Implications
Food intake by wild herbivores shapes the distribution of both plants and animals across the globe. The use of physiologically based models (PBMs) will help formalize the link between phytochemical consumption and population demography for vertebrate herbivores. Understanding and predicting demographic outcomes of foraging is critical for effective wildlife management, especially in light of rapidly changing food availability and quality across landscapes.
Introduction
A central focus in the field of foraging ecology is to understand how diet quality influences the behavior, distribution and population dynamics of animals. For vertebrate herbivores, the quality and quantity of phytochemicals (both nutrients and toxins) in foods can significantly alter the foraging behavior, physiology and growth of both captive (Dearing et al., Reference Dearing, Mangione and Karasov2002; Sorensen et al., Reference Sorensen, McLister and Dearing2005b; Marsh et al., Reference Marsh, Wallis, McLean, Sorensen and Foley2006) and free-ranging individuals (DeGabriel et al., Reference DeGabriel, Moore, Foley and Johnson2009; Moore et al., Reference Moore, Lawler, Wallis, Beale and Foley2010; Frye et al., Reference Frye, Connelly, Musil and Forbey2013; Stolter et al., Reference Stolter, Ball and Julkunen-Tiitto2013; Ulappa et al., Reference Ulappa, Kelsey, Frye, Rachlow, Shipley, Bond, Pu and Forbey2014). Captive studies have established causal relationships between phytochemicals and foraging (Wiggins et al., Reference Wiggins, McArthur, McLean and Boyle2003; McLean et al., Reference McLean, Boyle, Brandon, Davies and Sorensen2007; Kirmani et al., Reference Kirmani, Banks and McArthur2010; Torregrossa et al., Reference Torregrossa, Azzara and Dearing2012; Shipley et al., Reference Shipley, Davis, Felicetti, McLean and Forbey2012) and individual demographic outcomes such as changes in energy budgets, body mass or survival (Sorensen et al., Reference Sorensen, McLister and Dearing2005b; Kohl et al., Reference Kohl, Weiss, Cox, Dale and Denise Dearing2014 and Reference Kohl, Stengel and Dearing2016b). These studies generally focus on single phytochemicals as an analog for whole plants (Wiggins et al., Reference Wiggins, McArthur, McLean and Boyle2003; McLean et al., Reference McLean, Boyle, Brandon, Davies and Sorensen2007; Kirmani et al., Reference Kirmani, Banks and McArthur2010; Shipley et al., Reference Shipley, Davis, Felicetti, McLean and Forbey2012) and often do not capture the phytochemical complexity of herbivores foraging in landscapes with diverse plant communities.
In the 1950s and the decades following, it was common in studies of free-ranging vertebrate herbivores to measure a single currency (often energy) and use mathematical modeling to make foraging and demographic predictions based on that currency (e.g. optimal foraging theory: Emlen, Reference Emlen1966; MacArthur and Pianka, Reference MacArthur and Pianka1966; Schoener, Reference Schoener1971; Charnov, Reference Charnov1976; Pyke et al., Reference Pyke, Pulliam and Charnov1977; Belovsky, Reference Belovsky1978; dynamic energy budget (DEB) theory: Kooijman and Metz, Reference Kooijman and Metz1984; Nisbet et al., Reference Nisbet, Muller, Lika and Kooijman2000; adaptive herbivore ecology: Owen-Smith, Reference Owen-Smith2002 and Reference Owen-Smith2004). Since then, we have gained knowledge of the complexity of interacting phytochemicals, and the importance of assessing multiple constituents at the same time (e.g. ecological stoichiometry: Muller et al. Reference Muller, Nisbet, Kooijman, Elser and McCauley2001; Moe et al., Reference Moe, Stelzer, Forman, Harpole, Daufresne and Yoshida2005; geometric framework (GF): Simpson and Raubenheimer, Reference Simpson and Raubenheimer1993 and Reference Simpson and Raubenheimer2001; Raubenheimer and Simpson, Reference Raubenheimer and Simpson1998). Field studies on vertebrate herbivores have increasingly incorporated aspects of complex phytochemicals inherent in natural systems when assessing links to population demography (Rode and Robbins, Reference Rode and Robbins2000; Wam et al., Reference Wam, Felton, Stolter, Nybakken and Hjeljord2018). However, field studies often provide only correlative links between phytochemicals and demographic parameters. As a consequence, we lack a mechanistic understanding how the capacity to process phytochemicals by individual herbivores (e.g. Solon-Biet et al., Reference Solon-Biet, McMahon, Ballard, Ruohonen, Wu, Cogger, Warren, Huang, Pichaud, Melvin, Gokarn, Khalil, Turner, Cooney, Sinclair, Raubenheimer, Le Couteur and Simpson2014; Simpson et al., Reference Simpson, Le Couteur, James, George, Gunton, Solon-Biet and Raubenheimer2017) translates to changes in nutritional condition (Parikh et al., Reference Parikh, Forbey, Robb, Peterson, Vucetich and Vucetich2017), reproduction (Brittas, Reference Brittas1988; DeGabriel et al., Reference DeGabriel, Moore, Foley and Johnson2009), survival (Wing and Messmer, Reference Wing and Messmer2016) or population density (Fauchald et al., Reference Fauchald, Park, Tømmervik, Myneni and Hausner2017) of free-ranging vertebrates. Theoretical advances have attempted to use estimates of individual physiological responses to toxic phytochemicals in order to link browsing to population stability of vertebrate herbivores (Feng et al., Reference Feng, Liu, DeAngelis, Bryant, Kielland, Chapin and Swihart2009; Liu et al., Reference Liu, Gourley, DeAngelis and Bryant2012). However, these approaches are limited by a lack of empirical physiological data required to test and refine the parameters that underlie demographic models of wild herbivores consuming complex phytochemicals.
A key to linking foraging behavior to demography is greater ability to predict variation in the physiological consequences of consuming phytochemicals among individuals, among populations within a species (biogeographic variation) and among species (evolutionary variation). The paucity of genomic data for non-model, wild vertebrate herbivores continues to be a critical barrier (although see Ge et al., Reference Ge, Cai, Shen, San, Ma, Zhang, Yi, Chen, Yang and Huang2013; Zhao et al., Reference Zhao, Zheng, Dong, Zhan, Wu, Guo, Hu, He, Zhang and Fan2013; Campbell et al., Reference Campbell, Oakeson, Yandell, Halpert and Dearing2016; Gordon et al., Reference Gordon, Huddleston, Chaisson, Hill, Kronenberg, Munson, Malig, Raja, Fiddes and Hillier2016). The application of functional genomic analyses to wild invertebrate systems demonstrates the great promise for identifying physiological tolerance to phytochemicals (Li et al., Reference Li, Ma, Liang, Chen, Liu and Gao2017; Snoeck et al., Reference Snoeck, Greenhalgh, Tirry, Clark, Leeuwen and Dermauw2017), but such applications are still in their earliest stages for vertebrates (Campbell et al., Reference Campbell, Oakeson, Yandell, Halpert and Dearing2016; Kohl et al., Reference Kohl, Connelly, Dearing and Forbey2016a). In addition, relatively diverse foraging behaviors associated with long life spans and wide geographic dispersal potential of vertebrates often confound the ability to mechanistically link foraging behavior with physiological mechanisms and demographic consequences (Forbey et al., Reference Forbey, Dearing, Gross, Orians, Sotka and Foley2013; DeGabriel et al., Reference DeGabriel, Moore, Felton, Ganzhorn, Stolter, Wallis, Johnson and Foley2014).
We propose that PBMs, particularly those developed in modern biomedical research, provide the needed framework to generate physiological data that can strengthen existing ecological approaches used to predict demographic consequences of plant–herbivore interactions (Figure 1). Specifically, the field of pharmacology has developed PBMs designed to predict the effectiveness and health consequences of pharmaceuticals and nutraceuticals in humans (Esch et al., Reference Esch, Bahinski and Huh2015; Sager et al., Reference Sager, Yu, Raguenau-Majlessi and Isoherranen2015; Ting et al., Reference Ting, Zhao, Xia and Huang2015; Tsamandouras et al., Reference Tsamandouras, Rostami-Hodjegan and Aarons2015). These PBMs use data on molecular expression and physiological function of proteins in the body of consumers to predict what the body does to ingested drugs or nutrients (pharmacokinetics) and what the resultant concentrations of drugs or nutrients do to the body (pharmacodynamics). One objective of PBMs is ‘personalized pharmacology,’ where genetic variation (sequence and expression) of the proteins that absorb, metabolize or bind to specific chemical structures is used to determine the optimal combination and concentration of drugs and nutrients that elicit desired changes in cellular function, body mass and survival (Alyass et al., Reference Alyass, Turcotte and Meyre2015; Locke et al., Reference Locke, Kahali, Berndt, Justice, Pers, Day, Powell, Vedantam, Buchkovich and Yang2015; Wang et al., Reference Wang, Zaman, Mcgee, Milanese, Masoudi-Nejad and O’Connor-McCourt2015; Bray et al., Reference Bray, Loos, McCaffery, Ling, Franks, Weinstock, Snyder, Vassy, Agurs-Collins and Group2016; Rao et al., Reference Rao, Fan, Kobetz and Sussman2017). The result is an accurate prediction of human health at the individual level based on how genes govern the physiological response to ingested chemicals. Similarly, we propose that PBMs could increase the knowledge of physiological interactions between herbivores and ingested phytochemicals to more accurately predict fitness outcomes.

Figure 1 Overview illustrating the relationship between physiological and demographic parameters that can predict physiological and demographic responses in vertebrate herbivores consuming phytochemicals. Key physiological responses include exposure (concentration [Conc]-time course) to both toxic [T] and nutrient [N] phytochemicals based on the expression of genes, proteins and microbes, and functional changes in rates of absorption, digestion, and metabolism of toxin and nutrients that result in concentration-dependent changes in available energy that links to body mass and density of herbivores. aEnergy represents one example of a predicted currency that is influenced by toxin and nutrient exposure in the body of a theoretical herbivore (systemic concentration over time) that can link to body mass and demographic parameters.
Although existing PBMs in humans typically focus on predicting responses to a single drug, there is increased interest to predict health responses to drug–drug and drug–herb interactions (Mano et al., Reference Mano, Sugiyama and Ito2015; Zhao et al., Reference Zhao, Zhang, Grillo, Liu, Bullock, Moon, Song, Brar, Madabushi, Wu, Booth, Rahman, Reynolds, Berglund, Lesko and Huang2011; Gurley et al., Reference Gurley, Markowitz, Williams and Barone2017; Lepist and Ray, Reference Lepist and Ray2017). Similarly, the GF for nutrition provides an analytical framework that considers fitness responses to the multi-dimensional phytochemical environment consumed by herbivores. The underlying idea behind this framework is that the ingestion of one dietary item can affect the ingestion or digestion of another (Simpson and Raubenheimer, Reference Simpson and Raubenheimer2012). For example, the effects of a toxin on an animal’s physiology may be intricately linked to the relative balance of nutrients in the food (Villalba and Provenza, Reference Villalba and Provenza2005; Au et al., Reference Au, Marsh, Wallis and Foley2013). The GF provides an analytical construct that can empirically deal with several phytochemical currencies at the same time, to describe, understand, and predict foraging and fitness outcomes of individuals and groups of animals (Simpson and Raubenheimer, Reference Simpson and Raubenheimer2012). The focus of the GF is on observing the choices that individuals make when faced with different dietary options within a nutritional, multi-dimensional, space to identify patterns of diet selection without a priori assumptions as to what may be guiding that selection. The GF has revealed that the degree to which animals can regulate their intake of multiple nutrients results in a balance (or imbalance) among nutrients that can have demographic consequences (Solon-Biet et al., Reference Solon-Biet, McMahon, Ballard, Ruohonen, Wu, Cogger, Warren, Huang, Pichaud, Melvin, Gokarn, Khalil, Turner, Cooney, Sinclair, Raubenheimer, Le Couteur and Simpson2014; Simpson et al., Reference Simpson, Le Couteur, James, George, Gunton, Solon-Biet and Raubenheimer2017). Geometric framework links multi-dimensional nutrient intake to fitness, and was initially demonstrated in invertebrate species (Simpson and Raubenheimer, Reference Simpson and Raubenheimer1993 and Reference Simpson and Raubenheimer2001). More recently, this framework has been applied to both captive (Sørensen et al., Reference Sørensen, Mayntz, Raubenheimer and Simpson2008; Hewson-Hughes et al., Reference Hewson-Hughes, Hewson-Hughes, Miller, Hall, Simpson and Raubenheimer2011 and Reference Hewson-Hughes, Hewson-Hughes, Colyer, Miller, McGrane, Hall, Butterwick, Simpson and Raubenheimer2012; Solon-Biet et al., Reference Solon-Biet, McMahon, Ballard, Ruohonen, Wu, Cogger, Warren, Huang, Pichaud, Melvin, Gokarn, Khalil, Turner, Cooney, Sinclair, Raubenheimer, Le Couteur and Simpson2014; Felton et al., Reference Felton, Felton, Raubenheimer, Simpson, Krizsan, Hedwall and Stolter2016; Simpson et al., Reference Simpson, Le Couteur, James, George, Gunton, Solon-Biet and Raubenheimer2017) and free-ranging (Rode and Robbins, Reference Rode and Robbins2000; Felton et al., Reference Felton, Felton, Raubenheimer, Simpson, Foley, Wood, Wallis and Lindenmayer2009; Rothman et al., Reference Rothman, Raubenheimer and Chapman2011; Machovsky-Capuska et al., Reference Machovsky-Capuska, Priddel, Leong, Jones, Carlile, Shannon, Portelli, McEwan, Chaves and Raubenheimer2016) vertebrates. Extensive work on the mouse (Mus musculus) under a wide range of dietary conditions demonstrates how the ratios of multiple nutrients consumed directly alters life history traits and metabolic function of individuals (Simpson et al., Reference Simpson, Le Couteur, James, George, Gunton, Solon-Biet and Raubenheimer2017). As demonstrated in invertebrates, post-ingestive absorption and use of nutrients can be accounted for in GF models to explain metabolic and fitness costs of diets (Simpson and Raubenheimer, Reference Simpson and Raubenheimer2001). One important application of GF is an increased capacity to manage fitness through the regulation of nutrient balances. Physiologically based model have potential to aid in GF outcomes by identifying and quantifying the physiological mechanism that increase or decrease the assimilation and concentrations of ingested nutrients within the body compartments of individuals.
Here we describe how PBMs can be used to predict individual variation in phytochemical exposure based on the absorption and metabolism of ingested phytochemicals (kinetics) and nutritional consequences based on concentration-dependent physiological effects of ingested phytochemicals (dynamics). It is important to note that the PBMs we describe focus on predictions made after the animal has selected a food item. In other words, PBMs do not address the complexities of food preferences and other behavioral aspects of food selection (Shipley et al., Reference Shipley, Illius, Danell, Hobbs and Spalinger1999; Felton et al., Reference Felton, Wam, Stolter, Mathisen and Wallgren2018). Instead, we focus on how PBMs can be used to explain variation in physiological parameters that are a consequence of food selection. In addition, we show how PBMs can leverage the GF to treat intake and post-ingestive physiological processes as an integrated system (Raubenheimer and Simpson, Reference Raubenheimer and Simpson1998; Simpson and Raubenheimer, Reference Simpson and Raubenheimer2001) to better predict individual fitness and population-level demographic outcomes. For example, PBMs can predict changes in nutrient assimilation based on known individual variation in genetically determined capacity to detoxify toxins that inhibit digestion and metabolism of nutrients. When applied to GF modeling, knowledge of how concentrations of multiple nutritional currencies are physiologically constrained by toxins can create a united context to explain how animals reach nutritional balance through selection or avoidance of both nutrients and toxins. For example, understanding the physiological capacity to absorb, metabolize, exploit and resist the bioactivity of phytochemicals could explain why some individuals are more strongly inhibited by toxins than others (Pass et al., Reference Pass, McLean, Stupans and Davies2001; Sorensen and Dearing, Reference Sorensen and Dearing2003; Sorensen et al., Reference Sorensen, Turnbull and Dearing2004; Shipley et al., Reference Shipley, Davis, Felicetti, McLean and Forbey2012; Kohl et al., Reference Kohl, Weiss, Cox, Dale and Denise Dearing2014 and Reference Kohl, Pitman, Connelly, Dearing and Forbey2015). Mechanistic PBMs adopted from biomedicine could help provide the physiological knowledge needed to improve management of herbivores. Specifically, PBMs help estimate the individual mechanisms that could, along with the GF and existing demographic models, better connect individual foraging decisions to population demographic consequences.
Physiologically based models
Several key features of PBMs make them particularly applicable to generating the data needed to predict demographic responses of vertebrate herbivores to phytochemicals. First, PBMs have a strong genetic basis that can explain demographic outcomes. For example, genetic variation in efflux transporters and metabolizing enzymes that influence systemic exposure to drugs predict survival of human patients (Nowell et al., Reference Nowell, Ahn, Rae, Scheys, Trovato, Sweeney, MacLeod, Kadlubar and Ambrosone2005; Ekhart et al., Reference Ekhart, Rodenhuis, Smits, Beijnen and Huitema2009). Similarly, microbial genetic diversity within hosts can influence changes in body mass and survival of humans (Turnbaugh et al., Reference Turnbaugh, Ley, Mahowald, Magrini, Mardis and Gordon2006; Celleno et al., Reference Celleno, Tolaini, D’Amore, Perricone and Preuss2007; Tucci et al., Reference Tucci, Boyland and Halford2010). Second, the in vivo and in vitro assays developed to estimate physiological responses to drugs and nutrients for personalized medicine provide ecologists with the necessary experimental tools to quantify physiological data to parameterize PBMs (see Supplementary Material S1). Lastly, while the focus of pharmacological PBMs is to predict human responses to drugs, the physiological processes of absorption and metabolism of drugs are transferable to understanding exposure to both toxic and nutritional phytochemicals in wild herbivores.
While there are numerous physiological mechanisms that could link intake of phytochemicals to demographics, we focus on the physiological processes that influence exposure to phytochemicals. We define phytochemical exposure as the concentration-time course of phytochemicals in the gut, blood or tissues that are linked to changes in physiological function and body mass. Phytochemical exposure is influenced by expression of genes, and activities of proteins and microbes that regulate the absorption and distribution (e.g. efflux transporters), as well as metabolism and excretion (e.g. metabolizing enzymes) of ingested phytochemicals (Sorensen et al., Reference Sorensen, Skopec and Dearing2006; Forbey et al., Reference Forbey, Dearing, Gross, Orians, Sotka and Foley2013). For physiological function, we focus on concentration-dependent changes in digestive and metabolic function because phytochemicals alter digestion and cellular metabolism by vertebrates (Spalinger et al., Reference Spalinger, Collins, Hanley, Cassara and Carnahan2010; Au et al., Reference Au, Marsh, Wallis and Foley2013; Kohl et al., Reference Kohl, Pitman, Connelly, Dearing and Forbey2015) and microbes (Calsamiglia et al., Reference Calsamiglia, Busquet, Cardozo, Castillejos and Ferret2007; Patra and Saxena, Reference Patra and Saxena2011; Al-Jumaili et al., Reference Al-Jumaili, Goh, Jafari, Rajion, Jahromi and Ebrahimi2017). For example, diet interacts with the microbiome to change short chain fatty acid absorption that regulates histone acetylation and cellular energy (West and Meng, Reference West and Meng1968; Schilderink et al., Reference Schilderink, Verseijden and de Jonge2013; den Besten et al., Reference den Besten, van Eunen, Groen, Venema, Reijngoud and Bakker2013). In addition, many toxic phytochemicals can inhibit digestive enzymes (Kohl et al., Reference Kohl, Pitman, Connelly, Dearing and Forbey2015) as well as enzymes responsible for cellular energy production (Forbey et al., Reference Forbey, Pu, Xu, Kielland and Bryant2011). This variation in the efficiency of digestion and cellular metabolism may influence the nutritional resources available for animals to grow, survive and reproduce.
There are no examples, to our knowledge, in wild vertebrate systems that predict phytochemical exposure and resultant changes in available nutrients through PBMs. As such, we describe the specific parameter estimates that can be used in PBMs and demonstrate how PBM outputs can be integrated into existing ecological models to predict demographic consequences (Figure 1). The equations we describe here have been developed for toxic phytochemicals. However, we provide initial ideas of how these equations can be used to predict changes in the nutritional balances that translate to demographic consequences. Our mathematical example chooses energy as the nutritional currency using the assumption that the animal may be limited in energy. Focusing on energy allows PBM predictions to directly integrate into existing bioenergetic models that predict demographics (e.g. DEB model, Kooijman and Metz, Reference Kooijman and Metz1984). However, we acknowledge that to fully understand the underlying nutritional drivers behind the foraging decisions and consequent effects on population dynamics of herbivores, a whole range of nutritional and anti-nutritional constituents and the balance among them must be considered (Simpson and Raubenheimer, Reference Simpson and Raubenheimer2001 and Reference Simpson and Raubenheimer2012). Therefore, other nutritional constituents, such as protein, carbohydrates, fats or minerals, can be similarly used as currencies in the models.
Predicting systemic exposure to ingested phytochemicals
The ability to predict systemic exposure of ingested chemicals has a long history in pharmacology (Teorell, Reference Teorell1937a and 1937Reference Teorellb; Bellman et al., Reference Bellman, Jacquez and Kalaba1960; Jacquez et al., Reference Jacquez, Bellman and Kalaba1960). The addition of PBMs to pharmacological models increased the ability to predict drug concentrations and responses among species with distinct genetic, morphological and physiological traits (Boxenbaum, Reference Boxenbaum1982; Dsouza and Boxenbaum, Reference Dsouza and Boxenbaum1988). Likewise, PBMs that predict systemic exposure of phytochemicals in the body could help ecologists predict phytochemical responses within and among species that are known to differ in physiological capacity and dietary specialization (Pass et al., Reference Pass, McLean, Stupans and Davies2001; Sorensen and Dearing, Reference Sorensen and Dearing2003; Sorensen et al., Reference Sorensen, Turnbull and Dearing2004; Shipley et al., Reference Shipley, Davis, Felicetti, McLean and Forbey2012).
We use mathematical kinetic models and physiological parameter estimates to predict phytochemical exposure (i.e. concentration of phytochemical over time) in the gastrointestinal tract (hereafter, gut; sub index G in models) and blood (sub index B in models) of an herbivore after a single meal (Figure 2, Table 1). For our models, we let I(t) be the food intake of an herbivore at time t. The parameter p is the concentration of toxic phytochemical consumed, and parameter (1−p) is the concentration of nutritional phytochemical consumed. For nutritional phytochemicals, they are further divided into two categories: those that can be absorbed directly across the gut (e.g. glucose and protein) and those that must first be digested or altered by host or microbial enzymes before absorption (e.g. by pancreatic (Whitcomb and Lowe, Reference Whitcomb and Lowe2007) and brush border (Pontremoli et al., Reference Pontremoli, Mozzi, Forni, Cagliani, Pozzoli, Menozzi, Vertemara, Bresolin, Clerici and Sironi2015) enzymes of hosts; cellulase of microbes (Svartström et al., Reference Svartström, Alneberg, Terrapon, Lombard, de Bruijn, Malmsten, Dalin, Muller, Shah and Wilmes2017; Comtet-Marre et al., Reference Comtet-Marre, Chaucheyras-Durand, Bouzid, Mosoni, Bayat, Peyret and Forano2018)). We let (1–r) be the proportion of the nutrient that can be absorbed directly and r is the proportion of the same nutrient, which requires digestion or other modification before absorption. To determine exposure to phytochemicals in the gut, we let T G (t) be the amount of toxic phytochemical in the gut at time t. We let k aG be the absorption rate of the phytochemical from the gut compartment into the blood and we let k mG be the rate of phytochemical metabolized (for toxins) or digested (for nutrients) by both host and microbial enzymes in the gut before absorption. These rates are dependent on the physio‐chemical properties (e.g. tissue/blood partition coefficients, Camp et al., Reference Camp, Garavelas and Campitelli2015a; Daina et al., Reference Daina, Michielin and Zoete2017) of each ingested phytochemical and the expression and activity of transport and metabolizing proteins by hosts and associated microbiome (Peters and McLeod, Reference Peters and McLeod2008; Peters et al., Reference Peters, Jones, Ungell and Hatley2016; Wilson and Nicholson, Reference Wilson and Nicholson2017; Cui, Reference Cui2018; Santos et al., Reference Santos, Niemi, Hiratsuka, Kumondai, Ingelman-Sundberg, Lauschke and Rodríguez-Antona2018). Rates may also be influenced by interactions with other co-occurring phytochemicals consumed by the herbivores (Chung et al., Reference Chung, Jang, Han, Jang, Kwon, Seo and Lee2007; Androutsopoulos et al., Reference Androutsopoulos, Papakyriakou, Vourloumis, Tsatsakis and Spandidos2010; Chen et al., Reference Chen, Zhou and Ji2010).

Figure 2 Example of concentrations of toxic phytochemicals ([Toxin], toxin exposure) resulting from food intake in gut compartments (a) and blood (b) predicted from physiologically based models that translate to changes in assimilated energy (c) for a theoretical vertebrate herbivore that has high (solid line) and low (dashed line) efficiency of digestion and metabolism of energy from ingested food (see Table 1 for parameter definitions). Specifically, the solid line represents the scenario for a specialized herbivore with relatively high tolerance to a given toxic phytochemical that has specialized molecular mechanisms that limit absorption (k aG = 0.01) and maximize metabolism in the gut by both herbivore and microbes (k mG =0.05) and in the liver of the herbivore (k mL =0.1). The dashed line represents the scenario for a generalized herbivore with relatively low tolerance to the same toxic phytochemical that has generalized molecular mechanisms resulting in relatively higher absorption rates (k aG = 0.03) and slower metabolism in the gut (k mG = 0.05) and liver (k mL = 0.05). Other parameter values are constant: G 0 and B 0 =20, V=1 (equations (1) to (4)). Efficiency of digestion (e.g. microbial function) and energy metabolism in the cells (e.g. mitochondrial metabolism) are dependent on [Toxin] and mediate assimilated energy for animals used to determine body mass.
Table 1 Definition of parameters used in models to predict physiological responses to the intake of phytochemicals by vertebrate herbivores
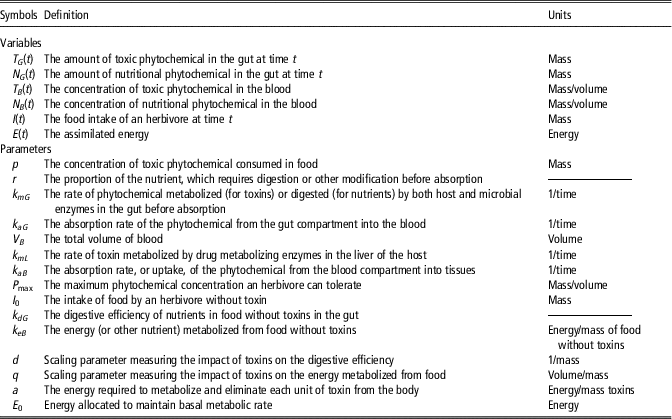
For toxic phytochemicals, the variable T G (t) satisfies the following differential equation:

For nutritional phytochemicals, N G (t) satisfies the following differential equation:

both with given initial values. For toxins, this equation means the rate of change of toxin in the gut (i.e. phytochemical exposure in the gut, Figure 2) equals the amount of toxin absorbed from the gut compartment into the blood minus the amount of phytochemical metabolized by herbivores and gut microbes before absorption. For nutrients, the rate of change of nutrient in the gut equals the amount of nutrient directly absorbed from the gut compartment into the blood plus the amount of nutrient digested (or liberated) by herbivores and gut microbes before absorption. In other words, host and microbial modification in the gut reduces T G (t) of toxic phytochemicals, whereas host and microbial modification, specifically digestion of larger macronutrients in the gut, increases N G (t) of nutritional phytochemicals in the gut.
To determine systemic exposure to phytochemicals (i.e. concentration over time in blood), we let T B (t) and N B (t) denote the concentration of toxic phytochemical and nutritional phytochemical in the blood, respectively. For toxins, systemic exposure is reduced by first pass metabolism in the liver and distribution of toxin into tissues. We let k mL be the rate of toxin metabolized by drug metabolizing enzymes in the liver of the host and k aB be the absorption rate, or distribution, of the phytochemical from the blood compartment into tissues. We let V B be the total volume of blood estimated from allometric equations (Lindstedt and Schaeffer, Reference Lindstedt and Schaeffer2002).
For toxins, the dynamics of T B (t) can be described by the following differential equation which represents a one compartment model:

Existing pharmacological pharmacokinetic models allow for this equation to include multiple compartments where the distribution of toxins from blood into other tissue compartments can be included (Macheras and Iliadis, Reference Macheras and Iliadis2006). For nutrients, the dynamics of N B (t) can be described by the following differential equation:

with given initial conditions for both equations. The toxin equation means that the rate of change of the total amount of toxin in the blood (i.e. phytochemical exposure in the blood, Figure 2) equals the amount of toxin absorbed from the gut compartment into the blood minus the amount of toxin metabolized by the liver or distributed into other compartments. For nutrients, the rate of change of the total amount of nutrient in the blood is the amount of nutrient absorbed from the gut compartment into the blood minus the uptake rate of nutrient from the blood into tissue compartments where it is used for cellular metabolism. If the animal is in a deficit for a particular nutrient, which means that the value of N B (equation (4)) is too low, the equation can be modified to account for the additional amount of nutrient generated endogenously by the liver or other body compartments (e.g. glucose, lipids; Schultz et al., Reference Schultz, Barbosa-da-Silva, Aguila and Mandarim-de-Lacerda2015; Yu et al., Reference Yu, Wang, Huang, Li, Zeng, Lei, Hu, Chen, Lu, Xie and Zeng2016).
Predicting change in nutritional resources
Phytochemical exposure in the gut or blood estimated from PBMs can be used to predict changes in the availability of nutritional resources required for herbivores to gain body mass (Figure 3). In the simplest scenario, I(t) (food intake) is decided by the following:

where, P max is the maximum phytochemical concentration an herbivore can tolerate. The term I 0 the intake of food by an herbivore without toxin or that does not contain nutrients in excess of requirements whose value may be taken as the ratio of the bite size of food and the time used to take this bite. The novel component in our initial PBM is that we allow food intake of an herbivore to be instantaneously influenced by concentration of toxic and nutritional phytochemicals that are in excess of what herbivores can tolerate. Several studies demonstrate upper limits, thresholds or rules of compromise for single or interacting phytochemicals (Sorensen et al., Reference Sorensen, McLister and Dearing2005b and 2005Reference Sorensen, Heward and Dearinga; Felton et al., Reference Felton, Felton, Raubenheimer, Simpson, Foley, Wood, Wallis and Lindenmayer2009; Raubenheimer et al., Reference Raubenheimer, Simpson and Mayntz2009 and Reference Raubenheimer, Machovsky-Capuska, Felton and Simpson2014; Torregrossa and Dearing, Reference Torregrossa and Dearing2009; Rothman et al., Reference Rothman, Raubenheimer and Chapman2011; Frye et al., Reference Frye, Connelly, Musil and Forbey2013; Solon-Biet et al., Reference Solon-Biet, McMahon, Ballard, Ruohonen, Wu, Cogger, Warren, Huang, Pichaud, Melvin, Gokarn, Khalil, Turner, Cooney, Sinclair, Raubenheimer, Le Couteur and Simpson2014; Camp et al., Reference Camp, Shipley, Johnson, Forbey, Rachlow and Crowell2015b). However, we recognize that few thresholds are absolute and estimates of P max must be determined based on specific genetic, physiological, behavioral and environmental conditions (see Supplementary Material S1). Parameters estimated from PBMs could help explain the physiological conditions that influence thresholds and nutritional compromises observed within and among species (see Supplementary Material S2).

Figure 3 Example of how estimated concentrations of toxic phytochemicals ([Toxin], toxin exposure) resulting from food intake influences body mass through changes in nutrient assimilation (digested and metabolized) in a theoretical vertebrate herbivore predicted by physiologically based models (see Table 1 for parameter definitions).
We present a simplified example of how the nutritional currency of energy (E) needed for mass gain can be influenced by toxin exposure estimated from PBMs (Figure 2). We let E + (t) denote the energy intake at time t. The energy intake from food is decided by how much food is consumed, the concentration of toxin in food, and the toxin-dependent changes in digestive and metabolic function that converts food into assimilated energy. We assume the energy intake at time t is defined as:

where k dG is the digestive efficiency of nutrients in food without toxins in the gut, which is decreased by higher toxin concentrations in the gut (T G (t)) (Robbins et al., Reference Robbins, Hagerman, Austin, McArthur and Hanley1991; Kohl et al., Reference Kohl, Pitman, Connelly, Dearing and Forbey2015) with a scaling parameter d. k eB is the energy (or other nutrient) metabolized from food without toxins in the blood, which is decreased by higher toxin concentrations in the blood (T B (t)) (e.g. Forbey et al., Reference Forbey, Pu, Xu, Kielland and Bryant2011) with a scaling parameter q.
The rate of change of total assimilated energy E(t), or net energy assimilated, equals the difference between energy intake E + (t) and the sum of: energy used to eliminate the toxins (before and after absorption), energy lost due to toxin-dependent reduction in digestive efficiency in the gut (Robbins et al., Reference Robbins, Hagerman, Austin, McArthur and Hanley1991; Kohl et al., Reference Kohl, Pitman, Connelly, Dearing and Forbey2015), toxin-dependent reduction in energy metabolism in cells (Forbey et al., Reference Forbey, Pu, Xu, Kielland and Bryant2011), and energy allocated to maintain basal metabolic rate (E 0). Net energy assimilated is shown by the equation:

The second term on the right side of the equation (
$a V_{B} k_{{mL}} T_{B} (t))$
is the loss of endogenous energy and a is the energy required to metabolize and eliminate each unit of toxin from the body. Endogenous loss of energy (or other nutrient currencies) could arise from increased metabolic costs above basal metabolism (Bozinovic and Novoa, Reference Bozinovic and Novoa1997; Boyle and Dearing, Reference Boyle and Dearing2003; McLister et al., Reference McLister, Sorensen and Dearing2004) or loss of endogenous substrates, such as glucose in the form of glucuronic acid conjugates, required for detoxification (Guglielmo et al., Reference Guglielmo, Karasov and Jakubas1996; Sorensen et al., Reference Sorensen, McLister and Dearing2005b; Marsh et al., Reference Marsh, Wallis, McLean, Sorensen and Foley2006; Au et al., Reference Au, Marsh, Wallis and Foley2013; Parikh et al., Reference Parikh, Forbey, Robb, Peterson, Vucetich and Vucetich2017).
Predicting change in body mass
From the available energy E(t), we can predict relative toxin-dependent changes in body mass that determine demographic rates. In some cases, we know the energetic costs associated with the intake of toxic plants can result in loss of body mass (Guglielmo et al., Reference Guglielmo, Karasov and Jakubas1996; Sorensen et al., Reference Sorensen, McLister and Dearing2005b). As such, we predict smaller body mass is associated with lower assimilated energy predicted from PBMs when a given animal is consuming toxic phytochemicals (Figure 3). While the initial physiological models we present focus on energy, any nutritional currency that is known to change body mass or other demographic parameters could be modeled with these equations and then integrated into population models.
Predicting population-level responses to phytochemicals
For physiological predictions, we have relied on human biomedical insights used to predict individual responses to specific doses of phytochemicals (e.g. personalized medicine, Alyass et al., Reference Alyass, Turcotte and Meyre2015; Aarnoutse et al., Reference Aarnoutse, de Vos-Geelen, Penders, Boerma, Warmerdam, Goorts, Damink, Soons, Rensen and Smidt2017). However, the ultimate measure of whether phytochemical exposure and resultant changes in physiological function associated with foraging matter for ecology, evolution and conservation of wildlife is whether variations in these parameters change individual fitness and, ultimately, population growth rates. There is an extensive and sustained effort to model the links among foraging behavior, physiology and demography (e.g. Kooijman and Metz, Reference Kooijman and Metz1984; Moen et al., Reference Moen, Pastor and Cohen1997; Nisbet et al., Reference Nisbet, Muller, Lika and Kooijman2000; Wikelski and Cooke, Reference Wikelski and Cooke2006). The most salient challenge remaining in this context is to build models that are structured in ways that make an insightful connection between physiology and demography, yet are estimable via statistical analysis of available data. The DEB growth model, or Kooijman–Metz model (Kooijman and Metz, Reference Kooijman and Metz1984), offers one approach where physiological data from individuals (e.g. body size) is used to describe growth and reproduction parameters that predict population dynamics (Smallegange et al., Reference Smallegange, Caswell, Toorians and Roos2017). The application of DEB models in the field of ecotoxicology demonstrate how energetic responses to exposure to environmental toxins within individuals predict population changes (Kooijman and Metz, Reference Kooijman and Metz1984; Jager et al., Reference Jager, Barsi, Hamda, Martin, Zimmer and Ducrot2014; Desforges et al., Reference Desforges, Sonne and Dietz2017). In addition, recent agent-based models (ABMs) demonstrate how energy budgets of individual organisms can be used to predict population-level responses to food availability (Sibly et al., Reference Sibly, Grimm, Martin, Johnston, Kułakowska, Topping, Calow, Nabe‐Nielsen, Thorbek and DeAngelis2013).
Despite these well-established theories and modeling advances, there remains a mechanistic gap between knowledge of the phytochemicals consumed by herbivores and the energetic consequences used in DEB models that lead to changes in demographic rates. One major complication in establishing physiology-dependent links between foraging, energetics and demographics is that nutritional currencies do not act in isolation (Felton et al., Reference Felton, Wam, Stolter, Mathisen and Wallgren2018). Even when devoid of toxin or excess nutrients, intake and thus nutritional balance and changes in body mass are influenced not only by intake rates, but also by synergies among simultaneously ingested foods. For example, dietary protein can interact with toxins to influence body mass (Au et al., Reference Au, Marsh, Wallis and Foley2013) while tannins can reduce digestion and metabolism of protein (DeGabriel et al., Reference DeGabriel, Wallis, Moore and Foley2008) thereby reducing reproductive success (DeGabriel et al., Reference DeGabriel, Moore, Foley and Johnson2009). These types of interactions can be captured through estimates of P max (maximum phytochemical concentration, equation (5)) or adjusting scaling parameters d and q to account for known changes in the efficiency of digestion and metabolism (equation (6)), respectively, that are explicitly dependent on P max. Estimated exposure to each individual phytochemical consumed from PBMs can be used to predict interactions or identify conditions whereby an animal may experience a systemic excess or deficit of a nutrient to explain foraging behaviors.
While consequences of nutrient deficiencies or excess toxins for an animal is relatively easy to understand, it is less obvious that excess levels of nutrients can cause problems. However, examples from wildlife show that excess amounts of specific amino acids in food items can result in reduced food intake (DeGabriel et al., Reference DeGabriel, Foley and Wallis2002) or increased demand of thermal energy (Soppela et al., Reference Soppela, Nieminen and Saarela1992). Furthermore, in ruminant herbivores, overingestion of non-structural carbohydrates causes rumen pH to decline, potentially causing health issues such as ruminal acidosis (Wobeser and Runge, Reference Wobeser and Runge1975; Cynthia and Scott, Reference Cynthia and Scott2005; Butler et al., Reference Butler, Jensen, Johnson and Scott2008; Müller et al., Reference Müller, Lackey, Streich, Fickel, Hatt and Clauss2010). The GF is currently the only way to disentangle the effects of nutrient deficiencies from concurrent surpluses on imbalanced diets (Raubenheimer et al., Reference Raubenheimer, Lee and Simpson2005).
Physiologically based models integrated into the geometric framework for nutrition
The PBMs we present only estimate toxin-dependent changes in nutrient balance in response to a single phytochemical. Although concentrations of specific phytochemicals are associated with loss of body mass in simplified captive feeding trials (McLean et al., Reference McLean, Boyle, Brandon, Davies and Sorensen2007; Shipley et al., Reference Shipley, Davis, Felicetti, McLean and Forbey2012), our described PBMs do not capture the complex phytochemical state of an individual herbivore. Much research has shown that it is not enough to use a single nutritional proxy to predict fitness (Simpson and Raubenheimer, Reference Simpson and Raubenheimer2001; Raubenheimer et al., Reference Raubenheimer, Simpson and Mayntz2009; Rothman et al., Reference Rothman, Raubenheimer and Chapman2011; Solon-Biet et al., Reference Solon-Biet, McMahon, Ballard, Ruohonen, Wu, Cogger, Warren, Huang, Pichaud, Melvin, Gokarn, Khalil, Turner, Cooney, Sinclair, Raubenheimer, Le Couteur and Simpson2014; Felton et al., Reference Felton, Felton, Raubenheimer, Simpson, Krizsan, Hedwall and Stolter2016; Simpson et al., Reference Simpson, Le Couteur, James, George, Gunton, Solon-Biet and Raubenheimer2017). Even when we account for direct affects of toxic phytochemicals on nutrient digestibility, it is the balance among nutrients that is important for predicting fitness (Simpson and Raubenheimer, Reference Simpson and Raubenheimer2001). The GF provides a framework for using estimated exposure to nutrients in the body derived from PBMs to predict how deficits or excess of nutrient concentrations interact to affect fitness in individuals. The GF takes into consideration that the mixture of many different nutritional currencies (energy and specific macro- and micronutrients) interact with each other and with toxic phytochemicals to influence food intake and resultant fitness (Simpson and Raubenheimer, Reference Simpson and Raubenheimer2001 and Reference Simpson and Raubenheimer2012). Outcomes of GF experiments include response surfaces that predict fitness relative to the intake of specific absolute intakes and ratios of multiple nutrients and therefore capture multi-dimensional parameters of foraging (Simpson and Raubenheimer, Reference Simpson and Raubenheimer2012).
The benefit of PBMs is that they have the potential to reduce the residual in these response surfaces and more accurately predict fitness outcomes. We propose that individual physiological parameters such as gut and blood concentrations of interacting phytochemicals are more functionally relevant than absolute intake of nutrients alone. In other words, estimates of phytochemical exposure from PBMs provide a mechanistic explanation for fitness consequences relative to observed intake of multiple nutrients. As a simplified example, take two individual herbivores (1 and 2) that consume the same amount of plant material with a known concentration of a toxic phytochemical that inhibits assimilation of an essential nutrient in a concentration-dependent manner (Figure 4). Subject 1 is a specialized herbivore with physiological mechanisms that limit absorption and maximize metabolism of the toxin resulting in relatively low systemic toxin exposure compared to subject 2 (Figures 2 and 4a). In this scenario, subject 2 is exposed to higher concentrations of the toxin and therefore assimilates a lower concentration of the essential nutrient in the body than subject 1 (Figure 4b). Assuming both subjects have similar requirements for the nutrient, subject 2 must consume higher absolute amounts of the nutrient than subject 1 due to lower nutrient assimilation (Figure 4c). Animals not able to compensate for toxin-dependent deficits in assimilation efficiency of nutrients by increasing intake are predicted to have reduced fitness. Based on the GF, the theoretical response surface that includes both of these subjects would show variation in the absolute intake of this nutrient associated with maximum fitness (x-axis in Figure 4d). We propose that estimates of the concentration of the nutrient in the body generated from PBMs would reduce variation along this nutritional axis and more precisely correlate with fitness outcomes (Figure 4e) than use of absolute intake alone. This approach could be repeated for any number of nutrients included in the GF using physiological predictions derived from PBMs (Figures 2 and 3). This simplified example demonstrates how estimates of phytochemical exposure offered through PBMs could provide more mechanistically accurate predictions of fitness in individual herbivores within the GF.

Figure 4 Example showing how predicted concentrations of toxic phytochemicals ([Toxin], toxin exposure, (a)) and toxin-dependent changes in concentrations of an essential nutrient ([Nutrient X], nutrient exposure, (b)) from physiologically based models influence interpretation of response surfaces (red is greater fitness) predicted from the geometric framework (d, e) for a theoretical vertebrate herbivore subject (1) that has physiological mechanisms that limit absorption and maximize metabolism of the toxin more than another theoretical herbivore subject (2). Both herbivores consume the same amount of a toxic phytochemicals and nutrients and the toxin reduces concentrations of Nutrient X in the body in a concentration-dependent manner (b). Subject (2) is exposed to higher concentrations of the toxin and lower concentrations of the essential nutrient in the body (b). To maintain concentrations of Nutrient X (dashed line) required for maintenance (below excess and above deficit), subject (2) must consume higher amounts of the nutrient than subject (1) (c). The theoretical response surface based on absolute intake of Nutrient X generated from the geometric framework would show variation in the absolute intake of Nutrient X associated with maximum fitness (d). In contrast, estimates of the concentration of the nutrient in the body generated from physiologically based models reduce variation along the Nutrient X axis (e) compared to using absolute intake because both subjects are consuming food to reach an optimal body concentration of nutrient X.
Physiologically based models integrated into demographic models
While including more accurate fitness outcomes from physiological exposure of nutrients into the GF is ideal for predicting population consequences, testing model predictions of fitness in vertebrates is often logistically challenging. Changes in body mass represent a realistic fitness surrogate that can be measured in a wide range of vertebrates and then used to develop and test demographic models that predict population-level outcomes of consuming phytochemicals. Body mass is dependent on mechanisms that increase or decrease physiological exposure to nutrients that can create nutritional imbalances (Simpson et al., Reference Simpson, Batley and Raubenheimer2003; Simpson and Raubenheimer, Reference Simpson and Raubenheimer2005; Solon-Biet et al., Reference Solon-Biet, McMahon, Ballard, Ruohonen, Wu, Cogger, Warren, Huang, Pichaud, Melvin, Gokarn, Khalil, Turner, Cooney, Sinclair, Raubenheimer, Le Couteur and Simpson2014; Sorensen et al., Reference Sorensen, McLister and Dearing2005b and Reference Sørensen, Mayntz, Raubenheimer and Simpson2008). Quantifying physiology-dependent nutrient concentrations in the body and resultant changes in body mass of individuals within a population will get ecologists closer to accurately predicting demographic consequences for vertebrates. Nutrient concentrations in the body can be estimated from PBMs (Figures 2 and 3) parameterized with physiological data from in vitro and in vivo assays (described in Supplementary Material S1) and body mass can be measured directly from whole animals or indirectly from fecal pellet size in some species (Coe and Carr, Reference Coe and Carr1983; Smith et al., Reference Smith, Betancourt and Brown1995; Reilly, Reference Reilly2002; Morden et al., Reference Morden, Weladji, Ropstad, Dahl and Holand2011). Numerous studies have demonstrated that body size predicts the demography of long-lived animals, meaning that larger individuals tend to have better growth, survival and reproduction than smaller individuals (Rees et al., Reference Rees, Childs and Ellner2014; Barneche et al., Reference Barneche, Robertson, White and Marshall2018). Because toxin exposure can influence body size through changes in available energy both in real organisms (Sorensen et al., 2005Reference Sorensen, McLister and Dearingb and Reference Sorensen, McLister and Dearing2005c) and in the above physiological models, we propose that size-structured population models (sensu Rees et al., Reference Rees, Childs and Ellner2014) can translate toxin-dependent changes in assimilated nutrients and body mass in individual animals to changes in population growth rates.
Integral projection models (IPMs) are an example of size-structured population models that are widely used to quantify evolutionary fitness, forecast impacts of wildlife management and understand why population dynamics vary between geographically separated populations (Metcalf et al., Reference Metcalf, Rose and Rees2003; Coulson et al., Reference Coulson, MacNulty, Stahler, vonHoldt, Wayne and Smith2011; Smallegange et al., Reference Smallegange, Caswell, Toorians and Roos2017). Smallegange et al. (Reference Smallegange, Caswell, Toorians and Roos2017) demonstrated that DEB theory can be incorporated into IPMs and that predictions from the resultant DEB-IPM model closely matched empirical data on population growth rate. However, incorporating the physiological details of herbivory in IPMs remains a major research gap. To bridge this gap, we propose developing IPMs that predict demographic rates as a function of both body mass and phytochemical exposure (Figure 5). By including phytochemical exposure along with body mass, we can better account for the potentially interacting consequences of phytochemicals on energy balance as well as any other nutritional currency that influences demographic parameters of animals (e.g. fat or protein composition; Parker et al., Reference Parker, Barboza and Gillingham2009). Specifically, IPMs can be fitted with empirically derived physiological parameters to predict body mass or other fitness parameters (e.g. survival or number of offspring). The analytical tractability of IPMs leads to a suite of demographic metrics, including long- and short-term population growth rate, sensitivity of population growth rate to environmental conditions, and stable size distribution, that can be easily compared across species and study sites (Rees et al., Reference Rees, Childs and Ellner2014). The cost of IPMS is that they may be limited in the level of complexity they can handle.

Figure 5 Example of demographic changes based on body mass relative toxin exposure of individual animals predicted from integral projection models. Toxin exposure and body mass are predicted from physiologically based models (Figures 2 and 3) or measured directly from individuals. Body mass is one of several surrogates of fitness that could be used.
Agent-based models provide an additional modeling framework that can scale-up individual-level variation to population dynamics. Moreover, ABMs can incorporate complex data such as variable genotypes, physiological traits and environmental conditions (Golestani et al., Reference Golestani, Gras and Cristescu2012; Sibly et al., Reference Sibly, Grimm, Martin, Johnston, Kułakowska, Topping, Calow, Nabe‐Nielsen, Thorbek and DeAngelis2013; Khater et al., Reference Khater, Murariu and Gras2016). The ABMs represent individuals within a virtual environment that, based on their individual traits, exhibit differences in growth, survival and reproduction. The ABMs allow researchers to manipulate virtual genotypes (host and microbiome) or physiological functions that would not be feasible in the field (Torrens, Reference Torrens2010; Caughlin et al., Reference Caughlin, Ferguson, Lichstein, Bunyavejchewin and Levey2014 and Reference Caughlin, Ferguson, Lichstein, Zuidema, Bunyavejchewin and Levey2015; Ruktanonchai et al., Reference Ruktanonchai, DeLeenheer, Tatem, Alegana, Caughlin, Erbach-Schoenberg, Lourenço, Ruktanonchai and Smith2016; Pauli et al., Reference Pauli, Spaul and Heath2017). Virtual individuals can be assigned genome- and phytochemical-dependent physiological responses that are based on empirical data established from in vitro and in vivo experiments (see Supplementary Material S1). When simulated across multiple generations, even subtle differences in individual traits (genomes) and interactions with the environment, such as energetic responses to interacting concentrations of toxins and nutrients predicted from PBMs, can result in emergent patterns of population persistence and trait composition (Grimm et al., Reference Grimm, Revilla, Berger, Jeltsch, Mooij, Railsback, Thulke, Weiner, Wiegand and DeAngelis2005). A potential tradeoff of more complex ABMs relative to analytically tractable models (e.g. IPMs) is a lack of generalizability across study systems (Morris and Doak, Reference Morris and Doak2002).
Integrating complex physiological and demographic data into any demographic model presents a challenge because data often comes from disparate sources. Many wildlife monitoring programs offer extensive data on population time series, but rarely collect information on phytochemicals in the diets or physiological data for these populations. Instead, physiological data are most often limited to experimental trials on whole individuals or derived from in vitro assays (see Supplementary Material S1). Physiological data can also be derived from new databases (Daina et al., Reference Daina, Michielin and Zoete2017) that predict the physiological activity of a vast array of metabolites from plants and the excreta of animals based on the structure of the metabolite (Servello and Schneider, Reference Servello and Schneider2000; Sauvé and Côté, Reference Sauvé and Côté2006; Van Duynhoven et al., Reference Van Duynhoven, Vaughan, Jacobs, Kemperman, Van Velzen, Gross, Roger, Possemiers, Smilde and Doré2011; Tay-Sontheimer et al., Reference Tay-Sontheimer, Shireman, Beyer, Senn, Witten, Pearce, Gaedigk, Gana Fomban, Lutz and Isoherranen2014; Parikh et al., Reference Parikh, Forbey, Robb, Peterson, Vucetich and Vucetich2017). As such, a gap remains between observational data from populations and physiological data from individuals.
Hierarchical Bayesian methods present a way to integrate disparate data sources to model population dynamics, while accounting for imperfect detection (Zipkin and Saunders, Reference Zipkin and Saunders2018). For example, Bayesian models could integrate variation in individual responses to phytochemicals predicted from PBMs (Figures 2 and 3) or predicted from known metabolite structures (Daina et al., Reference Daina, Michielin and Zoete2017), predicted fitness metrics estimated from response surfaces generated from the GF (Figure 4), experimental data from animals in captivity (e.g. body size) or obtained from captured animals in the field, as well as long-term data on population density and abundance of herbivore populations. Hierarchical Bayesian models can be applied to fit both IPMs and ABMs (Caughlin et al., Reference Caughlin, Ferguson, Lichstein, Zuidema, Bunyavejchewin and Levey2015; Elderd and Miller, Reference Elderd and Miller2016). Once the joint models (i.e. PBMs within the GF coupled with IPMs or ABMs) are fit to data, sensitivity analysis (Saltelli et al., Reference Saltelli, Ratto, Tarantola and Campolongo2006) presents a way to evaluate the demographic importance of physiological variation. This analysis would enable researchers to quantify how variability in the input of phytochemical exposure (from PBMs), available energy, body mass and fitness metrics translates into output for demographic models (population growth rate). The sensitivity analysis can directly quantify which physiology-dependent parameters best explain population growth rates.
Test and revise models
While testing physiology-dependent predictions of population dynamics in long-lived species is logistically challenging, we identify a few options for testing and improving initial models.
In vitro manipulation to test physiologically based models
A variety of modifications to in vitro assays can be used to parameterize PBMs (see Supplementary Material S1) and test predictions of those models in specific study organisms (see Supplementary Material S2). Both host enzymes and microbial communities can be exposed in vitro to different combinations of phytochemicals that vary in concentration and composition. For example, Kriszan et al. (2018) quantified changes in efficiency of digestion (fermentation) by testing rumen liquid from free-ranging moose (a browsing species) and dairy cows (a grazing species) on a combination of spring and summer foods commonly eaten by moose in Northern Sweden. The naturally growing plants differed in their nutrient and toxin concentrations. The assay showed that fermentation efficiency is host-specific and related to the composition of the rumen microbial community. Moreover, the pattern was in agreement with the evolutionary adaptations related to feeding habitats and morphophysiological differences between browsers and grazers. Results such as these can be used to identify which phytochemical combinations, microbes and host enzyme activity minimize or maximize exposure to phytochemicals (fast rates of metabolism by microbes) and maximize or minimize physiological function (Figure 1).
In vivo manipulation to test demographic models
Model predictions can also be tested by in vivo manipulation of the molecular mechanisms regulating phytochemical exposure. Host and/or microbiome enzyme activity can be manipulated through drug–drug interaction assays. A number of phytochemicals naturally consumed by herbivores are known to inhibit transporters and metabolizing enzymes in humans and cause altered systemic exposure to medication (Chung et al., Reference Chung, Jang, Han, Jang, Kwon, Seo and Lee2007; Androutsopoulos et al., Reference Androutsopoulos, Papakyriakou, Vourloumis, Tsatsakis and Spandidos2010; Chen et al., Reference Chen, Zhou and Ji2010). For example, phytochemical exposure and demographic responses of populations can be assessed by varying phytochemical concentrations of food given to animals with and without co-administration of inducers (e.g. kaempferol, Chung et al., Reference Chung, Jang, Han, Jang, Kwon, Seo and Lee2007) or inhibitors (e.g. flavonoids, Androutsopoulos et al., Reference Androutsopoulos, Papakyriakou, Vourloumis, Tsatsakis and Spandidos2010) of transporters and metabolizing enzymes. In addition, tolerance to phytochemicals can be altered by pairing variation in dietary phytochemicals with manipulation of microbial communities of the gut (Kohl et al., Reference Kohl, Weiss, Cox, Dale and Denise Dearing2014 and Reference Kohl, Stengel and Dearing2016b). The gut microbiome can be manipulated by providing herbivores with food of varying phytochemistry that is mixed with feces or rumen fluid collected from other herbivores with specific physiological mechanisms of tolerance to specific phytochemicals (Jones and Megarrity, Reference Jones and Megarrity1986; Pratchett et al., Reference Pratchett, Jones and Syrch1991; Kohl et al., Reference Kohl, Stengel and Dearing2016b; Griffith et al., Reference Griffith, Ribeiro, Oba, McAllister and Beauchemin2017).
We suggest that coupling the manipulation of phytochemicals with the manipulation of host and microboime function would allow researchers to test model-predicted changes in a number of physiological and demographic parameters. Researchers could observe changes in intake of food of known phytochemistry through direct observations (McShea and Schwede, Reference McShea and Schwede1993; Parker et al., Reference Parker, Gillingham, Hanley and Robbins1996; Felton et al., Reference Felton, Felton, Raubenheimer, Simpson, Foley, Wood, Wallis and Lindenmayer2009; Johnson et al., Reference Johnson, Raubenheimer, Rothman, Clarke and Swedell2013) or animal-borne video (Hays, Reference Hays2015; Garnick et al., Reference Garnick, Barboza and Walker2018). Fecal collections can be used to monitor changes in microbial communities from metagenomic studies and phytochemical exposure in the gut from metabolomic studies. Phytochemical exposure and nutritional indices could be further assessed through concentrations of metabolites in excreta (Servello and Schneider, Reference Servello and Schneider2000; Sauvé and Côté, Reference Sauvé and Côté2006; Van Duynhoven et al., Reference Van Duynhoven, Vaughan, Jacobs, Kemperman, Van Velzen, Gross, Roger, Possemiers, Smilde and Doré2011; Tay-Sontheimer et al., Reference Tay-Sontheimer, Shireman, Beyer, Senn, Witten, Pearce, Gaedigk, Gana Fomban, Lutz and Isoherranen2014; Parikh et al., Reference Parikh, Forbey, Robb, Peterson, Vucetich and Vucetich2017) and taking direct or indirect measures of changes in body mass. Furthermore, researchers could partner with federal and state wildlife agencies to monitor relationships among physiological function and demographics in free-ranging wild and domestic vertebrate herbivores. State and federal agencies often collect long-term demographic data for game species and routinely monitor body mass, survival and reproductive success of individuals (Bishop et al., Reference Bishop, White, Freddy, Watkins and Stephenson2009; De Jager and Pastor, Reference De Jager and Pastor2009; Revermann et al., Reference Revermann, Schmid, Zbinden, Spaar and Schröder2012; Zhao et al., Reference Zhao, Zheng, Dong, Zhan, Wu, Guo, Hu, He, Zhang and Fan2013; Moss et al., Reference Moss, Leckie, Biggins, Poole, Baines and Kortland2014; Coates et al., Reference Coates, Ricca, Prochazka, Doherty, Brooks and Casazza2015; DeAngelis et al., Reference DeAngelis, Bryant, Liu, Gourely, Krebs and Reichardt2015; DeMay et al., Reference DeMay, Becker, Rachlow and Waits2017; Fauchald et al., Reference Fauchald, Park, Tømmervik, Myneni and Hausner2017). These demographic parameters could be coupled with the collection of excreta from animals they handle or track to quantify biomarkers of phytochemical exposure and consequences. For example, the moose (Alces alces) population in Sweden has been monitored in terms of relative density (number of individuals shot) since the mid-1900s. Since then, data on reproductive output (e.g. number of calves/adult female) and condition (carcass weights) of moose populations have been added to the official national database. There are also available data on the population trends of other species of herbivorous game in Sweden, such as roe deer (Capreolus capreolus), fallow deer (Dama dama), the European hare (Lepus timidus) and beaver (Castor fiber). This type of monitoring effort should begin to include collection of excreta from individuals that are handled or are collected from the habitat to quantify phytochemical exposure and physiological responses to those phytochemicals. In addition, researchers are encouraged to link physiological and demographic responses to the genetic expression and activity of host and microbial enzymes responsible for absorption and metabolism of ingested phytochemicals and digestive function (see Supplementary Material S1). While collection of tissues may be problematic in some species, this effort could be aided by collaborations with hunters who can offer access to tissues of game species for coupled genomic and physiological analysis.
Conclusions
Two key advances are needed to increase our capacity to predict variable demographic outcomes in vertebrate herbivores from complex diets. The first is to identify mechanistic responses to diets that are common across individuals in populations. For example, any animal that consumes and absorbs toxic alkaloids from plants has a high degree of probability for toxicosis (Cheeke, Reference Cheeke1989; Panter et al., Reference Panter, James and Gardner1999). The second is to identify mechanisms that explain why individuals within populations differ in their molecular expression, physiological processing of phytochemicals and physiological responses to phytochemical exposure. For example, why one individual has a higher intake of a given nutrient, faster rate of detoxification for a given toxin, or lower sensitivity to cellular toxicity than another individual, to such an extent that it results in differences in ecological or evolutionary dietary specialization (Shipley et al., Reference Shipley, Forbey and Moore2009). The PBMs described here can help increase our capacity to make initial physiological predictions about variable demographic outcomes associated with the degree of dietary specialization.
The immediate challenge for mechanistic models of ecology is to estimate physiological parameters among individuals, populations and species that differ in foraging strategies. Estimates of many physiological parameters can be independently assessed using a number of in vitro and in vivo studies (see examples in Supplementary Material S1). We stress that environmental conditions must be considered in both experimental studies, field observations, and in model parameterization and outputs. Ambient temperature can change nutritional demands of herbivores (e.g. metabolic costs, Hillebrand et al., Reference Hillebrand, Borer, Bracken, Cardinale, Cebrian, Cleland, Elser, Gruner, Harpole, Ngai, Sandin, Seabloom, Shurin, Smith and Smith2009; Smith-Ramesh et al., Reference Smith-Ramesh, Rosenblatt and Schmitz2017; Hristov et al., Reference Hristov, Degaetano, Rotz, Hoberg, Skinner, Felix, Li, Patterson, Roth, Hall, Ott, Baumgard, Staniar, Hulet, Dell, Brito and Hollinger2018) and the toxicity of ingested phytochemicals (Dearing, Reference Dearing2013; Connors et al., Reference Connors, Malenke and Dearing2017; Beale et al., Reference Beale, Marsh, Foley and Moore2018). As such, estimating biologically relevant physiological parameters requires knowledge of phytochemical intake within an environmental context, which is always challenging in wild herbivores. Inspiration can be drawn from primate (Felton et al., Reference Felton, Felton, Raubenheimer, Simpson, Foley, Wood, Wallis and Lindenmayer2009; Johnson et al., Reference Johnson, Raubenheimer, Rothman, Clarke and Swedell2013) and ungulate (McShea and Schwede, Reference McShea and Schwede1993; Parker et al., Reference Parker, Gillingham, Hanley and Robbins1996) research where the ability to habituate wild individuals to the presence of researchers provides the opportunity to determine detailed dietary intake from free-ranging animals. In addition, there are emerging opportunities for researchers to quantify intake of food through observations of animal-borne video (Hays, Reference Hays2015; Garnick et al., Reference Garnick, Barboza and Walker2018) that could be coupled with remote sensors that detect forage species and phytochemical concentrations (Stolter et al., Reference Stolter, Julkunen-Tiitto and Ganzhorn2006; Yule et al., Reference Yule, Pullanagari, Irwin, McVeagh, Kereszturi, White and Manning2015; Lim et al., Reference Lim, Kawamura, Yoshitoshi, Yayota and Ogura2017) as well as environmental conditions (Faye et al., Reference Faye, Rebaudo, Yánez-Cajo, Cauvy-Fraunié and Dangles2016; Cristóbal et al., Reference Cristóbal, Jiménez-Muñoz, Prakash, Mattar, Skoković and Sobrino2018) at the same spatial and temporal scales. The next challenge is to then develop mechanistic models that can accommodate known concentrations of phytochemicals in body compartments (PBMs), and resulting fitness consequences (GF), as well as genetic and metagenomic data (e.g. Zhao et al., Reference Zhao, Zheng, Dong, Zhan, Wu, Guo, Hu, He, Zhang and Fan2013; Alyass et al., Reference Alyass, Turcotte and Meyre2015; Simpson et al., Reference Simpson, Le Couteur, James, George, Gunton, Solon-Biet and Raubenheimer2017) and environmental conditions to validate how individuals and populations will respond to ingested phytochemicals under different climate scenarios.
There are no studies to our knowledge, outside of pharmacogenomic work in biomedical model organisms (Peters and McLeod, Reference Peters and McLeod2008; Santos et al., Reference Santos, Niemi, Hiratsuka, Kumondai, Ingelman-Sundberg, Lauschke and Rodríguez-Antona2018), that demonstrate how modeling phytochemical exposure and physiological function can predict individual responses to phytochemicals and help manage population dynamics. The described PBMs could help fill that gap. The coupling of a PBM, the GF and demographic models, along with advanced technology that allow us to better estimate behavioral, physiological and environmental parameters will increase our ability to link intake of phytochemicals by herbivores to demographic consequences. The genomic, metabolomic and modeling advances made in biomedicine have increased the predictive power and management tools needed to increase the health of humans. Ecologists should draw on these tools along with investment by state and federal agencies to monitor demographics of wild herbivores and the environment to better understand and manage the physiological mechanisms driving existing or desired demographic trends.
Acknowledgments
The authors thank Isabelle Cassar-Malek and René Baumont for their invitation to the 10th edition of the International Symposium on the Nutrition of Herbivores (ISNH) and providing a platform for synthesizing and sharing ideas within this manuscript. The authors thank D. Raubenheimer and S. Simpson for sharing their recent advances associated with the geometric framework of nutrition and two anonymous reviewers for valuable recommendations to improve the manuscript. J.S.F. was funded by the National Science Foundation (DEB-1146194, OIA-1826801). T.T.C. was funded by NSF SBE-1415297. M.D.M. was supported by NSF-IOS 1457209. M.D.D. was funded by NSF-IOS 1256383. A.M.F. was funded by the Swedish Environmental Protection Agency (13/274), the Södra Research Fund, and the Swedish Council for Sustainable Development (2016-01140-3). J.S.F. and A.M.F. equally conceived the manuscript. R.L. conceived the physiology-base models and outputs and T.T.C. conceived the demographic model outputs. J.S.F., R.L., T.C.C., M.D.M., J.A.V., K.D.K., M.D.D. and A.M.F. equally contributed to the integrating ideas across biological scales and writing.
Declaration of interest
All authors declare no conflicts of interest and no competing interests.
Ethics statement
Not applicable.
Software and data repository resources
None of the data were deposited in an official repository.
Supplementary materials
To view supplementary material for this article, please visit https://doi.org/10.1017/S1751731118002264