INTRODUCTION
London has the highest incidence of gonorrhoea in the UK [Reference Choudhury1], with particularly high rates in a few inner city boroughs and in certain socio-demographic groups, such as young adults, men who have sex with men (MSM), and black ethnic minority groups [Reference Hughes, Simms and Leong2, Reference Risley3].
Modelling theories suggest that a few core high-risk individuals in a few core areas play an important role in maintaining gonorrhoea transmission in the community [Reference Garnett and Anderson4, Reference Kretzschmar, van Duynhoven and Severijnen5]. Previous studies in urban areas in the USA and in England have highlighted the spatial clustering of gonorrhoea, with a few areas accounting for most of the disease burden, in keeping with the ‘core area’ theory [Reference Delpech6–Reference Potterat10]. This suggests that local targeted approaches to disease control and prevention might be more efficient than population-wide approaches.
It is likely that some of the spatial distribution of gonorrhoea reflects the geographical distribution of particular socio-demographic population groups in whom incidence is high. However, part of the spatial distribution may also be accounted for by factors not routinely captured through surveillance. These include particular sexual networks where behavioural and social factors, such as high partner turnover and unprotected sex, increase the risk for gonorrhoea transmission [Reference Rice11, Reference Ward12]. In addition, although individual risk factors are important determinants of sexually transmitted infection (STI) patterns, these are themselves influenced by wider social determinants of health, such as education, poverty or unemployment [Reference Law8, Reference Peterson13, Reference Sullivan14].
Recent studies have shown that most sexual networks, including those with a high prevalence of STIs, are formed by individuals living in close proximity to each other [Reference Zenilman9, Reference Jennings15]. A study in Baltimore used spatial scan techniques to detect core clusters of transmission, after adjusting for ethnicity [Reference Jennings7] and two other studies in North Carolina, USA found strong spatial clustering of gonorrhoea through spatial cluster detection techniques [Reference Law8] and Bayesian modelling [Reference Sullivan14]. A study on the epidemiology of gonorrhoea in London in 2004 showed some evidence of spatial clustering at the borough level but was not adjusted for age, ethnicity or other possible risk factors that may account for some of the clustering [Reference Risley3].
Since 2008 pseudonymized disaggregated data from genitourinary medicine (GUM) clinics in England have been reported to the Health Protection Agency through the GUM Clinical Activity Dataset (GUMCAD), an electronic surveillance system which collects data at the small geographical level, replacing the previous KC60 returns which only collected patient data at the clinic level [Reference Savage16]. Exploring the spatial distribution and clustering (correlation) of gonorrhoea at the small geographical level is therefore now possible.
Full Bayesian methods using Markov Chain Monte Carlo (MCMC) simulations have increasingly been used in the context of spatial analysis of infectious diseases particularly to account for highly multidimensional data, measurements errors and spatial correlation [Reference Dunson17]. Bayesian analysis in the analysis of small-scale data such as those reported through surveillance is particularly useful to account for the latter. Such approaches have recently been used to explore the spatial epidemiology of other infections, such as rotavirus in Berlin [Reference Wilking18], tuberculosis in urban Brazil [Reference Souza19] or global Plasmodium falciparum malaria incidence [Reference Hay20].
The aim of this study was therefore to identify particular areas and socio-demographic groups at high risk for gonorrhoea in London, UK, using a Bayesian spatial modelling approach. Specifically, we aimed to (i) explore the degree of spatial correlation of gonorrhoea at the small geographical level and (ii) identify socio-demographic risk factors for gonorrhoea at both the individual and geographical levels.
METHODS
Data sources
We extracted information on London residents diagnosed with microbiologically confirmed Neisseria gonorrhoeae infection and notified through GUMCAD between January 2009 and December 2010. These notifications included those from any of the 32 GUM clinics in London, as well as those from GUM clinics attended by London residents outside London. As we wanted to explore factors associated with first infection, episodes of repeat infection (defined as two episodes of gonorrhoea more than 6 weeks apart) were excluded from the main analysis. Information collected included area of residence, age, sex, sexual orientation, ethnic group and country of birth [21]. We obtained population denominator data by age, sex and ethnic group from the Office for National Statistics (ONS) [22]. Denominator data were obtained for each of the 983 Middle-layer Super Output Areas (MSOAs) in London, which are statistical geographical areas with a median population of 7200 individuals.
For each MSOA we obtained from the ONS the number of teenage pregnancies (all pregnancies in the 15–17 years age group using birth registrations and abortion notifications, excluding miscarriages or spontaneous abortions), the number of higher education students and the index of multiple deprivation (IMD). The IMD is an index combining information from seven indicators including income, employment, education, housing, health, crime and living environment [23].
The above-mentioned ecological covariates were chosen based on pragmatic considerations of the variables that would be meaningful for planning, development and delivery of health services for gonorrhoea, based on indicators which have previously been associated with either higher (IMD, teenage pregnancy) or lower (students) STI risk, although not necessarily for gonorrhoea. Hence we decided to explore the ecological associations between those variables and gonorrhoea in London.
A previous study on the epidemiology of gonorrhoea in London found no association with deprivation, using the Townsend index of deprivation. Gonorrhoea, however, as most other STIs, has been linked with deprivation in other settings, hence we wanted to explore this specific association based on comprehensive London-wide data. Individual-level associations between teenage pregnancies and STIs have been reported by others [Reference Ickovics24] and a previous study on Chlamydia suggests that students are generally at lower risk than the general population [25].
Analysis
Descriptive analysis
We first calculated the crude incidence rates of diagnosis by MSOA. We obtained the IMD score for each Lower-layer Super Output Area (LSOA), a lower geographical unit than the MSOA. We assigned a deprivation score to each MSOA nationally by calculating the mean IMD score based on the sum of IMD scores of each LSOA contained in an MSOA, then ranked IMD scores by MSOA and divided MSOAs according to their national quintile of deprivation. We grouped ethnicity into five broad categories of ethnic origin: white, black Caribbean, black African, South Asian (Bangladeshi, Pakistani, Indian) and other (including mixed race).
Bayesian analysis
A Bayesian mixed-effects multilevel Poisson regression model was built as described by Besag et al. [Reference Besag, York and Mollié26]. Details of the model and modelling approach can be found in the Supplementary online Appendix.
We first built a Poisson model in a stepwise fashion, and considered one after another each individual (age, sex, ethnicity) and ecological (IMD, teenage pregnancies, students) covariate. In a second step, we added two random effects; a spatially structured effect and an unstructured effect. These accounted for the variance not explained by the model covariates (i.e. the deviation from the expected incidence rates). The stronger the spatial correlation, the more variation is explained by the spatial random effect. In contrast, the unstructured random effect captures deviance from the model estimates with no spatial correlation. We compared models using the Deviance Information Criterion (DIC), a Bayesian equivalent of Akaike's Information Criterion [Reference Spiegelhalter27].
In Bayesian models prior distributions are assigned to all parameter covariates and combined with the likelihood of the data to obtain posterior distributions, i.e. the estimates, here being the incidence rate ratios (IRRs) of the model covariates, the random effects, and predicted incidence. We assigned vague, uninformative prior distributions for all parameters (i.e. no a priori information was taken into account). The priors of covariates were set with mean 0 and precision 0·0001 (variance = 10000), and standard deviations of the spatial and unstructured effects were both assigned a uniform distribution ranging from 0 to 10.
We obtained posterior distributions using a MCMC sampling algorithm implemented in WinBUGS (BUGS project, http://www.mrc-bsu.cam.ac.uk/bugs/winbugs/). Medians and 95% credible intervals (CrIs) were obtained on the basis of 20000 iterations after a 5000 iteration ‘burn-in’ period, with convergence assessed via the Gelman–Rubin diagnostic [Reference Gelman39]. Finally, we explored the relative contribution of random-effect variables and model covariates by exploring how much variance is explained by the covariates only and how much is explained by the random effects, in particular the spatial effect. This was achieved by examining the deviance of MSOA-level incidence from the grand mean and comparing the mean covariate contributions and mean contribution of the spatial effect.
We exported the results to Stata 11·0 Intercooled (StataCorp., USA) to produce maps to examine the geographical distribution of covariate levels and their contribution to predicted risk, and maps of spatial and unstructured effects to show areas where spatial aggregation of gonorrhoea is not explained by the covariates included in the model.
RESULTS
Descriptive epidemiology
A total of 12452 episodes of gonorrhoea were reported for London residents attending London GUM clinics over the 2-year period. The number of episodes in those aged 16–59 years was 5859 in 2009 and 6402 in 2010, which translates into an annual cumulative incidence rate of diagnosis in that age group of 115·6/100000 in 2009 and 132·4/100000 in 2010.
After excluding the 861 (6·9%) episodes of repeat infection, 11591 cases remained for the analysis. Of these, almost three-quarters (n = 8463, 73%) were males (Table 1). The median age was 27 years (range 13–78 years), higher in males than in females (median 28 years in males vs. 22 years in females, P < 0·001). More than 60% of the patients were in the 16–29 years age group (Table 1).
Table 1. Description of the study population by age, sex, sexual orientation and ethnicity

MSM, Men who have sex with men.
More than half (55·3%) were white, 15·0% black Caribbean, 7·0% black African, 3·6% South Asian and 19·1% were from other backgrounds, including mostly people from mixed white and black backgrounds (5·2%), other black backgrounds (5·5%), and any other Asian backgrounds (1·4%). A third (33·3%) were MSM, and MSM accounted for 45% of all men; for 13·1% the sexual orientation was unknown.
Figure 1 shows the incidence of gonorrhoea diagnoses per 1000 by MSOA over the 2-year period. Patients with missing LSOA codes (1·2%) were not included in this figure, or in the spatial model. These patients did not differ from the others in terms of age, sex or ethnic group.

Fig. 1 [colour online]. Chloropleth map of incidence rate (per 1000) of diagnosed gonorrhoea by MSOA in London over the 2-year period.
Analytical epidemiology
Several models were tested in the Bayesian framework. Table 2 summarizes the results of some of the main models explored. The best model included all covariates and two interaction terms (age × sex, ethnicity × sex), and included both an unstructured and a spatial random effect. The unstructured effect was negligible (σ U 0·02, 95% CrI 0·00–0·06), and most of the deviance from the expected incidence (i.e. the remaining MSOA-level variance) was thus explained by the spatial effect (σ S 0·61, 95% CrI 0·56–0·66). Individual and ecological covariates together accounted for nearly half (48%) of the MSOA-level variation. This was calculated from the ratio of the spatially structured random effect, σ S, which was 0·61, compared to the standard deviation of MSOA-averaged covariate effect, σ C, which was 0·56.
Table 2. Model output: comparison of the different Bayesian models tested
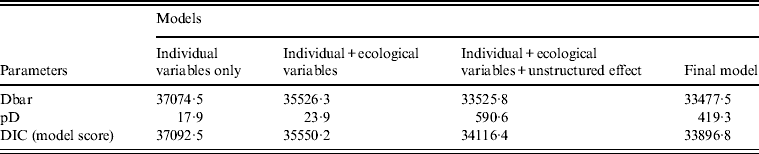
Dbar, Posterior mean of the deviance; pD, effective number of parameters; DIC, Deviation Information Criteria, which is equal to pD + Dbar (the best model has the smallest DIC).
The estimates of the adjusted cumulative IRRs and their 95% CrIs for the individual and ecological covariates are shown in Table 3. We found the highest cumulative incidence rates of diagnosis in the 16–29 years age group. There were marked variations across ethnic groups, with the highest rates found in black Caribbean, compared to whites, for males (IRR 2·61, 95% CrI 2·44–2·80) and for females (IRR 5·01, 95% CrI 4·13–6·04), and lowest rates in South Asians, compared to whites, for males (IRR 0·39, 95% CrI 0·35–0·44) and for females (IRR 0·55, 95% CrI 0·39–0·78).
Table 3. Final Bayesian Poisson model output: adjusted parameters [IRR (95% CrI)] for covariates and s.d. (95% CrI) of the random effects

IRR, Incidence rate ratio; CrI, credible interval; s.d., standard deviation.
We found a positive ecological association at the MSOA level between the rate of gonorrhoea diagnosis and the rate of teenage pregnancies and also the proportion of students. Finally, there was a near linear association between the rate of gonorrhoea diagnosis and deprivation at the MSOA level (Table 3).
Figure 2 a shows a plot of the IRR adjusted for all covariates and Figure 2 b shows a plot of the spatial effect only. Figure 2 b overlaps mostly with the covariates-only model, but also shows some areas of spatial dependence not explained by the covariates, in particular in western and southern parts of London. Supplementary Figure S2c (online) shows a plot of the unstructured effect only. The specific covariate effects can be found in Supplementary Figure S3a–c (online), which display individual maps of specific model covariates, namely age and sex (Fig. S3a), ethnicity (Fig. S3b) and IMD (Fig. S3c).

Fig. 2. Plot by Middle-layer Super Output Area (MSOA) (statistical geographical area with a median population size of ≈7200) of the incidence rate ratio (IRR) for gonorrhoea by MSOA of residence, as predicted by (a) model covariates only; (b) the spatial effect only, London, 2009–2010.
Given that sexual orientation could not be included as a variable in the model, we ran a model excluding all MSM diagnosed with gonorrhoea in order to assess the contribution of MSM to the spatial effect. This model included all covariates but had no interaction term between sex and ethnicity. The spatial effect was equally strong (σ S 0·60) and the unstructured effect equally negligible (σ U 0·09).
DISCUSSION
This study of gonorrhoea risk factors in London was based on comprehensive disaggregate London-wide surveillance data. We found marked differences in the burden of gonorrhoea across socio-demographic groups and found that a few areas accounted for most of the gonorrhoea cases diagnosed. One of the strengths of our approach was to account for a spatial effect not explained by model covariates, and to adjust our estimates for multiple individual- and area-level covariates at the small geographical level. This enabled us to identify possible ‘core’ areas and ‘core groups’ at high risk.
Our results concur with those from previous studies in the USA, which found strong evidence of spatial clustering of gonorrhoea [Reference Jennings7, Reference Law8, Reference Sullivan14]. A study in North Carolina, USA, found spatial effect at the census level – of similar size to MSOAs – after adjusting for other factors such as age, sex, ethnicity and deprivation [Reference Sullivan14].
The spatial effect captures an unmeasured variable, or a set of unmeasured variables distributed spatially. We believe – although we have no proof of this – that these capture local densely connected sexual networks with high rates of gonorrhoea transmission [Reference Ward12]. Because sexual orientation was not included in the model, there is a possibility that the spatial effect accounted for areas with large populations of MSM and associated social and sexual networks. However, the spatial effect in the full model best matched that of the model excluding MSM, suggesting that spatial clustering also reflects the distribution of pockets of highly active heterosexual sexual networks as well as those of MSM. Risley et al. [Reference Risley3] explored the spatial correlation of identical molecular gonorrhoea strains in London on a sample of patients attending GUM clinics. They found that out of 21 common strains, which accounted for about half the patients, eight were spatially clustered, of which six strains were found in heterosexual networks, and two in MSM networks.
The hypothesis that most of the spatial effect captures local sexual and social networks with high gonorrhoea transmission is in line with the modelling theories of ‘core transmission’. Pockets of transmission are probably sustained by assortative mixing in sexual networks with a high prevalence of concurrent partnerships, short gaps between partnerships and high rates of re-infection [Reference Garnett and Anderson4, Reference Chen and Ghani28, Reference Morris29]. Concurrency and short gaps between partnerships are more common in the population subgroups at higher risk of gonorrhoea identified in this and other studies [Reference Chen and Ghani28].
Most of the individual- and area-level risk factors found were not unexpected. Age differences in the risk of STIs, including gonorrhoea, have been well documented by others [Reference Hughes, Simms and Leong2, Reference Risley3, Reference Delpech6, Reference Jennings7, Reference Fenton30] and result from age-related differences in sexual behaviour, such as condom use, and the stability of relationships [Reference Delpech6, Reference Chen and Ghani28, Reference Fenton30]. The lower incidence rate in females most likely reflects both the higher incidence in MSM compared to heterosexual men as well as possible differences in gonorrhoea symptomatology, with fewer women developing acute symptoms than men [Reference Delpech6, 31]. The interaction between age and sex in our model probably reflects younger sexual debut in women, age-discordant heterosexual partnerships, and greater sexual activity in MSM in older age groups [Reference Fenton30].
Higher rates in black ethnic minority groups have consistently been described in studies in the UK and elsewhere [Reference Risley3, Reference Rice11, Reference Sullivan14, Reference Kaplan32, Reference Lacey33]. This study found a higher risk in black Caribbeans than in black Africans, a difference not highlighted in a previous London study where black ethnic groups were combined [Reference Risley3]. Cultural differences in sexual behaviour including condom use and partner concurrency may contribute to higher risk in some populations [Reference Chen and Ghani28, 31]. The observed interaction between ethnicity and sex in the model may reflect higher incidence of gonorrhoea in MSM, who were predominantly white. This is supported by the heterosexual-only model, which had no interaction term with ethnicity.
Interestingly, we found an almost linear increase in gonorrhoea incidence with increasing quintiles of deprivation, after adjusting for individual- and area-level factors. Although a few studies in other cities in the UK and the USA have shown a link with deprivation [Reference Rice11, Reference Peterson13, Reference Sullivan14, Reference Lacey33, Reference Fichtenberg34], our findings contrast with those of Risley et al. [Reference Risley3], who found no association with deprivation at the borough level in London, using the Townsend deprivation score. This may be due to differences in the geographical level used (borough vs. MSOA), given the wide socioeconomic disparities of gonorrhoea within boroughs. It is also possible that the Townsend score, which includes car ownership as one of its four indicators, may not correlate with socioeconomic deprivation as well as the IMD score in a city like London, where socioeconomic status may not be reflected by car ownership.
Various factors may explain the link with deprivation, although it is unclear to what extent these contribute to gonorrhoea transmission. A study in San Francisco, USA, found that the structure of sexual networks was strongly associated with poverty, as people from the poorest areas tended to be part of large sexual networks, whereas those from affluent backgrounds were more often in stable, monogamous relationships [Reference Fichtenberg34]. Other factors could also explain the association with deprivation. Although GUM services are free of charge, intangible barriers such as travel costs, language barriers, or knowledge about the services may hamper timely and adequate access. Other factors such as low education and poor knowledge about STIs and their prevention might contribute to gonorrhoea transmission in most deprived areas [Reference Annang35]. It is also possible that a higher prevalence of depression and mental health issues in more deprived areas, which can result in riskier sexual behaviour [Reference Pratt36], increases the risk of gonorrhoea transmission in these areas. Further research into the causal effect of deprivation on gonorrhoea is warranted.
Although ecological, the positive association between rates of gonorrhoea diagnosis and teenage pregnancy at the MSOA level is consistent with other studies reporting individual-level associations [Reference Ickovics24]. This emphasizes the need for effective, integrated sexual health services offering STI testing and management, contraception, and comprehensive sexual health promotion, interventions and advice. Studies have also shown that postpartum teenagers remain highly vulnerable to STIs [Reference Ickovics24] and STI screening and sexual health promotion messages should also be delivered during postnatal care of postpartum adolescents.
Perhaps more surprising is the small but significant ecological association between the rate of gonorrhoea and the proportion of students living in a particular MSOA. Little is known about the incidence of gonorrhoea in students in the UK, but previous studies on Chlamydia suggest that students are generally at lower risk than the general population [25]. Further studies should investigate whether the association found here truly reflects a higher rate of gonorrhoea in students, or whether it is due to confounding.
Overall, our study findings suggest that the strengthening of control and prevention strategies targeting specific high-risk localities and population groups could deliver significant reductions in gonorrhoea transmission. Strategies could include improved outreach through community-based STI screening programmes, in particular in non-GUM settings and outside healthcare premises (e.g. clubs, bars, saunas, gyms) offering testing to those who do not attend GUM clinics or those who are asymptomatic, alongside intensified health promotion materials and the use of social venues to encourage testing. Within the GUM clinic setting, one-to-one risk reduction counselling and effective partner notification and contact tracing, particularly focusing on casual partners, could have an even greater impact on infection control and public health [Reference Mercer37, 38]. In addition to individual-centred strategies, the link with deprivation is a call to strengthen more global approaches to tackle wider social determinants of health and reduce inequalities.
Limitations
Our study has a number of limitations. The analysis was based on surveillance data from GUM clinics but not from primary care, where an estimated 4% of all gonorrhoea diagnoses are made in London [31]. In addition, we did not capture asymptomatic cases not identified through partner notification. Our estimates of diagnosis incidence are therefore conservative and some degree of bias may have occurred if primary-care diagnoses vary by area and socio-demographic group. In particular, diagnosis estimates in women are likely to underestimate the true incidence of infection more than in men, given the higher proportion of asymptomatic infection in women [31].
We were unable to account for sexual orientation in our analysis, given the lack of a valid population denominator that includes sexual orientation. We also used broad categories for ethnic groups, which overlooks differences in sexual risk, religion, culture and health-seeking behaviour within each of these groups [Reference Fenton30]. Moreover, we were unable to explore behavioural factors such as condom use, number and types of sexual partnerships and health-seeking behaviour, all of which have been shown to be associated with STIs [Reference Fenton30] and will partly explain differences found across areas, age, gender and ethnic groups.
Given the ecological nature of some of the associations (such as IMD, teenage pregnancies, students), those findings need to be interpreted with care and cannot be translated into individual-level associations as such (ecological fallacy).
Finally, the spatial analysis was based only on the area of residence of cases. Sexual networks connected through the workplace rather than place of residence could have appeared geographically disconnected in our study.
SUPPLEMENTARY MATERIAL
For supplementary material accompanying this paper visit http://dx.doi.org/10.1017/S0950268813000745.
ACKNOWLEDGEMENTS
Data were obtained from the Genitourinary Medicine Clinic Activity Dataset (GUMCAD), compiled by the Health Protection Agency (HPA). The authors are grateful to all GUM clinic staff who report GUMCAD data to the HPA. We also thank Helen Maguire for her scientific advice during the preparation phase of this study. Finally, we thank the reviewers for their helpful comments.
DECLARATION OF INTEREST
None.