The use of mobile applications (apps) for health promotion and disease management is commonplace in the USA. As of 2016, 77 % of American adults owned smartphones( 1 ) and in 2015, 58 % of those with mobile phones reported having downloaded a health-related app (health app)( Reference Smith 2 ). In 2017, 320 000 health apps were available in the Google Play and Apple App stores( 3 ).
Nutrition-related apps (nutrition apps) are among the most frequently used type of health app. In a cross-sectional survey of US mobile phone owners conducted in 2015, 46 % of those who had downloaded a health app reported using the health app they downloaded to track their food intake( Reference Smith 2 ).
Dietitians are among those recommending the use of nutrition apps( Reference Suceda, Frederico and Pellechia 4 ). In a 2015 survey of registered dietitians in the USA, 83 % indicated that they recommend nutrition and health apps to their patients/clients, with MyFitnessPal and Fitbit being the apps most frequently recommended( Reference Suceda, Frederico and Pellechia 4 ). A three-country study that included dietitians from Australia, New Zealand and the UK found that 84 % recommended apps( Reference Chen, Lieffers and Bauman 5 ).
Numerous studies of consumer-oriented nutrition apps have been carried out to describe the content and features of available apps( Reference Schoeppe, Alley and Rebar 6 – Reference Chen, Cade and Allman-Farinelli 8 ); adherence to self-monitoring( Reference Carter, Burley and Nykjaer 9 ); and effects on dietary compliance( Reference Coughlin, Whitehead and Sheats 10 ). But less is known about the validity of nutrient intake estimates from these apps. To date, research evaluating the accuracy of nutrient intake estimates from apps has focused primarily on software developed for use in research( Reference McNutt, Zimmerman and Colaiezzi 11 , Reference Rangan, O’Connor and Giannelli 12 ). Just two studies have evaluated the accuracy of nutrient intake estimates from consumer-oriented nutrition tracking apps by comparing nutrient intake estimates from the apps with those from traditional food records as the standard( Reference Chen, Cade and Allman-Farinelli 8 , Reference Carter, Burley and Nykjaer 13 ).
Evaluating the accuracy of nutrient intake estimates from consumer-oriented nutrition tracking apps is important because many in the public may be relying on these apps to gauge the extent to which they are meeting nutrition targets (e.g. limiting energy intake for weight loss, controlling Na intake for blood pressure control) in the absence of dietetic professional guidance. In addition, accuracy is not assured due to minimal governmental oversight of these types of apps. In 2015 the Food and Drug Administration issued guidance to the industry and its administrative staff, stating the need to regulate some mobile medical apps as medical devices under the Food Drug & Cosmetic Act and exercising enforcement discretion to other mobile health apps( 14 ), such as nutrition tracking apps for health promotion.
To provide additional data regarding the accuracy of nutrient intake estimates from consumer-oriented nutrition tracking apps in the marketplace, we carried out a small-scale evaluation of five leading apps. The evaluation focused on assessing the quality of the food and nutrient database and associated nutrient calculation algorithms supporting each app. Descriptive information about each of the apps (e.g. nutrients available in the app, sources of nutrition composition data for foods in the app, etc.) was also gathered and is reported in the current communication.
Methods
Overview
Nutrient intake estimates from interviewer-administered 24 h dietary recalls collected using the Nutrition Data System for Research (NDSR) 2014 were compared with intake calculations from these recalls entered by the researcher into five free nutrition tracking apps. The apps chosen were MyFitnessPal, Fitbit, Lose It!, MyPlate and Lifesum. A set of coding rules was followed to ensure similar entry procedures were followed for each app. Apps were selected from the Apple App Store based on consumer popularity from the list of free ‘Health and Fitness’ apps classified as a nutrition tracking app for iPhones.
Twenty-four-hour dietary recall data
The 24 h dietary recall data collected from adults who participated in another study (Grocery Assistance Program Study (GAPS)) were used for the present study( Reference Harnack, Oakes and Elbel 15 ). Participants in GAPS were primarily female (81 %) and ethnically diverse (29 % White, 52 % African American, 10 % Hispanic). Most were overweight or obese (82 %) and lower income (household income less than or equal to 200 % of the federal poverty level). All were from the Minneapolis-St. Paul, Minnesota metropolitan area.
Thirty GAPS participants were chosen through random selection. For each of these participants, the first 24 h dietary recall collected from them in GAPS was selected for use in the present study. In GAPS the dietary recalls were collected over the telephone using NDSR. NDSR provides a detailed report about each food recorded (e.g. full food name, food amount, etc.) in a 24 h dietary recall. This report was used as the basis for manually entering food intake information into each of the popular nutrition tracking apps evaluated in the present study.
Nutrient intake estimates from NDSR for each dietary recall were used as the criterion (reference) measure in the present study. NDSR is a dietary analysis software application developed by the University of Minnesota Nutrition Coordinating Center for use in research( Reference Dennis, Ernst and Hjortland 16 ). A set of well-established procedures was followed in assembling and maintaining the food and nutrient database that supports this program( Reference Schakel, Sievert and Buzzard 17 – Reference Buzzard and Feskanich 20 ). In brief, food and nutrient composition information available from the US Department of Agriculture’s (USDA) National Nutrient Database for Standard Reference (SR) is the primary source of information relied upon in assigning the nutrient values to foods in the NDSR database. Because values for some nutrients and food components are not available in the USDA SR and information is lacking for many brand name food products, additional resources are utilized. These resources include nutrient composition values published in scientific journal articles in which appropriate analytic techniques were used, information provided by food manufacturers and international food composition tables. A set of imputation procedures is also utilized to minimize missing/incomplete nutrient composition data for foods.
Selection of nutrition tracking apps
The top five nutrition tracking apps available for free download in the Apple store were accessed in October 2016. Apps were selected based on consumer popularity from the list of free ‘Health and Fitness’ apps classified as nutrition tracking apps for iPhones. For the present study’s purposes, a nutrition tracking app is defined as an application that tracks and provides a summary of at least energy and macronutrients (total fat, carbohydrate and protein) and is not tailored to any specific disease (e.g. carbohydrate counter for diabetics) aside from obesity (apps oriented towards supporting weight loss were included). Popularity in selecting the top five apps was determined by the number of times the Apple store indicated the app had been downloaded.
Apps were selected in the Apple store only, as an informal comparison of apps available in the Apple store and the Google Play store for android phones and tablets indicated substantial overlap in popular apps between stores. Only free apps on iPhones were included in the five most popular apps, as there are generally more apps available for iPhones compared with iPads.
Data entry
Food items in each participant’s 24 h dietary recall NDSR food report were entered into each of the five apps by the first author, who has a background in dietetics and nutrition. Food items on the food reports were selected and entered into the apps based on a set of coding rules created to maximize consistency in data entry across dietary recalls and apps. These codes outlined procedures to take if a match could not be found for reasons such as discrepancies in portion size and/or description of a food item. After all the food items from a participant’s food report were entered into an app, a nutrient intake report was generated and nutrient intake values in the report were entered into a Microsoft® Excel 2016 spreadsheet.
To assess how well each food selected in the apps matched the original food item in the NDSR food report, food items were classified as: (i) a close match; (ii) a partial match due to poorly matched food amount; (iii) a partial match due to a poorly matched food description; or (iv) a poor match. A food item was classified as a close match if the food item selected and the amount entered in the app matched both the food description and the amount consumed in the NDSR food report (e.g. ½ cup of 2 % milk in NDSR food report could be entered as ½ cup or equivalent conversion of 2 % milk in the app). Food items were classified as a partial match due to poorly matched food amount if the food selected in the app had a description that closely matched the food description in NDSR, but the amount consumed as entered in NDSR could not be entered in the app (e.g. 2 % milk option available in the app, but portion could not be entered in the amount of ½ cup or equivalent conversion). Food items were classified as a partial match due to a poor food description if the food description match was poor, but the amount consumed as entered in NDSR could be entered in the app (e.g. could not find 2 % milk selection but could enter amount of ½ cup or equivalent conversion). Lastly, food items were classified as a poor match if the food description match was poor and the amount consumed as entered in NDSR could not be entered into the app (e.g. could not find a 2 % milk selection and portion could not be entered in the amount of ½ cup or equivalent conversion).
Analysis
Two statistical analysis methods were used to assess the accuracy of nutrient intake estimates from each app: (i) Pearson correlation coefficients were calculated to compare the linear association between total daily nutrient intake estimates from each app and NDSR and each of the other apps; and (ii) paired two-tailed t tests were run to compare mean total daily nutrient intake estimates from each app with mean total daily nutrient intake estimates from NDSR. For each app, the percentages of foods classified as a ‘close match’, ‘partial match due to poorly matched food amount’, ‘partial match due to poorly matched food description’ and ‘poor match’ were calculated.
Results
Descriptive information obtained through observation of the apps, site FAQs and emails with customer support is provided in Table 1. To summarize, the apps provided intake estimates for between four and twelve nutrients. Two of the apps (Fitbit and Lifesum) offered a greater number of nutrients for the upgraded (paid) versions of the apps. The most common sources of nutrient composition data for foods in the apps were the USDA SR, food manufacturers and restaurants. In four of the apps (Lose It!, MyPlate, Lifesum and MyFitnessPal), members can upload food entries and their nutrient composition information to become available to all app users. All four of these apps utilize an icon to indicate if an item is ‘site verified’. The icon for Lose It!, MyPlate and Lifesum represents accurate nutrient information, defined as being from their nutrient data sources or cross-checked with the USDA SR or food manufacturer/restaurant sources. The verification icon for MyFitnessPal indicates that a food listing in its database has complete nutrition information (energy and macronutrients) and may be subject to nutrient inaccuracies.
Table 1 Descriptive information on the Nutrition Data System for Research (NDSR) and the nutrition tracking apps evaluated in the present study
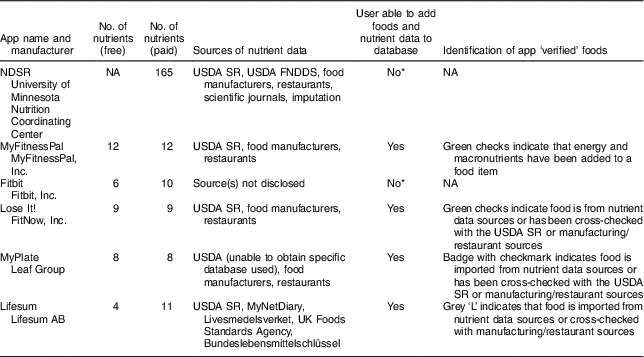
USDA SR, US Department of Agriculture National Nutrient Database for Standard Reference; USDA FNDDS, US Department of Agriculture Food and Nutrient Database for Dietary Studies; NA, not applicable.
* Not able to add foods and nutrients to database accessible to all users of the application.
Correlations between nutrient intake estimates from NDSR and the nutrition tracking apps ranged from 0·73 to 0·96 for energy and macronutrients (total carbohydrate, protein and fat). The correlations for the other nutrients examined (Na, total sugars, fibre, cholesterol and saturated fat) ranged from 0·57 to 0·93 (Table 2). The range in correlation coefficients between nutrient intake estimates from NDSR and each app was as follows: MyFitnessPal, r=0·71–0·93; Fitbit, r=0·77–0·94; Lose It!, r=0·57–0·89; MyPlate, r=0·70–0·93; Lifesum, r=0·88–0·96.
Table 2 Pearson correlationsFootnote * between total daily nutrient intake estimates from nutrition tracking apps and the Nutrition Data System for Research (NDSR; n 30 dietary recalls)
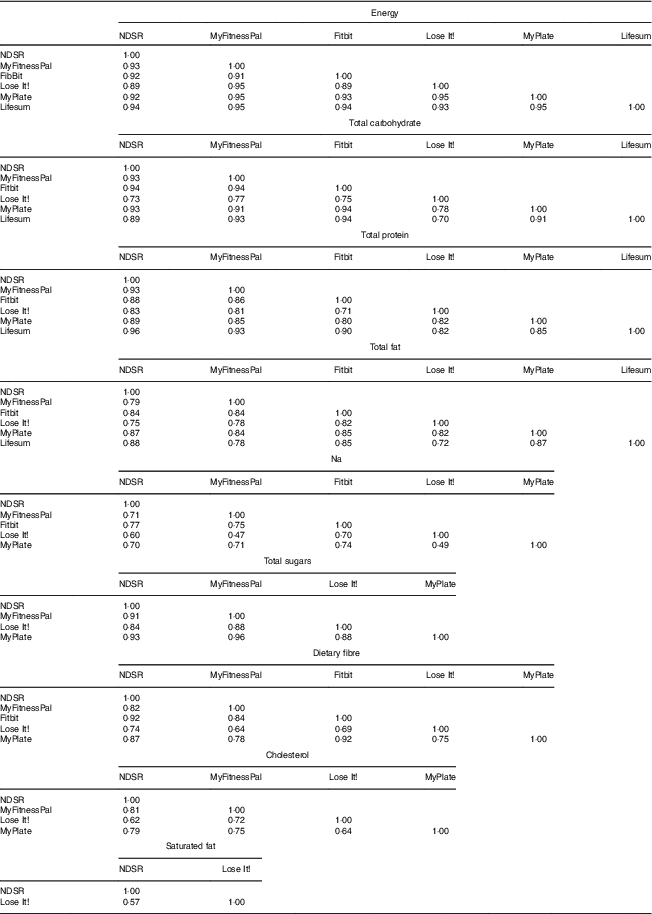
* All correlations were statistically significant (P<0·05).
Mean differences between nutrient calculations for NDSR and the nutrition tracking apps are shown in Table 3. For each app, one or more mean nutrient intake calculations were significantly lower than those from NDSR, with underestimation ranging from 7 to 41 %. These differences included total protein (P=0·03), total fat (P=0·005), Na (P=0·02) and cholesterol (P=0·005) for MyFitnessPal; dietary fibre (P=0·04) for Fitbit; total protein (P=0·0004), total fat (P=0·008), Na (P=0·002), sugars (P=0·007), cholesterol (P=0·0006) and saturated fat (P=0·005) for Lose It!; Na (P=0·03) and dietary fibre (P=0·005) for MyPlate; and total fat (P=0·03) for Lifesum.
Table 3 Mean total daily nutrient intakes estimates from the Nutrition Data System for Research (NDSR) and each nutrition tracking app (n 30 dietary recalls)

NA, not available.
P values from two-tailed paired t tests comparing nutrient intake estimates with those from NDSR; significant P values indicated in bold.
* To convert to kJ, multiply kcal values by 4·184.
With respect to the extent to which foods and food amounts entered into each app matched those in the NDSR foods reports, most foods were classified as close matches (78–83 %) for each app. MyFitnessPal had the highest percentage of close matches (83 %) and MyPlate had the lowest (78 %). For all apps, mismatches due to poorly matched food amount (13–17 %) occurred more frequently than mismatches due to poorly matched food description (4–6 %). Poor matches (neither food amount or food description matched) were infrequent, with MyFitnessPal having no identified poor matches and Lifesum having the highest percentage of occurrences at 1 %.
Discussion
Results from the present evaluation of popular nutrition apps indicate: (i) a pattern of underestimation of nutrient intake by the apps for most of the nutrients examined; (ii) the comparative validity of calculations for energy and macronutrients to NDSR (r=0·73–0·96) may be somewhat better than estimates for other nutrients and food components (r=0·57–0·93); and (iii) some apps may have better accuracy than others.
The pattern of underestimation of nutrient intake observed is concerning because it has the potential to magnify the well-documented phenomenon of underestimation of food and nutrient intakes with self-report methods of dietary assessment such as dietary recalls and food records( Reference Willett 21 ). One possible reason for this observation is the lack of collection of food preparation detail in the apps. In NDSR, detailed information is collected on how foods are prepared (e.g. type of fat used in preparing a fried egg, whether salt was added in preparation, etc.). In contrast, in the apps, food items often lack this level of detail. Incomplete (missing) nutrient composition information in apps is another possible explanation for the apparent systematic underestimation and the lower correlations observed for some nutrients. When app users add foods and nutrient information into the apps’ food and nutrient database, they are not required to enter each nutrient that the app reports on. Thus, there may be missing values for some nutrients for foods added by app users. In addition, it is possible that some nutrient composition values are missing for some foods in the food and nutrient database assembled for the app.
Results from the present study are mostly consistent with findings from previous studies that have evaluated the comparability of nutrient intake estimates from consumer-oriented nutrition tracking apps to traditional food records( Reference Chen, Cade and Allman-Farinelli 8 , Reference Carter, Burley and Nykjaer 13 ). In 2014 Chen et al. evaluated the most popular smartphone apps for weight loss available on Google Play and iTunes App Store in Australia and found average energy intake estimates across the apps (n 23) to be similar to energy intake estimates from weighed food records for the same three days (the researchers entered foods and amounts in the weighed records into each app and a nutritional analysis software package designed for use in Australia)( Reference Chen, Cade and Allman-Farinelli 8 ). The mean absolute difference in energy intake estimates between the apps and the weighed food records was just 127 (95 % CI −45, 299) kJ/d. But there was a wide range in the level of concordance between energy intake estimates from specific apps and weighed food records (range in energy differences was 1001 to −700 kJ/d). Energy was the only nutrient evaluated in their study. Carter et al. evaluated the accuracy of energy and macronutrient intake estimates from an app designed to support weight loss (My Meal Mate)( Reference Carter, Burley and Nykjaer 13 ). Study participants (n 50) were instructed to complete seven consecutive days of food tracking using the My Meal Mate app. During the 7 d recording period, two unscheduled interviewer-administered 24 h dietary recalls were collected over the telephone as a reference measure. The correlation between energy and macronutrient intake estimates from the My Meal Mate app and interviewer-administered dietary recalls was moderate to high (r=0·63–0·83). Mean energy and macronutrient intake estimates from the My Meal Mate app tended to be lower than estimates from interviewer-administered dietary recalls, but these differences were generally not statistically significant. Findings suggested that underestimation of energy and macronutrient intake estimates with the app may be more problematic with increasing days of use of the app for diet tracking.
With respect to the extent to which foods consumed are included in apps and may be entered in an appropriate amount, in the present study a close-matching food description or food amount could not be entered for 17–22 % of foods entered into each app. This finding is consistent with a study conducted by Darby et al., which included an assessment of the quality of food databases in diabetes apps with a diet tracking function( Reference Darby, Strum and Holmes 7 ). In that study each app’s food database was ranked on a 3-point scale (excellent, acceptable, poor) based on the extent to which nine food items were included in the app. Out of the forty-two apps assessed, thirteen were ranked as poor (31 %), meaning that less than six of the nine food items could be found in the app. These findings in combination with findings from the present study suggest that those using consumer-oriented diet tracking apps may face challenges in entering foods consumed, which could lead to inaccurate nutrient intake estimates.
One strength of the present study is that it is among the first to evaluate the accuracy of nutrient calculations of consumer-oriented commercial nutrition tracking apps and the first to evaluate nutrients beyond energy and macronutrients. Another study strength is that of the apps evaluated: four out of the five were in the top 100 grossing apps in the ‘Health and Fitness’ category in the Apple App Store, indicating that the present study results may have implications for a sizeable proportion of nutrition tracking app users.
The most notable limitation of the present study was that it analysed only the top five free nutrition tracking apps, thus the generalizability of findings to less popular apps may be limited. Also, the study did not evaluate the level of accuracy that may result if an individual entered her/his own foods and food amounts. Rather, a trained professional searched for and entered foods in the apps in the current study. Factors such as a poor user interface, technology literacy and cultural competency of the apps are among the factors that could interfere with an individual’s ability to accurately enter the foods and food amounts consumed( Reference Illner, Freisling and Boeing 22 ).
Conclusion
Study findings, taken together with results from previous studies( Reference Darby, Strum and Holmes 7 , Reference Chen, Cade and Allman-Farinelli 8 , Reference Carter, Burley and Nykjaer 13 ), suggest that dietitians and other health professionals should be cautious in recommending use of consumer-oriented nutrition tracking apps. Some apps may provide more accurate nutrient intake estimates than others, hence this should be considered when deciding whether to recommend an app and which app(s) to recommend. For consumers wishing to use a nutrition tracking app, dietetic professionals’ guidance should be sought in choosing an app.
App developers should consider developing and implementing testing plans to identify issues with their app that require resolution to improve the accuracy of nutrient intake estimates. Dietitians with joint expertise in dietary assessment and food and nutrient databases may be useful to app development teams as they work to develop and implement testing and product improvement plans.
Government agencies responsible for overseeing consumer-oriented nutrition tracking apps should regularly review available research on the integrity of these apps, as well as consider supporting or carrying out studies that are needed to inform regulatory decisions related to these types of health apps.
Study findings contribute to a sparse literature on the accuracy of nutrient intake estimates from nutrition tracking apps. Further research is needed, including replicating the present study with a larger number of dietary recalls or food records and a wider variety of nutrition tracking apps. Also, validation studies in which consumers are asked to log their food intake using the apps would provide a more complete assessment of the accuracy of food and nutrient intake estimates from consumer-oriented nutrition tracking apps.
Acknowledgements
Financial support: This study received no financial assistance from any funding agency in the public, commercial or not-for-profit sectors. Conflict of interest: None. Authorship: C.G. was a graduate student at the University of Minnesota Twin Cities when the study was conducted. L.H. and C.G. collaborated on the design of the study and formulated the research question. C.G. carried out the study, conducted the statistical analysis and drafted the initial paper. L.H. checked the data, assisted in the interpretation of the results, revised the manuscript and assisted in the submission process. M.A.P. revised the article critically and assisted in the submission process. Ethics of human subject participation: As this was a secondary data analysis with no human subjects, no ethical approval was necessary.