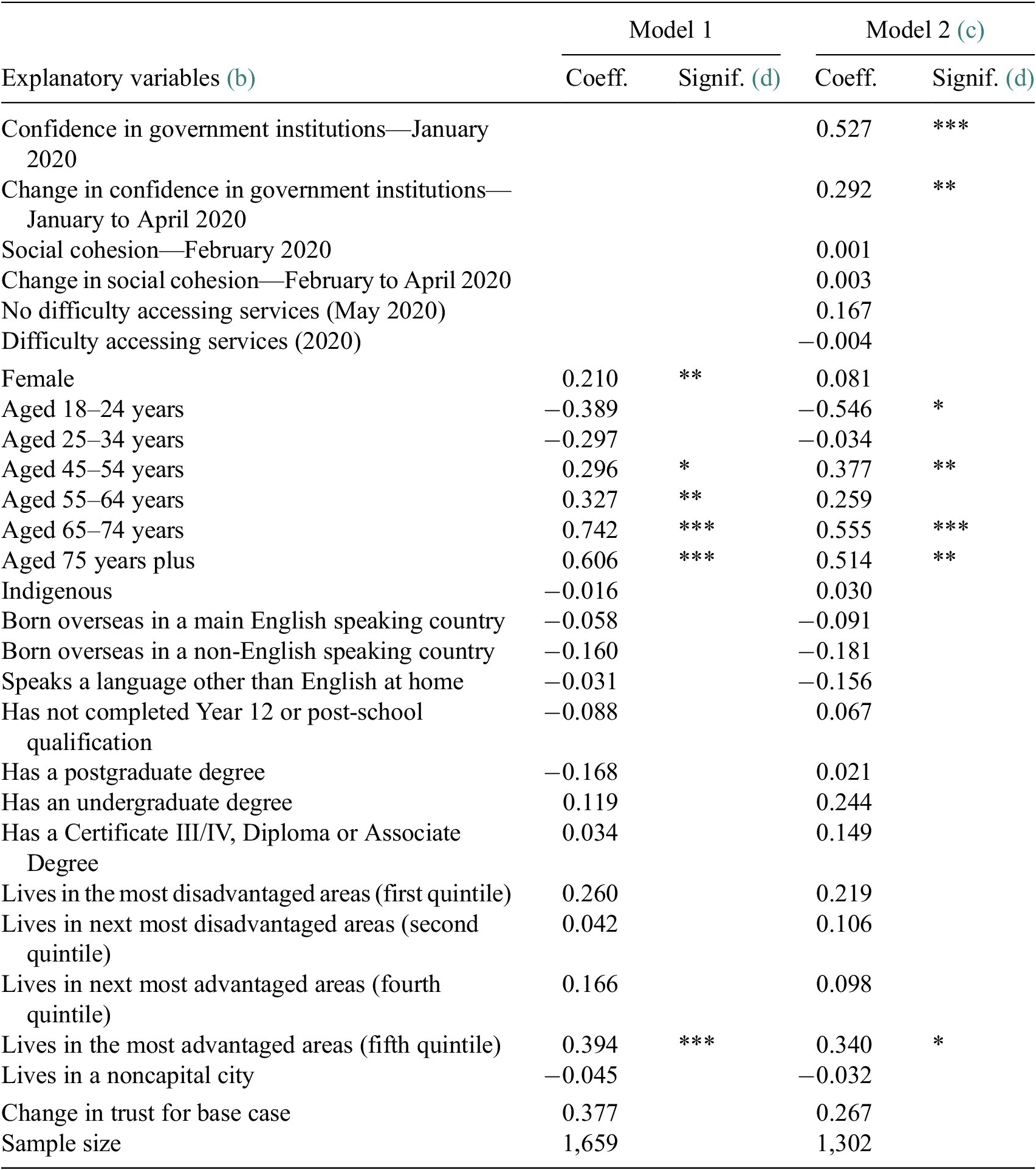
a Linear Regression Model.
b The base case individual for Model 1 is female; aged 35–44; non-Indigenous; born in Australia; does not speak a language other than English at home; has completed Year 12 but does not have a postgraduate degree; lives in neither an advantaged or disadvantaged suburb (third quintile); and lives in a capital city.
c For Model 2, the base case is further defined as having the average levels of confidence in government institutions as observed in January 2020 (2.47) and the average levels of social cohesion as observed in February 2020 (5.65), but no change in these measures from the baseline until April.
d Coefficients that are statistically significant at the 1% level of significance are labeled ***, those significant at the 5% level of significance are labeled **, and those significant at the 10% level of significance are labeled *.
Source: ANUpoll, October 2018, January 2020, April 2020, and May 2020 and Life in Australia, February 2020.
The second important thing to note is that of the three sets of variables included in Model 2, levels and changes in confidence in government institutions have the strongest association with change in trust. There is a positive and not insubstantial coefficient for the positive interaction with service providers variable, but it is not quite statistically significant at the standard levels of significance (p-value = .163). There is only weak evidence therefore that a positive interaction with service providers during the COVID-19 pandemic has led to an improvement in trust with regards to data privacy.
There is much stronger evidence that improvements in overall confidence in government institutions is associated with improvements in trust with regards to data privacy. Average levels of confidence in January 2020 is associated with improvements in trust between October 2018 and May 2020. This may be due to reverse causality (change in trust with regards to data leading to changes in confidence in government), or it may reflect the effect of any changes in confidence from October 2018 to January 2020. Unfortunately, we do not have data from the same individuals on confidence in government prior to October 2018 in order to measure this directly.
The finding that changes in confidence in government between January 2020 and April 2020 is also associated with changes in trust in organizations does, however, suggest that the generally positive view that individuals had toward government in Australia during the first few months of the pandemic is a key predictor of the improvement in trust with regards to data privacy (Evans et al., Reference Evans, Valgardsson, Jennings and Stoker2020). The counterpoint to that though is that if views toward the government with regards to how the COVID-19 pandemic is being handled worsen, then trust in organizations with regards to data also might change. This is reinforced by the final point from Table 2, which is that measures of social cohesion (i.e., the extent to which people trust other Australians) were not statistically significant and that the predicted change in trust for the omitted category declined between Model 1 and Model 2. Improvements in trust may not be permanent and enduring if the general positive views toward government because of the relatively low infection and mortality rate at the early stages of the COVID-19 pandemic do not continue.
3.2. Level of concern regarding personal data and information
While trust in organizations to maintain data privacy is a somewhat abstract concept, the way in which people make decisions with regards to their own personal data may also be impacted on by more immediate and direct threats and concerns. There have been some reductions in concern with regards to data breaches and data privacy with individual’s own data between October 2019 and May 2020 (Figure 2). However, the change has not been as large and consistent as for trust in organizations (possibly because the change in level of concern about personal data and information is measured over a shorter time period).
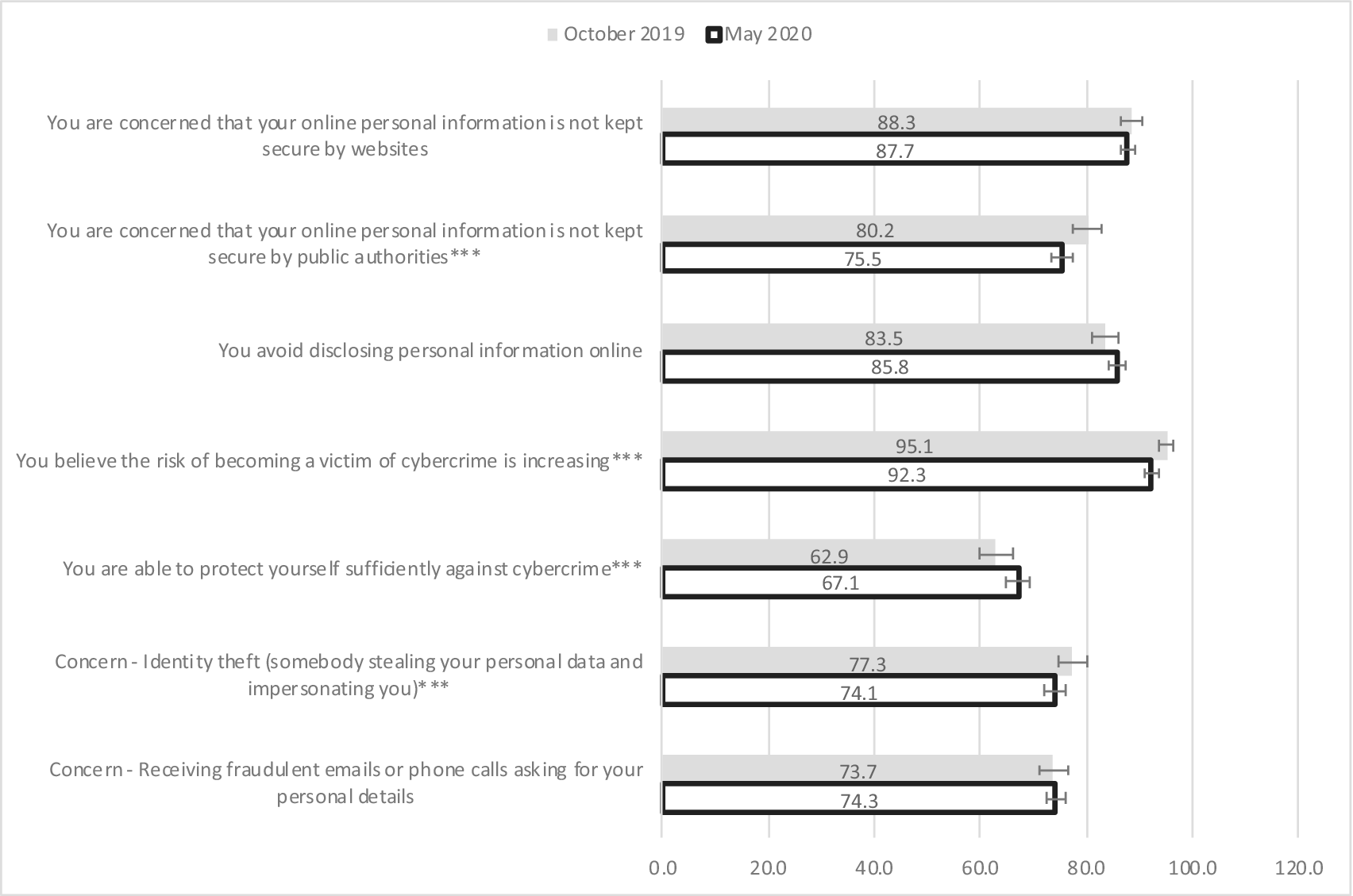
Figure 2. Per cent of Australians who tended to agree or totally agree that they are concerned about the security of their personal data and information, October 2019 and May 2020. The “whiskers” on the bars indicate the 95% confidence intervals for the estimate. Differences between October 2019 and May 2020 that are statistically significant at the 1% level of significance are labeled ***; those significant at the 5% level of significance are labeled **, and those significant at the 10% level of significance are labeled *.
Source: ANUpoll, October 2019 and May 2020.
In May 2020 the highest level of concern was about their personal information not being kept secure by websites (87.7% tend to agree or totally agree that they were concerned about this), with very similar levels of concerns about their information not being kept secure by public authorities (75.5%), identity theft (74.1%) and about receiving fraudulent emails or phone calls asking for personal details (74.3%).
There was a statistically significant decline in concern about public authorities (from 80.2% in October 2019 to 75.5% in May 2020) and no change in in concern about websites in general (from 88.3% in October 2019 and 87.7% in May 2020).
We did not observe any change in self-reported behavior, with roughly the same percentage of people in May 2020 saying that they avoid disclosing personal information online (85.8%) compared to October 2019 (83.5%). However, there was a significantly smaller per cent of the population who think that the risk of being a victim of cybercrime is increasing (92.3% in May 2020 compared to 95.1% in October 2019) and a significantly larger per cent who feel that they are able to protect themselves against cybercrime (from 62.9 to 67.1%). Finally, there was a small decline in the level of concern about identity theft (77.3% in May 2020 compared to 74.1% in October 2019), but no significant change in concern about receiving fraudulent emails or phone calls.
Using the index measuring level of concern of personal data, which ranges from a value of 7 for those who were least concerned about personal information and data, to 28 for those who were more concerned, we found that between October 2019 and May 2020, the index declined from 21.4 to 20.8, equivalent to around one-fifth of a standard deviation of the baseline (October 2019) data.Footnote 10 By comparison, the decline in the aggregate score for the trust in organizations additive index was equal to slightly over one-half of a standard deviation.
There is a reasonably strong negative correlation between people’s trust in organizations with regards to data privacy and their concern about personal data (coefficient = −0.3039). There is a weaker (though still negative) correlation between changes in the two measures (coefficient = −0.0909). As shown in Table 3, the determinants of concern are also quite different.
Table 3. Factors associated (b) with index of concern (a) with regards to security of personal information and data, May 2020
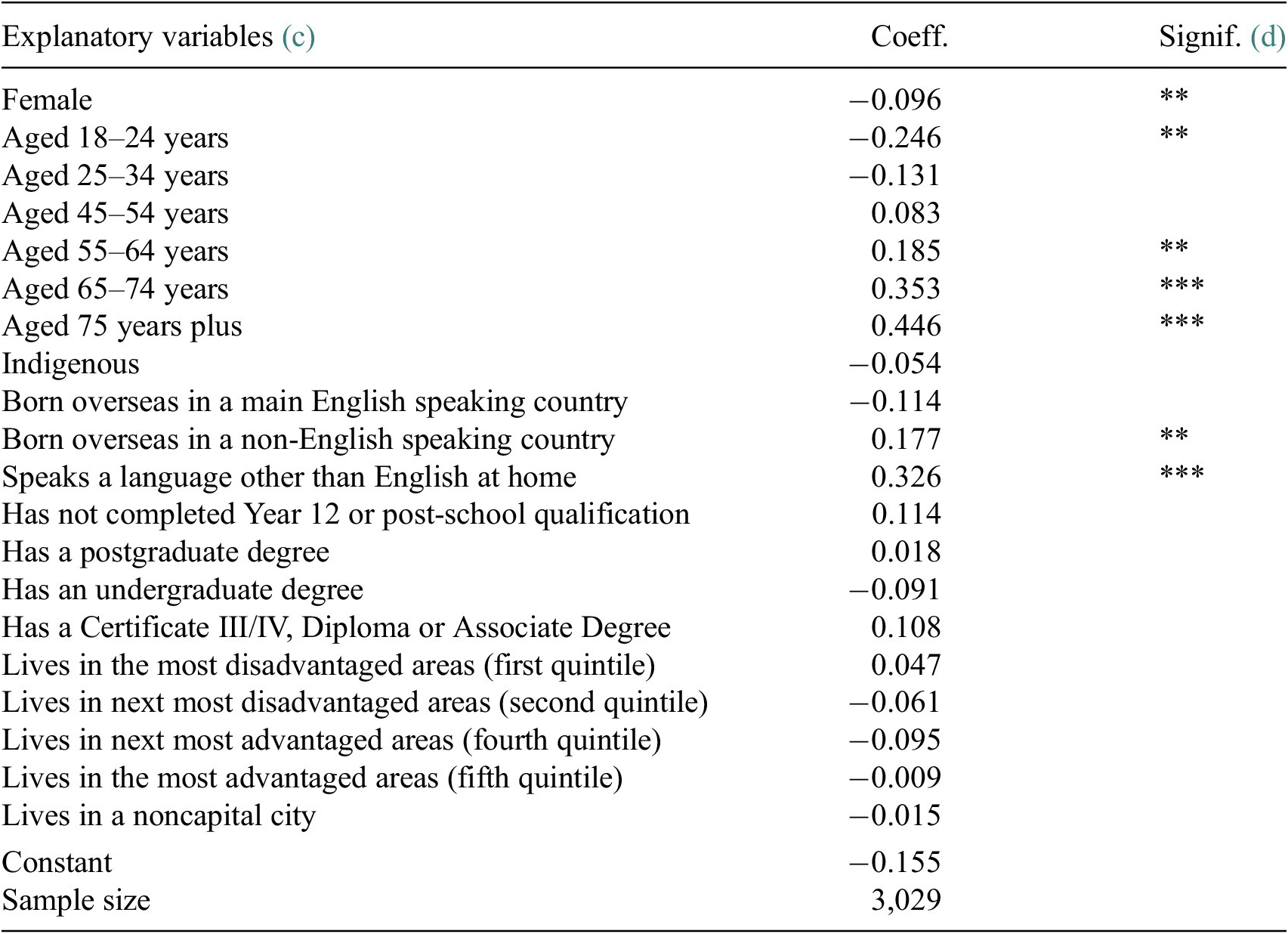
a Indexed of concern about security of data and personal information is derived using the results of principal component analysis and has a mean of zero and a standard deviation of one.
b Linear Regression Model.
c The base case individual is female; aged 35–44; non-Indigenous; born in Australia; does not speak a language other than English at home; has completed Year 12 but does not have a postgraduate degree; lives in neither an advantaged or disadvantaged suburb (third quintile); and lives in a capital city.
d Coefficients that are statistically significant at the 1% level of significance are labeled ***, those significant at the 5% level of significance are labeled **, and those significant at the 10% level of significance are labeled *.
Source: ANUpoll, May 2020.
Using a second index based only on the May 2020 survey developing using principal components analysis, the association between individual level demographic and socioeconomic characteristics and geographic variables and the index of concern about security of data and personal information is estimated using a regression model and the results reported in Table 3. Females are estimated to report a lower level of concern than males, which is consistent with the finding from above that they have more trust in organizations with regards to their own data. The age differences, however, go in opposite directions to what we might expect based on the earlier results. Specifically, although older Australians have a greater level of trust in organizations with regards to data privacy, they are significantly (and substantially) more likely to express concerns with regards to their personal information and data. Specifically, controlling for other factors, those aged 75 years and over had an almost one-half a standard deviation higher value in the index than those aged 35–44 years, and an almost three-quarters of a standard deviation higher value than those aged 18–24 years. Finally, there are no differences with regards to education or socioeconomic measures, but there are some differences by ethnicity with those born in a non-English speaking country or who speak a language other than English at home being more likely to be concerned.
There are no demographic or socioeconomic variables that are associated with change in concerns regarding data and personal information, apart from those aged 65–74 years having a greater increase than those aged 35–44 years. There was also no correlation with generalized confidence in the Federal Government. There was, however, a significant association with difficulties accessing services. Specifically, those who did not need to access any services in the two months leading up to the May 2020 survey had a decline in the additive index of 0.91. For those who had a need, but had no barriers to access, the decline was equal to 0.97 (difference not statistically significant). However, for those who had barriers to accessing services, we observed an increase in the level of concern regarding personal information and data of 0.13, with that difference statistically significant at the 1% level of significance.
4. Conclusion
As a result of the COVID-19 threat, there has been an increased focus on the use of individual level data to track and respond to the spread of the pandemic. The level of trust, confidence and concerns of the Australian population about sharing their personal data and how it is shared and used is critical to the extent to which governments are able to use personal data to monitor and control the spread of COVID-19. And in turn the extent to which governments’ protect personal data will help shape the views of Australians about how their data is shared and used into the future.
This article provides new data on Australian’s attitudes toward data privacy and security and how these attitudes have changed since COVID-19. A novel feature of the data used in this article is that it collected from the same group of people before and after COVID-19 which allows the individual level factors associated with changes in attitudes to be estimated. On balance, it would appear that during the COVID-19 period Australians have become more trusting of organizations with regards to data privacy and less concerned about their own personal information and data. An important finding that is slightly different to that found in Gerdon et al. (Reference Gerdon, Nissenbaum, Bach, Kreuter and Zins2021) is that in Australia trust in institutions increased regardless of their relevance to the pandemic itself. Combined, the two studies help to add nuance to the contextual integrity theory outlined in Gerdon et al. (Reference Gerdon, Nissenbaum, Bach, Kreuter and Zins2021).
The increases in trust in organizations to maintain the privacy of personal data appears to be strongly related to the increases in confidence in the Federal government, State/Territory governments and the Public Service. That is, domain-specific trust in institutions with regards to data privacy is strongly correlated with generalized trust measures.
There are three key limits to this article that should be mentioned that are of relevance when interpreting the results. First, compared to most other high income countries in Europe and North America, Australia has had very low COVID-19 infection and mortality rates. That is in some ways what makes the Australian case study interesting, but it does limit the comparability. Second, our longitudinal sample is much smaller than the May 2020 cross-section. While we have tested and rejected the longitudinal sample having different outcome measures compared to the rest of the May 2020 survey, it is still possible that multiple biases may be operating in different directions. A final limitation is that our outcome measures capture perceptions, rather than behaviors directly related to data privacy. In future work, we intend to make use of other questions on the survey to test the associations with a limited set of COVID-specific behaviors.
There is a certain irony in us as researchers using somewhat sensitive data on individuals who are tracked through time to measure trust in data privacy and its effects. However, respondents to our surveys provide informed consent for their data to be used in such a way, with their data kept confidential and no personally identifiable information available to us as researchers. We would argue also that papers like this highlight the value of high-quality longitudinal survey data to supplement and corroborate data generated through the delivery of services and administration of public policy. We would also note that, unlike some of the other data collected and used to make policy recommendations and evaluations during the COVID-19 period that we have made the data freely available through the Australian Data Archive for validation and interrogation. We would argue that not providing data to individuals outside one’s own organization or research team or not doing so in a safe and privacy preserving way has the potential to undermine trust in the data ecosystem.
Ultimately, what we think the data analyzed and presented in this article has shown is that there are large negative externalities of eroding trust amongst the general population in the privacy of data. It may be tempting for individuals in all the types of organizations that we ask about in our survey (including academic institutions) to take risks with people’s data, to be less than transparent, and to use that data to cause the individuals harm. However, taking such risks undermines trust in the data ecosystem, which makes policy interventions that much harder, less effective, and more costly when they are really needed, like during a global pandemic. On a more positive note though, there are positive externalities and the relatively transparent use of high-quality data to help track the health, economic, and social impacts of COVID-19 appears to have rebuilt some of that trust. It would be a real shame if that was again eroded.
Acknowledgments
The authors would like to thank Matthew James and Cathy Claydon for the considerable input into the design of the survey. The authors would also like to thank a number of people who were involved in the development of the May 2020 ANUpoll questionnaire, including Diane Herz, Dr Benjamin Phillips, Dr Paul Myers, Matilda Page, and Charles Dove from the Social Research Centre, as well as Professor Ian McAllister.
Funding Statement
The May ANUpoll was partially funded by the Australian Institute of Health and Welfare (AIHW).
Competing Interests
The authors declare no competing interests exist.
Author Contributions
All authors approved the final submitted draft. Conceptualization: N.B., M.H.; Data curation: N.B., B.E.; Formal analysis: N.B.; Methodology: N.B., B.E., M.G., M.H.; Writing—original draft: N.B.; Writing—review and editing: B.E., M.G., S.M., K.S.
Data Availability Statement
Data can be accessed through the Australian Data Archive, doi: 10.26193/GNEHCQ.
Appendix
Table A1. Wave by Wave data description and linkage
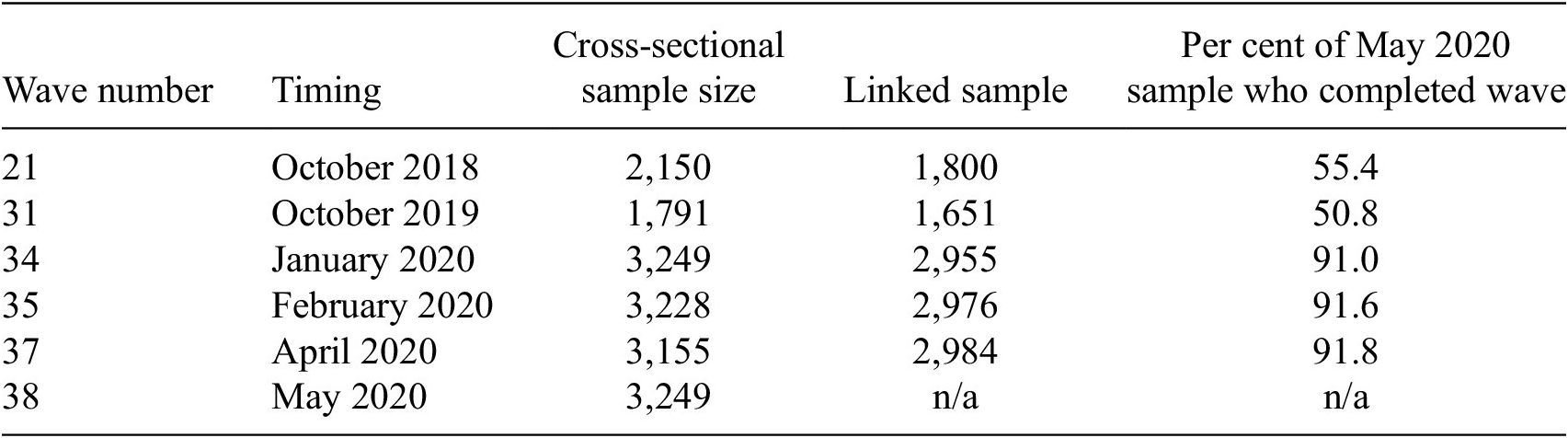
Table A2. Correlation with trust in different types of organizations to maintain privacy of data, May 2020
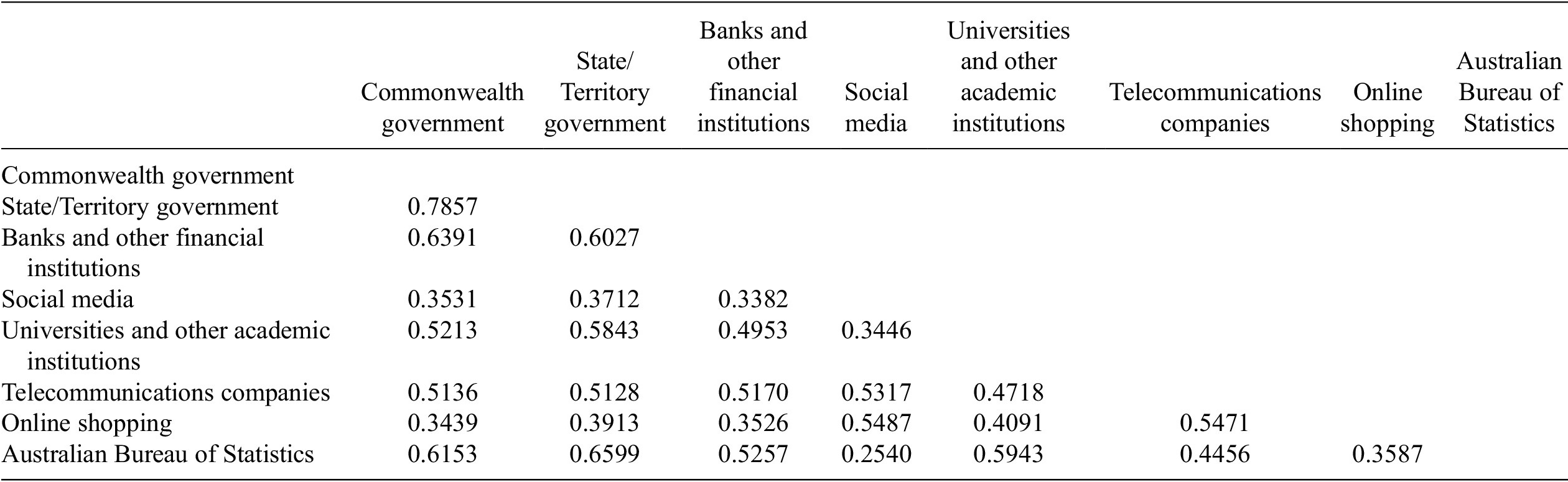
Source: ANUpoll, May 2020.
Comments
No Comments have been published for this article.