Book contents
- The Cambridge Handbook of Intelligence and Cognitive Neuroscience
- Reviews
- The Cambridge Handbook of Intelligence and Cognitive Neuroscience
- Copyright page
- Dedication
- Contents
- Figures
- Tables
- Contributors
- Preface
- Part I Fundamental Issues
- Part II Theories, Models, and Hypotheses
- Part III Neuroimaging Methods and Findings
- Part IV Predictive Modeling Approaches
- Part V Translating Research on the Neuroscience of Intelligence into Action
- Index
- References
Part I - Fundamental Issues
Published online by Cambridge University Press: 11 June 2021
- The Cambridge Handbook of Intelligence and Cognitive Neuroscience
- Reviews
- The Cambridge Handbook of Intelligence and Cognitive Neuroscience
- Copyright page
- Dedication
- Contents
- Figures
- Tables
- Contributors
- Preface
- Part I Fundamental Issues
- Part II Theories, Models, and Hypotheses
- Part III Neuroimaging Methods and Findings
- Part IV Predictive Modeling Approaches
- Part V Translating Research on the Neuroscience of Intelligence into Action
- Index
- References
Summary
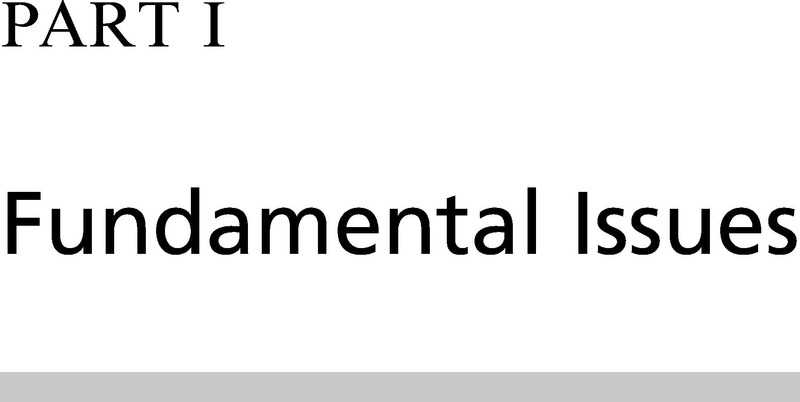
- Type
- Chapter
- Information
- Publisher: Cambridge University PressPrint publication year: 2021