Introduction
Coronavirus disease 2019 (COVID-19) is an infectious disease caused by severe acute respiratory syndrome coronavirus 2 (SARS-CoV-2) that was first identified in December 2019 in Wuhan, China and has since spread globally, causing a pandemic [1]. Brazil surpassed 190 000 people killed by COVID-19 in 2020, with a total of 7 447 625 people infected since the beginning of the pandemic. These facts mean that the country is ranked in second place in terms of the highest incidence of deaths caused by COVID-19, being surpassed only by the United States of America, which accounts for more than 330 000 deaths [2].
According to Treweek et al. [Reference Treweek3], the COVID-19 toll is not the same for everyone. Some studies show global evidence that elderly people, males, those with co-morbidities and those in poverty are at a disproportionate risk of serious complications and death from COVID-19 [Reference Khunti4, Reference Haynes, Cooper and Albert5]. Groups of ethnic minorities with these factors have shown worse clinical results, including higher mortality and a higher rate of admission to intensive care units [Reference Sze6].
In Brazil, the indigenous tribes have already seen their communities ravaged by exogenous diseases in the past. During the 2009 H1N1 influenza pandemic, their death rate was 4.5 times higher than among the rest of Brazil' general population [Reference La Ruche7]. Even with the creation of the Emergency Plan to Combat COVID-19 in Indigenous Lands, through Law No. 14201, of 7 June 2020 [8], at the height of the pandemic that occurred in July 2020, mortality among indigenous peoples reached 6.5 times greater than in the rest of the population of Brazil [9, 10].
In view of the failure to implement a reactive Emergency Plan that ignored the peculiarities already evidenced by other calamities that have decimated indigenous peoples throughout history, it is necessary to search for new strategies capable of assisting in the decision-making process to combat COVID-19 in indigenous lands.
Spatial analysis technologies have stood out in the context of the current pandemic for helping to understand the spatial and temporal dynamics of COVID-19, which has been instrumental in mitigating them. Recognising the disease as a potentially mappable geographical phenomenon enables strategic planning and the direction of actions, as well as the formulation of more appropriate scientific and political conducts [Reference Franch-Pardo11, Reference Rex, Borges and Käfer12].
Studies carried out in the United States of America that used space–time scanning analysis proved to be effective in identifying space–time clusters of risk for COVID-19. In addition, the results were particularly useful for identifying active and emerging clusters, which represent valuable information for disease surveillance and for formulating faster responses to the pandemic [Reference Hohl13, Reference Desjardins, Hohl and Delmelle14].
Although geospatial tools are a strategic device, in terms of understanding situational diagnosis and monitoring disease progression, studies using these tools in the context of the indigenous population are still scarce [Reference Franch-Pardo11, Reference Weiss, Jellingsø and Sommer15].
Thus, considering the high degree of vulnerability of the indigenous population and the urgent need to identify priority territories, this study aimed to analyse the geographical distribution of COVID-19 and to identify high-risk areas in space and time for the occurrence of cases of, and death from, the disease in the indigenous population of Brazil.
Method
Study design and observation units
This is an ecological study using spatial analysis techniques [Reference Rothman, Greenland and Lash16], the units of which were the Special Indigenous Health Districts (SIHDs).
Description of the study area and population
In Brazil, the self-declared indigenous population is estimated to be 817 000 individuals, which represents 0.4% of the country' total population. Adding together all the peoples who live on indigenous lands and have declared themselves to be of another colour or race, the total indigenous population residing in the national territory is now 896 900 people. There are approximately 305 ethnicities and 274 languages spoken, representing one of the highest levels of sociodiversity in the world [17].
The indigenous health subsystem in Brazil is organised into 34 SIHDs and these areas are strategically divided by territorial criteria, based on the geographical occupation of indigenous communities. Its service structure has Indigenous Basic Health Units (IBHUs), base centres and Indigenous Health Support Houses (IHSHs) [18]. Figure 1 exhibits the location of each SIHD.
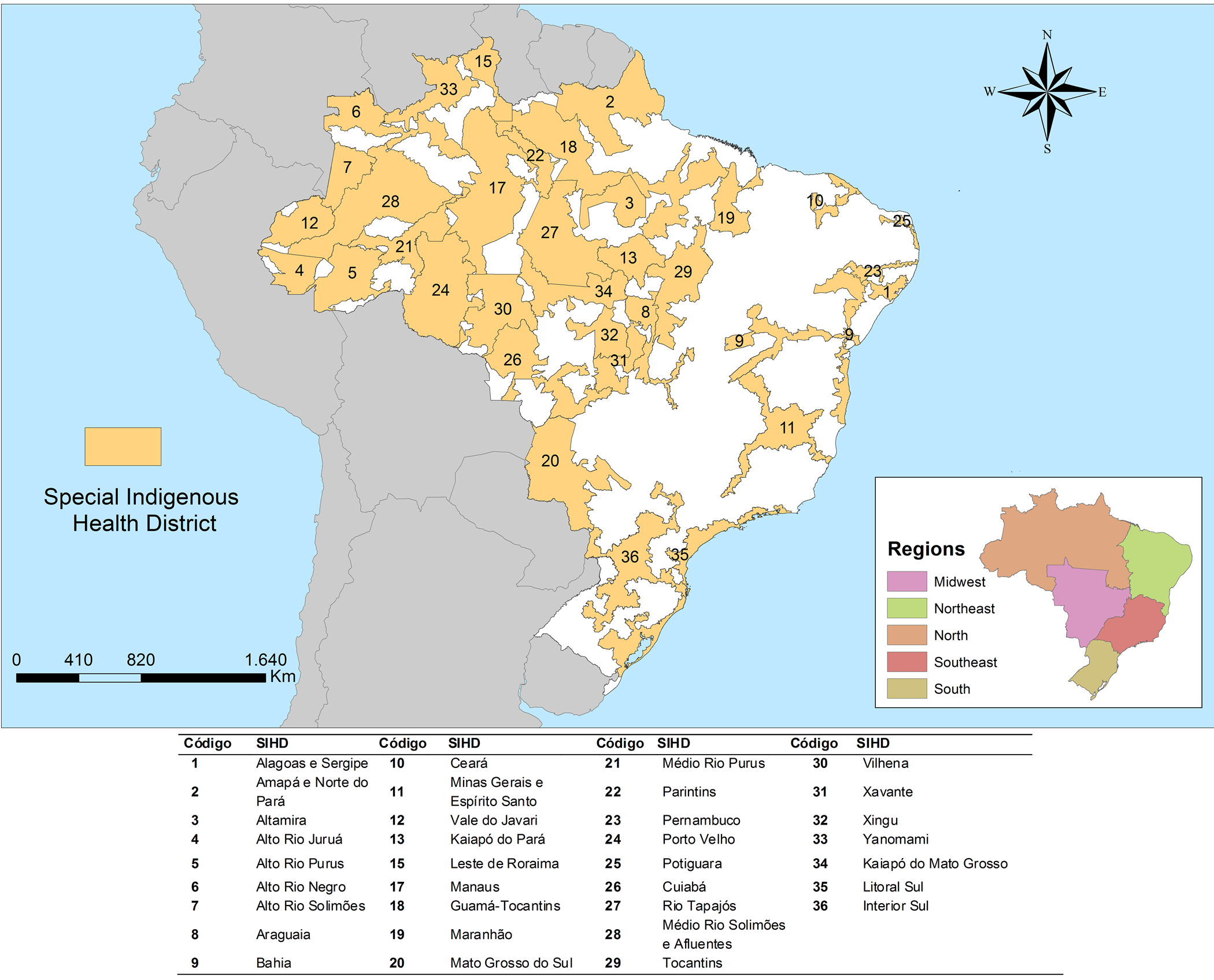
Fig. 1. Map of Special Indigenous Health Districts and their geographical location. Brazil, 2020.
The study population consisted of cases with confirmed diagnosis of, and death by, COVID-19 reported in the Brazilian indigenous population from 24 March to 26 October 2020. It is worth noting that confirmed cases are characterised as cases with a positive result by laboratory confirmation or confirmed by clinical, clinical−epidemiological and clinical−image criteria [10].
Study information sources
The study used the free-access epidemiological bulletins published by the Special Secretariat for Indigenous Health (https://saudeindigena.saude.gov.br/corona), the institution responsible for coordinating and implementing the National Health Care Policy on Indigenous Peoples in the national territory. The data refer to the situation of the coronavirus in indigenous people served by the Indigenous Health Care Subsystem in each of the 34 SIHDs [10].
Data analysis
First, we conducted an exploratory analysis of the cases and deaths that occurred in the period, calculating the absolute number and the incidence and mortality rates according to each month investigated. Then, the same calculations were performed according to sex and age group. To calculate the incidence and mortality rates, the ratio between the total number of cases or deaths that occurred during the study period and the total indigenous population was used. The result was subsequently multiplied by 100 000. The same rates were calculated by SIHDs, using the number of cases or deaths recorded in each SIHD and the indigenous population of each.
Spatial analysis
To verify the spatial association between the incidence and the rates of mortality by COVID-19, the Getis-Ord General G and Getis-Ord Gi* techniques were used [Reference Getis A19]. The Incremental Spatial Autocorrelation tool in the ArcGIS® software version 10.6 was used to obtain the best distance at which the spatial clusters were most pronounced [20].
The High/Low Clustering (Getis-Ord General G) statistic tool gives a general analysis of the area. The null hypothesis states that there is no spatial clustering of feature values. When the P-value returned by this tool is small and statistically significant, the null hypothesis can be rejected. If the null hypothesis is rejected, the sign of the z-score becomes important, with values of ± 3 representing a 99% confidence level. If the z-score value is positive, the observed General G index is larger than the expected General G index, indicating that high values for the attribute are clustered in the study area. If the z-score value is negative, the observed General G index is smaller than the expected index, indicating that lower values are clustered in the study area [21].
To examine spatial patterns in detail, the hot-spot technique (Getis-Ord Gi*) was used, which analyses the spatial association locally, based on a spatial concentration indicator. In Getis-Ord Gi* the values for each locality, that is, each SIHD, are considered from a neighbourhood matrix. In this technique, statistically significant spatial clusters of high values (hotspot) and low values (cold spot) are identified [Reference Getis A19].
This analysis also generates a z-score for statistically significant SIHDs. The higher the z-score, the more intense the grouping of high values (hotspot), while the lower the value, the more intense is the grouping of low values or the fewer the occurrences of the event (coldspot). In addition to the z-score, the P-value and significance level (Gi-Bin) are provided [Reference Getis A19].
To identify high-risk space–time clusters for cases of and deaths from COVID-19, retrospective space–time scan was performed. This method is defined by a cylindrical window whose circular base represents geographic space and its height represents time. The cylindrical window is then moved, in order to identify clusters that may occur in space and time simultaneously. In a retrospective analysis, the analysis is done only once for a fixed geographical region and a fixed study period. SaTScan® scans over multiple start dates and end dates, evaluating both ‘alive clusters’, lasting until the study period and date, as well as ‘historic clusters’ that ceased to exist before the study period end date [Reference Kulldorff22].
Thus, the expected and observed numbers of cases and deaths are calculated by COVID-19 for each cylinder and a likelihood ratio (LR) test is used to identify scanning windows with a high risk of the studied phenomena. The null hypothesis considered was that there is no difference in the risk of occurrence of cases and deaths from COVID-19 between the inside and outside of the cylinder. The alternative hypothesis is that there is a greater risk of these events occurring inside the cylinder. Thus, the LR is a measure of how the risk within a cylinder differs from the external risk and its logarithmic transformation is reported as a logarithmic likelihood ratio (LLR) [Reference Kulldorff22].
In addition, the method provides the relative risk (RR), which is a value that represents how much a location and time period are more or less susceptible to having the presence of the event. Then a general RR was calculated for each reported cluster and an RR for each SIHD within the cluster [21]
For the analysis of this study, the following criteria were adopted: identification of retrospective high-risk space−time clusters, with aggregation of time in days, use of the discrete Poisson model and 999 Monte Carlo replications. In addition, each possible risk group must contain at least five cases or five deaths and have a minimum duration of 2 days. These criteria were established according to studies already carried out that used similar methods for COVID-19 [Reference Desjardins, Hohl and Delmelle14, Reference Hohl, Delmelle and Desjardins23].
The spatial scan window was restricted to include 8% and 4% of the population at risk, for the analysis of cases and deaths, respectively. To define the exact nature of the scan window in relation to space, a Gini index was used. With this criterion, a group of non-overlapping clusters is selected that maximises the Gini index, so that there is a big difference in rates between the areas of clusters and non-clusters, thus allowing the identification of a more refined collection of non-overlapping clusters [Reference Kulldorff22, Reference Han24]. The maximum size of the temporal cluster was increased to 50% of the study period, which is the standard value recommended by Kulldorf [Reference Kulldorff22]. A value of P < 0.05 was adopted for statistically significant clusters.
Software
The tabulation and descriptive analysis of the data set used spreadsheet software. SaTScan® software version 9.6 was used for the spatial scan statistic technique. The spatial association and the construction of maps were performed using ArcGIS® software version 10.6.
Ethical considerations
Because it is research with secondary data of public access using data in an aggregated form and without the nominal identification of the subjects, permission from a Research Ethics Committee was waived, according to the National Health Council Resolution 510/2016.
Results
During the study period, 32 041 cases of COVID-19 and 471 deaths were reported. Women represented 52.10% (n = 16 686) of reported, and 49.29% (n = 15 792) of cases were in the 20–49 age group. With regard to deaths, 66.24% (n = 312) occurred among men and the most affected age groups were 50- to 79-year-old (50.11%; n = 236) and aged over 80 years (34.18%; n = 161). When analysing the incidence and mortality rates, we show that both cases and deaths increase with the age of the indigenous population, regardless of gender (Fig. 2).

Fig. 2. Distribution of the incidence rate (a) and mortality rate (b) by COVID-19 among the indigenous population according to sex and age group. Brazil, 2020.
In Figure 3(a, b), we present the temporal evolution of cases of and deaths by COVID-19 among the indigenous population and their respective population rates per 100 000 inhabitants. We observed that the number of cases and deaths has been increasing since the beginning of the pandemic in March 2020, with the peak occurring after 5 months, in July 2020.

Fig. 3. Temporal evolution of cases (a) and deaths (b) by COVID-19 among the indigenous population and their respective population rates per 100 thousand inhabitants. Brazil, 2020.
The distribution of incidence rates and mortality rates of COVID-19 for each SIHD are presented in Figure 4a and b, respectively. When analysing the incidence of the disease, it was found that the incidence rate varied between 758.14 and 18 530.56 cases per 100 000 inhabitants, with the SIHDs located in the midwest and north regions being most affected. The SIHDs with the highest incidence were: Kaiapó do Pará, Altamira, Cuiabá, Kaiapó do Mato Grosso and Rio Tapajós. In relation to mortality, the rate varied between 5.96 and 265.37 deaths per 100 000 inhabitants. The north, midwest and south regions had the highest rates. The SIHDs with the highest mortality rates were: Cuiabá, Vilhena, Xavante, Xingu and Kaiapó do Pará.

Fig. 4. Distribution of incidence (a) and mortality (b) rates to COVID-19 in Special Indigenous Health District. Brazil, 2020.
The G Getis-Ord technique presented in Figure 5a, points to a z-score of 5.40 whose pseudo-significance test value was P < 0.001, which shows the non-randomness of COVID-19 cases, since the distribution high spatial values in the data set are spatially more grouped than expected indicating the formation of clusters. Figure 5b shows the local spatial association of the COVID-19 incidence rate, using the Getis-Ord Gi* technique, which allowed the identification of hotspots formed by SIHDs located in the midwest and north regions of Brazil, with confidence levels of 90 and 99%. The areas with the largest group of high values for the incidence of the disease are in the hot spots.

Fig. 5. Hotspots and coldspots for COVID-19 cases in Special Indigenous Sanitary Districts. Brazil, 2020. (a) Level of statistical significance of Getis-Ord G for COVID-19 cases. (b) Spatial clusters of COVID-19 cases according to the level of confidence.
Figure 6a shows the result of the Getis-Ord General G technique (z-score = 3.83; P < 0.001), which indicates the non-randomness of deaths by COVID-19 and the presence of spatial clusters. The Getis-Ord Gi* technique showed hotspots with confidence levels of 90, 95 and 99% concentrated mainly in the midwest region of the country.

Fig. 6. Hotspots and coldspots for COVID-19 deaths in Special Indigenous Sanitary Districts. Brazil, 2020. (a) Level of statistical significance of Getis-Ord G for COVID-19 cases. (b) Spatial clusters of COVID-19 deaths according to the confidence level.
In the northeast region of the country, coldspots were identified, which are groups with low values, both for the occurrence of cases (Fig. 5b) and deaths (Fig. 6b) by COVID-19.
When applying the space-time scan statistic, 16 high-risk clusters were identified in relation to the occurrence of COVID-19 cases that were statistically significant. In total, 23 SIHDs were part of the risk areas and were spread across all regions of the country, mainly in the north and midwest regions. Figure 7 shows the high-risk clusters with RR values for the entire cluster as well as the local RR in each SIHDs.
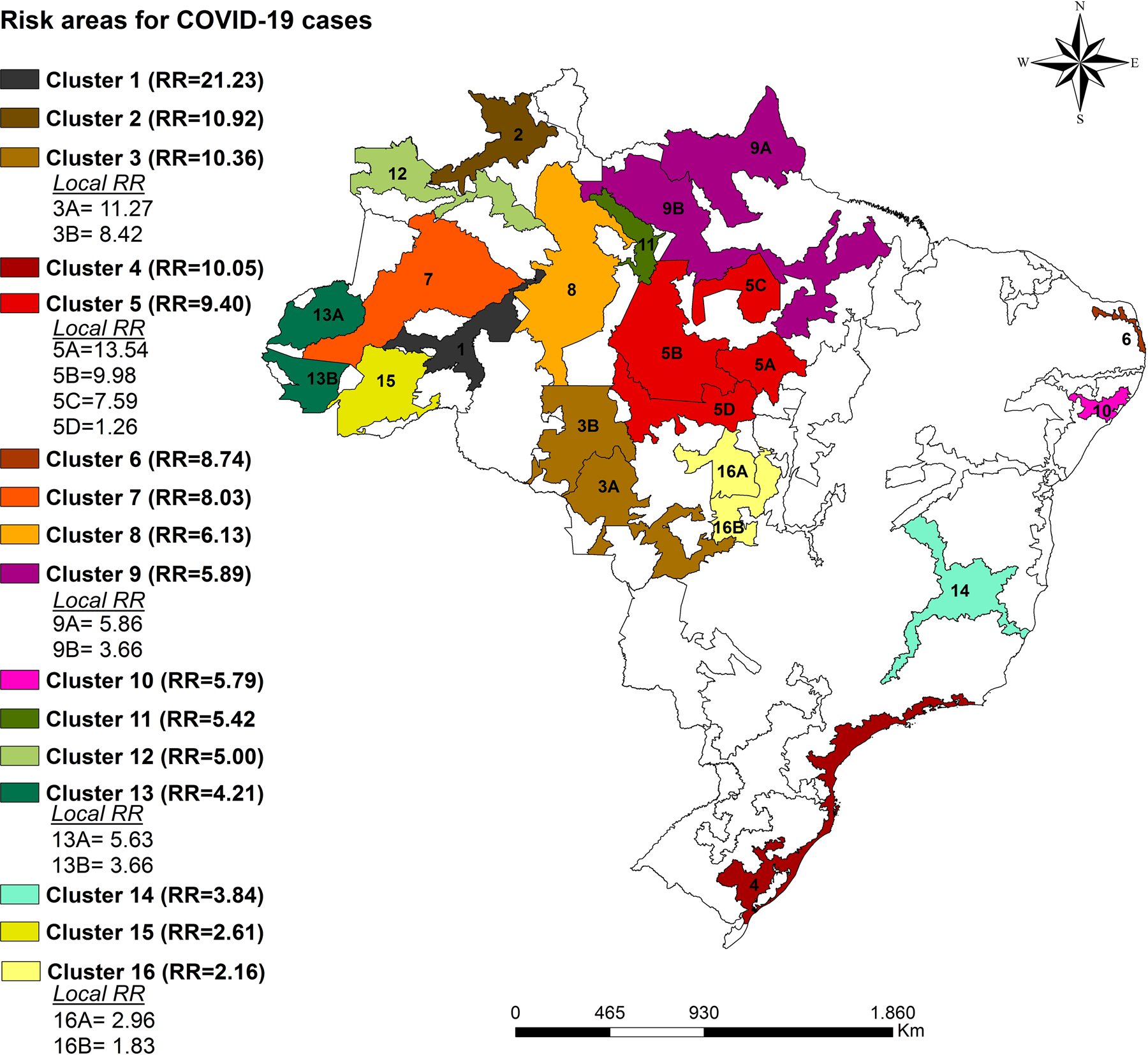
Fig. 7. Space−time clusters for the incidence of COVID-19 in the indigenous population according to a Special Indigenous Health District. Brazil, 2020.
Cluster one was the one with the highest relative risk, which is formed by the SIHD Médio Rio Purus. The population at risk in this cluster comprised 10 721 indigenous people, the total number of cases was 88 and the RR was 21.23 (P < 0.001), which indicates that indigenous people living in this cluster are 21.23 times more likely to become infected with COVID-19 compared to those who do not live in these locations. The cluster with the largest population at risk was cluster nine (Amapá/Norte do Pará and Guamá-Tocantins), whose population at risk was composed of 30 162 indigenous people with 1899 recorded cases and RR = 5.89. The characteristics of the identified space–time risk clusters are specified in Table 1.
Table 1. Characteristics of space−time clusters for the occurrence of cases of COVID-19 in the indigenous population. Brazil, 2020

LLR, logarithmic likelihood ratio.
Four high-risk space−time clusters have been identified regarding COVID-19 mortality and are located in the north and midwest regions of the country (Fig. 8). Cluster one includes the SIHD Rio Tapajós and presents an RR = 80.33 (P < 0.001) with eight registered deaths. The SIHDs from Cuiabá and Xavante also had high local RR with RR = 11.63 and RR = 11.46, respectively. In total, the four clusters have a population of 91 275 indigenous people at risk of mortality from COVID-19 (Table 2).

Fig. 8. Space−time clusters for the mortality due of COVID-19 in the indigenous population according to the special indigenous health district. Brazil, 2020.
Table 2. Characteristics of space−time clusters for COVID-19 mortality in the indigenous population. Brazil, 2020

LLR, logarithmic likelihood ratio.
It is worth noting that the clusters identified for both the occurrence of cases and deaths started on 22 May and remained active until 10 October 2020.
Discussion
This study sought to verify the spatial distribution of COVID-19 in the indigenous population of Brazil and to identify high-risk space−time areas for the occurrence of cases of and deaths from the disease. The results demonstrated that COVID-19 is present in all SIHDs in the national territory and that there are high-risk space−time clusters of the investigated events, mainly in the midwest and north regions of the country.
One of the limitations of this study is the use of secondary sources of information, which does not exclude the possibility of incomplete data. However, the information was obtained from each of the 34 SIHDs and, afterwards, was validated by the Department of Attention to Indigenous Health [10]. Another issue is that the data provided by the Ministry of Health are aggregated by SIHDs, which include several ethnic groups and villages within the same region. The provision of aggregated data in smaller geographical units would allow a more detailed analysis, with the inclusion of variables related to the sociocultural diversity and health determinants of these communities. Finally, we do not have individual sex and age variables for each SIHD, as well as the number of diagnostic tests applied for COVID-19 to perform adjusted analyses. The possibility of under-reporting COVID-19 cases should also be considered.
Admittedly, the indigenous population has a high degree of vulnerability to both COVID-19 and other diseases, and our study showed that this population suffers strongly from the impacts of this worldwide pandemic, since it has indicators that far exceed those of the general population.
The results showed that the cases of and deaths by COVID-19 peaked in July, and increased even in the face of the contingency plans launched by the government [25]. The incidence and mortality rates in the SIHDs for the period studied reached 18 530.56 cases per 100 000 people and 265.37 deaths per 100 000 people, respectively, significantly exceeding the national rates registered in July, which were 1139.4 cases per 100 000 people and 41.1 deaths per 100 000 people [9, 10].
Most of the deaths investigated occurred in males, following the worldwide trend, in which the majority of deaths occurred in the elderly male population [2]. Our findings also showed that incidence and mortality rates increase with the aging of the indigenous population, regardless of gender, which is in line with data from the general population of Brazil [9].
In order to better understand the impacts of the COVID-19 pandemic, it is necessary to consider some questions about vulnerabilities present in the Brazilian indigenous population. Firstly, these individuals already have a higher prevalence of infectious diseases, respiratory diseases, chronic diseases such as diabetes and hypertension, in addition to high rates of malnutrition and obesity [26]. Secondly, there is the social issue related to the source of income, with indigenous peoples being among those with the lowest income in the country [17]. Thirdly, it should be noted that the indigenous population has many sociocultural specificities, which influence their relationship with the environment in which they live, their way of life and also the health–disease process. An example of this is the high number of residents per household, the sharing of personal utensils, and collective ceremonies such as weddings and funerals [Reference Santos, Pontes and Coimbra27].
Fourthly, we highlight territorial vulnerability. A large part of the indigenous population suffers from the devastation of their lands due to the lack of demarcation, illegal invasion, mining and deforestation [Reference Ramos and Dos Santos Rodrigues28]. Finally, it is necessary to draw attention to the precarious health structures, lack of supplies, equipment, lack of training and high turnover of professionals, the difficulty in removing patients who need assistance in medium- and high-complexity systems. In addition, healthcare is still focused on palliative and emergency practices, due to the lack of strengthening of primary care [Reference Garnelo and Pontes29].
These issues became even more evident with the arrival of the COVID-19 pandemic and certainly contribute to the high rates of disease incidence and mortality among this population. The results of this study showed that there are clusters of risk for both the occurrence of cases and deaths, which suggests that there are different degrees of vulnerability to COVID-19 among indigenous territories.
Azevedo et al. [Reference Azevedo30] constructed an index of demographic and infrastructural vulnerability of indigenous lands to COVID-19, which included variables related to the number of elderly people, number of residents per household, sanitary conditions of the household, proximity to places with availability of treatment beds, and intensive and land tenure regularisation of the indigenous land. The index varies between zero and one – the closer to one the greater the vulnerability. The SIHD with critical levels of vulnerability (highest level) were: Alto Rio Negro, Yanomami, Xavante, Xingu, Kaiapó do Pará and Rio Tapajós. Coincidentally, these SIHDs are part of the risk areas identified in our study. The SIHD in the northeast and south of the country have a lower vulnerability index compared to the others, which corroborates the results of our study, which identified coldspots in these locations using the Gi* analysis.
Two Brazilian regions stood out in the spatial analysis carried out in this study, the north and midwest regions. The north region has a larger indigenous population compared to other regions. The state of Amazonas, which has the largest number of indigenous people in the country, is in this region [17]. In addition, most of the SIHDs found to be at high-risk of coronavirus infection in this study are located in this region.
Some regional specificities are challenging for the provision of health care to the indigenous population of the north region, such as access, which is only possible by air or river to several villages as they are affected by seasonal influences, especially during periods of drought in the rivers, increasing the travel time and late delivery of inputs. Work difficulties in these areas involve health risks, language barriers and poor housing conditions, leading to high professional turnover in the most remote regions [Reference Borges, da Silva and Koifman31].
The study by Silva et al. [Reference Silva32] points out the main problems related to indigenous health in the Amazon region: scheduling appointments; long waiting times for hospital procedures (consultations, examinations and surgeries); financial difficulty in buying medicines or having private consultations and examinations, and the precarious physical structure of the services.
Another factor that may be favouring the transmission of COVID-19 in the north region is the number of residents per household, with households with up to 41 residents, approximately double that found in the south and southeast regions. In addition, the indigenous population of the north region suffers from a high level of illiteracy, low income and poor sanitary conditions [17, 26].
The midwest region had a high incidence of COVID-19 and was the region with the second highest number of inhabitants at risk of the disease. The midwest has also drawn attention nationally because it is an area with high mortality due to COVID-19. Our results showed that the two high-risk death clusters that were identified belong to this region. Among the five SIHDs that were part of these clusters, the SIHD Xavante stands out it is the most populous in the midwest region and is located in the state of Mato Grosso. This SIHD differs from the others in that it is composed of only one ethnic group, totalling more than 21 000 individuals [18].
In the month of July, which was the peak of the pandemic, this SIHD reached the highest mortality rate and the highest lethality rate among all Brazilian SIHDs, reaching 139.7 deaths per 100 000 inhabitants with a lethality rate of 9.7% [10].
Although it is the most populous, Xavante is the SIHD with the most reduced structure in proportional terms. For each IBHU there are 670 indigenous people, while in the SIHD Xingu, the second most critical, that number drops to almost half - 348. Another worrying issue is that only 8.5% of Xavante villages have an IBHU, that is, 91.5% of local communities do not have surveillance measures most of the time [18, 33].
It is not only the availability of the basic health care structure that positions the Xavante as one of the most vulnerable peoples to COVID-19 in the midwest region. Aspects of their sociocultural organisation and of their epidemiological profile in particular, marked by high rates of hypertension, diabetes mellitus and obesity, tend to increase the risk of developing severe forms of COVID-19 [Reference Soares34, Reference Soares35].
In addition, the organisation of the Xavante villages, with their very close houses and many residents, combined with the practice of collective events, such as the burial of their dead, may explain the high spread of COVID-19 in these territories [33].
Kang et al. [Reference Kang36] found in their study in China that COVID-19 presents strong spatial autocorrelation, with an increase in the number of cases in the areas adjacent to the focus. Thus, being located near an area with excess occurrences indicates the need for attention and possible measures to restrict contact with their surroundings, and these cases like need special attention from health managers.
The study by Martines et al. [Reference Martines37] detected, between the months of February and July, emerging spatiotemporal clusters for cases of COVID-19 in Brazil, located mainly in the northern region. The existence of these clusters in this period may be related to the high number of cases in indigenous people living in the region, as evidenced in our study. The authors identified that places with greater social inequality are more affected, which becomes even more worrying in the case of the indigenous population, which has extreme social fragility, as already mentioned here.
In view of the above, the current vulnerability of these peoples is not only the result of an unforeseen pandemic, but part of a history of political and social exclusion that has historically violated their citizens' rights and fundamental dimensions of their ways of life.
High transmission of the virus combined with the low resolution of actions aimed at controlling the disease has culminated in a constant threat to life within indigenous communities. Although there is a national COVID-19 contingency plan for the indigenous population, the efforts made so far are reactive and late in nature. The control of the disease is difficult and becomes even more complex with the specificities of the indigenous population, requiring multisectoral actions. If control strategies are focused on areas of potential risk, it is likely that the impacts of COVID-19 will be mitigated and deaths will be avoided, especially in view of the current trend of reducing protection measures.
This study advances knowledge because it targets the indigenous population, considered one of the populations with the highest degree of vulnerability to COVID-19, and because it uses space−time analysis techniques as a tool to address this problem.
Conclusion
This study allowed the identification of hot and cold spots of the occurrence of and deaths by COVID-19 in the Brazilian indigenous population. High-risk space−time clusters were also identified for for cases and deaths, which were mainly concentrated in the north and midwest regions of the Brazil. In this context, we suggest that actions to combat COVID-19 in indigenous territories be strengthened and better targeted, mainly considering the risk areas identified in this study.
Acknowledgements
The authors would like to thank the Araguaia Epidemiology and Geoprocessing Research Group (EpiGeo-Araguaia) for the scientific discussions that supported this work.
Author contributions
JDA and ASA: conceptualisation; WPP, SMS, JEB: data curation; JDA, ASA, ARS: formal analysis; JDA and ARS: methodology; JDA and WPP: project administration; JDA, ASA, WPP, JEB and SMS: writing – original draft; WPP and ASA: Validation; JDA, ASA, WPP, JEB, SMS and ARS: writing – review and editing.
Financial support
This research received no specific grant from any funding agency, commercial or not-for-profit sectors.
Conflict of interest
None.
Data availability statement
The data that support the findings of this study are openly available in at https://coronavirus.saude.gov.br/boletins-epidemiologicos