Introduction
Bilingual speakers and writers sometimes switch from one language to another within a single sentence, known as intrasentential code-switching (Poplack, Reference Poplack1980). Code-switching is relatively common (Beatty-Martínez & Dussias, Reference Beatty-Martínez and Dussias2017; Guzzardo Tamargo et al., Reference Guzzardo Tamargo, Valdés Kroff and Dussias2016; Poplack, Reference Poplack1980), which may be surprising given that it can induce significant processing costs (Beatty-Martínez et al., Reference Beatty-Martínez, Valdés Kroff and Dussias2018; Valdés Kroff et al., 2018; Van Hell et al., Reference Van Hell, Fernandez, Kootstra, Litcofsky and Ting2018). For example, during comprehension, bilinguals take longer to read code-switched regions of a sentence (e.g., Bultena et al., Reference Bultena, Dijkstra and Van Hell2015) and have longer first-fixation durations on code-switched words as compared to single-language equivalents, suggesting difficulty in the initial processing of linguistic input that switches languages mid-utterance (e.g., Altarriba et al., Reference Altarriba, Kroll, Sholl and Rayner1996).
Why is bilinguals’ processing of code-switches costly? One hypothesis is that, while comprehending a sentence, a code-switch brings cross-linguistic knowledge sources into direct conflict (Adler et al., Reference Adler, Valdés Kroff and Novick2020; Wu & Thierry, Reference Wu and Thierry2013). Consider (1):
(1) “La electricista tiene la box with her tools.” [The electrician has the box with her tools.] (adapted from Johns et al., Reference Johns, Valdés Kroff and Dussias2019)
During sentence processing, readers and listeners make parsing commitments as linguistic input is perceived incrementally, and even predictively (Altmann & Kamide, Reference Altmann and Kamide1999), guided by phonological, syntactic, semantic, and discourse-level cues to interpretation (e.g., Boland & Blodgett, Reference Boland and Blodgett2001; Chambers et al., Reference Chambers, Tanenhaus, Eberhard, Filip and Carlson2002; Trueswell et al., Reference Trueswell, Tanenhaus and Garnsey1994). Upon encountering the feminine determiner “la,” bilingual comprehenders may form expectations about the upcoming input – e.g., Spanish phonology, a feminine Spanish noun, or perhaps just Spanish in general based on the speaker's identity (Beatty-Martínez & Dussias, Reference Beatty-Martínez and Dussias2019). The arrival of the English word “box” may conflict with their expectations, and the resolution of this conflict may incur a switch cost. Findings from language processing studies support this interpretation: bilinguals increase their engagement of cognitive control – a mechanism that assists in conflict resolution (Botvinick et al., Reference Botvinick, Braver, Barch, Carter and Cohen2001) – when reading code-switched sentences or when exposed to mixed-language environments (Adler et al., Reference Adler, Valdés Kroff and Novick2020; Wu & Thierry, Reference Wu and Thierry2013). A reasonable inference then is that switch costs are indicative of a representational conflict in sentence processing that takes time to resolve.
However, switch costs can be attenuated under supporting contexts (Beatty-Martínez et al., Reference Beatty-Martínez, Valdés Kroff and Dussias2018; Guzzardo Tamargo et al., Reference Guzzardo Tamargo, Valdés Kroff and Dussias2016) and in fact are not always found (Gullifer et al., Reference Gullifer, Kroll and Dussias2013; Johns et al., Reference Johns, Valdés Kroff and Dussias2019; Moreno et al., Reference Moreno, Federmeier and Kutas2002). Some suggest that bilinguals experience no switch costs when code-switches are naturalistic and appropriate in a particular social situation (Beatty-Martínez et al., Reference Beatty-Martínez, Guzzardo Tamargo and Dussias2021; Gosselin & Sabourin, Reference Gosselin and Sabourin2021; Johns et al., Reference Johns, Valdés Kroff and Dussias2019; Moreno et al., Reference Moreno, Federmeier and Kutas2002). Bilinguals also regularly produce and understand code-switches naturally without suffering any serious impediment to communication (e.g., Poplack, Reference Poplack1980). Yet the reasons underlying why switch costs emerge to varying degrees across studies remains an open question. One reason may be that processing demands differ by the grammatical categories involved at the switch: certain code-switches may involve lessened or even no representational conflict, and thereby elicit decreased costs compared to other switch types. The purpose of the current study is to investigate factors that modulate the magnitude of switch costs across a broad range of switch types to better understand when and why costs emerge.
Linking production patterns to switch costs during comprehension
The extent to which integrating code-switches in comprehension generates cross-language competition – which in turn may lead to measurable switch costs – likely depends on many factors, such as patterns of code-switching use in the community. For example, returning to the code-switched phrase in Example (1), the English noun “box” is preceded by the Spanish article “la,” which encodes for feminine grammatical gender. In U.S. Spanish–English bilingual communities, it is common to find English nouns preceded by the Spanish article “el” instead (e.g., Beatty-Martínez & Dussias, Reference Beatty-Martínez and Dussias2017; Valdés Kroff, Reference Valdés Kroff, Guzzardo, Mazak and Parafita2016). Interestingly, “el” marks for masculine grammatical gender, thus potentially creating cross-linguistic conflict because the Spanish translation equivalent for “box” is the Spanish feminine noun “caja.” Despite this potential conflict, these mixed Spanish-article English-noun constructions are among the most common code-switch types in Spanish–English bilingual speech (Poplack, Reference Poplack1980). Consequently, for bilinguals in the U.S., these code-switches might not seem implausible and thus may generate relatively little conflict across linguistic representations.
Indeed, research indicates that context and rates of exposure matter: the ease with which code-switches are processed varies on the basis of an individual's code-switching experience (Beatty-Martínez & Dussias, Reference Beatty-Martínez and Dussias2017), the community norms (Vaughan-Evans et al., Reference Vaughan-Evans, Parafita Couto, Boutonnet, Hoshino, Webb-Davies, Deuchar and Thierry2020), the language background of others in the interaction (Kaan et al., Reference Kaan, Kheder, Kreidler, Tomić and Valdés Kroff2020), and the type of code-switch itself (Beatty-Martínez & Dussias, Reference Beatty-Martínez and Dussias2017; Guzzardo Tamargo et al., Reference Guzzardo Tamargo, Valdés Kroff and Dussias2016). However, the overarching factors that determine switch-cost magnitude are still being mapped out. While code-switch type can affect switch costs, prior work focuses primarily on within-category comparisons (e.g., switches within the noun phrase: masculine vs. feminine noun phrases, Beatty-Martínez & Dussias, Reference Beatty-Martínez and Dussias2017; adjective-noun vs. noun-adjective, Vaughan-Evans et al., Reference Vaughan-Evans, Parafita Couto, Boutonnet, Hoshino, Webb-Davies, Deuchar and Thierry2020; switches within the verb phrase: progressive auxiliary verbs vs. perfective auxiliary verbs, Guzzardo Tamargo et al., Reference Guzzardo Tamargo, Valdés Kroff and Dussias2016). What exactly makes one code-switch more difficult to process than another? Is there a parsimonious explanation to describe the general switch-cost patterns for the many types of code-switches that bilinguals confront?
Here, we examine the idea that exposure to certain types of switches in production makes them easier to integrate during comprehension, owing to increased experience: switches like “el box” may be easier to process for code-switching bilinguals in the U.S. who hear or read this type of code-switch frequently. More generally, the reason why bilinguals are exposed more to certain types of switches than others may originate from production-based demands that cause certain types of switches to be more or less commonly produced, leading to asymmetrical distributions of code-switch types. Outside the domain of code-switching, this notion has been formalized as the Production-Distribution-Comprehension (PDC) model (MacDonald, Reference MacDonald2013), which posits that demands on production increase the frequency of certain constructions over others, thereby adjusting rates of exposure that affect comprehension: more frequent constructions become easier to understand than less frequent ones.
Applying the PDC model to the comprehension of code-switches
We start by evaluating why we might observe asymmetrical distributions of code-switches in production within the PDC framework. Consider MacDonald's proposal that production is partially guided by organizing easier elements in an utterance first (easy-first principle). Code-switches are likelier to appear later in a prosodic unit (Johns & Steuck, Reference Johns and Steuck2021), which may indicate that code-switches are intentionally associated with more difficult content to be expressed (e.g., lower frequency words, Tomić & Valdés Kroff, Reference Tomić and Valdés Kroff2022) or that they are used as a discursive strategy by the speaker to switch into a more readily accessible language. A second principle is the use and reuse principle, in which speakers are likely to repeat aspects of prior productions, such as how speakers are likely to reuse the syntactic structure of earlier utterances (i.e., structural priming; Bock, Reference Bock1986). However, code-switching itself is not overtly produced material or a particular syntactic structure, but rather a point at which language choice changes. Thus, extending this principle to code-switching suggests that speakers may prefer to code-switch at similar syntactic junctures (i.e., between a determiner and noun), which accumulate into predictable distributions over time. In a series of studies using a structural priming methodology, Kootstra and colleagues demonstrated that naïve participants are more likely to produce a code-switch immediately after a confederate has code-switched and when lexical and structural factors such as cognate status and word order are congruent across bilinguals’ languages (Kootstra et al., Reference Kootstra, Van Hell and Dijkstra2010, Reference Kootstra, Van Hell and Dijkstra2012, Reference Kootstra, Dijkstra and Van Hell2020). Similarly, Fricke and Kootstra (Reference Fricke and Kootstra2016) examined a Spanish–English bilingual corpus and found that preceding utterances that contain a code-switch are more likely to predict a code-switch in a current utterance. Of course, not all patterns of code-switches in production can be explained by production demands, which becomes apparent when we consider that different bilingual populations display different code-switching behaviors (e.g., Beatty-Martínez et al., Reference Beatty-Martínez, Navarro-Torres, Dussias, Bajo, Guzzardo Tamargo and Kroll2020b; Blokzijl et al., Reference Blokzijl, Deuchar and Parafita Couto2017; Poplack, Reference Poplack1988). Here, we do not focus on why code-switches occur in production, but rather on how comprehenders deal with different code-switch types based on their frequency of use.
Critically, the PDC model also posits that comprehenders can use their exposure to production distributions to guide real-time processing: they track the frequency of various grammatical constructions that speakers produce, and more frequent ones become easier to process with experience (e.g., Wells et al., Reference Wells, Christiansen, Race, Acheson and MacDonald2009). For example, object-extracted relative clauses (ORCs) such as “the man that the woman hugged” are generally less frequently produced than subject-extracted relative clauses (SRCs), e.g., “the man that hugged the woman.” Consequently, SRCs are generally easier to process in comprehension (Gennari & MacDonald, Reference Gennari and MacDonald2009), but increased exposure to relative clauses overall increases sensitivity to the syntactic forms, and ORCs become easier to process with that experience (Wells et al., Reference Wells, Christiansen, Race, Acheson and MacDonald2009). Similarly, the frequency of different types of code-switches may support learning over time, where exposure to a variety of often-produced switch types elicits less conflict and thus decreases processing difficulty. Rates of exposure to bilingual production patterns would therefore modulate the apparent costs associated with integrating code-switches during comprehension.
It is unclear though if the logic of the PDC model applies to bilinguals’ comprehension of code-switches. Is the syntactic juncture at which a switch occurs the type of statistical information that people track? Moreover, the PDC model was formulated to explain how alternative syntactic structures are processed. A code-switched word or phrase often maintains the same meaning and lexical properties as a more expected word or phrase that continues in the same language, and in many cases code-switching may not alter the syntactic structure in any way, especially in typologically close languages. It is thus an open issue whether the PDC model can accommodate code-switching patterns – namely, whether bilingual comprehenders implicitly exploit the statistics of sentence production distributions that differ only in the languages used, and not necessarily in meaning or sentence structure.
If the PDC model applies to the comprehension of code-switches, we would expect smaller switch costs during comprehension of code-switch types that are more frequent in production. Past studies have provided preliminary evidence that switch-cost magnitude in comprehension aligns with corpus patterns of switch types in production. In an electroencephalography (EEG) study, bilinguals with frequent code-switching experience demonstrated a larger late positive component (LPC) – indicating more integration difficulty – for uncommon feminine determiner-noun switches as compared to masculine ones (Beatty-Martínez & Dussias, Reference Beatty-Martínez and Dussias2017). Similarly, eye-tracking studies indicate that more frequent code-switches within verb phrases are easier to comprehend than less frequent ones (Dussias et al., Reference Dussias, Guzzardo Tamargo, Valdés Kroff and Gerfen2014; Guzzardo Tamargo et al., Reference Guzzardo Tamargo, Valdés Kroff and Dussias2016; Valdés Kroff et al., Reference Valdés Kroff, Guzzardo Tamargo and Dussias2018).
However, these studies each narrowly focus on a single class of code-switch (e.g., switches in noun or verb phrases) without comparing switch types in different categories, limiting the conclusions that can be drawn and perhaps strongly skewing the production distributions within the experiments. It is difficult then to determine if the PDC model explains switch costs outside of specific paired comparisons, particularly both across and within categories of switches at noun and verb sites. In fact, there are currently no sentence processing studies that compare costs for switches in noun and verb phrases (but see Ng et al., Reference Ng, Gonzalez and Wicha2014; Rossi et al., Reference Rossi, Dussias, Diaz, van Hell and Newman2021). Thus, there remain open questions about how broadly the PDC model applies to the comprehension of code-switches, as well as questions about how other factors might influence switch-cost magnitude.
If the PDC model does not apply to the comprehension of code-switches, then we expect that switch costs will not vary consistently based on production patterns found in corpora. In this case, more specific contextual factors may drive most of the variation in switch costs. Although the PDC model allows for other factors – such as the comprehender's expectations for a specific interaction or the speaker's attempts to tailor their speech to their audience – to play a role in modulating the ease of comprehension, the model highlights frequency in production as a more important factor. If production frequency is not a crucial ingredient, then we expect contextual factors such as the topic of conversation, the specific sentence's context, or the identity of the interlocutor (e.g., Kaan et al., Reference Kaan, Kheder, Kreidler, Tomić and Valdés Kroff2020) to play a large part in determining switch-cost magnitude. Moreover, prior work suggests that the relative engagement status of cognitive control – whether it is in a stronger or weaker state – can dynamically affect language processing. For example, listeners revise their misinterpretations of temporarily ambiguous sentences faster and more accurately when cognitive control has been experimentally upregulated (Hsu & Novick, Reference Hsu and Novick2016; Hsu et al., Reference Hsu, Kuchinsky and Novick2021; see also Ovans et al., Reference Ovans, Hsu, Bell-Souder, Gilley, Novick and Kim2022). The state of cognitive control in a particular interaction may also impact the comprehension of code-switches (see Salig et al., Reference Salig, Valdés Kroff, Slevc and Novick2021 for a discussion). Here, we test how such contextual and cognitive factors impact switch-cost variation apart from more general distribution learning.
The present study
The overall goal of this study is to characterize the factors that influence processing costs associated with bilingual language comprehension, in particular to: 1) determine if distributions in production can predict processing ease; 2) identify language experience factors that might additionally modulate switch costs; and 3) investigate if cognitive control engagement modulates switch costs.
To address the first and primary objective, Spanish–English bilinguals read single-language sentences, in either English or Spanish, or they read sentences that code-switched from Spanish to English in a noun or a verb phrase. Bilinguals read at their own pace in a task administered remotely via the internet, allowing us to calculate the difference in time spent reading code-switched regions as compared to single-language equivalents – for instance, how much longer it takes to read “la box” as compared to “the box” or “la caja.”
We predicted that, consistent with the PDC model, more frequent switch types should generate smaller switch costs compared to less frequent types. To make specific predictions, we examined corpora of Spanish–English bilingual language use. Although we recruited participants remotely from many communities and cannot know their specific individualized exposure to code-switch types, we take prior corpora patterns as a reasonable estimate of which types of code-switches Spanish–English bilinguals are regularly exposed to. As can be seen in Figure 1, masculine determiner-noun code-switches like “el soap” are more common than feminine ones like “la box” (Beatty-Martínez & Dussias, Reference Beatty-Martínez and Dussias2017; Valdés Kroff, Reference Valdés Kroff, Guzzardo, Mazak and Parafita2016). Progressive tense verb-phrase switches (e.g., “mis amigos están cooking”) are more common than perfective ones (e.g., “mis amigos han cooked”); perfective tense verb-phrase switches at the auxiliary location (e.g., “mis amigos have cooked”) are more common than perfective tense verb-phrase switches at the participle location (e.g., “mis amigos han cooked,” Guzzardo Tamargo et al., Reference Guzzardo Tamargo, Valdés Kroff and Dussias2016); and noun phrase code-switches are more common than verb phrase code-switches (Poplack, Reference Poplack1980). Note that these distributions were drawn from corpora of both verbal and written language when possible. While code-switching is typically considered to be a spoken language phenomenon, corpus analyses that have compared written and spoken language corpora find similarities in grammatical patterns of code-switching across the two modalities (Montes-Alcalá, Reference Montes-Alcalá and Jacobson2000, Reference Montes-Alcalá and Cruz Cabanillas2001; Callahan, Reference Callahan2003; Guzzardo Tamargo et al., Reference Guzzardo Tamargo, Valdés Kroff and Dussias2016), although discourse functions may differ (Barasa, Reference Barasa2016) and variation can exist between corpora and genres (Enghels, Reference Enghels2018; Guzmán et al., Reference Guzmán, Richard, Serigos, Bullock and Toribio2017). Thus, smaller costs should emerge generally for a range of more frequently produced, and thus more commonly experienced, types of code switches. Based on data from corpora of bilingual Spanish–English language use, our hypotheses are:
1. Masculine determiner-noun switches (e.g., “el soap”) will be easier to process (have smaller switch costs) than feminine determiner-noun switches (e.g., “la box”).
2. Perfect tense switches at the auxiliary location (e.g., “mis amigos have cleaned”) will be easier to process than perfect tense switches at the participle location (e.g., “mis amigos han cleaned”).
3. Progressive tense switches (e.g., “mis amigos están/are cleaning”) will be easier to process than perfect tense switches (e.g., “mis amigos han/have cleaned”) at both the participle and the auxiliary location.
4. Mixed noun-phrase switches (switch at the noun) will be easier to process than mixed verb-phrase switches (switch at the participle).
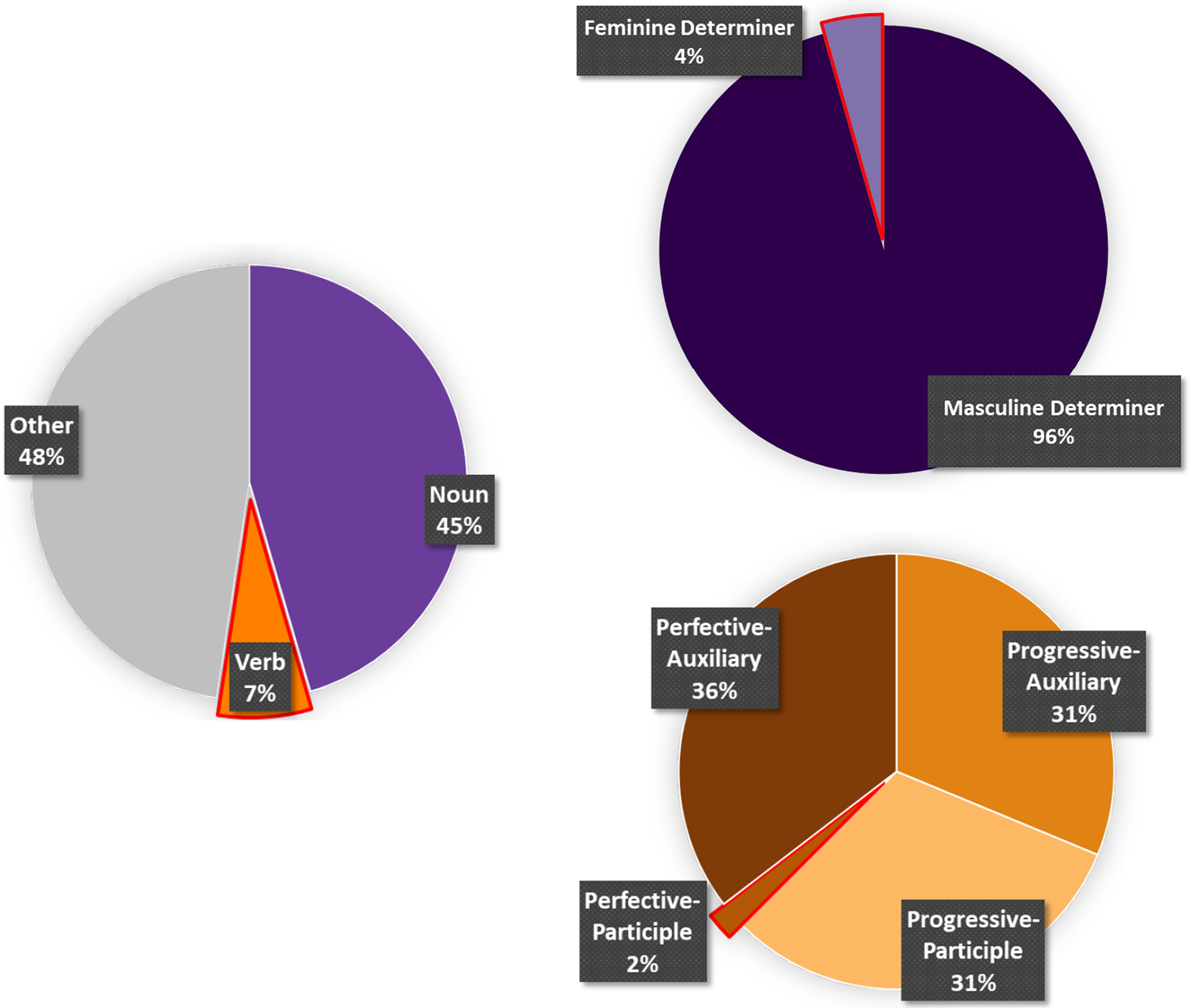
Figure 1. Distributions of Code-switch Types in Bilingual Language Production. The left pie chart represents all intrasentential code-switches from a corpus of speech from Puerto Rican bilinguals in New York City; the Other category accounts for all code-switches not in noun or verb phrases, such as switches at prepositions or adverbs (Poplack, Reference Poplack1980, Table 2). The upper right pie chart in purple represents all Spanish determiner to English noun code-switches from a corpus of speech from code-switching bilinguals in the U.S. (Beatty-Martínez & Dussias, Reference Beatty-Martínez and Dussias2017, Table 9). The lower right pie chart in orange represents all Spanish to English code-switches within perfective or progressive verb phrases from an analysis of an oral corpus from bilinguals in Miami and a written corpus from a Gibraltar newspaper (Guzzardo Tamargo et al., Reference Guzzardo Tamargo, Valdés Kroff and Dussias2016, Table 1). Red-outlined wedges indicate the least frequent type of switch within each pie chart.
Table 1. Participants’ Language History (n = 101).

Note. Standard deviation in parentheses unless otherwise noted. AoA = Age of Acquisition.
Self-rated ability was calculated by combining participants’ self-rating of their proficiency in writing, reading, speaking, and understanding in the language on a 1-10 point scale.
Alternatively, if the PDC model does not apply to the comprehension of code-switches, then we would expect variation in switch costs to be unrelated to production frequency, suggesting that immediate contextual factors may play a much larger role in switch-cost magnitude than longer-term learning. To preview our results, we find that the PDC model explains variation in switch costs but may not alone capture the full picture of factors at play in the comprehension of code-switches – offering a novel contribution to the literature on how production and comprehension interact in code-switch processing.
For the second objective, we were interested in whether switch costs are modulated by individual factors beyond switch type, specifically code-switching experience and exposure to each language. To capture exposure to each language, we calculated a language entropy measure (Gullifer & Titone, Reference Gullifer and Titone2020) based on percent of daily exposure to each language; higher language entropy indicates more balanced exposure. We made the exploratory prediction that:
5. Individual language experience, defined as code-switching experience and language entropy, will modulate switch costs.
Although this prediction and associated analyses were exploratory, it was motivated by prior studies demonstrating differential code-switch processing based on experience (Beatty-Martínez & Dussias, Reference Beatty-Martínez and Dussias2017; Blanco-Elorrieta & Pylkkänen, Reference Blanco-Elorrieta and Pylkkänen2017; Gosselin & Sabourin, Reference Gosselin and Sabourin2021; Valdés Kroff et al., Reference Valdés Kroff, Guzzardo Tamargo and Dussias2018). Given their greater experience with code-switches, we also thought it plausible that habitual code-switchers may show a greater modulation of switch costs based on switch type – showing even smaller costs for frequent switch types and even larger costs for infrequent switch types, compared to non-switching bilinguals. While we expected code-switching experience to be the best individual factor in explaining switch cost variation, we also anticipated that a bilinguals’ daily exposure to both languages may affect switch costs. Given that our primary hypothesis focuses on how the learning of frequency distributions through exposure may affect processing, we were interested in how a direct measure of exposure to both languages may interact with our results, so we used a language entropy measure to operationalize daily language exposure (Gullifer & Titone, Reference Gullifer and Titone2020).
For the third objective, we expected that a bilingual's cognitive state at the time of reading a code-switch – particularly their relative engagement of cognitive control – might affect switch costs. Recall that, on one account, switch costs derive from conflict between the code-switched input and listeners’ expectations. Past work demonstrates that bilinguals increase cognitive control in code-switched or mixed-language contexts (Adler et al., Reference Adler, Valdés Kroff and Novick2020; Wu & Thierry, Reference Wu and Thierry2013), presumably to help them resolve the conflict that a code-switch presents. Given that code-switches seem to require conflict resolution operations, we asked if bilinguals might process code-switches more easily (i.e., have reduced switch costs) if their state of cognitive control engagement was experimentally increased before reading the code-switch (Hsu & Novick, Reference Hsu and Novick2016; Hsu et al., Reference Hsu, Kuchinsky and Novick2021; Ovans et al., Reference Ovans, Hsu, Bell-Souder, Gilley, Novick and Kim2022; Thothathiri et al., Reference Thothathiri, Asaro, Hsu and Novick2018).
To address this, bilinguals completed trials from a Flanker task before reading our critical sentences. On incongruent Flanker trials, participants indicated the direction of a central arrow despite flanking arrows pointing in a conflicting direction; these trials are known to increase cognitive control compared to congruent trials in which the central and flanking arrows point in the same direction and thus do not involve conflict (Eriksen & Eriksen, Reference Eriksen and Eriksen1974; Gratton et al., Reference Gratton, Coles and Donchin1992). Therefore, we predicted that:
6. Experimentally increasing cognitive control engagement with an incongruent Flanker trial will assist processing of an immediately subsequent code-switch (i.e., result in a smaller switch cost).
Method
This study was pre-registered. The materials, R script used for analysis, and data from participants who consented to data sharing are available on OSF (https://osf.io/5yzna/).
Participants
A total of 152 self-identified Spanish–English bilinguals participated online using their own devicesFootnote 1 and received class credit or were entered into a gift card drawing as compensation. Of those, 45 people were excluded from analysis based on pre-registered exclusion criteria: scoring less than 80% on comprehension questions (to ensure attention to the task), having accuracy lower than 50% on Flanker trials (to require above-chance performance), and/or scoring lower than 30% on a grammar assessment (to verify approximately above-chance performance on these multiple-choice assessments). We intentionally set the grammar assessment criteria to greater than chance level to be widely inclusive of bilingual participants, since testing was conducted in an online modality. While the Spanish grammar test that we use (DELE) has been widely used in past studies on Spanish–English bilinguals (e.g., Cuza & Frank, Reference Cuza and Frank2011; Montrul et al., Reference Montrul, Foote and Perpiñán2008; Montrul & Slabakova, Reference Montrul and Slabakova2003; Pascual y Cabo & Gómez Soler, Reference Pascual y Cabo and Gómez Soler2015), there are important criticisms to its use as the sole determinant of proficiency, especially in U.S.-based heritage speakers of Spanish (Pascual y Cabo, Reference Pascual y Cabo2013). In particular, its primary objective is as a proficiency certification assessment for second language speakers and is based on Peninsular Spanish norms. As a further check against these limitations, participants performed a comprehension task during the main experiment. An additional 6 participants were excluded due to a recording error. Therefore, 101 bilinguals (86 female, 15 male) were included in analyses. On average, the bilinguals reported being exposed to English more frequently than Spanish in their daily lives, although 59% of the bilinguals learned Spanish before English. Additional participant characteristics are shown in Table 1. Overall, questionnaire responses suggest that the sample consists of relatively balanced bilinguals who are slightly English dominant.
Materials and design
Participants completed the experiment remotely on PCIbex (Zehr & Schwarz, Reference Zehr and Schwarz2018). In the experiment, we pseudorandomly interleaved Flanker arrow trials with self-paced reading sentence trials. After completing the experimental task, participants completed the language history questionnaire and grammar assessments on Qualtrics.
Sentence stimuli
We included 96 critical sentences. In any given list, half of the critical sentences were code-switched and half were in a single-language – either English or Spanish (single-language condition). Forty-eight of the critical sentences involved a determiner-noun code-switch or single-language equivalent, and 48 involved a verb-phrase code-switch or single-language equivalent. Determiner-noun switches could be feminine or masculine. Verb-phrase switches could occur at either the auxiliary or at the participle and could be in the perfective tense or progressive tense. Each participant read 48 critical single-language sentences (24 determiner-noun, 24 verb-phrase) and 48 critical code-switched sentences (12 feminine determiner-noun, 12 masculine determiner-noun, 6 auxiliary-perfective, 6 auxiliary-progressive, 6 participle-perfective, 6 participle-progressive). Whether a particular item appeared as code-switched or as single-language depended on the participant's randomly assigned list. Table 2 outlines the distribution of sentence types. All code-switches were from Spanish to English to align with typical code-switching practices in the U.S. (Beatty-Martínez & Dussias, Reference Beatty-Martínez and Dussias2017; Blokzijl et al., Reference Blokzijl, Deuchar and Parafita Couto2017). Determiner-noun stimuli were a subset of materials found in Adler et al. (Reference Adler, Valdés Kroff and Novick2020) and Johns et al. (Reference Johns, Valdés Kroff and Dussias2019). Within our materials, the determiner gender always agreed with the gender of the noun in Spanish: we included switches like “la box” (Spanish equivalent: “la caja”) and “el soap” (Spanish equivalent: “el jabón”), but not “el box,” ensuring that there was not an additional grammatical mismatch variable along with the code-switch type manipulation. Verb stimuli were a subset of materials found in Adler et al. (Reference Adler, Valdés Kroff and Novick2020) and Guzzardo Tamargo et al. (Reference Guzzardo Tamargo, Valdés Kroff and Dussias2016). We also included 72 filler sentences from Adler et al. (Reference Adler, Valdés Kroff and Novick2020). Of these, only 8 contained code-switches so that 33% of all sentence stimuli in the experiment contained code-switches, which approximates observed rates of code-switching in U.S. bilinguals (Beatty-Martínez et al., Reference Beatty-Martínez, Navarro-Torres and Dussias2020a; Johns et al., Reference Johns, Valdés Kroff and Dussias2019; Piccinini & Arvaniti, Reference Piccinini and Arvaniti2015; Torres Cacoullos & Travis, Reference Torres Cacoullos and Travis2018).
Table 2. Types of Code-switches in Critical Sentences.

Note. Here we show code-switched examples of each type of item. However, participants would only see half of the critical sentences as code-switched and would see the other half in a single-language (either in Spanish or in English depending on the single-language condition of their list).
Flanker trials
There were 96 critical Flanker trials (48 congruent, 48 incongruent) as well as 112 filler Flanker trials (85 congruent, 27 incongruent). Flanker stimuli showed a central arrow in the middle of the screen flanked by two arrows on each side. On congruent trials, the flanking arrows pointed in the same direction as the center arrow. On incongruent trials, the flanking arrows pointed in the opposite direction as the center arrow. Participants were instructed to press “J” if the central arrow was pointing right or “F” if the central arrow was pointing left.
Comprehension questions
A yes/no comprehension question followed all 96 critical sentences and 18 of the filler sentences. Questions tested attention to main ideas in the sentences. For example, the question for Example (1) above (repeated here: “La electricista tiene la box with her tools”) was: “Are the tools in a bag?” (No). Questions were adapted from prior studies (Adler et al., Reference Adler, Valdés Kroff and Novick2020; Johns et al., Reference Johns, Valdés Kroff and Dussias2019) or written by the first author. Participants were instructed to press “J” to answer yes or “F” to answer no, with response key reminders visible on screen.
Interleaved Flanker-sentence trial sequences
The 96 critical trial sequences started with a Flanker trial, followed by a sentence trial, followed by a comprehension question. We also pseudorandomly interleaved 112 filler Flanker trials and 72 filler sentences to create additional sentence-to-Flanker, sentence-to-sentence, Flanker-to-Flanker, and Flanker-to-sentence pairings to discourage participants from strategically predicting upcoming trial type.
There were 8 fixed-order lists: each list contained 24 congruent Flanker-single-language sentence, 24 congruent Flanker-code-switched sentence, 24 incongruent Flanker-single-language sentence, and 24 incongruent Flanker-code-switched sentence trial pairs. List number determined the condition for any given Flanker-sentence pair. For each list, the single-language condition was either Spanish or English, and this determined the language of single-language sentences and comprehension questions (between-subjects manipulation). In all lists, all code-switches were from Spanish to English. To create the fixed-order for presentation, the 96 critical trial pairs were split into two experimental blocks and their order randomized within each block; then, filler trials were inserted.
Procedure
Within the experiment, each stimulus (sentence, Flanker, or comprehension question) was preceded by a 500-ms fixation cross with a 100-ms inter-stimulus interval between stimuli. Sentence trials were presented using a self-paced moving window paradigm in which participants saw one word at a time with the others masked with dashes (Just et al., Reference Just, Carpenter and Woolley1982). Participants used the spacebar to advance through the sentence, re-masking all previous words as they advanced.
Participants read code-switched instructions, completed a 15-trial practice block, and then completed the interleaved experimental trials in two blocks, with a short break in between. After completing the second block, participants completed a demographic and language history questionnaire followed by two grammar assessments that were presented in random order. The language history questionnaire included a question asking about what percent of participants’ time was spent in Spanish, English, and other languages. Responses to this question were used to calculate language entropy (Gullifer & Titone, Reference Gullifer and Titone2020). The questionnaire also asked participants four 5-point Likert questions about how frequently they: code-switch when speaking; code-switch when using social media; hear others code-switch in conversation; and see others code-switch when using social media. Responses to these questions were combined to create a code-switch experience score. The grammar assessments were shortened 20-question versions of those used in Adler et al. (Reference Adler, Valdés Kroff and Novick2020): an adapted English assessment from the Michigan English Language Institute College English Test (English Language Institute, 2001) and an adapted Spanish assessment from the Diploma de español como lengua extranjera [Diploma of Spanish as a Foreign Language; DELE] (Ministry of Education, Culture, and Sport of Spain, 2006). The experiment took about one hour to complete.
Data analysis
The regression models used to analyze the data were pre-registered before data collection. After applying participant exclusion criteria, individual trial exclusion criteria were applied before analysis. Sentence trials were excluded from analysis if: the prior Flanker response was inaccurate; the prior Flanker response time was more than 2.5 standard deviations away from that participant's mean; and/or the reading time in the region of interest was more than 2.5 standard deviations away from the participant's mean. At least 85% of trials were included in each analysis.
For each hypothesis (except the hypothesis about the effect of individual language experience, which is exploratory), we pre-registered analyses in four regions of interest, which are underlined and bolded in Examples (2) and (3): the critical word (the switch or single-language equivalent), the word after, the second word after, and the combined 3-word region. Prior findings from self-paced reading paradigms have shown that effects can be observed at least a word or two after a manipulation in a critical region, which is why we included a “spillover” analysis two words after the switch (Bultena et al., Reference Bultena, Dijkstra and Van Hell2015; Just et al., Reference Just, Carpenter and Woolley1982; inter alia). When analyzing verb phrases alone, the auxiliary-participle 2-word verb phrase was treated as the critical word. Auxiliaries tend to be processed in a qualitatively different way than participles and are often skipped during reading (Rayner, Reference Rayner1998). To prevent this auxiliary-specific reading effect from influencing our inferences, we analyzed the auxiliary + participle verb phrase as if it were a single word, following the design choice made in Guzzardo Tamargo et al. (Reference Guzzardo Tamargo, Valdés Kroff and Dussias2016), which included the same types of verb compounds in an eye-tracking-while-reading study. When comparing noun- to verb-phrase switches, only participle-location verb switches were included to allow for a comparison of switches that occur at a content word. For brevity, we report only results analyzing the combined 3-word critical region (or 4-word region for verb-only analyses), although all results reported as significant survive a Bonferroni correction for four analyses per hypothesis. Results in the individual word regions largely align with the combined region analyses, although switch effects generally tapered off by the second word after the switch (all analyses available on OSF at https://osf.io/5yzna/).
(2) El luchador ganó la fight in five minutes. [The wrestler won the fight in five minutes.] (adapted from Johns et al., Reference Johns, Valdés Kroff and Dussias2019)
(3) El entrenador piensa que los atletas han celebrated their win at the bar. [The coach thinks that the athletes have celebrated their win at the bar.] (adapted from Guzzardo Tamargo et al., Reference Guzzardo Tamargo, Valdés Kroff and Dussias2016)
Linear mixed-effects models were run using the lme4 package (Bates et al., Reference Bates, Maechler, Bolker and Walker2015), and p-values were approximated via the Satterthwaite degrees of freedom method with the lmerTest package in R (Kuznetsova et al., Reference Kuznetsova, Brockhoff and Christensen2017), with the predicted variable as the region's logged reading time. All models included crossed random effects for participant and item number; models that did not converge were simplified using pre-registered criteria. Two-level factors were contrast coded as -0.5 and +0.5. Centered region length (in characters) was included as a covariate in models to account for the fact that longer words tend to be read more slowly (Ferreira & Clifton, Reference Ferreira and Clifton1986; Rayner, Reference Rayner1998; Trueswell et al., Reference Trueswell, Tanenhaus and Garnsey1994). Marginal R2 values were extracted with the MuMIn R package (Barton, Reference Barton2020), and effect sizes were extracted with the sjstats R package (Lüdecke, Reference Lüdecke2020).
Results
Participants had high overall accuracy on Flanker trials (M = 0.89, SD = 0.31) and comprehension questions (M = 0.91, SD = 0.29), indicating that they were paying attention during the experiment.
Switch cost effects
Switches at noun sites
We predicted that noun switches with masculine determiners would have smaller switch costs than ones with feminine determiners because of their higher frequency in corpora. As shown in Figure 2 and Table 3, there were switch costs for noun switches, but masculine switches seemed to elicit similar switch costs as feminine switches, counter to our prediction. A mixed-effect model predicting logged reading time of the 3-word region of critical noun stimuli confirmed these observations, with a main effect of sentence type (β = −0.03, ηp2 = 0.10, p < 0.01), but no main effect of the determiner's grammatical gender (p = 0.99, ηp2 < 0.01) and no interactions involving grammatical gender (p ≥ 0.60, ηp2<0.01; model marginal R2=0.08). The model included fixed effects for sentence type (code-switched or single-language), the determiner's grammatical gender (masculine or feminine), and single-language condition (single-language sentences in English or in Spanish).

Figure 2. Noun Switches: Comprehension Switch Costs by Production Frequency. The y-axis represents the difference between the model's predicted logged reading time (in ms) for the region of interest in code-switched sentences vs. single-language equivalents. Bars represent standard error generated by the emmeans R package.
Table 3. Raw Switch Costs for 3-word Noun Region.

The model also revealed a two-way interaction between single-language condition and sentence type (β = 0.12, ηp2 = 0.22, p < 0.01) and a main effect of single-language condition (β = 0.18, ηp2 = 0.12, p < 0.01). Figure 3 demonstrates the interaction: overall, reading speeds were faster for participants who were in the English single-language condition. Additionally, there was a clear switch cost when comparing a portion of a sentence that code-switched into English with an identical portion of a single-language English sentence (in Figure 3, compare the blue and green bars in the English single-language condition). For participants in the Spanish single-language condition, however, reading times were overall longer, and participants read a portion of a sentence that code-switched into English just as slowly as a translation-equivalent portion of a single-language Spanish sentence (in Figure 3, compare the blue and green bars in the Spanish single-language condition). Indeed, exploratory analysis revealed a significant switch cost when comparing code-switches to English-only sentences, but no switch cost when comparing code-switches to Spanish-only sentences. Given that all code-switches were from Spanish to English, the lack of difference between reading times for code-switched English and single-language Spanish may reflect a switch cost in itself, as the switch temporarily negated bilinguals’ faster English reading ability, a point we return to in the Discussion. The character length covariate was also significant in the model (β < 0.01, ηp2 = 0.02, p < 0.01).

Figure 3. Noun Switches: Reading Differences by Single-Language Condition. The y-axis represents the model's predicted logged reading time (in ms) for the region of interest. Bars represent 95% confidence intervals generated by the emmeans R package using the emmip function. Since all code-switches were from Spanish to English, here we see reading times for English-language regions in both code-switched conditions and the English single-language condition; reading times for the Spanish single-language conditions are for Spanish-language regions.
Switches at verb sites
Based on corpora analyses, we predicted that perfect tense switches would have a smaller switch cost at the auxiliary location (e.g., “los editores have approved”) than the participle location (e.g., “los modelos han signed”) and that progressive tense switches would have smaller switch costs than perfective tense switches. One model was run on critical verb stimuli to address these hypotheses, with logged 4-word region (auxiliary, participle, and two subsequent words) reading time as the predicted variable. Fixed effects included sentence type, verb tense (progressive or perfective), switch location (auxiliary or participle), and single-language condition, with centered region length as a covariate.
There were no interactions including both verb tense and switch location (p ≥ 0.13, ηp2<0.02; model marginal R2=0.09), so we consider each factor individually. As before, the model showed main effects of single-language condition (β = 0.19, ηp2 = 0.10, p < 0.01), sentence type (β = −0.03, ηp2 = 0.06, p = 0.01), and their interaction (β = 0.17, ηp2 = 0.41, p < 0.01). Here, exploratory analysis of the two-way interaction again showed a switch cost when comparing code-switched English to single-language English, but a switch benefit when comparing code-switched English to single-language Spanish, as reading sped up quickly upon the switch to English. The character length covariate was significant (β<0.01, ηp2 = 0.01, p < 0.01).
Verb switch tense
As can be seen in Figure 4A and Table 4, progressive tense switches seem to elicit a smaller switch cost than perfective tense switches, in line with our prediction. The model confirmed this observation, as verb tense interacted with sentence type (β = 0.03, ηp2 = 0.03, p = 0.01); there was no main effect of verb tense (p = 0.82, ηp2 < 0.01). Exploratory analysis showed that the interaction followed the predicted pattern: progressive tense switches had no significant switch cost, while perfective tense switches did.

Figure 4. Verb Switches by Tense and Switch Location: Comprehension Switch Costs by Production Frequency. The y-axis represents the difference between the model's predicted logged reading time (in ms) for the region of interest in code-switched sentences vs. single-language equivalents. Bars represent standard error generated by the emmeans R package. Plots A and B show the same verb-phrase stimuli data, represented in different ways.
Table 4. Raw Switch Costs for 4-word Verb Region, by Verb Tense and by Switch Location.

Verb switch location
Figure 4B and Table 4 show that participle switches appeared to elicit much larger costs than auxiliary switches, which seem to elicit no cost at all. The model confirmed that switch location interacted with sentence type (β = −0.06, ηp2 = 0.12, p < 0.01); there was no main effect of switch location (p = 0.08, ηp2=0.06). Exploratory analysis showed that the interaction was in the predicted direction: switches at the auxiliary location had no significant switch cost, while switches at the participle location did. This interaction applied for both perfective and progressive code-switches, not just for perfective tense switches as we predicted.
Comparing noun and verb switch sites
Given their higher frequency in production, we predicted that noun switches would have smaller switch costs than verb switches. Figure 5 and Table 5 show that noun switches did indeed seem to elicit smaller switch costs than verb switches, at least when compared to single-language English; when compared to single-language Spanish, there appear to be no switch costs. The model predicting logged 3-word reading time for nouns and participle-location verbs confirmed this observation. There was a three-way sentence type by single-language condition by code-switch type interaction (β = 0.07, ηp2 = 0.08, p < 0.01). Exploratory analysis indicated that in the English single-language condition, there were switch costs, and switch costs were larger for verb switches than noun switches. In the Spanish single-language condition, there were no switch costs or any reading difference for nouns as compared to verbs. This is consistent with the results described above in which switch costs are washed out when we compare code-switched English to single-language Spanish due to faster English reading.

Figure 5. Noun vs. Verb Switches: Comprehension Switch Costs by Production Frequency. The y-axis represents the difference between the model's predicted logged reading time (in ms) for the region of interest in code-switched sentences vs. single-language equivalents. Bars represent standard error generated by the emmeans R package. Plot is split by the single-language condition: whether the switch cost is calculated by comparing code-switched English reading to reading of fully English equivalent regions or of fully Spanish translation-equivalent regions.
Table 5. Raw Switch Costs for 3-word Region.

The model's fixed effects included sentence type, code-switch type (noun or verb), and single-language condition, with centered region length in characters as a covariate. In addition to the three-way interaction described above, there was a sentence type by single-language condition two-way interaction (β = 0.16, ηp2 = 0.34, p < 0.01; model marginal R2 = 0.08), a main effect of sentence type (β = −0.04, ηp2=0.11, p < 0.01), a main effect of single-language condition (β = 0.18, ηp2 = 0.11, p < 0.01), and an effect of character length (β = 0.01, ηp2 = 0.02, p < 0.01). There was no main effect of code-switch type (β = −0.06, ηp2 = 0.04, p = 0.06).
Language experience
We predicted that individual language experience would affect switch costs. As an exploratory analysis, we re-ran the three models with two additional language experience factors in each: (1) code-switching experience, averaged across four self-rating questions on a 5-point scale, and (2) language entropy. Language entropy captures individuals’ daily language diversity and was calculated using the languageEntropy package (Gullifer & Titone, Reference Gullifer and Titone2020) by inserting the fraction of time that the bilinguals reported exposure to English, Spanish, and if applicable, another language. Higher language entropy values indicate more balanced exposure: an individual exposed to English 50% of the time and Spanish 50% of the time would receive a score of 1, while an individual exposed to English 100% of the time would receive a score of 0. Since participants could indicate exposure to English, Spanish, and other languages, the maximum entropy score possible was 1.58 (or log2(3) given three language choices), if exposure was split equally between three languages. Because these models were exploratory, we used a p-value threshold of 0.05.
In the model comparing determiner-noun switches, there were no significant effects including code-switching experience or language entropy (model marginal R2=0.11).
In the model comparing different types of verb code-switches, there was a three-way interaction between sentence type, switch location (at the participle vs. auxiliary), and language entropy (β=0.46, ηp2<0.01, p = 0.03; model marginal R2=0.12). There were no significant effects of code-switching experience. Using the interactions package (Long, Reference Long2019) to explore further, it seemed that as language entropy increased (exposure became more balanced), reading times became faster, and this was particularly true for code-switches at the participle location.
In the model comparing noun and verb code-switches, there was a three-way interaction between sentence type, code-switch type (noun vs. verb), and language entropy (β=0.43, ηp2=0.05, p = 0.03; model marginal R2=0.11); there were no significant effects of code-switching experience. Exploring further, it again seemed that more balanced language exposure was associated with faster reading. This effect appeared uniform within single-language sentences, but was greater for code-switched verbs than for code-switched nouns.
Cognitive control manipulation
We predicted that after incongruent Flanker trials – which experimentally increase cognitive control engagement relative to congruent trials – bilinguals would demonstrate smaller switch costs. A model was run to predict logged 3-word reading time with fixed effects of sentence type, prior Flanker congruency, experimental block, and single-language condition, along with a centered length covariate. Block was included because a similar cognitive control manipulation had different effects in different experimental halves (Adler et al., Reference Adler, Valdés Kroff and Novick2020).
The model revealed similar effects of sentence type and single-language condition as models discussed above. There was a main effect of block (β = −0.21, ηp2=0.41, p < 0.0001; model marginal R2 = 0.14); responses became faster in the second half of the experiment. Against our prediction, there were no effects of or interactions involving prior Flanker congruency (ηp2 = 0.003, p = 0.52): prior cognitive control engagement did not seem to affect switch costs.
Three additional exploratory models with additional predictors also found no effects of prior Flanker congruency, even when including code-switch type (determiner-noun vs. perfective vs. progressive), language experience (code-switching experience and language entropy), or the location of the first switched word in the sentence. In two more exploratory models, we re-ran the model with comprehension question reaction time or accuracy as the dependent variable to determine if cognitive control engagement had a later effect on offline comprehension; again, we found no effects of prior Flanker congruency.
As a manipulation check, we confirmed that Flanker congruency did affect responses to Flanker trials, observing the classic effect of longer response times (β=0.188, ηp2=0.90, p < 0.01) and lower accuracy (β=−6.15, p < 0.0001) for incongruent trials than congruent ones. That is, the cognitive control manipulation worked but did not affect switch costs.
Discussion
Summary of main findings
In this study, we tested whether the statistical regularities of various types of code-switches that speakers produce regulate the costs of integrating a code-switch during real-time comprehension. Bilinguals experienced smaller switch costs for progressive verb-phrase switches than perfective verb-phrase switches; for auxiliary-location verb-phrase switches than participle-location verb-phrase switches; and for switches within noun-phrases as compared to verb-phrases. These findings align with the predictions of the PDC model: more frequent switch types in production elicited smaller switch costs in comprehension. This suggests that bilinguals’ processing of code-switches is influenced by probabilistic constraints on where intrasentential switches are likely to occur, and provides insight into why the magnitude of switch costs may vary in previous observations. Overall, this pattern links frequency in production to ease of comprehension and suggests a role for statistical learning mechanisms in bilingual language comprehension that modulate the magnitude of switch costs. Masculine noun switches, however, induced similar switch costs to feminine noun switches, which is ostensibly inconsistent with the predictions of the PDC model (but see further discussion below). Our findings support the general idea that the PDC model applies to the processing and comprehension of code-switched input.
However, we found no effect of code-switching experience and no consistent effect of language entropy on switch costs. This may be surprising given that our general patterns show that bilinguals are sensitive to the relative frequency with which different code-switching forms are produced, and that exposure to (and thus experience with) those forms is an important factor that influences the cost of integrating a code switch during comprehension. We also found no evidence that an individual's state of cognitive control affects their processing of a code-switch despite prior evidence demonstrating such a relationship (e.g., Adler et al., Reference Adler, Valdés Kroff and Novick2020). Below, we elaborate on the full range of our results and attempt to consolidate some ideas about what we did and did not observe in this study, and why.
Conflict in comprehension of code-switches
Our findings indicate that bilinguals learn the distributions of code-switch types and process frequent switches more easily than infrequent ones. How might the statistically more common switches in production result in smaller switch costs during comprehension?
Recall that switch-cost magnitude may reflect the amount of conflict that bilinguals experience when processing the code-switch (Adler et al., Reference Adler, Valdés Kroff and Novick2020; Wu & Thierry, Reference Wu and Thierry2013). Under this explanation, more frequent switches may come to elicit less conflict given repeated exposure over time. Consequently, more frequent switches may require less time for conflict resolution and result in smaller switch costs. This largely aligns with the results we found in which bilinguals read more frequent switch types faster.
If this explanation is correct and switch costs do reflect the time needed for cognitive control operations to resolve cross-linguistic conflict, then we would predict that increased engagement of cognitive control before encountering a code-switch would also result in smaller switch costs. This is because when cognitive control is experimentally upregulated, it remains engaged during the processing of ensuing conflict on a subsequent trial, helping people meet task demands, including during language processing (e.g., Hsu & Novick, Reference Hsu and Novick2016; Hsu et al., Reference Hsu, Kuchinsky and Novick2021; Ovans et al., Reference Ovans, Hsu, Bell-Souder, Gilley, Novick and Kim2022; Thothathiri et al., Reference Thothathiri, Asaro, Hsu and Novick2018). However, we found that upregulating cognitive control with an incongruent Flanker trial, compared to a congruent one, before a code-switch did not impact our measure of switch costs. Given the null result, we are not licensed to draw any inferences, but we speculate on a few reasons why the Flanker manipulation failed to modulate sentence processing as it has in prior studies. One possibility is that, while our self-paced reading paradigm was sensitive enough to detect differences in switch costs, it may not have been sensitive enough to detect a small interaction between prior Flanker and current sentence trial types. As compared to a method like eye-tracking, self-paced reading is relatively coarse-grained because it is limited by how quickly participants press buttons to reveal the words, rather than taking samples of eye position on the scale of milliseconds. Indeed, prior research observing such interactions has done so using dependent measures that have better temporal resolution, like changes in eye-movement patterns during spoken comprehension (Hsu & Novick, Reference Hsu and Novick2016; Hsu et al., Reference Hsu, Kuchinsky and Novick2021; Navarro-Torres et al., Reference Navarro-Torres, Garcia, Chidambaram and Kroll2019; Thothathiri et al., Reference Thothathiri, Asaro, Hsu and Novick2018) and evoked-response potentials in the EEG record (e.g., the P600 effect; Ovans et al., Reference Ovans, Hsu, Bell-Souder, Gilley, Novick and Kim2022). Future research should address whether cognitive-control engagement impacts processing costs associated with comprehending a code-switch using methods that have more responsive timing characteristics.
In addition, we note that if more frequent switch types elicit relatively little conflict, as our findings primarily suggest, then the need for cognitive control may be reduced in such situations. Thus, in general, variations in the state of cognitive control should have limited effect on the processing of code-switch types that recur with high statistical regularity in natural input. While an exploratory analysis that includes code-switch type did not show that cognitive control engagement differentially affected the processing of frequent versus infrequent forms, future studies that explicitly manipulate frequency of code-switch structures in a similar design should further investigate this hypothesis.
Given these considerations, we continue to interpret our results as indicating that bilinguals are able to learn the statistical distributions of code-switch types and apply that knowledge to comprehension processes. In what follows, we consider why the statistical learning account failed to accurately predict some of our results. We also consider alternate explanations of our results and the limitations of those explanations.
Why did code-switching experience not modulate switch costs?
Although many of our results support the interpretation that the PDC model can explain switch-cost variation, we did not find any effect of individuals’ code-switching experience, as the model would predict. Under the PDC model, it is specifically those with exposure to various constructions (here, switches) that learn the statistical regularities in the input, which affects processing. Against our prediction, we found no effect of code-switching experience – as calculated based on self-reported exposure to and production of code-switches – on switch-cost magnitude. We also found no reliable pattern for effects of language entropy, a measure of daily language exposure balance: more balanced language exposure seemed to afford participants a faster reading speed at times, but not in any pattern that clearly aligned with production frequency.
One possibility is that our remote self-paced reading technique was not sensitive enough to detect interactions between PDC effects and individual experience. It is also possible that our self-report code-switching questions did not measure code-switching experience with enough sensitivity. The field is moving towards well-validated measures of code-switching experience (e.g., Bilingual Code-switching Profile, Olson, Reference Olson2022), which will provide a more sensitive measure. Similarly, our sample may have been too homogenous on the experience dimension, or perhaps only a small amount of code-switching exposure is needed to observe the predicted frequency effects; it may be the case that after a certain threshold, additional exposure to code-switching distributions will not affect frequency effects on processing in comprehension. Prior studies that have examined code-switching experience have focused on between-group comparisons where greater differences are observed in habitual code-switching practices (e.g., Beatty-Martínez & Dussias, Reference Beatty-Martínez and Dussias2017; Valdés Kroff et al., Reference Valdés Kroff, Guzzardo Tamargo and Dussias2018). Given these possibilities, we do not interpret the lack of individual language experience effects as necessarily problematic for the PDC model. Future work should continue to test for the effect of individual experience and community-based habits on comprehension of code-switches and how it interacts with other predictors of switch-cost magnitude such as production frequency.
Relatedly, future work should test whether a person's level of experience specifically affects their processing of less regular code-switches, as expected under a constraint-based model of language comprehension (MacDonald & Christiansen, Reference MacDonald and Christiansen2002). This is because the ease with which irregular constructions are interpreted (e.g., ORCs, or perhaps code-switches at idiosyncratic syntactic sites) relies heavily on how much exposure to that identical construction an individual receives (Juliano & Tanenhaus, Reference Juliano and Tanenhaus1994; Wells et al., Reference Wells, Christiansen, Race, Acheson and MacDonald2009). Such a frequency-by-exposure interaction might be especially relevant to capturing effects of experience on switch costs during comprehension. In line with such predictions, one might expect that such frequency effects may result in differential priming (i.e., less frequent code-switch constructions should result in greater priming effects).
Why did frequency not predict noun-phrase switch costs?
Most of our findings support the inference that the PDC model applies to the comprehension of code-switches; however, we found that more frequent masculine noun switches were not easier to process than less frequent feminine noun switches. One explanation for this null effect is that masculine noun switches are easier to comprehend due to their frequency, but feminine noun switches evoke a similar processing “boost” that balances out the expected frequency effect. Feminine determiner-noun switches, unlike masculine ones, provide the comprehender with advanced information about the imminent noun. Despite their relatively low frequency, this informativeness might facilitate processing of feminine noun switches. Because it is acceptable for a Spanish masculine determiner to occur before an English noun with either a masculine or feminine Spanish translation (e.g., Valdés Kroff, Reference Valdés Kroff, Guzzardo, Mazak and Parafita2016), masculine determiners are not informative about the approaching noun in the way that feminine determiners are: feminine determiners reliably precede a feminine Spanish noun or an English noun that would be feminine in Spanish.
Eye-tracking research supports this view: Spanish monolinguals, but not Spanish–English bilinguals, use masculine determiners as a reliable predictive cue during comprehension, whereas both groups use feminine determiners as a predictive cue (Valdés Kroff et al., Reference Valdés Kroff, Dussias, Gerfen, Perrotti and Bajo2017). Thus, while masculine code-switched noun phrases are a more frequent code-switch type than feminine code-switched noun phrases, they may not be read any faster because feminine determiners, though infrequent, evoke facilitatory predictive processes. This explanation is consistent with the main idea of the PDC model – that comprehenders learn distributional patterns, and this knowledge guides real-time processing. However, simply comparing more and less frequent types of switches may mask some of the subtlety of the learning process and the type of distributional information that people track. Although we find this to be a reasonable description of what may be happening in the current study, a null result cannot be taken as evidence; future research should test how such learned patterns of predictability might offset other effects of frequency.
There are additional possible explanations for this null result. One reason for the lack of a grammatical gender effect could be that the self-paced reading paradigm is not sensitive enough to detect comprehension differences previously observed in the EEG record (Beatty-Martínez & Dussias, Reference Beatty-Martínez and Dussias2017, 2019). Yet the presence of other frequency effects on comprehension makes this explanation unlikely. Additionally, although our participants indicated moderate levels of code-switching experience, research indicates that bilingual communities exhibit a range of different code-switching practices – with some using code-switched noun phrases more than others (Beatty-Martínez & Dussias, Reference Beatty-Martínez and Dussias2019). Without directly testing the participants’ production tendencies or exposure, we cannot eliminate the possibility that their code-switching experience simply does not follow the pattern of using more frequent masculine determiners that we observed in our reference corpus (Beatty-Martínez & Dussias, Reference Beatty-Martínez and Dussias2017). However, this is unlikely to be a satisfactory explanation, as even non-habitual code-switchers show a preference for masculine determiner code-switches to some degree (Beatty-Martínez & Dussias, Reference Beatty-Martínez and Dussias2017).
Alternative interpretations of current findings
Thus far, we have assumed that the observed differences in switch costs are caused by the variable of interest: production frequency. However, other interpretations of our results remain. One possibility is that our effects are due to linguistic complexity. For instance, switches within verb phrases may elicit larger switch costs than switches within noun phrases not because they are less frequent, but because they are more morphologically complex (Myers-Scotton, Reference Myers-Scotton1993; Myers-Scotton & Jake, Reference Myers-Scotton and Jake1995, Reference Myers-Scotton, Jake and Nicol2001, 2009). Indeed, morphological complexity is central to Myers-Scotton's Matrix Language Framework, which takes the morpheme as the most important unit for where code-switches can take place (Myers-Scotton & Jake, Reference Myers-Scotton, Jake, Bullock and Toribio2009). Nouns are generally likelier junctures for code-switches to take place because they involve switching at content morphemes. Verbs often involve greater inflectional morphology, thus making verb sites less likely candidates for code-switching or candidates for creative bilingual constructions, such as verb switching where the root content morpheme remains uninflected and the inflectional morphology is carried by a light verb (e.g., Los residentes hacen cook; English gloss: the residents do[3rd person plural morpheme] cook; English translation: “The residents cook;” Balam et al., Reference Balam, Parafita Couto and Stadthagen-González2020; Chan, Reference Chan, Bullock and Toribio2009). However, linguistic complexity alone cannot explain why progressive tense switches and switches at the participle location result in smaller switch costs than perfect tense switches and switches at the auxiliary location. Progressive and perfective tense verbs are similarly complex (Giancaspro, Reference Giancaspro2015; Guzzardo Tamargo et al., Reference Guzzardo Tamargo, Valdés Kroff and Dussias2016); thus, we assert that distributional patterns in production frequency are a more consistent predictor of switch-cost magnitude.
Another possibility is that predictability of code-switches within the context of our experiment better explains our results than lifelong exposure to and learning of regular and irregular types of switches. For instance, if our switches within noun phrases were located in more consistent and predictable sites than our switches within verb phrases, this could have resulted in smaller switch costs. However, this was not the case. Noun-phrase switches could occur at any noun in critical sentences, resulting in a fairly unpredictable switch location. Often, the switch occurred at the first noun after the first verb of the sentence such as in Example (4) below, but this was variable as multiple critical noun sentences had switches at other points in the sentence, such as in (5). However, verb-phrase switches followed a much more predictable pattern, with switches always occurring in the second verb phrase of somewhat formulaic sentences as shown in (6) and (7) (following the controlled stimuli in Guzzardo Tamargo et al., Reference Guzzardo Tamargo, Valdés Kroff and Dussias2016). If our results were driven by predictability rather than production frequency, we would expect more predictable sentences such as the verb-site switches to result in smaller switch costs. Instead, our results show that more frequent noun switches are easier to process than less frequent switches within verb phrases despite the lower predictability of noun switches in our experiment.
(4) La familia buscó el neighborhood with the best neighbors. [The family searched for the neighborhood with the best neighbors.] (adapted from Johns et al., Reference Johns, Valdés Kroff and Dussias2019)
(5) Asombrosamente, el detective encontró el violín que perdió en el south of Italy. [Surprisingly, the detective found the violin that he lost in the south of Italy.] (adapted from Adler et al., Reference Adler, Valdés Kroff and Novick2020)
(6) El dueño dijo que los arquitectos have signed the documents for the construction. [The owner said that the architects have signed the documents for the construction.] (adapted from Guzzardo Tamargo et al., Reference Guzzardo Tamargo, Valdés Kroff and Dussias2016)
(7) El reportero confirmó que los senadores están requesting the funds for the project. [The reporter confirmed that the senators are requesting the funds for the project.] (adapted from Guzzardo Tamargo et al., Reference Guzzardo Tamargo, Valdés Kroff and Dussias2016)
It is also worth noting that participants in different lists may have adopted different strategies based on the single-language condition they were assigned. Some participants saw all single-language sentences and questions in English, while others saw them in Spanish. However, code-switched sentences always started in Spanish and switched to English. We made this methodological decision based on the sociolinguistic observation that code-switches are likelier to occur from Spanish to English for U.S. Spanish–English bilingual populations (Beatty-Martínez & Dussias, Reference Beatty-Martínez and Dussias2017; Blokzijl et al., Reference Blokzijl, Deuchar and Parafita Couto2017; Valdés Kroff et al., Reference Valdés Kroff, Guzzardo Tamargo and Dussias2018), which follows a more general trend for code-switches to go from a minority language into a majority language in minority-majority language settings (Parafita Couto & Gullberg, Reference Parafita Couto and Gullberg2019). Therefore, participants in an English single-language condition list would know with certainty that a switch was coming if the sentence started in Spanish, potentially allowing them to be more prepared for the switch, although they would not necessarily know where exactly the switch would occur, especially for the noun switches. On the other hand, participants in a Spanish single-language condition list may have increased their global suppression of English (most participants’ dominant language) given that the majority of input was in Spanish. This could have made switches even more difficult for participants in the Spanish single-language condition lists. If these strategies were adopted, we might expect those in the English single-language condition lists to experience smaller switch costs as they more easily predict switches and globally activate English. Against this alternative prediction, as shown in Figures 3 and 5, those in the English single-language condition actually experienced larger switch costs.
However, the larger switch costs could be due to another factor obscuring possible benefits to the participants in the English single-language condition. When calculating switch costs for only the participants in the English single-language condition, we compare reading times of code-switched English words with non-switched English words. Here, we see clear switch costs. When calculating switch costs for only the participants in the Spanish single-language condition, we compare reading times of code-switched English words with non-switched Spanish words. Here, we see no switch costs. Our participants were largely English-dominant and read much faster in English than in Spanish, as can be seen by the differences in single-language reading times in Figure 3. Thus, the apparent lack of difference between code-switched and single-language reading in the Spanish single-language condition may actually reflect a switch cost: participants were overall faster when reading in English, but they read the code-switched English region as slowly as a non-switched Spanish region. Given this reading time difference, direct comparisons of switch-cost magnitudes between these groups may not be easily interpretable. Therefore, we re-ran our models separately for each single-language condition to determine if different experimental strategies could be affecting our results. The main patterns were largely unchanged when analyzing the data in this way, indicating that the different single-language conditions did not seem to result in meaningfully different strategies adopted in the experimental setting.
Closing remarks
The current study provides evidence that the PDC model is a useful framework for explaining variation in switch costs during comprehension for a wide range of code-switch types. Overall, consistent with prior findings, switches that are more frequent in production tended to evoke less processing difficulty during comprehension. However, we expand on past work by delineating how code-switching patterns in production influence the costs of comprehending them, linking bilingual sentence processing to a broader set of effects that motivate the PDC model. Although there remain many questions about the type of conflict involved, the role of cognitive control, and the effects of individual experience on the comprehension of code-switches, this study is a step towards understanding patterns in code-switching behavior.
Additionally, although it was not our primary focus, our study provides insights into the feasibility and suitability of remote reading experiments on code-switching. Prior work has raised the issue of ecological validity of different experimental methodologies (Beatty-Martínez et al., Reference Beatty-Martínez, Valdés Kroff and Dussias2018; Valdés Kroff et al., Reference Valdés Kroff, Guzzardo Tamargo and Dussias2018) when testing the online processing of code-switches. This issue is particularly salient for Spanish–English code-switching due to its stigmatization (Zentella, Reference Zentella1997) and that it is primarily a spoken language phenomenon. Our results corroborate online sensitivities to distinct code-switch types using a remote self-paced reading paradigm, despite the ability for participants to be distracted and that they participated from disparate communities across the U.S. Our findings thus suggest that code-switching research can be expanded to remote studies, which will, we hope, lead to an increase in experimental studies focused on bilingual language practices with more diverse samples.
Characterizing the conditions under which bilinguals experience switch costs can spell out the processing demands involved in situations where multiple languages are in use and the contexts in which code-switching might be beneficial to integrate into educational environments. In the U.S., a common policy in educational practice is to separate languages (for discussion, see Lin, Reference Lin2013; Macaro, Reference Macaro, Turnbull and Dailey-O'Cain2009), citing concerns about student confusion that are not empirically supported (Antón et al., Reference Antón, Thierry and Duñabeitia2015, Reference Antón, Thierry, Goborov, Anasagasti and Duñabeitia2016; see also Byers-Heinlein & Lew-Williams, Reference Byers-Heinlein and Lew-Williams2013; Mallikarjun et al., Reference Mallikarjun, Newman and Novick2017). Here, we offer additional psycholinguistic evidence against such policies by demonstrating that frequent, naturalistic code-switches incur little to no cost for bilinguals to comprehend (see also Beatty-Martínez et al., Reference Beatty-Martínez, Guzzardo Tamargo and Dussias2021; Gosselin & Sabourin, Reference Gosselin and Sabourin2021; Johns et al., Reference Johns, Valdés Kroff and Dussias2019; Moreno et al., Reference Moreno, Federmeier and Kutas2002). This psycholinguistic perspective better aligns with educational research showing that code-switching may also promote learning and student-teacher trust (Canagarajah, Reference Canagarajah2006; Cook, Reference Cook2001; Lin, Reference Lin2013). Our data contribute to a growing understanding of code-switching not as a communicative liability, but rather as a useful communicative tool that can enhance bilinguals’ communication.
Acknowledgments
We thank the researchers who shared their materials with us or made them publicly available, the research assistants who helped with this project, and the researchers who shared our study recruitment text with their communities. This material is based upon work supported by the National Science Foundation under Grants #1449815 (LKS), #2020932 (JMN), and #2020813 (LRS).
Data availability
The data that support the findings of this study are openly available on OSF at https://osf.io/5yzna/. Note that data are only available for participants who consented to data sharing. The materials used to conduct the study are also available at the same link.
Competing interests
The author(s) declare none.