Significant outcomes
-
Cerebrospinal fluid neurofilament light chain (CSF NfL) test accurately predicted whether a patient had a neurodegenerative or psychiatric disorder for 88% of cases in a real-world, inclusive, clinical cohort.
-
On the other hand, clinical assessment alone based on gold standard comprehensive diagnostic work-up still saw 23% of patients experience diagnostic delay with longitudinal follow-up.
-
CSF NfL, in retrospect, could have aided clinicians in avoiding a delayed diagnosis for 11% of patients compared to clinical assessment alone adds further weight to the current international recommendations that CSF biomarkers are incorporated into routine practice.
Limitations
-
The retrospective design comes with inherent drawbacks, including the reliance on historical diagnostic formulations.
-
Despite obtaining several years of follow-up information (average ∼3 years) with serial assessments for most patients, more insights may be yielded with longer follow-up and post-mortem pathological confirmation.
Introduction
Accurate diagnosis of neuropsychiatric and cognitive presentations can be challenging due to reliance on clinical symptoms, which often overlap between neurodegenerative (ND) and psychiatric disorders (PSY) (Woolley et al., Reference Woolley, Khan, Murthy, Miller and Rankin2011; Lanata and Miller, Reference Lanata and Miller2016; de Boer et al., Reference de Boer, Gossink, Krudop, Vijverberg, Schouws, Reus, Pijnenburg and Dols2023). Low mood and memory loss can occur in both Alzheimer’s disease (AD) and late-life depression, creating diagnostic uncertainty (Woolley et al., Reference Woolley, Khan, Murthy, Miller and Rankin2011). Behavioural variant frontotemporal dementia (bvFTD) is particularly prone to misdiagnosis, as it mimics PSY (Tsoukra et al., Reference Tsoukra, Velakoulis, Wibawa, Malpas, Walterfang, Evans, Farrand, Kelso, Eratne and Loi2021).
The difficulty of differentiating between ND and PSY is evident in the high rate of misdiagnoses. A large clinical trial found that 35% of patients with a clinical diagnosis of AD had negative amyloid-beta positron emission tomography scans (Salloway et al., Reference Salloway, Sperling, Fox, Blennow, Klunk, Raskind, Sabbagh, Honig, Porsteinsson, Ferris, Reichert, Ketter, Nejadnik, Guenzler, Miloslavsky, Wang, Lu, Lull, Tudor, Liu, Grundman, Yuen, Black and Brashear2014). Recent studies have found that 15–21% of patients assessed at tertiary memory clinics switched diagnoses between PSY and ND during follow-up (Tsoukra et al., Reference Tsoukra, Velakoulis, Wibawa, Malpas, Walterfang, Evans, Farrand, Kelso, Eratne and Loi2021; de Boer et al., Reference de Boer, Gossink, Krudop, Vijverberg, Schouws, Reus, Pijnenburg and Dols2023) with a mean delay of 3.4 years (Loi et al., Reference Loi, Goh, Mocellin, Malpas, Parker, Eratne, Farrand, Kelso, Evans, Walterfang and Velakoulis2020).
To address this problem, international groups have recommended that the diagnosis of dementia should incorporate both clinical and biomarker findings. For example, the recently established Neuropsychiatric International Consortium for Frontotemporal Dementia recommended cerebrospinal fluid (CSF) and blood biomarkers to aid clinicians in reaching an accurate diagnosis (Ducharme et al., Reference Ducharme, Dols, Laforce, Devenney, Kumfor, van den Stock, Dallaire-Théroux, Seelaar, Gossink, Vijverberg, Huey, Vandenbulcke, Masellis, Trieu, Onyike, Caramelli, de Souza, Santillo, Waldö, Landin-Romero, Piguet, Kelso, Eratne, Velakoulis, Ikeda, Perry, Pressman, Boeve, Vandenberghe, Mendez, Azuar, Levy, Le Ber, Baez, Lerner, Ellajosyula, Pasquier, Galimberti, Scarpini, van Swieten, Hornberger, Rosen, Hodges, Diehl-Schmid and Pijnenburg2020). Similarly, the current conceptual framework for AD incorporates CSF biomarkers in its criteria (Dubois et al., Reference Dubois, Feldman, Jacova, Hampel, Molinuevo, Blennow, DeKosky, Gauthier, Selkoe, Bateman, Cappa, Crutch, Engelborghs, Frisoni, Fox, Galasko, Habert, Jicha, Nordberg, Pasquier, Rabinovici, Robert, Rowe, Salloway, Sarazin, Epelbaum, de Souza, Vellas, Visser, Schneider, Stern, Scheltens and Cummings2014; Lewczuk et al., Reference Lewczuk, Riederer, O’Bryant, Verbeek, Dubois, Visser, Jellinger, Engelborghs, Ramirez, Parnetti, Jack, Teunissen, Hampel, Lleó, Jessen, Glodzik, de Leon, Fagan, Molinuevo, Jansen, Winblad, Shaw, Andreasson, Otto, Mollenhauer, Wiltfang, Turner, Zerr, Handels, Thompson, Johansson, Ermann, Trojanowski, Karaca, Wagner, Oeckl, van Waalwijk van Doorn, Bjerke, Kapogiannis, Kuiperij, Farotti, Li, Gordon, Epelbaum, Vos, Klijn, Van Nostrand, Minguillon, Schmitz, Gallo, Lopez Mato, Thibaut, Lista, Alcolea, Zetterberg, Blennow and Kornhuber2018).
Despite the heterogeneity of neurodegenerative and psychiatric disorders, neurofilament light chain (NfL) in CSF has shown promise as a diagnostic discriminator (Eratne et al., Reference Eratne, Loi, Walia, Farrand, Li, Varghese, Walterfang, Evans, Mocellin, Dhiman, Gupta, Malpas, Collins, Masters and Velakoulis2020; Eratne, Keem, et al., Reference Eratne, Keem, Lewis, Kang, Walterfang, Farrand, Loi, Kelso, Cadwallader, Berkovic, Li, Masters, Collins, Santillo and Velakoulis2022; Eratne, Loi, et al., Reference Eratne, Loi, Li, Stehmann, Malpas, Santillo, Janelidze, Cadwallader, Walia, Ney, Lewis, Senesi, Fowler, McGlade, Varghese, Ravanfar, Kelso, Farrand, Keem, Kang, Goh, Dhiman, Gupta, Watson, Yassi, Kaylor‐Hughes, Kanaan, Perucca, Dobson, Vivash, Ali, O'Brien, Hansson, Zetterberg, Blennow, Walterfang, Masters, Berkovic, Collins and Velakoulis2022; Fourier et al., Reference Fourier, Formaglio, Kaczorowski, Mollion, Perret‐Liaudet, Sauvee and Quadrio2020; Karantali et al., Reference Karantali, Kazis, Chatzikonstantinou, Petridis and Mavroudis2020). NfL, a neurofilament subunit, makes up the structure of the axonal skeleton expressed in large myelinated axons (Gafson et al., Reference Gafson, Barthélemy, Bomont, Carare, Durham, Julien, Kuhle, Leppert, Nixon, Weller, Zetterberg and Matthews2020). Elevated concentrations of NfL can indicate axonal injury and neurodegeneration (Bridel et al., Reference Bridel, van Wieringen, Zetterberg, Tijms, Teunissen, Andreasson, Axelsson, Bäckström, Bartos, Bjerke, Blennow, Boxer, Brundin, Burman, Christensen, Fialová, Forsgren, Frederiksen, Gisslén, Gray, Gunnarsson, Hall, Hansson, Herbert, Jakobsson, Jessen-Krut, Janelidze, Johannsson, Jonsson, Kappos, Khademi, Khalil, Kuhle, Landén, Leinonen, Logroscino, Lu, Lycke, Magdalinou, Malaspina, Mattsson, Meeter, Mehta, Modvig, Olsson, Paterson, Pérez-Santiago, Piehl, Pijnenburg, Pyykkö, Ragnarsson, Rojas, Romme Christensen, Sandberg, Scherling, Schott, Sellebjerg, Simone, Skillbäck, Stilund, Sundström, Svenningsson, Tortelli, Tortorella, Trentini, Troiano, Turner, van Swieten, Vågberg, Verbeek, Villar, Visser, Wallin, Weiss, Wikkelsø and Wild2019). NfL is elevated in other neurological disorders, including inflammatory, traumatic, and cerebrovascular disease (Gaetani et al., Reference Gaetani, Blennow, Calabresi, Filippo, Parnetti and Zetterberg2019). Furthermore, CSF NfL in mild cognitive impairment (MCI), a heterogenous group thought to capture the pre-dementia phase of cognitive decline (Petersen et al., Reference Petersen, Caracciolo, Brayne, Gauthier, Jelic and Fratiglioni2014), is elevated compared to healthy controls (Bridel et al., Reference Bridel, van Wieringen, Zetterberg, Tijms, Teunissen, Andreasson, Axelsson, Bäckström, Bartos, Bjerke, Blennow, Boxer, Brundin, Burman, Christensen, Fialová, Forsgren, Frederiksen, Gisslén, Gray, Gunnarsson, Hall, Hansson, Herbert, Jakobsson, Jessen-Krut, Janelidze, Johannsson, Jonsson, Kappos, Khademi, Khalil, Kuhle, Landén, Leinonen, Logroscino, Lu, Lycke, Magdalinou, Malaspina, Mattsson, Meeter, Mehta, Modvig, Olsson, Paterson, Pérez-Santiago, Piehl, Pijnenburg, Pyykkö, Ragnarsson, Rojas, Romme Christensen, Sandberg, Scherling, Schott, Sellebjerg, Simone, Skillbäck, Stilund, Sundström, Svenningsson, Tortelli, Tortorella, Trentini, Troiano, Turner, van Swieten, Vågberg, Verbeek, Villar, Visser, Wallin, Weiss, Wikkelsø and Wild2019; Zhang et al., Reference Zhang, Cheng, Liu, Li, Song and Jia2022).
Previous studies based on clinical settings, including our recent studies, have assessed whether CSF NfL concentrations correlated with the consensus diagnosis based on cross-sectional multimodal and multidisciplinary assessments (Eratne, Fourier et al., Reference Fourier, Formaglio, Kaczorowski, Mollion, Perret‐Liaudet, Sauvee and Quadrio2020; Loi, et al., Reference Loi, Walterfang, Kelso, Bevilacqua, Mocellin and Velakoulis2022; Gleerup et al., Reference Gleerup, Simonsen and Høgh2022). However, despite these comprehensive assessments, a significant proportion of patients’ clinical diagnoses are revised longitudinally, including between diagnostic categories (i.e. ND↔PSY) (Woolley et al., Reference Woolley, Khan, Murthy, Miller and Rankin2011; Tsoukra et al., Reference Tsoukra, Velakoulis, Wibawa, Malpas, Walterfang, Evans, Farrand, Kelso, Eratne and Loi2021). To our knowledge, no studies have examined whether a single CSF NfL test can predict the final diagnostic category in a clinical cohort with longitudinal diagnostic information, with the potential to reduce misdiagnosis for this diagnostically challenging cohort.
This study aimed to determine whether CSF NfL at baseline assessment in a clinical setting could predict the final diagnostic category (ND/MCI/other neurological disorders vs. PSY) using a pre-specified cut-off. We hypothesised that CSF NfL would accurately predict the final diagnostic category. More specifically, a NfL concentration above the pre-specified cut-off would predict a final diagnosis of ND/MCI/other, and NfL below the cut-off would predict a final PSY diagnosis. In instances where there was a diagnostic change (i.e. initial PSY changed to ND/MCI/other, and initial ND/MCI/other changed to PSY), we hypothesised that baseline CSF NfL would have correctly predicted the final diagnostic category. The secondary aim was to identify situations where clinicians should be more cautious in interpreting NfL.
Methods
Study setting
This study extends on our previous work, as part of The Markers in Neuropsychiatric Disorders Study (https://themindstudy.org), incorporating new patients and longitudinal data (Eratne et al., Reference Eratne, Loi, Walia, Farrand, Li, Varghese, Walterfang, Evans, Mocellin, Dhiman, Gupta, Malpas, Collins, Masters and Velakoulis2020; Eratne, Loi, et al., Reference Eratne, Loi, Li, Stehmann, Malpas, Santillo, Janelidze, Cadwallader, Walia, Ney, Lewis, Senesi, Fowler, McGlade, Varghese, Ravanfar, Kelso, Farrand, Keem, Kang, Goh, Dhiman, Gupta, Watson, Yassi, Kaylor‐Hughes, Kanaan, Perucca, Dobson, Vivash, Ali, O'Brien, Hansson, Zetterberg, Blennow, Walterfang, Masters, Berkovic, Collins and Velakoulis2022).
We included 228 consecutive patients who had undergone a diagnostic lumbar puncture as part of their baseline assessment at Neuropsychiatry (previously known as Neuropsychiatry Unit; ‘Neuropsychiatry’ from now on), Royal Melbourne Hospital, between 2009–2021. The only exclusion criterion was that patients had insufficient remnant CSF available for NfL analysis. Neuropsychiatry is a state-wide service providing comprehensive diagnostic assessment for people with complex mental health issues, neuropsychiatric, neurocognitive, neurodegenerative, and neurological conditions. Ongoing management is offered for people with younger-onset dementia. Re-assessments occurred on a 6-18 monthly basis depending on clinical need.
As previously described (Eratne et al., Reference Eratne, Loi, Walia, Farrand, Li, Varghese, Walterfang, Evans, Mocellin, Dhiman, Gupta, Malpas, Collins, Masters and Velakoulis2020; Eratne, Loi, et al., Reference Eratne, Loi, Li, Stehmann, Malpas, Santillo, Janelidze, Cadwallader, Walia, Ney, Lewis, Senesi, Fowler, McGlade, Varghese, Ravanfar, Kelso, Farrand, Keem, Kang, Goh, Dhiman, Gupta, Watson, Yassi, Kaylor‐Hughes, Kanaan, Perucca, Dobson, Vivash, Ali, O'Brien, Hansson, Zetterberg, Blennow, Walterfang, Masters, Berkovic, Collins and Velakoulis2022; Loi et al., Reference Loi, Walterfang, Kelso, Bevilacqua, Mocellin and Velakoulis2022), patients were assessed in inpatient and/or outpatient settings by a multidisciplinary team (neuropsychiatry, neurology, neuropsychology, occupational therapy, speech pathology, social work, nursing, and physiotherapy) and received multimodal investigations (brain magnetic resonance imaging, single-photon emission computerised tomography, fluorodeoxyglucose-positron emission tomography and lumbar puncture). CSF was analysed for biochemistry profile, inflammatory markers, and Alzheimer’s proteins. Clinicians were blinded to NfL, as NfL was retrospectively measured after the diagnostic assessment from remnant CSF samples. Patients received consensus diagnosis, with the classification of ND being based on established diagnostic criteria (van Straaten et al., Reference van Straaten, Scheltens, Knol, van Buchem, van Dijk, Hofman, Karas, Kjartansson, de Leeuw, Prins, Schmidt, Visser, Weinstein and Barkhof2003; McKeith et al., 2017; Emre et al., Reference Emre, Aarsland, Brown, Burn, Duyckaerts, Mizuno, Broe, Cummings, Dickson, Gauthier, Goldman, Goetz, Korczyn, Lees, Levy, Litvan, McKeith, Olanow, Poewe, Quinn, Sampaio, Tolosa and Dubois2007; Rascovsky et al., Reference Rascovsky, Hodges, Knopman, Mendez, Kramer, Neuhaus, van Swieten, Seelaar, Dopper, Onyike, Hillis, Josephs, Boeve, Kertesz, Seeley, Rankin, Johnson, Gorno-Tempini, Rosen, Prioleau-Latham, Lee, Kipps, Lillo, Piguet, Rohrer, Rossor, Warren, Fox, Galasko, Salmon, Black, Mesulam, Weintraub, Dickerson, Diehl-Schmid, Pasquier, Deramecourt, Lebert, Pijnenburg, Chow, Manes, Grafman, Cappa, Freedman, Grossman and Miller2011; Armstrong et al., Reference Armstrong, Litvan, Lang, Bak, Bhatia, Borroni, Boxer, Dickson, Grossman, Hallett, Josephs, Kertesz, Lee, Miller, Reich, Riley, Tolosa, Troster, Vidailhet and Weiner2013; Jack et al., Reference Jack, Bennett, Blennow, Carrillo, Dunn, Haeberlein, Holtzman, Jagust, Jessen, Karlawish, Liu, Molinuevo, Montine, Phelps, Rankin, Rowe, Scheltens, Siemers, Snyder, Sperling, Masliah, Ryan and Silverberg2018; van Straaten et al., Reference van Straaten, Scheltens, Knol, van Buchem, van Dijk, Hofman, Karas, Kjartansson, de Leeuw, Prins, Schmidt, Visser, Weinstein and Barkhof2003). Diagnosis of MCI was based on the Petersen criteria (Petersen, Reference Petersen2004). PSY diagnoses were defined by the Diagnostic and Statistical Manual of Mental Disorders 4th and 5th Editions (American Psychiatric Association and American Psychiatric Association, 2000, 2013).
Data collection
Three authors (Kang/Dobson/Keem) who were not involved with the baseline clinical assessments and blinded to NfL performed a file review using a codebook to ensure internal reliability, including discharge summaries and outpatient assessments. The data included demographic and clinical information and the initial diagnosis from the patient’s presentation to the service. For patients with follow-up within Neuropsychiatry, we used subsequent multidisciplinary assessment letters to collect their final diagnosis. For patients who were discharged from Neuropsychiatry, we contacted the primary doctor still involved in the patient’s care (private neurologist/psychiatrist, other specialty clinic, family physician) and for the final diagnosis. Clinicians were blinded to CSF NfL when they provided their final diagnosis. A minimum of 12 months of follow-up information was available for all participants.
We grouped initial diagnoses into categories:
Neurodegenerative disorders (ND) included all dementias and other disorders associated with neurodegeneration (e.g. Parkinson’s disease; PD).
MCI/other – MCI, considered a pre-dementia entity (Petersen et al., Reference Petersen, Caracciolo, Brayne, Gauthier, Jelic and Fratiglioni2014), and other non-progressive neurological disorders (i.e. epilepsy and acquired brain injury; ABI).
Psychiatric disorders (PSY) included psychoses, mood disorders, anxiety disorders, post-traumatic stress disorder, alcohol/substance use disorder, functional neurological/cognitive disorders (cognitive symptoms not caused by medical, neurological, or neurodegenerative disease (Stone et al., Reference Stone, Pal, Blackburn, Reuber, Thekkumpurath, Carson, Tales, Jessen, Butler, Wilcock, Phillips and Bayer2015)), and psychiatric-mixed (multiple psychiatric diagnoses). FTD phenocopy, defined as a non-progressing illness that is aetiologically heterogenous, comprising people with a psychiatric disorder, stressors-related exacerbation of underlying personality, was included in this group (Eratne, Keem, et al., Reference Eratne, Keem, Lewis, Kang, Walterfang, Farrand, Loi, Kelso, Cadwallader, Berkovic, Li, Masters, Collins, Santillo and Velakoulis2022).
Diagnosis unclear – some patients were diagnostically unclear despite comprehensive assessment, with further interval assessment being scheduled.
We grouped the final diagnoses similarly. Of note, patients with ’stable MCI' as their final diagnosis were cases where their cognition and functioning were stable and not progressive (Clem et al., Reference Clem, Holliday, Pandya, Hynan, Lacritz and Woon2017), representing ∼50% that do not progress to dementia. Similarly, other neurological disorders in the MCI/other group were non-progressive including historical ABI and stable epilepsy. On the other hand, progressive neurological illnesses including Niemann–Pick Type C, multiple sclerosis, and CNS vasculitis were included in the ND group.
Included in this study were 201/498 (40.4%) patients from our recent study (Eratne, Loi, et al., Reference Eratne, Loi, Li, Stehmann, Malpas, Santillo, Janelidze, Cadwallader, Walia, Ney, Lewis, Senesi, Fowler, McGlade, Varghese, Ravanfar, Kelso, Farrand, Keem, Kang, Goh, Dhiman, Gupta, Watson, Yassi, Kaylor‐Hughes, Kanaan, Perucca, Dobson, Vivash, Ali, O'Brien, Hansson, Zetterberg, Blennow, Walterfang, Masters, Berkovic, Collins and Velakoulis2022). Those not included were from non-clinical settings with most having come from an external registry. Consistent with this study’s aim in focusing on the utility of NfL in a cohort with significant diagnostic change longitudinally, 24% of participants (48/201) had their diagnosis category change since the previous publication (Eratne, Loi, et al., Reference Eratne, Loi, Li, Stehmann, Malpas, Santillo, Janelidze, Cadwallader, Walia, Ney, Lewis, Senesi, Fowler, McGlade, Varghese, Ravanfar, Kelso, Farrand, Keem, Kang, Goh, Dhiman, Gupta, Watson, Yassi, Kaylor‐Hughes, Kanaan, Perucca, Dobson, Vivash, Ali, O'Brien, Hansson, Zetterberg, Blennow, Walterfang, Masters, Berkovic, Collins and Velakoulis2022).
Cerebrospinal fluid measurements
As previously described (Eratne, Loi, et al., Reference Eratne, Loi, Li, Stehmann, Malpas, Santillo, Janelidze, Cadwallader, Walia, Ney, Lewis, Senesi, Fowler, McGlade, Varghese, Ravanfar, Kelso, Farrand, Keem, Kang, Goh, Dhiman, Gupta, Watson, Yassi, Kaylor‐Hughes, Kanaan, Perucca, Dobson, Vivash, Ali, O'Brien, Hansson, Zetterberg, Blennow, Walterfang, Masters, Berkovic, Collins and Velakoulis2022), CSF was stored at –80°C, and NfL was measured using a commercial enzyme-linked immunosorbent assay (ELISA; NF-light; UmanDiagnostics, Sweden, distributed by abacus dX), according to the manufacturer’s protocols, at the National Dementia Diagnostic Laboratory, Melbourne. CSF was diluted 1:1, and reconstituted standards were added to the plate in duplicate, incubated, and washed. Samples displaying concentrations above the highest standard point were further diluted and re-assayed. Two internal controls of pooled CSF were included in each NfL plate. Mean intra- and inter-assay coefficient of variation for NfL was 6.2% and 11.3%, respectively (Eratne, Loi, et al., Reference Eratne, Loi, Li, Stehmann, Malpas, Santillo, Janelidze, Cadwallader, Walia, Ney, Lewis, Senesi, Fowler, McGlade, Varghese, Ravanfar, Kelso, Farrand, Keem, Kang, Goh, Dhiman, Gupta, Watson, Yassi, Kaylor‐Hughes, Kanaan, Perucca, Dobson, Vivash, Ali, O'Brien, Hansson, Zetterberg, Blennow, Walterfang, Masters, Berkovic, Collins and Velakoulis2022).
NfL concentration cut-off
Although various cut-offs for CSF NfL have been reported, generalisability is limited, as concentrations vary between labs (Gaetani et al., Reference Gaetani, Blennow, Calabresi, Filippo, Parnetti and Zetterberg2019; Dreger et al., Reference Dreger, Steinbach, Gaur, Metzner, Stubendorff, Witte and Grosskreutz2021). We used a pre-specified cut-off from our previous study (582pg/mL; PPV = 95%; NPV = 78%) (Eratne, Loi, et al., Reference Eratne, Loi, Li, Stehmann, Malpas, Santillo, Janelidze, Cadwallader, Walia, Ney, Lewis, Senesi, Fowler, McGlade, Varghese, Ravanfar, Kelso, Farrand, Keem, Kang, Goh, Dhiman, Gupta, Watson, Yassi, Kaylor‐Hughes, Kanaan, Perucca, Dobson, Vivash, Ali, O'Brien, Hansson, Zetterberg, Blennow, Walterfang, Masters, Berkovic, Collins and Velakoulis2022). NfL was rated as accurate when (a) final diagnosis was ND/MCI/other and NfL > 582 pg/mL, or (b) final diagnosis was PSY and NfL < 582 pg/mL.
Ethics
This study was approved by Human Research Ethics Committees at Melbourne Health (2016.038, 2017.090, 2018.371, 2020.142), University of Melbourne (1341074), and Florey Institute of Neurosciences and Mental Health (1648441.1). Written informed consent was obtained from patients (or family members, where patients lacked capacity to provide consent). Ethics approval was obtained for retrospective biomarker analysis of samples collected prior 2016 when this project commenced. The authors assert that all procedures contributing to this work comply with the ethical standards of the relevant national and institutional committees on human experimentation and with the Helsinki Declaration of 1975, as revised in 2008.
Statistical analysis
Statistical analyses were performed using Jamovi and R version 4.2.1 (jamovi (Version 1.6), 2021; Wickham, Reference Wickham2016; Patil, Reference Patil2021; R Core Team, 2021; Xu et al., Reference Xu, Chen, Feng, Zhan, Zhou and Yu2021). As a result of the smaller sample in the diagnostically unstable group, Mann–Whitney U-tests were performed to test differences in numerical variables between diagnostically stable and unstable groups. Pearson’s chi-square tests of independence were performed to compare dichotomous variables. A two-sided p-value < 0.05 was considered statistically significant. General linear models (GLMs) were estimated to compare the differences in NfL between the diagnostic categories using age at CSF sampling as a covariate.
For all key model parameters (i.e. from GLM and receiver operator character (ROC) analyses), we computed 95% confidence intervals (CIs) to perform statistical inference. Findings were considered statistically significant if the CIs did not capture the null hypothesis value, which was 0.5 for ROC analyses (area under the curve) and 0 for GLM parameters. In order to ensure robust results, we used non-parametric bootstrapping to compute bias-corrected and accelerated (BCa) confidence intervals over 1000 samples. This technique ensures robust statistical inference even when distributional assumptions are not satisfied
We also performed sub-analyses of ND vs PSY, as the MCI/other group was small and heterogenous, and our previous finding (Eratne, Loi, et al., Reference Eratne, Loi, Li, Stehmann, Malpas, Santillo, Janelidze, Cadwallader, Walia, Ney, Lewis, Senesi, Fowler, McGlade, Varghese, Ravanfar, Kelso, Farrand, Keem, Kang, Goh, Dhiman, Gupta, Watson, Yassi, Kaylor‐Hughes, Kanaan, Perucca, Dobson, Vivash, Ali, O'Brien, Hansson, Zetterberg, Blennow, Walterfang, Masters, Berkovic, Collins and Velakoulis2022) that patients with MCI had an intermediary NfL concentration (n = 5739 pg/mL, 95%CI[478, 1001]) compared to ND (1528 pg/mL, 95%CI[1168, 1888]) and PSY (435 pg/mL, 95%CI[394, 476]).
We also performed sensitivity analyses to investigate alternative modelling strategies to compare mean differences, where indicated. These included log transformation of NfL values to induce normality, generalised linear models with non-Gaussian response families, and GLM estimation using heteroscedasticity consistent robust estimators, provided in the supplementary file. These methods provided comparable results, so the more familiar statistical methods are reported.
Using the updated final diagnoses, we performed post hoc analyses with this study’s participants to calculate specific cut-offs for participants older and younger than 60 years using ROC curves. Area under the ROC curve, sensitivity, and specificity of NfL in distinguishing ND/MCI/other from PSY were computed. Optimal cut-off was determined using Youden’s J statistic. We compared the performances of the age-specific and general pre-determined cut-offs using McNemar test.
We also repeated the same analysis for all patients, regardless of age, to observe any change in the optimal cut-off since the study given some patients’ diagnoses had been updated (Eratne, Loi, et al., Reference Eratne, Loi, Li, Stehmann, Malpas, Santillo, Janelidze, Cadwallader, Walia, Ney, Lewis, Senesi, Fowler, McGlade, Varghese, Ravanfar, Kelso, Farrand, Keem, Kang, Goh, Dhiman, Gupta, Watson, Yassi, Kaylor‐Hughes, Kanaan, Perucca, Dobson, Vivash, Ali, O'Brien, Hansson, Zetterberg, Blennow, Walterfang, Masters, Berkovic, Collins and Velakoulis2022).
Results
Study cohort (Table 1)
Of 228 eligible patients, 13 were lost to follow-up and three died within one year following their baseline assessment, further described in our supplementary file. The remaining 212 patients had a mean age of 55.4 years (SD 11.6), 41% (87/212) were female, and the mean follow-up period was 34.2 months (SD 24.1, range 12–148), which was similar among the final diagnostic groups. Participants with a final diagnosis of PSY were younger than the ND and MCI/other groups (mean difference of 8.2 and 8.8 years, respectively).
Table 1. Participant demographics, final diagnostic category, and NfL accuracy
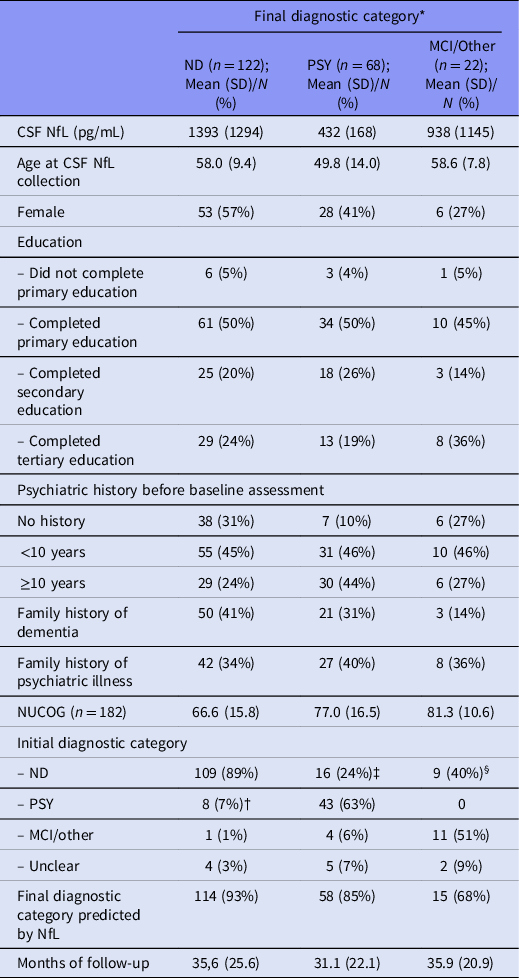
MCI, mild cognitive impairment; other, non-progressive neurological disorder; ND, neurodegenerative disorder; NUCOG, Neuropsychiatry Unit Cognitive Assessment Tool (Walterfang et al., Reference Walterfang, Siu and Velakoulis2006); PSY, primary psychiatric disorder.
* 53% were followed up externally. The proportion of patients whose diagnostic category changed was similar between those followed up internally/by Neuropsychiatry (36%), family physicians (30%), and specialists (24%).
† Specific diagnoses for the final ND group that were initially PSY; psychosis (n = 3), major depressive disorder (n = 3), bipolar affective disorder (n = 1), anxiety disorder (n = 1).
‡ For the final PSY group that were initially ND; bvFTD (n = 6), AD (n = 3), dementia not otherwise specified (n = 2), corticobasal syndrome (n = 1), primary progressive aphasia (n = 1), Parkinson’s disease-dementia (n = 1), vascular dementia (n = 1), Wilson’s disease (n = 1).
§ For the final MCI group that were initially ND; bvFTD (n = 8), AD (n = 1).
Adjusting for age, baseline CSF NfL concentrations were higher in patients with a final diagnosis of ND compared to PSY (GLM, mean difference = 929 pg/mL, 95%CI:[598, 1259], p < 0.001). Concentrations in the MCI/other group were higher than PSY (mean difference = 449 pg/mL, CI:[156, 743], p = 0.045). There was no significant difference between ND and MCI/other (mean difference = 428 pg/mL, CI:[−157.8, 1014], p = 0.091). These are presented in Fig. 1.

Fig. 1. Boxplot of NfL levels in diagnostic groups.
ND group
Fifty-eight per cent (122/212) of patients had a final diagnosis of ND, including AD (n = 51), frontotemporal dementia (FTD = 24, bvFTD = 16), dementia not otherwise specified (n = 13), corticobasal syndrome (CBS = 7), vascular dementia (n = 6), DLB (n = 4), mixed dementia (n = 3), PD (n = 3), and others including Niemann–Pick Type C, Huntington’s disease, multiple sclerosis, and CNS vasculitis. Four patients initially deemed as being diagnostically unclear at baseline assessment eventually received a ND diagnosis (AD, CBS, DLB, and mixed dementia).
MCI and other neurological illnesses
Ten per cent (22/212) of patients had a final diagnosis of MCI/other. There were 18 with MCI, two with epilepsy, one with ABI, and one whose neurocognitive symptoms completely resolved without any comorbid PSY.
PSY group
Thirty-two per cent (68/212) participants had a final PSY, including psychosis (n = 23), bipolar disorder (n = 6), major depression (n = 9), functional neurological disorder (n = 6), obsessive-compulsive disorder (n = 1), post-traumatic stress disorder (n = 1), and mixed psychiatric disorders (n = 18). Of five patients deemed diagnostically unclear at baseline assessment, all received a final diagnosis of PSY, which were depression (n = 2), FTD phenocopy (n = 1), and psychiatric-mixed (n = 2).
Diagnostic change from initial diagnosis at baseline assessment to final diagnosis
A visual representation of the participants’ diagnostic stability is shown in Fig. 2. There was a change in diagnostic category from initial to final diagnosis for 23% (49/212) patients. bvFTD was the most frequently changed diagnosis (14/49, 29%). Of note, 48 patients from our previous publication (Eratne, Loi, et al., Reference Eratne, Loi, Li, Stehmann, Malpas, Santillo, Janelidze, Cadwallader, Walia, Ney, Lewis, Senesi, Fowler, McGlade, Varghese, Ravanfar, Kelso, Farrand, Keem, Kang, Goh, Dhiman, Gupta, Watson, Yassi, Kaylor‐Hughes, Kanaan, Perucca, Dobson, Vivash, Ali, O'Brien, Hansson, Zetterberg, Blennow, Walterfang, Masters, Berkovic, Collins and Velakoulis2022) had their diagnosis category changed and updated with the incorporation of the new longitudinal data of this study.
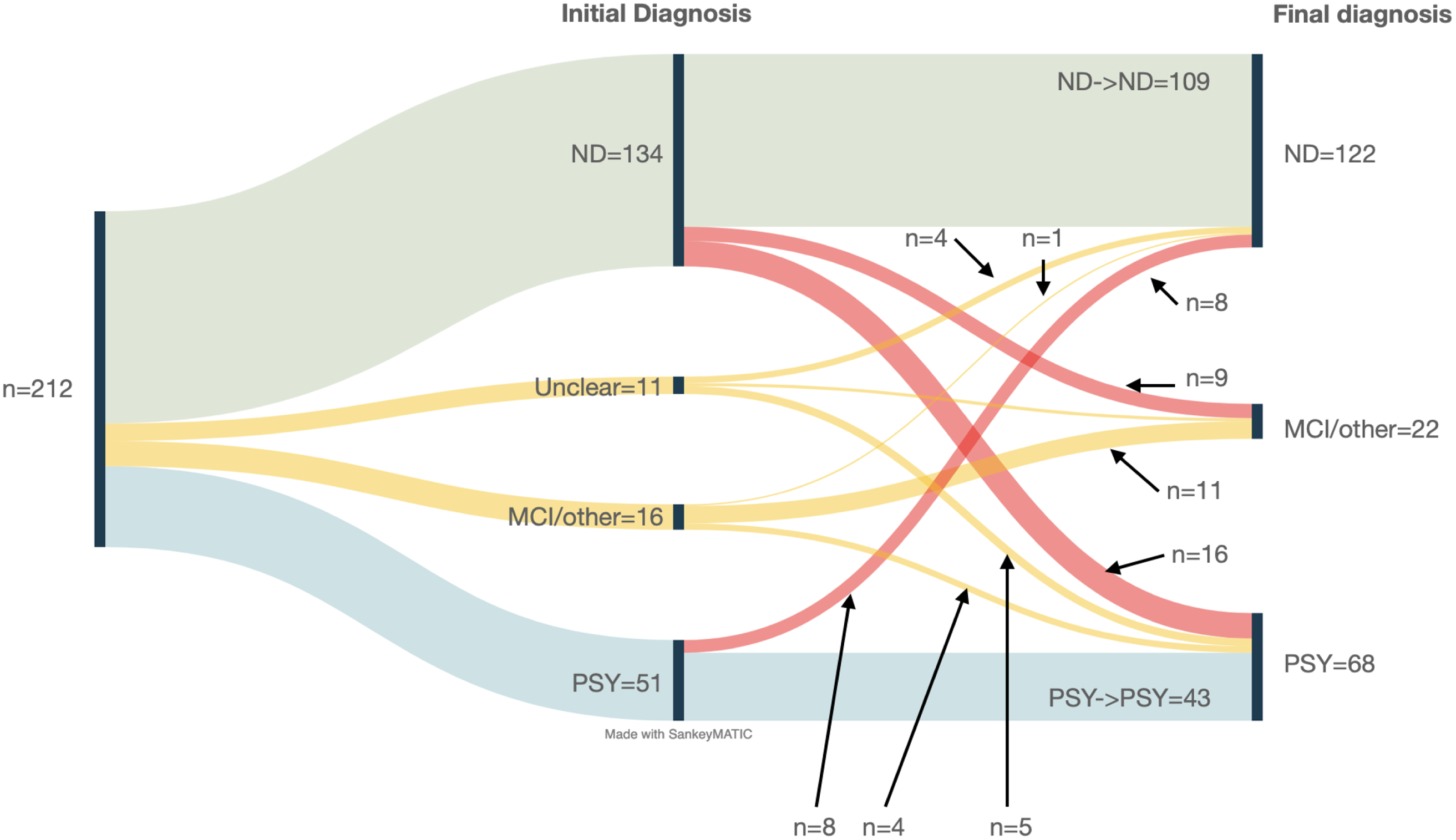
Fig. 2. Sankey diagram of diagnostic journey.
Twenty-four patients (11%, 24/212) patients had changed between ND and PSY as shown in Table 2. Eight patients (8%, 8/122) who were given a final diagnosis of ND were initially diagnosed with PSY at their baseline diagnosis before it was revised (PSY→ND). Sixteen patients (24%, 16/68) were initially diagnosed with ND before being revised to a final diagnosis of PSY (ND-PSY). Half (9/18) of the MCI patients initially had baseline diagnoses of ND. Patients who changed diagnostic categories (ND→PSY or PSY→ND) were more likely to have a long psychiatric history (>10 years, n = 14/22, x 2 = 10.6, p = 0.005). None of the other clinical variables were statistically different.
Table 2. Comparison of those with diagnostic category stability vs change
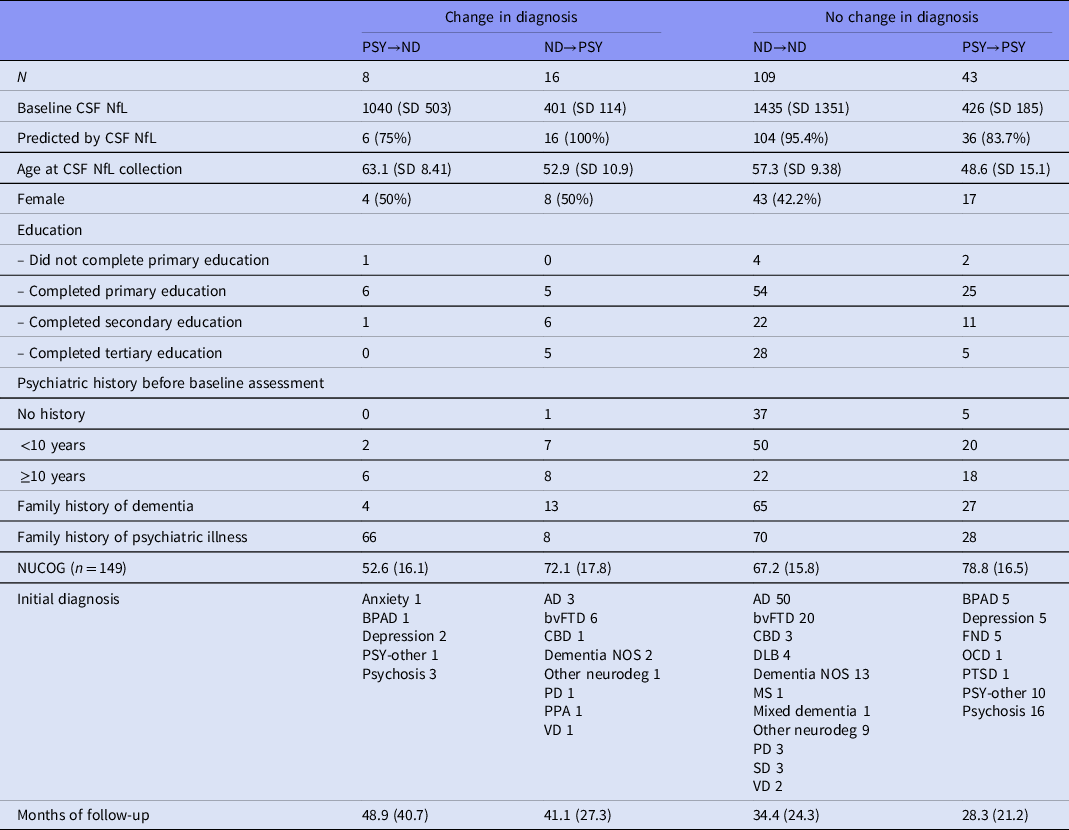
AD, Alzheimer’s disease; BPAD, bipolar affective disorder; bvFTD, behaviour variant frontotemporal dementia; CBD, corticobasal degeneration; DLB, dementia with Lewy bodies; Dementia NOS, Dementia not otherwise specified; FND, functional neurological disorder; MS, multiple sclerosis; NUCOG, Neuropsychiatry Unit Cognitive Assessment Tool (Walterfang et al., 2006); OCD, obsessive-compulsive disorder; PD, Parkinson’s disease; PPA, primary progressive aphasia; PSY-other, other primary psychiatric disorder; PTSD, post-traumatic stress disorder; SD, semantic dementia; VD, vascular dementia.
A table detailing description of the patients with diagnostic category change can be found in the supplementary file.
Accuracy of CSF NfL in predicting the final diagnosis category
Final diagnosis (Table 1)
NfL at baseline assessment predicted the final diagnostic category (ND/MCI/other vs. PSY) for 88% of patients (187/212). The accuracy was higher for predicting ND (93%) and PSY (85%), compared to MCI/other (68%). We found NfL to be similarly accurate (88%) for the subset of new patients since the previous study (Eratne, Loi, et al., Reference Eratne, Loi, Li, Stehmann, Malpas, Santillo, Janelidze, Cadwallader, Walia, Ney, Lewis, Senesi, Fowler, McGlade, Varghese, Ravanfar, Kelso, Farrand, Keem, Kang, Goh, Dhiman, Gupta, Watson, Yassi, Kaylor‐Hughes, Kanaan, Perucca, Dobson, Vivash, Ali, O'Brien, Hansson, Zetterberg, Blennow, Walterfang, Masters, Berkovic, Collins and Velakoulis2022).
NfL improved the accuracy for all diagnostic categories (ND, MCI/other, and PSY) compared to initial diagnosis from the baseline assessment (Fig. 3).

Fig. 3. Accuracy of baseline CSF NfL in predicting final diagnosis versus initial clinical assessment.
For the 24 patients whose diagnostic category changed between ND and PSY, NfL accurately predicted the final diagnostic category for 92% (22/24). The two patients where the baseline NfL did not predict the final diagnosis were both PSY→ND, and, notably, their follow-up duration between their initial and final diagnosis was 90 and 100 months, respectively, compared to the other 22 patients (mean = 38.5 months, SD 27.6).
Of the nine who were initially diagnostically unclear at their baseline assessment and then had a final diagnosis of PSY or ND diagnosis, 78% (7/9) were predicted by their baseline NfL. Four of the five (80%) patients initially diagnosed with MCI before they were given a final diagnosis of PSY/ND were predicted by NfL.
When comparing NfL to only the initial diagnosis from the baseline assessment (i.e. before longitudinal follow-up and the final diagnosis after changes/refinement), its apparent accuracy was only 79% (159/201).
Age-specific & overall cut-offs (Fig. 4)
The optimal cut-off for distinguishing ND/MCI/other from PSY for <60 years was the same as our pre-determined cut-off (582 pg/mL; sensitivity = 86%, specificity = 92%, Youden’s index = 0.78). The optimal cut-off for ≥ 60 years was 716 pg/mL.
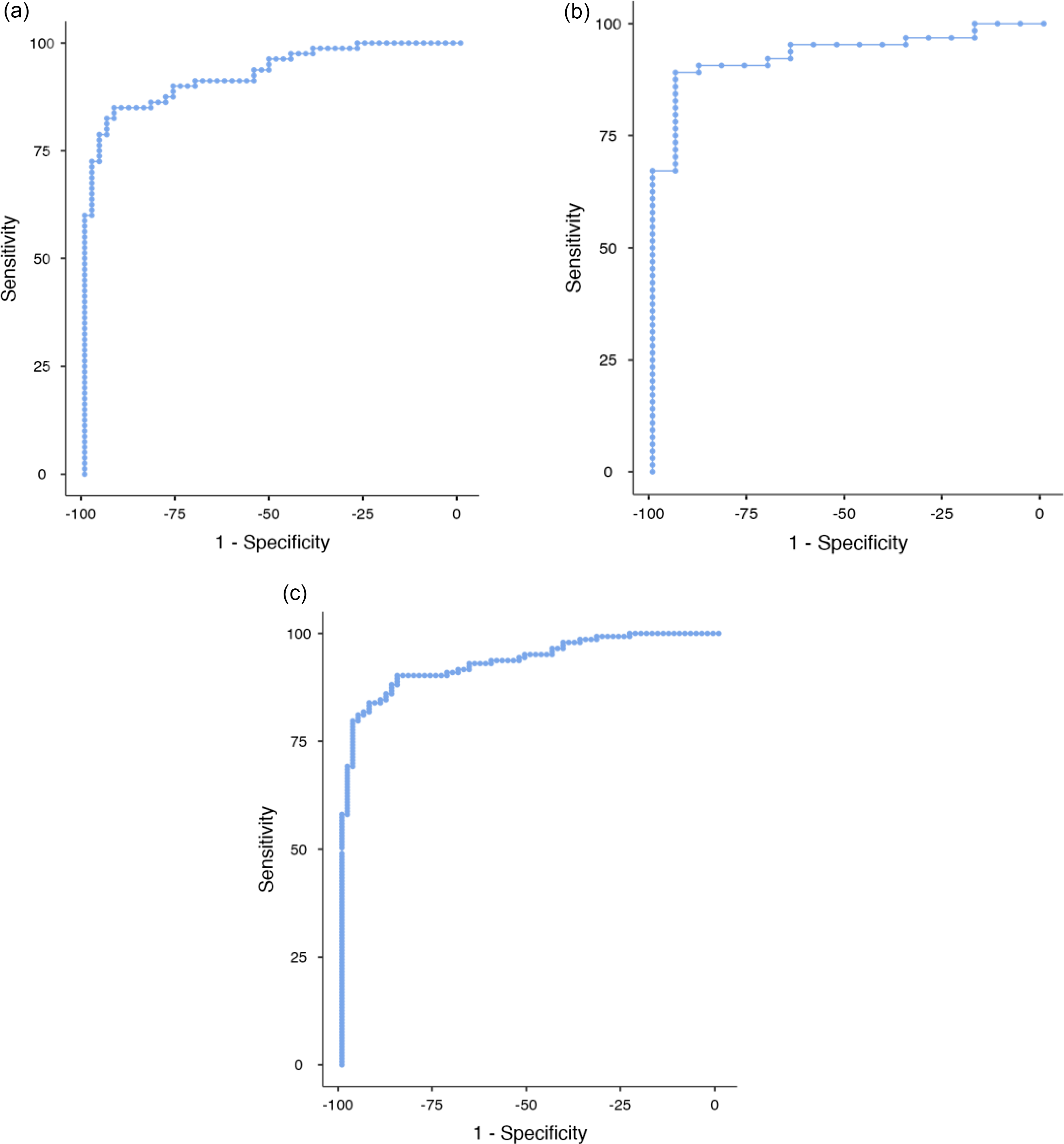
Fig. 4 ROC curves for patients <60 years of age (A), 60+ years of age (B) and all ages (C).
Using this, 716 pg/mL cut-off for the ≥60 cohort improved accuracy of baseline CSF NfL in predicting final ND/MCI/other vs PSY diagnoses from 90% (64/71) to 93% (66/71) with no significant difference (p = 0.157). The number of people ≥60 years was small (n = 81, ND = 54, PSY = 17, MCI/other = 17); therefore, results must be interpreted with caution.
Despite the participants’ updated diagnoses, the optimal cut-off for all participants regardless of age was the same as previous (582 pg/mL; sensitivity = 90%, specificity = 85%, Youden’s index = 0.76).
Discussion
We found that a single CSF NfL test could have accurately predicted a final diagnosis of a neurodegenerative disorder or MCI/other neurological disorder, versus a primary psychiatric, for 88% of cases in a real-world, inclusive, clinical cohort assessed for cognitive and neuropsychiatric presentations. Furthermore, we found that CSF NfL, in retrospect, could have aided clinicians in avoiding a delayed diagnosis for 11% of patients compared to relying on baseline clinical diagnostic assessment alone. Our finding of a change in diagnostic category longitudinally in 23% of patients based on gold standard assessments in a tertiary cognitive neuropsychiatry service is consistent with the literature on the challenges faced in clinical practice (Woolley et al., Reference Woolley, Khan, Murthy, Miller and Rankin2011; Tsoukra et al., Reference Tsoukra, Velakoulis, Wibawa, Malpas, Walterfang, Evans, Farrand, Kelso, Eratne and Loi2021). The potential for NfL to improve diagnostic accuracy supports the translation of NfL from research labs into routine patient care. This is in line with recent recommendations from international groups to use CSF biomarkers such as NfL to assist in challenging clinical distinctions such as differentiating AD and bvFTD from psychiatric and non-neurodegenerative causes (Dubois et al., Reference Dubois, Feldman, Jacova, Hampel, Molinuevo, Blennow, DeKosky, Gauthier, Selkoe, Bateman, Cappa, Crutch, Engelborghs, Frisoni, Fox, Galasko, Habert, Jicha, Nordberg, Pasquier, Rabinovici, Robert, Rowe, Salloway, Sarazin, Epelbaum, de Souza, Vellas, Visser, Schneider, Stern, Scheltens and Cummings2014; Lewczuk et al., Reference Lewczuk, Riederer, O’Bryant, Verbeek, Dubois, Visser, Jellinger, Engelborghs, Ramirez, Parnetti, Jack, Teunissen, Hampel, Lleó, Jessen, Glodzik, de Leon, Fagan, Molinuevo, Jansen, Winblad, Shaw, Andreasson, Otto, Mollenhauer, Wiltfang, Turner, Zerr, Handels, Thompson, Johansson, Ermann, Trojanowski, Karaca, Wagner, Oeckl, van Waalwijk van Doorn, Bjerke, Kapogiannis, Kuiperij, Farotti, Li, Gordon, Epelbaum, Vos, Klijn, Van Nostrand, Minguillon, Schmitz, Gallo, Lopez Mato, Thibaut, Lista, Alcolea, Zetterberg, Blennow and Kornhuber2018; Ducharme et al., Reference Ducharme, Dols, Laforce, Devenney, Kumfor, van den Stock, Dallaire-Théroux, Seelaar, Gossink, Vijverberg, Huey, Vandenbulcke, Masellis, Trieu, Onyike, Caramelli, de Souza, Santillo, Waldö, Landin-Romero, Piguet, Kelso, Eratne, Velakoulis, Ikeda, Perry, Pressman, Boeve, Vandenberghe, Mendez, Azuar, Levy, Le Ber, Baez, Lerner, Ellajosyula, Pasquier, Galimberti, Scarpini, van Swieten, Hornberger, Rosen, Hodges, Diehl-Schmid and Pijnenburg2020).
Strengths of this study include the generalisable cohort, inclusion of all patients regardless of comorbidities, longitudinal follow-up, and diagnoses based on serial multimodal and multidisciplinary assessments, and blinded comprehensive review of files and follow-up. This study builds on previous work with new longitudinal diagnostic information (Eratne et al., Reference Eratne, Loi, Walia, Farrand, Li, Varghese, Walterfang, Evans, Mocellin, Dhiman, Gupta, Malpas, Collins, Masters and Velakoulis2020; Eratne, Loi, et al., Reference Loi, Walterfang, Kelso, Bevilacqua, Mocellin and Velakoulis2022), demonstrating that CSF NfL could assist clinicians in ruling in or out PSY being the cause of a patient’s neuropsychiatric presentation with diagnostic stability over time. To our knowledge, no other studies have examined the diagnostic utility of NfL to predict and reduce diagnostic delay in such an inclusive, real-world clinical cohort, with a focus on younger people where diagnostic delay are more common (Loi et al., Reference Loi, Goh, Mocellin, Malpas, Parker, Eratne, Farrand, Kelso, Evans, Walterfang and Velakoulis2020). In particular, we saw that bvFTD was the most frequently changed diagnosis, further supporting the utility of biomarkers in this challenging diagnosis (Ducharme et al., Reference Ducharme, Dols, Laforce, Devenney, Kumfor, van den Stock, Dallaire-Théroux, Seelaar, Gossink, Vijverberg, Huey, Vandenbulcke, Masellis, Trieu, Onyike, Caramelli, de Souza, Santillo, Waldö, Landin-Romero, Piguet, Kelso, Eratne, Velakoulis, Ikeda, Perry, Pressman, Boeve, Vandenberghe, Mendez, Azuar, Levy, Le Ber, Baez, Lerner, Ellajosyula, Pasquier, Galimberti, Scarpini, van Swieten, Hornberger, Rosen, Hodges, Diehl-Schmid and Pijnenburg2020).
We found that the accuracy of CSF NfL in predicting the diagnosis improved (88% final diagnosis with longitudinal follow-up vs 79% initial diagnosis from baseline assessment) with longitudinal diagnostic information (mean follow-up = 34 months, SD 24.1). This is consistent with clinical practice, where diagnoses are often revised over time. Therefore, it is possible that studies that relied only on diagnostic criteria without longitudinal information may have underestimated the accuracy of NfL compared to this study.
Our findings where baseline CSF NfL was not consistent with the final diagnosis highlight scenarios where clinicians should interpret NfL with caution. This was in the cases where patients had PD, cerebrovascular burden, and MCI. The small number of patients with PD had a low baseline NfL concentration despite PD being a progressive neurodegenerative disorder. This is in line with previous findings that suggest NfL, found primarily in myelin-rich axons, may not be affected in PD where there is no widespread axonal degeneration (Gaetani et al., Reference Gaetani, Blennow, Calabresi, Filippo, Parnetti and Zetterberg2019). Patients with a final diagnosis of PSY but with evidence of chronic small vessel disease on their neuroimaging may still have mildly elevated NfL. However, their NfL concentrations were within 20% of the cut-off and not as markedly increased as the ND group. This may be NfL detecting PSY associated with white matter hyperintensities, including ‘vascular depression’ (Culang-Reinlieb et al., Reference Culang-Reinlieb, Johnert, Brickman, Steffens, Garcon and Sneed2011; Wang et al., Reference Wang, Leonards, Sterzer and Ebinger2014). Future studies characterising the relationship between NfL in patients with PSY and chronic small vessel disease will help inform clinicians on how to interpret NfL in this context. NfL was higher in those with MCI compared to patients diagnosed with PSY, consistent with previous literature (Zetterberg et al., Reference Zetterberg, Skillbäck, Mattsson, Trojanowski, Portelius, Shaw, Weiner and Blennow2016; Bridel et al., Reference Bridel, van Wieringen, Zetterberg, Tijms, Teunissen, Andreasson, Axelsson, Bäckström, Bartos, Bjerke, Blennow, Boxer, Brundin, Burman, Christensen, Fialová, Forsgren, Frederiksen, Gisslén, Gray, Gunnarsson, Hall, Hansson, Herbert, Jakobsson, Jessen-Krut, Janelidze, Johannsson, Jonsson, Kappos, Khademi, Khalil, Kuhle, Landén, Leinonen, Logroscino, Lu, Lycke, Magdalinou, Malaspina, Mattsson, Meeter, Mehta, Modvig, Olsson, Paterson, Pérez-Santiago, Piehl, Pijnenburg, Pyykkö, Ragnarsson, Rojas, Romme Christensen, Sandberg, Scherling, Schott, Sellebjerg, Simone, Skillbäck, Stilund, Sundström, Svenningsson, Tortelli, Tortorella, Trentini, Troiano, Turner, van Swieten, Vågberg, Verbeek, Villar, Visser, Wallin, Weiss, Wikkelsø and Wild2019). As CSF NfL has been linked to the progression rate of ND (Dreger et al., Reference Dreger, Steinbach, Gaur, Metzner, Stubendorff, Witte and Grosskreutz2021), it may in turn indicate the prognosis of those with MCI and whether the condition will progress to ND, such as AD. Furthermore, there may be a role for serial NfL in these higher-risk individuals, as the trend of NfL may help indicate whether there is a neurodegenerative process that may be too slow for other biomarkers such as neuroimaging to be able to detect. Studies exploring the utility of serial NfL are underway.
Strengths of this study include the generalisable cohort, inclusion of all patients regardless of comorbidities, longitudinally confirmed diagnoses, and blinded data collection. While this cohort was inclusive in the sense that patients were not excluded based on their psychiatric comorbidities, our file reviews lacked consistent detailed ethnicity/race and socio-economic status required to report in this study. This is an important area to investigate in future studies.
While a strength of our study was using a pre-determined cut-off, this cut-off may need to be adjusted for other services due to variabilities between labs (Gaetani et al., Reference Gaetani, Blennow, Calabresi, Filippo, Parnetti and Zetterberg2019; Dreger et al., Reference Dreger, Steinbach, Gaur, Metzner, Stubendorff, Witte and Grosskreutz2021). Therefore, ongoing international work to develop reference ranges for research and clinical use, such as the Global Biomarker Standardization Consortium initiatives, is critical (Carrillo et al., Reference Carrillo, Blennow, Soares, Lewczuk, Mattsson, Oberoi, Umek, Vandijck, Salamone, Bittner, Shaw, Stephenson, Bain and Zetterberg2013). Furthermore, our findings support age-specific cut-offs to increase diagnostic accuracy. These age-specific cut-offs require validation in an external cohort to ensure that the classification accuracy is robust, especially given the difference in age between the ND and PSY cohorts. In addition, more recent studies examining blood NfL in multiple sclerosis used age-adjusted z-scores to mitigate the non-linear relationship between NfL and age (Benkert et al., Reference Benkert, Meier, Schaedelin, Manouchehrinia, Yaldizli, Maceski, Oechtering, Achtnichts, Conen, Derfuss, Lalive, Mueller, Müller, Naegelin, Oksenberg, Pot, Salmen, Willemse, Kockum, Blennow, Zetterberg, Gobbi, Kappos, Wiendl, Berger, Sormani, Granziera, Piehl, Leppert, Kuhle, Aeschbacher, Barakovic, Buser, Chan, Disanto, D’Souza, Du Pasquier, Findling, Galbusera, Hrusovsky, Khalil, Lorscheider, Mathias, Orleth, Radue, Rahmanzadeh, Sinnecker, Subramaniam, Vehoff, Wellmann, Wuerfel and Zecca2022), which was not possible for this study due to the lack of a normative dataset for CSF NfL, but could be considered for future studies.
Limitations of this study included those inherent to a retrospective design, including the reliance on historical diagnostic formulations. Furthermore, re-assessments were done based on clinical need; therefore, there was variability in the frequency and type of assessment between patients. This was mitigated by longitudinal follow-up data collection, and blinded, rigorous, and comprehensive reviews. As this study was completed at a single tertiary site, its generalisability is limited. This is especially the case for older cohorts, as the majority of our study cohort was less than 65 years old. As NfL levels have greater variability with increasing age (Simrén et al., Reference Simrén, Andreasson, Gobom, Suarez Calvet, Borroni, Gillberg, Nyberg, Ghidoni, Fernell, Johnson, Depypere, Hansson, Jonsdottir, Zetterberg and Blennow2022), its use in older patients may be challenging. Despite obtaining several years of follow-up with serial assessments for most patients, more insights may be yielded with longer follow-up and post-mortem pathological confirmation. For example, there may be some participants diagnosed with PSY, who may instead have an extremely slowly progressing ND. In these cases, we may have underestimated the number of ND. Conversely, long interval between initial and final diagnosis may result in the baseline NfL level being outdated. In addition, our approach in delineating PSY and ND does not account for the complex relationship between psychiatric and neurodegenerative disorders, with ongoing debate as to whether depression is a prodrome or risk factor for dementia (Singh-Manoux et al., Reference Singh-Manoux, Dugravot, Fournier, Abell, Ebmeier, Kivimäki and Sabia2017; Cantón-Habas et al., Reference Cantón-Habas, Rich-Ruiz, Romero-Saldaña and Carrera-González2020).
This study showed that CSF NfL can assist clinicians differentiating neurodegenerative disorders from psychiatric disorders. This could function not too dissimilar to C-reactive protein in infective/inflammatory conditions. An elevated NfL could help dismiss psychiatric differentials and potential misdiagnosis. Conversely, a low NfL could (especially if persistently low) reduce the risk of a neurodegenerative disorder misdiagnosis and increase confidence in a psychiatric diagnosis. A borderline NfL could prompt careful review of other clinical features and follow-up and potentially help with prognosticating in complex situations like MCI. While results from this study show potential diagnostic utility of CSF NfL, lumbar punctures are impractical for widespread use, including primary care settings. Blood NfL, which correlates well with CSF NfL (Wilke et al., Reference Wilke, Preische, Deuschle, Roeben, Apel, Barro, Maia, Maetzler, Kuhle and Synofzik2016; Khalil et al., Reference Khalil, Pirpamer, Hofer, Voortman, Barro, Leppert, Benkert, Ropele, Enzinger, Fazekas, Schmidt and Kuhle2018; Bridel et al., Reference Bridel, van Wieringen, Zetterberg, Tijms, Teunissen, Andreasson, Axelsson, Bäckström, Bartos, Bjerke, Blennow, Boxer, Brundin, Burman, Christensen, Fialová, Forsgren, Frederiksen, Gisslén, Gray, Gunnarsson, Hall, Hansson, Herbert, Jakobsson, Jessen-Krut, Janelidze, Johannsson, Jonsson, Kappos, Khademi, Khalil, Kuhle, Landén, Leinonen, Logroscino, Lu, Lycke, Magdalinou, Malaspina, Mattsson, Meeter, Mehta, Modvig, Olsson, Paterson, Pérez-Santiago, Piehl, Pijnenburg, Pyykkö, Ragnarsson, Rojas, Romme Christensen, Sandberg, Scherling, Schott, Sellebjerg, Simone, Skillbäck, Stilund, Sundström, Svenningsson, Tortelli, Tortorella, Trentini, Troiano, Turner, van Swieten, Vågberg, Verbeek, Villar, Visser, Wallin, Weiss, Wikkelsø and Wild2019), could be a more accessible first-tier test as part of a routine ‘dementia panel’. To test whether this is feasible, the Markers in Neuropsychiatric Disorders Study (MiND Study; https://themindstudy.org/) group is currently exploring blood NfL in people with neurological and psychiatric symptoms. This single test could reduce the diagnostic delay that many patients face, facilitating earlier precision care.
Supplementary material
The supplementary material for this article can be found at https://doi.org/10.1017/neu.2023.25.
Acknowledgements
The authors would like to thank all the staff, past and present, at Neuropsychiatry, The Royal Melbourne Hospital, and all the clinicians who provided follow-up clinical information. The authors would also like to thank all the patients and their families for their participation.
We are grateful for the funding that supported this work: the Trisno Family Research Grant in Old Age Psychiatry, three NorthWestern Mental Health Research Seed Grants, MACH MRFF, and NHMRC (1185180).
Matthew Kang is supported by the Research Training Program Scholarship from the Department of Psychiatry, University of Melbourne with contributions from the Australian Commonwealth Government.
Alexander F Santillo has been supported specifically for this project by The Fromma Foundation, The Ellen and Henrik Sjöbring Foundation, and the Fredrik and Ingrid Thuring Foundation.
Henrik Zetterberg is a Wallenberg Scholar supported by grants from the Swedish Research Council (#2018-02532), the European Research Council (#681712 and #101053962), Swedish State Support for Clinical Research (#ALFGBG-71320), the Alzheimer Drug Discovery Foundation (ADDF), USA (#201809-2016862), the AD Strategic Fund and the Alzheimer’s Association (#ADSF-21-831376-C, #ADSF-21-831381-C and #ADSF-21-831377-C), the Bluefield Project, the Olav Thon Foundation, the Erling-Persson Family Foundation, Stiftelsen för Gamla Tjänarinnor, Hjärnfonden, Sweden (#FO2022-0270), the European Union’s Horizon 2020 research and innovation programme under the Marie Skłodowska-Curie grant agreement No 860197 (MIRIADE), the European Union Joint Programme – Neurodegenerative Disease Research (JPND2021-00694), and the UK Dementia Research Institute at UCL (UKDRI-1003).
Kaj Blennow is supported by the Swedish Research Council (#2017-00915), the Alzheimer Drug Discovery Foundation (ADDF), USA (#RDAPB-201809-2016615), the Swedish Alzheimer Foundation (#AF-930351, #AF-939721 and #AF-968270), Hjärnfonden, Sweden (#FO2017-0243 and #ALZ2022-0006), the Swedish state under the agreement between the Swedish government and the County Councils, the ALF agreement (#ALFGBG-715986 and #ALFGBG-965240), the European Union Joint Program for Neurodegenerative Disorders (JPND2019-466-236), the National Institute of Health, USA, (grant #1R01AG068398-01), and the Alzheimer’s Association 2021 Zenith Award (ZEN-21-848495).
AHE reports honoraria for presentations from Merck, Allergan, Ipsen, Teva, UCB, Abbott, AbbVie, Pfizer, STADA. Participation in scientific advisory board meetings with Allergan, AbbVie, Ipsen, Pfizer, and STADA. He holds shares in GKC and CSL.
This manuscript has been published in a preprint server https://doi.org/10.1101/2022.09.08.22279663.
Collaborators of The MiND Study Group: Ms Claire Cadwallader, Neuropsychiatry, Royal Melbourne Hospital; Melbourne; The Turner Institute for Brain and Mental Health, Monash University, Victoria 3800; A/Prof Rosie Watson, Population Health and Immunity Division, The Walter and Eliza Hall Institute of Medical Research, Parkville, Australia; A/Prof Nawaf Yassi, Departments of Medicine and Neurology, Melbourne Brain Centre at the Royal Melbourne Hospital, University of Melbourne, Parkville, Australia; Population Health and Immunity Division, The Walter and Eliza Hall Institute of Medical Research, Parkville, Australia; Dr Cath Kaylor-Hughes, Integrated Mental Health Team; Dept of General Practice; University of Melbourne, 780 Elizabeth St, Melbourne, 3010; Prof. Richard Kanaan, Dept of Psychiatry, University of Melbourne, Austin Health, Heidelberg, VIC 3084; A/Prof Piero Perucca, Department of Medicine, Austin Health, The University of Melbourne; Comprehensive Epilepsy Program, Austin Health; Department of Neuroscience, Central Clinical School, Monash University; Department of Neurology, The Royal Melbourne Hospital; Department of Neurology, Alfred Health, Melbourne, VIC, Australia; Dr Lucy Vivash, The Department of Neuroscience, The Central Clinical School, The Alfred Hospital, Monash University; and The Departments of Medicine and Neurology, The Royal Melbourne Hospital, The University of Melbourne, Victoria, Australia; Ms Rashida Ali, Alfred Health; Monash University; Prof. Terence J. O’Brien, The Department of Neuroscience, The Central Clinical School, The Alfred Hospital, Monash University; and The Departments of Medicine and Neurology, The Royal Melbourne Hospital, The University of Melbourne, Victoria, Australia; Professor Anna King, The Wicking Dementia Centre, Tasmania; Professor Patrick Kwan, Alfred Hospital; Monash University, Melbourne; Professor Jane Gunn, Department of General Practice, The University of Melbourne; Dr Ilias Goranitis, Health Economics Unit, Centre for Health Policy, Melbourne School of Population and Global Health, The University of Melbourne; Dr Tianxin Pan, Health Economics Unit, Centre for Health Policy, Melbourne School of Population and Global Health, The University of Melbourne; Prof Christos Pantelis, Melbourne Neuropsychiatry Centre & Department of Psychiatry, University of Melbourne & Melbourne Health; Mid West Area Mental Health Service, Melbourne Health.
Author contributions
Matthew Kang – Study design and coordination, data collection, data analysis and interpretation, literature search, statistical analysis, writing manuscript.
Dhamidhu Eratne – Study design and coordination, data collection, data analysis and interpretation, literature search, statistical analysis, writing manuscript.
Hannah Dobson – Study design and coordination, data collection, and manuscript revision.
Charles B Malpas – Statistical analyses, manuscript writing, and revision.
Michael Keem – Study design and coordination, data collection, data analysis, and manuscript revision.
Courtney Lewis – Data collection, data analysis, and manuscript revision.
Jasleen Grewal – Data collection, data analysis, and manuscript revision.
Vivian Tsoukra – Data collection, data analysis, and manuscript revision.
Christa Dang – Data analysis & manuscript revision.
Ramon Mocellin – Data collection manuscript revision.
Thomas Kalincik – Literature review, data interpretation, and manuscript revision.
Alexander F Santillo – Literature review, data interpretation, and manuscript revision.
Henrik Zetterberg – Study design, literature review, data collection, and manuscript revision.
Kaj Blennow – Study design, literature review, data analysis, and manuscript revision.
Christiane Stehmann – Literature review, data analysis, and manuscript revision.
Shiji Varghese – Literature review, data analysis, and manuscript revision.
Qiao-Xin Li – Data analysis and manuscript revision.
Colin L Masters – Study design, data interpretation, literature search, and reviewing manuscript.
Steven Collins – Study design, data interpretation, literature search, and reviewing manuscript.
Samuel F Berkovic – Study design, data interpretation, literature search, and reviewing manuscript.
Andrew Evans – Data collection, interpretation, and manuscript revision.
Wendy Kelso – Data collection, interpretation, and manuscript revision.
Sarah Farrand – Data collection, interpretation, and manuscript revision.
Samantha M Loi – Study design and coordination, data collection and interpretation, literature review, and revising manuscript.
Mark Walterfang – Study design and coordination, data collection and interpretation, literature review, and revising manuscript.
Dennis Velakoulis – Study oversight including design conceptualisation and coordination, data collection and interpretation, writing and revising manuscript.
Financial support and Conflict of interest
No declarations for:
-
Matthew Kang
-
Dhamidhu Eratne
-
Christa Dang
-
Jasleen Grewal
-
Michael Keem
-
Alexander Santillo
-
Vivian Tsoukra
-
Hannah Dobson
-
Wendy Kelso
-
Ramon Mocellin
-
Christiane Stehmann
-
Shiji Varghese
-
Qiao-Xin Li
Steven Collins has acted as a paid consultant to Biogen Australia.
Henrik Zetterberg: HZ has served at scientific advisory boards and/or as a consultant for Abbvie, Acumen, Alector, ALZPath, Annexon, Apellis, Artery Therapeutics, AZTherapies, CogRx, Denali, Eisai, Nervgen, Novo Nordisk, Passage Bio, Pinteon Therapeutics, Red Abbey Labs, reMYND, Roche, Samumed, Siemens Healthineers, Triplet Therapeutics, and Wave, has given lectures in symposia sponsored by Cellectricon, Fujirebio, Alzecure, Biogen, and Roche, and is a co-founder of Brain Biomarker Solutions in Gothenburg AB (BBS), which is a part of the GU Ventures Incubator Program (outside submitted work).
Charles Malpas has received conference travel support from Merck, Novartis, and Biogen. He has received research support from the National Health and Medical Research Council, Multiple Sclerosis Research Australia, The University of Melbourne, The Royal Melbourne Hospital Neuroscience Foundation, and Dementia Australia.
Kaj Blennow has served as a consultant, at advisory boards, or at data monitoring committees for Abcam, Axon, BioArctic, Biogen, JOMDD/Shimadzu. Julius Clinical, Lilly, MagQu, Novartis, Ono Pharma, Pharmatrophix, Prothena, Roche Diagnostics, and Siemens Healthineers, and is a co-founder of Brain Biomarker Solutions in Gothenburg AB (BBS), which is a part of the GU Ventures Incubator Program, outside the work presented in this paper.
Mark Walterfang has served as a consultant, at advisory boards, or on data monitoring committees for Actelion, Vtesse, Sucampo, Mallinckrodt, and Biomarin Pharmaceuticals; has received investigator grants from Pfizer, Lilly, Bristol Meyers Squibb, Actelion, Vtesse, and Mallinckrodt pharmaceuticals; the National Health and Medical Research Council, the Royal Melbourne Hospital, the Ara Parseghian Medical Research Foundation, the Bethlehem Griffiths Foundation, and the CHDI Initiative. He has received travel support from Lilly, Pfizer, Actelion, Orphan, Biomarin, Vtesse and Sucampo Pharmaceuticals.
Tomas Kalincik served on scientific advisory boards for MS International Federation and World Health Organisation, BMS, Roche, Janssen, Sanofi Genzyme, Novartis, Merck and Biogen, steering committee for Brain Atrophy Initiative by Sanofi Genzyme, received conference travel support and/or speaker honoraria from WebMD Global, Eisai, Novartis, Biogen, Roche, Sanofi Genzyme, Teva, BioCSL and Merck and received research or educational event support from Biogen, Novartis, Genzyme, Roche, Celgene, and Merck.
Sarah Farrand has received honoraria from Abbvie, Abbott, and a fellowship from Medtronic.