Recent molecular genetic genome-wide association studies have made a major contribution to understanding the biological contributions to many common human diseases including diabetes, heart disease, Crohn's disease and rheumatoid arthritis. Reference Mathew1–Reference McCarthy, Abecasis, Cardon, Goldstein, Little and Ioannidis4 These approaches provide psychiatry with powerful research tools that offer the opportunity to identify the biological systems involved in the pathogenesis of major psychiatric illness. Reference Craddock, O'Donovan and Owen5 However, psychiatric phenotypes are currently defined according to sets of descriptive criteria that were developed to have acceptable reliability but with no expectation that the resulting categories would represent valid entities. This uncertainty over the validity of the diagnostic categories (phenotypes) that we use in psychiatry makes psychiatric research more challenging than for most non-psychiatric disorders Reference Angst6,Reference Craddock and Owen7 and, thereby, has an adverse impact on the evidence available on which to base clinical practice. In this paper we use genome-wide genetic association data on people with bipolar disorder (and controls) to explore the relative genetic support (a form of biological validity) for different descriptive operational diagnostic categories within a published, large UK case–control bipolar disorder study of 4806 individuals genotyped for 469 557 genetic polymorphisms that met basic quality control metrics (Wellcome Trust Case Control Consortium (WTCCC)). 3
Method
Samples
Our sample comprised the 1868 participants with bipolar disorder and 2938 controls investigated in the WTCCC study. A detailed description of the sample has been provided elsewhere. 3 All individuals were from the UK and over the age of 16 years. Clinical assessment included semi-structured interview and review of case notes. Ratings of symptom occurrence and course of illness were made including the operational criteria (OPCRIT) item checklist. Reference McGuffin, Farmer and Harvey8,Reference Craddock, Asherson, Owen, Williams, McGuffin and Farmer9 Diagnoses were based on all available data. The primary diagnostic system used for classifying participants was the Research Diagnostic Criteria (RDC) Reference Spitzer, Endicott and Robins10 because it provides more delineation between individuals on the basis of the pattern of mood and psychotic symptomatology than do DSM–IV 11 or ICD–10. 12 Participants with bipolar disorder had experienced at least one episode of clinically significant elevated mood according to RDC: bipolar I disorder (n = 1316), schizoaffective disorder, bipolar type (n = 279), bipolar II disorder (n = 171) and manic disorder (n = 102). The diagnoses according to DSM–IV for the same set of participants were: bipolar I disorder (n = 1594), schizoaffective disorder, bipolar type (n = 98), bipolar II disorder (n = 134), bipolar disorder, not otherwise specified (n = 42). (Each individual has a diagnosis according to both RDC and DSM–IV. The joint distribution of diagnoses is shown in online Table DS1. Diagnostic reliability as measured by mean kappa (κ) coefficients for a set of 20 individuals was 0.86 for RDC and 0.84 for DSM–IV.) With the exception of DSM–IV bipolar disorder, not otherwise specified (n = 42), all definitions of diagnosis were of large enough sample size to warrant further investigation. The controls, who were not screened for psychiatric illness, came from two sources: the UK 1958 birth cohort longitudinal epidemiological sample (n = 1458) and the UK Blood Donor Service (n = 1480). It has previously been shown that it is valid to combine these two control samples for use as controls in genetic association studies using UK disease samples, including the current bipolar disorder sample. 3
Genotypic data
Polymorphisms used in analyses
The WTCCC data-set comprised 469 557 single nucleotide polymorphisms (SNPs) distributed across the genome. For the current analysis we selected autosomal SNPs for analysis that had a minor allele frequency of at least 5% in our total sample and met stringent levels of genotyping quality. The large number of genotypes scored in a study such as this requires the use of generic approaches to quality control, allowing SNPs to be excluded where the quality of genotyping is in question. We used the following quality filter for inclusion of SNPs:
-
(a) call rate (i.e. the proportion of genotypes that could be scored confidently, out of all the genotypes that were attempted for that SNP) >99.5% in WTCCC participants with bipolar disorder and in controls;
-
(b) Hardy-Weinberg P>0.001 in participants with bipolar disorder (i.e. to exclude significant deviation from Hardy–Weinberg equilibrium at significance P<0.001);
-
(c) Hardy–Weinberg P>0.01 in controls.
We have demonstrated that SNPs meeting these criteria showed a very high level of genotype agreement with genotypes scored independently in our laboratory using the Sequenom platform (of over 67 000 genotypes typed for 140 SNPs we found 99.95% agreement; data not shown). Using these stringent quality filters there were autosomal 276 122 SNPs selected for analysis.
Statistical analyses
Principle of the analysis
In this analysis we were interested in whether any particular diagnostic phenotype definition(s) provided strong association evidence when a set of participants with bipolar disorder meeting this definition was analysed against controls for the full set of SNPs within the genome-wide analysis. We used a genome-wide analysis (i.e. a case–control comparison for each of the 276 122 SNPs) as the basic unit of study and summarised the overall association evidence in that analysis by counting the number of independent SNPs that showed association exceeding a specified significance threshold (chosen as P<10–5, the significance benchmark in the WTCCC study used to designate at least ‘moderately strong’ evidence for association). We refer to these associated SNPs as ‘hits’ (which is a shorthand term used in molecular genetics to indicate an independent association signal that meets a specified level of statistical significance). Our basic aim was to determine if one or more definitions of diagnosis possessed greater utility by virtue of identifying more hits. When comparing the number of hits from different diagnostic phenotype definitions there is an important complicating factor – the case sample size varies for the different diagnostic sets. The sample size affects the power to detect associations and must, therefore, be taken into account. Having provided this orientation towards our analysis, we will now explain the details of the methods used.
Genome-wide association analysis
Each set of participants with bipolar disorder was compared against the set of 2938 controls for all of the 276 122 SNPs that met our stringent quality control filter (see above). According to the various diagnostic definitions, the number of cases varied from 102 to 1868. For each SNP we employed the Cochran–Armitage trend test of genotype distributions to test association with disease (i.e. we compared the group with bipolar disorder with the 2938 controls). Assuming no association with disease, the trend test statistic follows the χ2 distribution on 1 degree of freedom (as implemented within the PLINK analytic suite of programs). Reference Purcell, Neale, Todd-Brown, Thomas, Ferreira and Bender13 This method is a standard approach to analysis of genetic association data and is robust to departures of the data from Hardy–Weinberg equilibrium. To allow for any systematic inflation of the test statistics we adjusted the trend test statistic of each SNP by λ, where λ is the genomic control inflation factor, estimated to be the median of all 276 122 test statistics divided by 0.456. Reference Devlin and Roeder14 Because SNPs that are near to each other can show correlated association signals (due to linkage disequilibrium) we filtered that set of association signals from each analysis to remove non-independent SNPs using the clumping facility within PLINK. Reference Purcell, Neale, Todd-Brown, Thomas, Ferreira and Bender13 (The non-independent SNPs lie within 250 kb and are in linkage disequilibrium at r 2>0.2 with the index SNP.) For each such genome-wide analysis we used the number of (λ-corrected) independent SNPs showing an association signal at P<10–5 (number of ‘hits’) as a summary measure of the genome-wide analysis.
Testing significance of observed number of hits
We formulated the null hypothesis that, for a subset of participants with bipolar disorder v. controls, the number of hits we observed follows the distribution expected by chance. This hypothesis assumes that the bipolar disorder sample is genetically homogeneous (i.e. minimal variation between individuals), and that the subset of participants with bipolar disorder under investigation is a truly random selection from the full bipolar disorder sample of 1868 individuals. It is important to note that the null hypothesis here is that the genetic effects within the sample with bipolar disorder are homogeneous in the sense that they do not vary according to diagnostic subset. It is not an assumption that there are no genetic effects (i.e. we do not assume that there are no differences between those with bipolar disorder and controls). The one-sided alternative is that we observed more hits than would be expected by chance, i.e. the total sample with bipolar disorder is genetically heterogeneous. Under the alternative hypothesis, the subset under investigation is postulated to have properties that facilitate the detection of genetic effects. Such properties include a higher genetic loading or, perhaps more plausibly since bipolar disorder broadly defined has already a high heritability, a greater degree of genetic homogeneity for the subset. Although it may seem counter-intuitive that more homogeneity (i.e. fewer risk genes) would lead to the detection of greater numbers of risk genes, such a scenario is entirely expected. This is because the reduction in the number of risk loci in the subset compared with that in bipolar disorder as a whole would effectively increase the effect size at any one locus and thus the ease of detection.
In order to allow for the effect of differing sample size we used a simulation procedure to generate the distribution of the number of hits for subsets of the sample with bipolar disorder v. controls under the null hypothesis. We randomly selected samples of individuals with bipolar disorder, where the number of individuals is the same size as the diagnostic set of interest, and used each in a case–control genome-wide analysis against the total set of controls. For each of the subsets we undertook a genome-wide analysis as described above (including both genomic control adjustment and filtering for independent signals) and counted the number of SNPs that exceeded the threshold of significance (P<10–5). This whole procedure was repeated 1000 times to produce a distribution of the number of SNPs expected by chance when testing individuals with bipolar disorder v. controls.
Determining empirical significance levels
The simulation procedure allowed us to compare the observed number of hits with the simulated distribution to determine whether there was evidence to accept or reject the null hypothesis at the 5% significance level. The empirical P was given by the proportion of times that the simulated sets achieved at least as many hits as were observed in the test set.
Results
Genome-wide analyses of diagnostic subsets
We undertook genome-wide association analyses for seven (non-independent) diagnostic subsets of the 1868 individuals with bipolar disorder v. controls. The number of hits at P<10–5 is shown in Table 1. It can be seen that the largest number of hits occurred with the RDC schizoaffective disorder, bipolar type set even though it was one of the smaller sets tested (n = 279 individuals).
Table 1 The number of independent single nucleotide polymorphisms (SNPs) that exceeded a significance threshold of P<10–5 when the diagnostic subset is compared with the controls (corrected for genomic control, λ)

Diagnostic subset | Genomic control, λ | Observed hits, n | Empirical significance,a (P) |
---|---|---|---|
Total bipolar disorder set (n = 1868) | 1.116 | 4 | N/A |
DSM—IV bipolar I disorder (n = 1594) | 1.100 | 5 | 0.10 |
DSM—IV bipolar II disorder (n = 134) | 1.002 | 4 | 0.27 |
DSM—IV schizoaffective disorder, bipolar type (n = 98) | 1.013 | 5 | 0.22 |
RDC bipolar I disorder (n = 1316) | 1.086 | 0 | 1.00 |
RDC bipolar II disorder (n = 171) | 1.000 | 3 | 0.47 |
RDC schizoaffective disorder, bipolar type (n = 279) | 1.061 | 9 | 0.0004 |
RDC manic disorder (n = 102) | 1.025 | 5 | 0.20 |
Empirical significance levels for diagnostic sets
The observed number of hits in the RDC schizoaffective disorder, bipolar type set was achieved only once in any of the 2500 simulations of 279 participants randomly selected from the bipolar disorder data-set and compared with the controls. This corresponds to an empirical significance of P = 0.0004 for the null hypothesis that the RDC schizoaffective disorder, bipolar type set does not differ genetically from the bipolar disorder sample as a whole. A conservative Bonferroni correction for multiple testing of seven subsets gives an empirical significance of P = 0.0028. None of the other diagnostic sets differed from chance expectation (P>0.05).
Genome-wide analysis in the RDC schizoaffective disorder, bipolar type group v. controls
As our analysis provided evidence that the RDC schizoaffective disorder, bipolar type diagnostic criteria identified a bipolar phenotype with a particularly strong utility for genetic studies, which can be considered a form of genetic validity, we present the results of the genome-wide analysis for the RDC schizoaffective disorder, bipolar type diagnostic set. For the strongest hits in our observed data, we visually inspected genotype clusterplots as a further check of genotyping quality. Independently associated SNPs that exceed a significance threshold of P<10–5 (all of which have good quality clusterplots) are shown in Table 2. For each independent signal, the table also shows the number of nearby SNPs that are in linkage disequilibrium (i.e. closely correlated) with the index SNP and thereby provide quality control criteria to check for any additional strongly supported association signals. This revealed one additional independent signal (rs4786811 on chromosome 16p13.3) at a significance threshold of P<10–5 that hadbothanacceptable clusterplotand also support from a closely correlated SNP. The clusterplots for these SNPs can be found in the online supplement, as can a list of all SNPs (online Table DS2) showing nominally significant association (P<0.05).
Table 2 Independent association signals at P<10–5 for comparison of participants in the Research Diagnostic Criteria (RDC) schizoaffective disorder, bipolar type group against controlsa
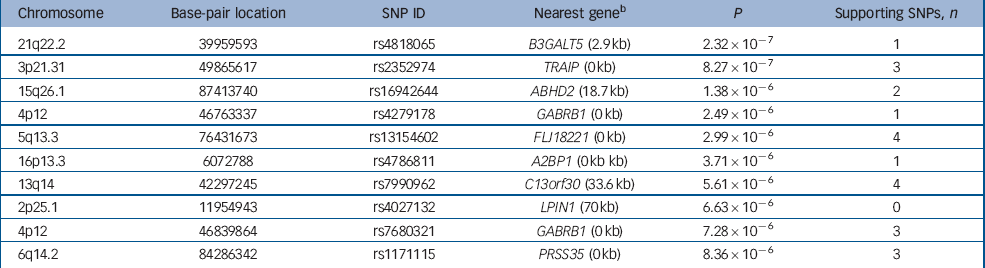
Chromosome | Base-pair location | SNP ID | Nearest geneb | P | Supporting SNPs, n |
---|---|---|---|---|---|
21q22.2 | 39959593 | rs4818065 | B3GALT5 (2.9 kb) | 2.32 × 10-7 | 1 |
3p21.31 | 49865617 | rs2352974 | TRAIP (0 kb) | 8.27 × 10-7 | 3 |
15q26.1 | 87413740 | rs16942644 | ABHD2 (18.7 kb) | 1.38 × 10-6 | 2 |
4p12 | 46763337 | rs4279178 | GABRB1 (0 kb) | 2.49 × 10-6 | 1 |
5q13.3 | 76431673 | rs13154602 | FLJ18221 (0 kb) | 2.99 × 10-6 | 4 |
16p13.3 | 6072788 | rs4786811 | A2BP1 (0 kb kb) | 3.71 × 10-6 | 1 |
13q14 | 42297245 | rs7990962 | C13orf30 (33.6 kb) | 5.61 × 10-6 | 4 |
2p25.1 | 11954943 | rs4027132 | LPIN1 (70 kb) | 6.63 × 10-6 | 0 |
4p12 | 46839864 | rs7680321 | GABRB1 (0 kb) | 7.28 × 10-6 | 3 |
6q14.2 | 84286342 | rs1171115 | PRSS35 (0 kb) | 8.36 × 10-6 | 3 |
Discussion
The aim of our analysis was to use the WTCCC bipolar disorder genome-wide association data-set to determine whether any of the bipolar-spectrum diagnostic subsets within the sample provided enhanced genetic utility, compared with the bipolar disorder sample as a whole and compared with the other diagnostic subsets. Of the four RDC and three DSM–IV diagnostic categories examined, only the RDC schizoaffective disorder, bipolar type category showed a significantly enhanced number of association signals above expectation based on the null hypothesis that all the diagnostic subsets were simply a random selection from the bipolar disorder sample as a whole, i.e. that the genetic contributions do not vary according to the diagnostic subset. It is important to stress that this null hypothesis allows for there being genetic differences between participants with bipolar disorder and controls – it is not a null hypothesis that there are no genetic effects operating in bipolar disorder. Thus, our analysis provides evidence that, within our data-set and at the association threshold considered (i.e. λ-corrected P<10–5), the RDC schizoaffective disorder, bipolar type sample is a particularly valuable phenotype for genetic studies. Indeed, the RDC schizoaffective disorder, bipolar type sample had more hits than the sum of the hits in the other three RDC diagnostic sets (P = 0.022).
It has often been argued that schizoaffective disorder may be closely related to schizophrenia – indeed, DSM–IV classifies schizoaffective disorder within diagnostic code category 295, as a subtype of schizophrenia. Thus, one important question is the following: is the enhanced number of hits seen in the RDC schizoaffective disorder, bipolar type group in our bipolar disorder data-set relatively specific to the RDC schizoaffective disorder, bipolar type group, or is it a general property of individuals with schizophrenia-like features (in which case it could be more usefully thought of as providing support for the genetic utility of schizophrenia). We have recently undertaken a genome-wide association study of schizophrenia using the same methodology as that used in the WTCCC study of bipolar disorder (same genotyping platform, same laboratory, same set of SNPs called at the same time using the same algorithm and with case–control comparisons made using the same set of 2938 controls). Reference O'Donovan, Craddock, Norton, Norton, Williams and Peirce15 When we apply our analytic approach to our set of people with ‘schizophrenia spectrum’ (i.e. schizophrenia and schizoaffective disorders – the latter set includes the 279 individuals from the bipolar disorder sample) we similarly observe that RDC schizoaffective disorder, bipolar type stands out in having more hits (RDC diagnoses: schizoaffective disorder, bipolar type (n = 299), hits 7, P = 0.023; schizoaffective disorder, depressed type (n = 114), hits 2, P = 0.81; schizophrenia (n = 257), hits 3, P = 0.53). Thus, within our data, and at the significance threshold considered, the participants with RDC schizoaffective disorder, bipolar type stand out from both the other participants with bipolar disorder and the schizophrenia groups.
We note that classical family, twin and adoption genetic studies have long been used as one of the key methods for validating psychiatric diagnoses. Reference Robins and Guze16 Classical approaches require twin and family samples, the availability of which is limited by the substantial difficulties and high costs inherent in their recruitment. Classical genetic studies estimate the contribution of all inherited forms of variation. Here we have used available genome-wide association study data to estimate the relative contribution of the common genetic variation to susceptibility to different phenotypes. This may be a useful approach for delineating phenotypic sets that could be particularly fruitful for study using genome-wide association studies. The major limitation of using genome-wide association study data is that current genome-wide association study genotype data-sets do not capture all genetic variation that may be relevant to illness. Rather, they provide information about a substantial proportion of common genetic variation within the genome. They do not currently provide information about the contribution of rare variants or mechanisms such as structural variation.
The choice of threshold used for counting the top hits is inevitably, to some extent, arbitrary. In general, the signal to noise ratio is expected to be higher for more significant P-value thresholds but there are progressively fewer hits that achieve more stringent thresholds. Thus, there is a trade off in choosing a threshold that is reasonably stringent but allowing a reasonable number of hits. A threshold of P<10–5 was a benchmark threshold used for reporting signals within the WTCCC study and we adopted this as an appropriate compromise. However, we note that our findings remain unchanged with a higher (P<5×10–6) or lower (P<5×10–5) threshold (data not shown). At these thresholds, RDC schizoaffective disorder, bipolar type again stood out as the only diagnostic subset having a significantly increased number of hits, although as would be expected, the actual number of hits was less or more respectively.
The number of hits at a certain threshold gives a guide to the ease of detection of genes contributing to the phenotype. The significance level of the individual association signals is also important. Broadly, the first issue relates to the number of loci of given effect size, whereas the second relates to whether some loci have particularly large effect sizes. We note that the strongest signal in the RDC schizoaffective disorder, bipolar type subset of participants was more significant than any hit in either the bipolar disorder set as a whole or the other subsets considered, which further supports the genetic utility of such cases.
Our findings, thus, suggest that the RDC schizoaffective disorder, bipolar type set of cases is likely to be particularly fruitful for genetic investigations aimed at identifying common polymorphisms that influence risk (i.e. the type of genetic variants that genome-wide association studies are designed to detect). If our findings generalise to other samples, it would suggest that careful phenotypic selection of participants could enhance power to identify genes conferring susceptibility to illness. At present there is a great deal of effort being invested in rapidly assembling the large samples of individuals with psychiatric illness that are expected to be necessary to provide power to detect susceptibility genes. The current findings suggest that it will also be important to pay sufficient attention to the phenotype so that genetically relevant distinctions can be made.
It is of substantial interest that the existence of one or more relatively discrete nosological entities with mixed mood–schizophrenia features is supported by latent class analyses (e.g. Kendler et al, Reference Kendler, Karkowski-Shuman, O'Neill, Straub, MacLean and Walsh17,Reference Kendler, Karkowski and Walsh18 McGrath et al, Reference McGrath, Nestadt, Liang, Lasseter, Wolyniec and Fallin19 Sham et al Reference Sham, Castle, Wessely, Farmer and Murray20 ) and that genetic epidemiology supports a strong genetic component to schizoaffective illness (e.g. Andreasen et al, Reference Andreasen, Rice, Endicott, Coryell, Grove and Reich21 Bertelsen et al, Reference Bertelsen and Gottesman22 Farmer et al, Reference Farmer, McGuffin and Gottesman23 Gershon et al, Reference Gershon, Hamovit, Guroff, Dibble, Leckman and Sceery24 Maier et al, Reference Maier, Lichtermann, Minges, Hallmayer, Heun and Benkert25 Slater & Cowie, Reference Slater and Cowie26 Cardno et al Reference Cardno, Rijsdijk, Sham, Murray and McGuffin27 ) with similar heritabilities to those in schizophrenia and bipolar disorder. Our findings are consistent with molecular genetic evidence for the existence of relatively specific genetic susceptibility for a form of major psychiatric illness that has features of both bipolar disorder and prominent psychosis. Reference Williams, Preece, Spurlock, Norton, Williams and Zammit28–Reference Potash, Zandi, Willour, Lan, Huo and Avramopoulos30 This could be interpreted as specific support for a category of ‘schizoaffective’ illness or for the existence of a region of overlap of schizophrenia and bipolar disorder clinical spectra in which the genetic variants that influence susceptibility are easier to identify than are those that confer specific risk to bipolar disorder or schizophrenia alone. In either case, this clinical entity has genetic utility and merits explicit recognition.
The RDC and other modern diagnostic criteria in psychiatry were developed on largely descriptive grounds and we consider it most unlikely that the schizoaffective disorder, bipolar type category will map directly onto the underlying biology. We do not believe that ‘schizoaffective disorder’ in general, or RDC schizoaffective disorder in particular, is a neatly defined, discrete, biological diagnostic entity. Our findings do, however, show that it can be useful for the purposes of research (and perhaps also clinical practice) to identify and classify together sets of cases with such clinical features. Whether, in the long run, this is best achieved by using categories, dimensions or some mixture of the two will require future study. Such further work aimed at refining the relationship between clinical phenotype and genetic risk factors has the potential to help psychiatry move towards a system of classification that relates more closely to underlying pathogenesis.
Clinical features of RDC schizoaffective bipolar disorder
The RDC schizoaffective disorder, bipolar type describes individuals that, in addition to clear-cut episodes of mania, display psychotic symptoms (delusions and/or hallucinations) that are not easily understood as being the result of extreme mood change and that are often seen also in individuals diagnosed with schizophrenia. It does not include all the people with bipolar disorder with psychosis. An analysis using the set of participants with bipolar disorder who had experienced psychotic symptoms during their lifetime did not reveal significantly more independent hits (data not shown). Thus, we can be confident that our finding does not simply relate to a subset of people with bipolar disorder having psychotic features. It is of interest that we did not observe evidence for increased hits in the DSM–IV schizoaffective disorder, bipolar type subset of individuals. It is possible that this may simply reflect the smaller sample size (n = 98) or it may be an indication that the RDC definition of schizoaffective disorder, bipolar type is more biologically useful than that of DSM–IV (at least with respect to identifying the contribution of common genetic variation to disease susceptibility). The RDC definition focuses on temporal co-occurrence of a major affective syndrome with specific types of psychotic features, whereas the focus of the DSM–IV definition is temporal separation of mood and psychotic symptomatology without reference to the quality of the psychotic features.
Implications for revisions of diagnostic classifications
We note that the imminent revision of official diagnostic classifications (i.e. DSM–V and ICD–11) may be influenced by the opinions articulated in several recent articles that the concept of schizoaffective disorder is unreliable, unhelpful and should be abandoned. Reference Maier31–Reference Malhi, Green, Fagiolini, Peselow and Kumari34 In contrast, our data suggest that what is needed is better recognition of such cases. Abandoning the schizoaffective concept is unlikely to be the optimal way of achieving that goal.
Genetic signals in the genome-wide analysis of RDC schizoaffective disorder, bipolar type data-set
As is expected in genome-wide association studies in a modestly sized sample, Reference Craddock, O'Donovan and Owen5 none of the association signals in our analysis of the RDC schizoaffective disorder, bipolar type data-set achieved accepted levels of genome-wide significance for European samples (P<7.2×10–8). Reference Dudbridge and Gusnanto35 Independent replication and meta-analysis will be required to confirm the role of any of the strongly associated loci in susceptibility to schizoaffective disorder. To date there has been no previous report of a systematic genetic association analysis of a set of individuals with schizoaffective disorder in comparison with controls. However, it is interesting to note that an analysis of the WTCCC bipolar disorder data-set that used a completely different analytic approach also identified the RDC schizoaffective disorder, bipolar type subset of participants as being of particular interest. Reference Craddock, Jones, Jones, Kirov, Green and Grozeva36 That analysis used phenotype refinement of a specific genetic association signal of interest in the complete bipolar disorder and control data-set (at GABRB1) and found the signal to be maximal within the RDC schizoaffective disorder, bipolar type subset of participants. Testing of independent SNPs within genes encoding gamma amniobutyric acid (GABA)A receptors showed this set of individuals to have significant system-wide association with variation across the set of SNPs at these receptors (P = 6.6×10–5) Reference Craddock, Jones, Jones, Kirov, Green and Grozeva36 with gene-wide evidence for association at GABRB1, GABRA4, GABRB3, GABRA5 and GABRR1. This is consistent with the current analysis of the same data-set in which we observe a signal at P<0.00001 at GABRB1 (Table 2), and multiple associated SNPs at these other genes within our set of nominally significant associations (online Table DS2). The strongest association signal within the RDC schizoaffective disorder, bipolar type data-set (P = 2.32×10–7) occurred on chromosome 21 with SNPs within the gene B3GALT5, a member of the beta-1,3-galactosyltransferase (beta3GalT) gene family which encode type II membrane-bound glycoproteins with diverse enzymatic functions. To our knowledge, these specific proteins have not been previously implicated in pathophysiology of mood or psychotic illness. The strong association we observe at chromosome 16p13.3 is of interest because it lies within the gene A2BP1, encoding ataxin 2-binding protein 1 isoform 4, a protein that binds to ataxin-2 and may contribute to the restricted pathology of familial neurodegenerative disease, spinocerebellar ataxia type 2. Disruption of A2BP1 has been described in association with neuropsychiatric phenotypes including autism, Reference Martin, Duvall, Ilkin, Simon, Arreaza and Wilkes37 mental retardation and epilepsy. Reference Bhalla, Phillips, Crawford, McKenzie, Mulley and Eyre38 Other genes of potential interest that show association signals at the less stringent significance threshold of P<0.0001 (online Table DS3) include autism susceptibility candidate 2 (AUTS2) on chromosome 7q11.2; BSN, the gene encoding the protein bassoon which is thought to be involved in the organisation of the cytomatrix at the nerve terminals active zone which regulates neurotransmitter release and which is essential in regulated neurotransmitter release from a subset of brain glutamatergic synapses; PTPRG, encoding a member of the protein tyrosine phosphatase family which are signalling molecules that regulate a variety of cellular processes including cell growth, differentiation, mitotic cycle and oncogenic transformation; GRIK2 encoding glutamate receptor, ionotropic kainate 2 precursor (glutamate receptor 6) (GluR-6) (GluR6); and CDH12 encoding cadherin 12, type 2 preproprotein, a type 2 classical cadherin from the cadherin superfamily of integral membrane proteins that mediate calcium-dependent cell–cell adhesion. It will require substantial additional work by us and others in order to confirm which of the polymorphisms showing strong association within the current study influence risk of illness.
Clinical implications
We have used molecular genetic genome-wide case–control association data to compare the genetic association signals according to several different operational categories in the bipolar disorder spectrum. The participants meeting RDC criteria for schizoaffective disorder, bipolar type (a broad definition of schizoaffective disorder) received strongest support (i.e. this was the most genetically useful, and by this criterion, most biologically valid diagnostic subset). It is important for research, and may be important for clinical practice, that such individuals are better recognised and distinguished from other people with mood–psychosis disorders.
Strong consideration is currently being given to abolishing the schizoaffective concept and category from the revisions of the official psychiatric diagnostic classifications (DSM–V and ICD–11). This is likely to be unhelpful to the progress of psychiatric knowledge, given that it is emerging as a diagnostic entity that receives strong research support. We hope that psychiatry is moving towards the time when our patients can benefit from diagnostic concepts that are built on solid foundations of empirical biological evidence rather than being perched precariously on the shifting sands of expert opinion.
Funding
Funding for recruitment and phenotype assessment has been provided by the Wellcome Trust (060620) and the Medical Research Council (G0000647). The genotype analyses were funded by the Wellcome Trust and undertaken within the context of the Wellcome Trust Case Control Consortium (WTCCC).
Acknowledgements
We are indebted to all individuals who have participated in our research. We thank MDF-The BiPolar Organization for the help of its staff and members. The members of the WTCCC are listed in the online supplement. We are grateful to WESC for use of computing resources.
eLetters
No eLetters have been published for this article.