Introduction
Repetitive negative thinking (RNT), a cognitive process that encompasses past (rumination) and future (worry) directed thoughts focusing on negative experiences and the self (Harvey, Watkins, Mansell, & Shafran, Reference Harvey, Watkins, Mansell and Shafran2004), is a common feature of major depressive disorder (MDD) and anxiety disorders. RNT is characterized by its focus on negatively valenced thoughts, especially on possible causes and consequences of one's negative emotions and experiences (current, past, and future) (Nolen-Hoeksema, Wisco, & Lyubomirsky, Reference Nolen-Hoeksema, Wisco and Lyubomirsky2008). RNT is consistently linked to a higher frequency, duration, and severity of depression and anxiety, and it also predicts suicidality (Krajniak, Miranda, & Wheeler, Reference Krajniak, Miranda and Wheeler2013; Surrence, Miranda, Marroquin, & Chan, Reference Surrence, Miranda, Marroquin and Chan2009; Watkins & Roberts, Reference Watkins and Roberts2020). Higher RNT is also associated with a slower response and poorer outcome to both antidepressant medication and cognitive-behavioral therapy (CBT) (Jones, Siegle, & Thase, Reference Jones, Siegle and Thase2008; Schmaling, Dimidjian, Katon, & Sullivan, Reference Schmaling, Dimidjian, Katon and Sullivan2002). Although effective treatments for MDD have been established, nearly two-thirds of patients do not respond adequately (Cain, Reference Cain2007), and treatment of MDD with currently available modalities still leaves some residual symptoms, including RNT (Nierenberg et al., Reference Nierenberg, Husain, Trivedi, Fava, Warden, Wisniewski and Rush2010).
RNT could serve as a common risk factor for depression and anxiety, and it may contribute to explaining why MDD and anxiety are highly comorbid. Longitudinal studies found that RNT predicts the level of both anxiety and depression symptoms (McLaughlin & Nolen-Hoeksema, Reference McLaughlin and Nolen-Hoeksema2011; Nolen-Hoeksema, Reference Nolen-Hoeksema2000). Thus, the transdiagnostic nature of RNT probably makes it a promising target for the prevention and treatment for MDD, where anxiety is often comorbid.
However, relatively little is known about the underlying neural circuitry of RNT. For example, some studies reported that RNT may result in increased connectivity within the default mode network (DMN) (Cooney, Joormann, Eugene, Dennis, & Gotlib, Reference Cooney, Joormann, Eugene, Dennis and Gotlib2010; Misaki, Tsuchiyagaito, Al Zoubi, Paulus, & Bodurka, Reference Misaki, Tsuchiyagaito, Al Zoubi, Paulus and Bodurka2020), a set of functionally connected brain nodes linked to self-referential processing, as well as increased connectivity of the DMN with the subgenual anterior cingulate cortex (sgACC) (Berman et al., Reference Berman, Misic, Buschkuehl, Kross, Deldin, Peltier and Jonides2014; Hamilton, Farmer, Fogelman, & Gotlib, Reference Hamilton, Farmer, Fogelman and Gotlib2015). Increased recruitment of the DMN and so-called ‘self-related’ regions, such as the medial prefrontal cortex (MPFC), posterior cingulate cortex (PCC), and precuneus, is often linked to the frequent occurrence of RNT in depressive patients (Hamilton et al., Reference Hamilton, Farmer, Fogelman and Gotlib2015; Misaki et al., Reference Misaki, Tsuchiyagaito, Al Zoubi, Paulus and Bodurka2020). A meta-analysis also reported that RNT is correlated with increased connectivity between the MPFC and PCC (Zhou et al., Reference Zhou, Chen, Shen, Li, Chen, Zhu and Yan2020).
Nonetheless, recent meta-analyses reported slightly reduced DMN in MDD, with RNT not predicting hyperconnectivity within the DMN components (Tozzi et al., Reference Tozzi, Zhang, Chesnut, Holt-Gosselin, Ramirez and Williams2021; Yan et al., Reference Yan, Chen, Li, Castellanos, Bai, Bo and Zang2019). Those meta-analyses were in line with other studies that found decreased connectivity in the DMN with MDD individuals with small effect sizes (Chen, Wang, Zhu, Tan, & Zhong, Reference Chen, Wang, Zhu, Tan and Zhong2015; Zhu, Zhu, Shen, Liao, & Yuan, Reference Zhu, Zhu, Shen, Liao and Yuan2017). Moreover, recent studies have reported that the DMN is not as homogenous as previously assumed and suggested that it can be further subdivided into smaller subsystems (Andrews-Hanna, Reidler, Sepulcre, Poulin, & Buckner, Reference Andrews-Hanna, Reidler, Sepulcre, Poulin and Buckner2010). Those subsystems of the DMN include a midline core composed of hub of the DMN, i.e., anterior medial prefrontal cortex (aMPFC) and PCC; the dorsal medial prefrontal cortex (dMPFC) subsystem involved in self-referential processing; and the medial temporal lobe (MTL) subsystem involved in episodic memory (see Andrews-Hanna et al. (Reference Andrews-Hanna, Reidler, Sepulcre, Poulin and Buckner2010) and online Supplemental material for more detailed explanations). Interaction within and between the DMN subsystems may explain contradictory findings linking DMN to MDD in general, and RNT in particular.
Concurrently, results regarding resting-state functional connectivity in MDD beyond the DMN have also offered nonuniform results. For example, some studies found increased functional connectivity within executive control and affective salience networks (Avery et al., Reference Avery, Drevets, Moseman, Bodurka, Barcalow and Simmons2014; Connolly et al., Reference Connolly, Wu, Ho, Hoeft, Wolkowitz, Eisendrath and Yang2013; Sheline, Price, Yan, & Mintun, Reference Sheline, Price, Yan and Mintun2010), while other studies found opposite results in the same areas (Alexopoulos et al., Reference Alexopoulos, Hoptman, Kanellopoulos, Murphy, Lim and Gunning2012; Veer et al., Reference Veer, Beckmann, van Tol, Ferrarini, Milles, Veltman and Rombouts2010). These contradictory findings might be due to various reasons, including the use of different approaches such as focusing on a predefined regions-of-interest approach or a whole-brain approach, as well as the heterogeneity of MDD populations. For these reasons, we used a whole-brain exploration approach to understand the connectivity patterns underlying RNT in MDD.
Finally and most importantly, the severity of RNT and depressive symptoms in general, could be closely associated; in this scenario, it might be difficult to disentangle the underlying brain mechanisms of either symptom dimension, and thus attribution of DMN abnormalities to RNT severity independently from overall depressive symptom severity is difficult to ascertain. As stated above, findings related to the role of DMN on RNT are not uniform. However, depression in general appears to be associated with increased functional connectivity within the DMN. For example, a higher baseline resting-state connectivity of the pregenual anterior cingulate cortex (pgACC) and ventromedial prefrontal cortex (vMPFC) predicted clinical response to antidepressant treatments, including repetitive transcranial magnetic stimulation (rTMS) treatment, pharmacotherapy, and psychotherapy (Long et al., Reference Long, Du, Zhao, Wu, Zheng and Lei2020). Correspondingly, symptom reduction after rTMS was associated with reduced connectivity between DMN, sgACC and insula (Hamilton et al., Reference Hamilton, Farmer, Fogelman and Gotlib2015; Liston et al., Reference Liston, Chen, Zebley, Drysdale, Gordon, Leuchter and Dubin2014; Philip et al., Reference Philip, Barredo, van 't Wout-Frank, Tyrka, Price and Carpenter2018). Pharmacotherapy and psychotherapy have been shown to normalize connectivity within the DMN (Evans et al., Reference Evans, Szczepanik, Brutsche, Park, Nugent and Zarate2018; Jacobs et al., Reference Jacobs, Watkins, Peters, Feldhaus, Barba, Carbray and Langenecker2016; Li et al., Reference Li, Liu, Friston, Shen, Wang, Zeng and Hu2013; Posner et al., Reference Posner, Hellerstein, Gat, Mechling, Klahr, Wang and Peterson2013; Straub et al., Reference Straub, Metzger, Plener, Koelch, Groen and Abler2017). Possibly, variable findings related to the role of DMN on RNT may be due to the fact that increased connectivity of the DMN reflect depression in general rather than RNT intensity. One way to reduce potential biases in studying RNT-related brain mechanisms is to use propensity scoring to match the severity of depression, anxiety, and demographic characteristics. The propensity score is defined as the conditional probability of assignment to a particular group given a vector of observed covariates (Rosenbaum & Rubin, Reference Rosenbaum and Rubin1983). In the randomized experiment, treatment or control is randomly assigned, and the propensity score equals 0.5 for all subjects; however, in an observational study, subjects with certain characteristics can be more likely to receive treatment (or control), and thus the propensity score to receive treatment (or control) varies among subjects based on these pre-treatment characteristics (Rosenbaum, Reference Rosenbaum and Wright2015). In our study, the certain treatment or exposure is having high RNT, and the propensity score for having high RNT could vary among subjects based on characteristics such as depression, anxiety and other demographic variables. By applying propensity score matching, we can control for these biases in a quasi-experimental manner.
The aim of the present study was to identify resting-state functional connectivity patterns that are specifically related to RNT in depression, by employing functional magnetic resonance imaging (fMRI) in a propensity-matched sample of patients with MDD and varying RNT severity. On the basis of previous observations related to the DMN findings (Tozzi et al., Reference Tozzi, Zhang, Chesnut, Holt-Gosselin, Ramirez and Williams2021; Yan et al., Reference Yan, Chen, Li, Castellanos, Bai, Bo and Zang2019) and other resting-state fMRI studies described above, we expected that clinically important connectivity patterns related to RNT may exist outside of the DMN, and conducted a data-driven, whole-brain voxel-to-voxel connectivity pattern analysis to examine functional connectivity alterations specifically related to RNT in MDD. Following prior studies (Andrews-Hanna et al., Reference Andrews-Hanna, Reidler, Sepulcre, Poulin and Buckner2010; Tozzi et al., Reference Tozzi, Zhang, Chesnut, Holt-Gosselin, Ramirez and Williams2021; Zhou et al., Reference Zhou, Chen, Shen, Li, Chen, Zhu and Yan2020; Zhu et al., Reference Zhu, Zhu, Shen, Liao and Yuan2017), we also conducted ROI-to-ROI connectivity analysis within the DMN subsystems to examine whether RNT as well as depression in general are related to altered connectivity within the DMN (online Supplementary Methods and Results).
Methods
Participants
We studied 158 subjects with MDD (with or without comorbid anxiety including generalized anxiety disorder, social phobia, panic disorder, or post-traumatic stress disorder) [128 females, mean age = 30.93 (s.d. = 10.63) years] from the Neuroscience-Based Mental Health Assessment and Prediction (NeuroMAP, P20GM121312) – Center of Biomedical Research Excellence (CoBRE) at the Laureate Institute for Brain Research (LIBR) (Kuplicki et al., Reference Kuplicki, Touthang, Al Zoubi, Mayeli, Misaki, Neuro and Bodurka2021). The diagnosis was based on an abbreviated version of the Mini International Neuropsychiatric Interview (MINI V.6.0 or 7.0) (Sheehan et al., Reference Sheehan, Lecrubier, Sheehan, Amorim, Janavs, Weiller and Dunbar1998), followed by a clinical case conference by a board-certified clinical psychiatrist (SSK or SMG). A research protocol of CoBRE NeuroMAP was approved by the Western Institutional Review Board (IRB). All participants provided written informed consent and received financial compensation for their time participating in this study.
Clinical measures
RNT was evaluated with the Ruminative Responses Scale (RRS) (Nolen-Hoeksema & Morrow, Reference Nolen-Hoeksema and Morrow1991). The RRS consists of 22 items with three subscales (i.e. brooding, reflective pondering and depression-related) (Nolen-Hoeksema & Morrow, Reference Nolen-Hoeksema and Morrow1991). The five-item reflective pondering (e.g. Go away by yourself and think about why you feel this way.) is considered an adaptive aspect of RNT, reflecting the degree to which individuals engage in cognitive problem-solving to alleviate the depressive mood. The 5-item brooding dimension of RNT (e.g. Think ‘Why can't I handle things better?’) is considered a maladaptive aspect of RNT, and it reflects the degree to which individuals passively focus on negative valence and experiences and their meaning. The other 12-item depression subscale captures content related to depressive symptoms (e.g. ‘Think about how sad you feel.’), and could be confounded with depressive symptoms. Among those three subscales, the brooding subscale usually implies a self-critical evaluative viewpoint (Treynor, Gonzalez, & Nolen-Hoeksema, Reference Treynor, Gonzalez and Nolen-Hoeksema2003), and studies have consistently demonstrated that MDD is specifically characterized by high levels of brooding (Armey et al., Reference Armey, Fresco, Moore, Mennin, Turk, Heimberg and Alloy2009; Burwell & Shirk, Reference Burwell and Shirk2007; Treynor et al., Reference Treynor, Gonzalez and Nolen-Hoeksema2003; Watkins, Reference Watkins2009). Thus, in the present study, we used the brooding subscale of RRS to measure RNT. We also used the Hamilton Depression Rating Scale (HAM-D) (Hamilton, Reference Hamilton1960), the Patient Health Questionnaire-9 (PHQ) (Kroenke, Spitzer, & Williams, Reference Kroenke, Spitzer and Williams2001), and Overall Anxiety Severity and Impairment Scale (OASIS) (Norman, Cissell, Means-Christensen, & Stein, Reference Norman, Cissell, Means-Christensen and Stein2006) to measure the severity of depression and anxiety symptoms.
Propensity score matching and experimental group assignment
Figure 1 shows the sampling and data flow in this study. We divided the present MDD sample into participants with high and low RNT (n = 50 per group), using a propensity score-matching approach. After excluding 10 subjects due to the excessive head motion during the scan, the dataset of 148 MDD from NeuroMAP (described in detail in 2.1.) was divided into two groups (high RNT and low RNT) based on the median of the brooding subscale of RRS, as described above. The median of RRS brooding subscale was 14 in the dataset, and MDD subjects who scored more than 14 for the RRS brooding were categorized as having high RNT, while MDD subjects who scored less than or equal to 14 for the RRS brooding were categorized as having low RNT. After the median split, 63 MDD subjects were categorized as having high RNT, and 85 MDD subjects were categorized as having low RNT. Of those, 50 subjects were assigned to either high RNT group or low RNT group, while 48 subjects were discarded due to missmatching. The MatchIt library (Ho, Imai, King, & Stuart, Reference Ho, Imai, King and Stuart2011) in R (https://cran.r-project.org) was used for the matching. Variables used for matching included diagnosis (MDD with or without anxiety comorbidity), PHQ score, OASIS score, age, sex, and body mass index (BMI); both groups resulted in matching also for ethnicity, education level, income, and employment status (Table 1). Using the default ‘nearest neighbor’ approach, the algorithm used logistic regression to estimate the predicted probability of group membership status (high RNT v. low RNT) given this set of matching covariates. Then, 50 MDD subjects with high RNT were randomly selected for high RNT group, and 1-to-1 matching was implemented to select the subject from the low RNT group with the nearest predicted probability (propensity score) for each participant from the high RNT group. Table 1 describes the demographic and clinical characteristics of the resulting groups.
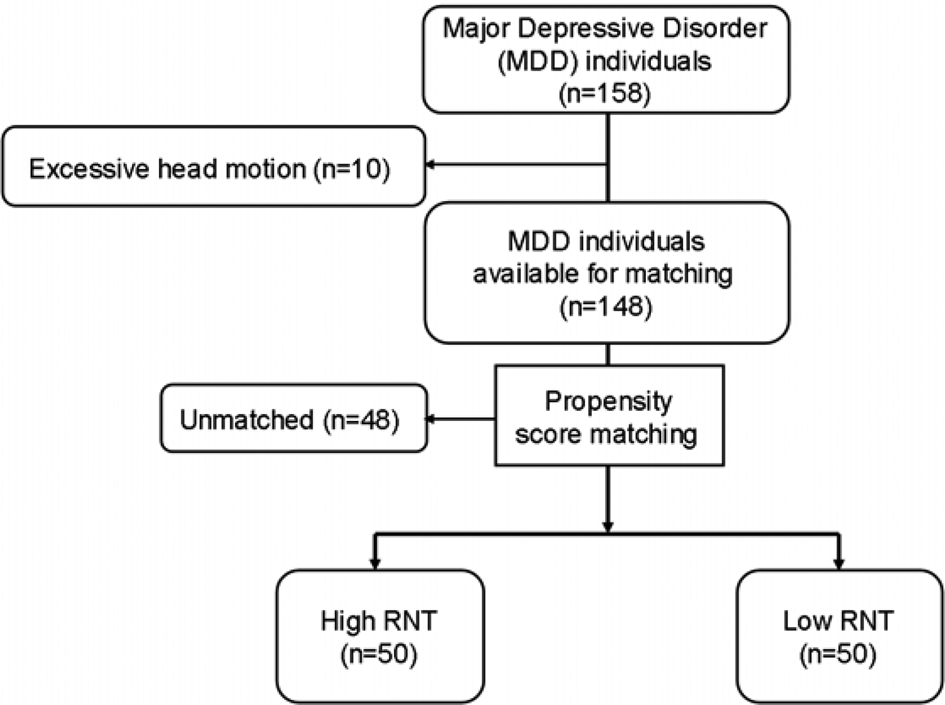
Fig. 1. Flow diagram of participant selection. MDD individuals were divided into two groups resulting from a median split of the total score of Ruminative Response Scale (RRS) as a measure of RNT intensity. Fifty MDD subjects with high RNT and 50 MDD subjects with low RNT were thus selected during propensity score matching, as described in 2.3.
Table 1. Demographic and symptom profile of propensity score-matched data

Neuroimaging data acquisition and preprocessing
Resting-state fMRI data were collected on a whole-body 3 Tesla MR750 MRI scanner (GE Healthcare, Milwaukee, WI) with an 8-channel receive-only head array coil. All the participants went through an anatomical scan and then two six-minute resting-state fMRI scans were obtained consecutively (Cho, Korchmaros, Vogelstein, Milham, & Xu, Reference Cho, Korchmaros, Vogelstein, Milham and Xu2021). Details on image acquisition and preprocessing can be found in the online Supplementary material.
Resting-state fMRI whole-brain voxel-to-voxel correlation analysis
A whole-brain voxel-to-voxel correlation analysis was conducted to detect brain areas with different functional connectivity patterns between the high and low RNT groups. We used group multivariate pattern analysis implemented in the CONN-toolbox (Whitfield-Gabrieli & Nieto-Castanon, Reference Whitfield-Gabrieli and Nieto-Castanon2012). The analysis creates multivariate correlation (MCOR) maps to conduct whole-brain voxel-wise functional connectivity pattern analysis (Anteraper et al., Reference Anteraper, Guell, D'Mello, Joshi, Whitfield-Gabrieli and Joshi2019; Anteraper et al., Reference Anteraper, Collin, Guell, Scheinert, Molokotos, Henriksen and Whitfield-Gabrieli2020; Byun et al., Reference Byun, Cha, Kim, Lee, Lee, Sunwoo and Jung2021; Guell et al., Reference Guell, Arnold Anteraper, Gardner, Whitfield-Gabrieli, Kay-Lambkin, Iverson and Stanwell2020; Kazumata et al., Reference Kazumata, Tha, Uchino, Ito, Nakayama and Abumiya2017; Morris et al., Reference Morris, Chaddock-Heyman, Ai, Anteraper, Castanon, Whitfield-Gabrieli and Kramer2021; Muehlhan et al., Reference Muehlhan, Alexander, Trautmann, Weckesser, Vogel, Kirschbaum and Miller2020; Takamiya et al., Reference Takamiya, Kishimoto, Hirano, Nishikata, Sawada, Kurokawa and Mimura2021; Wang et al., Reference Wang, Bernanke, Peterson, McGrath, Stewart, Chen and Posner2019; Westfall et al., Reference Westfall, Anteraper, Chaddock-Heyman, Drollette, Raine, Whitfield-Gabrieli and Hillman2020; Whitfield-Gabrieli et al., Reference Whitfield-Gabrieli, Ghosh, Nieto-Castanon, Saygin, Doehrmann, Chai and Gabrieli2016). A detailed description of the methodology is provided in online Supplementary material and elsewhere (Anteraper et al., Reference Anteraper, Guell, D'Mello, Joshi, Whitfield-Gabrieli and Joshi2019). In essence, pairwise correlations for a single (seed) voxel and all other voxels in the brain (voxel-to-voxel correlation) are calculated, and the dimensionality of the correlation maps across participants is reduced by singular value decomposition (SVD). MCOR is an SVD component score of each participant for a seed voxel, and the MCOR map is obtained by repeating this analysis voxel-wise in the entire brain.
Following previous studies (Anteraper et al., Reference Anteraper, Guell, D'Mello, Joshi, Whitfield-Gabrieli and Joshi2019; Pang et al., Reference Pang, Wei, Zhao, Li, Li, Lu and Wang2022; Wang et al., Reference Wang, Bernanke, Peterson, McGrath, Stewart, Chen and Posner2019; Westfall et al., Reference Westfall, Anteraper, Chaddock-Heyman, Drollette, Raine, Whitfield-Gabrieli and Hillman2020), we took the first five components, which explained more than 90% of the variance. Hence, the five participant-specific spatial maps were used as a low-dimensional proxy of the connectivity pattern characterizing each participants' whole-brain voxel-to-voxel connectivity. An omnibus F test was performed across five spatial components to evaluate a group difference in this five-dimensional representation of the spatial pattern of this connectivity to all other voxels. Mean head motion was used as a covariate of no interest in all second-level analyses. Clusters surviving threshold of voxel-wise p < 0.001 and cluster-level false discovery rate (FDR)-corrected p < 0.05 [topological FDR (Chumbley, Worsley, Flandin, & Friston, Reference Chumbley, Worsley, Flandin and Friston2010)] were subjected to post-hoc analyses.
Post-hoc characterization of significant MCOR clusters
Since the MCOR analysis in CONN toolbox is an omnibus test on the component scores (Anteraper et al., Reference Anteraper, Guell, D'Mello, Joshi, Whitfield-Gabrieli and Joshi2019), post-hoc analyses with two-sample t tests were conducted to further characterize how connectivity patterns differed between the groups. The significant clusters obtained from the MCOR map analysis were taken as seeds for seed-based whole-brain connectivity analysis. Pearson's correlation coefficients between the time courses of the cluster and all other voxels in the brain were computed and then converted to z scores using Fisher's transformation to carry out general linear model analyses in CONN toolbox. Mean head motion was used as a covariate of no interest in all second-level analyses. Thresholds of voxel-wise p < 0.001 and cluster-level FDR-corrected p < 0.05 were applied in the group comparison.
Results
Demographic and clinical characteristics
Table 1 shows the demographic and symptom profile of propensity score-matched RNT groups. The high RNT group showed higher scores of RRS and its subscales than the low RNT group. Other demographic variables and symptom profiles were similar in both groups.
Whole-brain voxel-to-voxel correlation pattern analysis
Figure 2 displays the clusters showing intergroup differences in the whole-brain voxel-to-voxel correlation analysis. Cluster 1 was found in the anterior part of the superior temporal sulcus (STS) in the right hemisphere, whereas Cluster 2 was located in the posterior part of the STS in the left hemisphere. Table 2 shows peak coordinates for each cluster.

Fig. 2. Regions showing connectivity differences between high RNT v. low RNT from the whole-brain voxel-to-voxel correlation pattern analysis. Cluster size (k) threshold of k ⩾ 49 (p < 0.05 FDR corrected) and height threshold of p < 0.001 (uncorrected) were used. L, left; R, right; RNT, repetitive negative thinking; FDR, false-discovery rate.
Table 2. Peak coordinates and statistics of the whole-brain voxel-to-voxel correlation pattern analysis (high RNT v. low RNT)

RNT, repetitive negative thinking; L, left; R, right, MNI, Montreal Neurological Institute; FDR, false-discovery rate.
A voxel-wise threshold of p < 0.001(uncorrected) and a cluster size (k) threshold of k ⩾ 49 (p < 0.05, FDR-corrected) were used.
Post-hoc analysis
Results from the post-hoc seed-based whole-brain functional connectivity analysis are shown in Fig. 3. The peak coordinates and t-statistics are summarized in online Supplementary material, Table S1. Right STS seed displayed significantly greater functional connectivity with the bilateral anterior insular cortex (AI) in high RNT compared to low RNT group (left AI: d = 1.15 and right AI: d = 1.19, Fig. 3a). Connectivity between this seed and left dorsolateral prefrontal cortex (DLPFC) was also greater in high RNT compared to low RNT group (d = 1.10, Fig. 3a). Those connectivity values were not correlated with the severity of depression or anxiety (online Supplementary material, Table S2).

Fig. 3. Seed-to-voxel whole brain analysis comparing high RNT and low RNT using clusters obtained from voxel-to-voxel correlation analysis as seeds. a. Cluster 1 in the right superior temporal sulcus (STS) (MNI: + 60, −30, −02) as a seed. b. Cluster 2 in the left STS (MNI: −42, −50, 12) as a seed. The spheres illustrate the locations of the peak voxel. Positive connectivity from the seed is presented as red spheres and red lines, and negative connectivity from the seed are presented as blue spheres and blue lines. The red colored and blue colored areas indicate cluster extensions for either positive or negative connectivity from each seed respectively. A height threshold of p < 0.001(uncorrected) and FDR-corrected cluster threshold of p < 0.05 were used. The bar graphs show the z values between each significant cluster and seeds (mean ± s.e.). RNT, repetitive negative thinking; L, left; R, right; AG, angular gyrus; AI, anterior insular; DLPFC, dorsolateral prefrontal cortex; FuG; fusiform gyrus; MTG, middle temporal gyrus; OrG, orbital gyrus; PhG, parahippocampal gyrus; SFG, superior frontal gyrus.
Discussion
This investigation aimed to compare the resting-state connectivity patterns in individuals with MDD and high v. low level of RNT. High v. low RNT MDD individuals differed in functional connectivity of bilateral STS. High RNT individuals showed greater connectivity between STS and AI/DLPFC. This study has demonstrated that those connectivity patterns in high RNT v. low RNT group are not attributed to potential confounders–depression and/or anxiety symptoms.
The role of the STS in RNT
The STS, located on the lateral aspect of the brain in the temporal lobe, is a brain region that is important for multiple perceptual and cognitive functions including speech processing, theory-of-mind (ToM), audiovisual integration, motion and face perception, interoception, and threat processing (Garcia-Cordero et al., Reference Garcia-Cordero, Sedeno, de la Fuente, Slachevsky, Forno, Klein and Ibanez2016; Kret, Pichon, Grezes, & de Gelder, Reference Kret, Pichon, Grezes and de Gelder2011; Lahnakoski et al., Reference Lahnakoski, Glerean, Salmi, Jaaskelainen, Sams, Hari and Nummenmaa2012; Liebenthal, Desai, Humphries, Sabri, & Desai, Reference Liebenthal, Desai, Humphries, Sabri and Desai2014; Pichon, de Gelder, & Grezes, Reference Pichon, de Gelder and Grezes2009). Studies have highlighted the pivotal role of the STS, together with other temporal regions and inferior frontal gyrus, in speech processing (Alderson-Day & Fernyhough, Reference Alderson-Day and Fernyhough2015a; Lahnakoski et al., Reference Lahnakoski, Glerean, Salmi, Jaaskelainen, Sams, Hari and Nummenmaa2012; Liebenthal et al., Reference Liebenthal, Desai, Humphries, Sabri and Desai2014). One possible interpretation of altered functional connectivity in the STS related to RNT is that this symptom assumes the clinical form of verbal thoughts, or inner speech (Alderson-Day & Fernyhough, Reference Alderson-Day and Fernyhough2015a; Moffatt, Mitrenga, Alderson-Day, Moseley, & Fernyhough, Reference Moffatt, Mitrenga, Alderson-Day, Moseley and Fernyhough2020; Oliver, Smith, & Leigh, Reference Oliver, Smith and Leigh2015; Perrone-Bertolotti, Rapin, Lachaux, Baciu, & Loevenbruck, Reference Perrone-Bertolotti, Rapin, Lachaux, Baciu and Loevenbruck2014). Indeed, the bilateral STS clusters showing intergroup differences in this study are encompassed in the predicted activation map related to the term ‘inner speech’ by the NeuroQuery (online Supplementary material, Fig. S3). Although inner speech can be considered as a mental simulation of overt speech (Alderson-Day & Fernyhough, Reference Alderson-Day and Fernyhough2015a), it is not solely related to speech production and comprehension, but also related to conversational and social features (Alderson-Day et al., Reference Alderson-Day, McCarthy-Jones, Bedford, Collins, Dunne, Rooke and Fernyhough2014; Alderson-Day & Fernyhough, Reference Alderson-Day and Fernyhough2015b). Similarly, in addition to ‘talking to oneself,’ RNT consists of self-focused thoughts and evaluations of one's emotional state. For example, RNT involves more evaluative and dialogic (conversational style) inner speech (e.g. think about the causes, consequences, and meanings of your current feelings) than emotionally neutral thoughts (e.g. thinking about a shopping list). Therefore, it has been proposed that RNT can be characterized as an internal conversation, rather than as a monologue (Jones & Fernyhough, Reference Jones and Fernyhough2009; Moffatt et al., Reference Moffatt, Mitrenga, Alderson-Day, Moseley and Fernyhough2020). Burgeoning evidence consistently reports that the generation of dialogic speech is associated with a range of regions beyond the classic left-sided perisylvian language network, for example the right STS (Linden et al., Reference Linden, Thornton, Kuswanto, Johnston, van de Ven and Jackson2011; Shergill et al., Reference Shergill, Bullmore, Brammer, Williams, Murray and McGuire2001; Yao, Belin, & Scheepers, Reference Yao, Belin and Scheepers2011, Reference Yao, Belin and Scheepers2012). Our observation of altered functional connectivity in the right STS as well as the left STS in high RNT individuals provides additional support to this view.
Moreover, it has been proposed that dialogic inner speech could draw on ToM capacities, requiring not just the representation of a voice but also the sense and intention of a plausible and realistic interlocutor; activity in the right STS was evident during both dialogic scenarios and ToM reasoning (Carrington & Bailey, Reference Carrington and Bailey2009), and this region is a major component of the ‘social brain’ (Blakemore, Reference Blakemore2008; Guinjoan, de Achaval, Villarreal, Abusamra, & Nemeroff, Reference Guinjoan, de Achaval, Villarreal, Abusamra and Nemeroff2015; Kennedy & Adolphs, Reference Kennedy and Adolphs2012). Together with other regions in the temporal lobe (e.g. anterior temporal lobe, and middle and superior temporal gyrus) and the precuneus, the STS and its neighboring temporoparietal junction constitute a critical node of the mentalization, or ToM, network (Carrington & Bailey, Reference Carrington and Bailey2009; Gobbini, Koralek, Bryan, Montgomery, & Haxby, Reference Gobbini, Koralek, Bryan, Montgomery and Haxby2007; Kennedy & Adolphs, Reference Kennedy and Adolphs2012; Watson, Latinus, Charest, Crabbe, & Belin, Reference Watson, Latinus, Charest, Crabbe and Belin2014). The STS plays a key role in social perception and cognition, including the perception of faces and human motion, as well as understanding others' actions, mental states, and language (Deen, Koldewyn, Kanwisher, & Saxe, Reference Deen, Koldewyn, Kanwisher and Saxe2015). Notably, the activation of this mentalizing network to process social information is usually stronger in the right hemisphere (Deen et al., Reference Deen, Koldewyn, Kanwisher and Saxe2015; Dodell-Feder, Koster-Hale, Bedny, & Saxe, Reference Dodell-Feder, Koster-Hale, Bedny and Saxe2011; Goldschmidt et al., Reference Goldschmidt, Villarreal, de Achaval, Drucaroff, Costanzo, Castro and Guinjoan2014). In most clinical scenarios, RNT consists of thoughts about relationships with other people, and about how past interpersonal events might have played out differently (Newby & Moulds, Reference Newby and Moulds2012). Our observation of different voxel-to-voxel connectivity in the bilateral STS distinguishing high RNT from low RNT suggests that RNT is indeed related to the ToM circuit.
The STS, together with salience network (SN), also plays an important role in interoception and threat processing (Drabant et al., Reference Drabant, Kuo, Ramel, Blechert, Edge, Cooper and Gross2011; Garcia-Cordero et al., Reference Garcia-Cordero, Sedeno, de la Fuente, Slachevsky, Forno, Klein and Ibanez2016; Sripada et al., Reference Sripada, King, Welsh, Garfinkel, Wang, Sripada and Liberzon2012). During an interoceptive attention task (relative to an exteroceptive task), greater activity in regions of the superior temporal gyrus, insula cortex, and precentral gyrus was associated with reduced attentional control and greater distraction and worry (Stern et al., Reference Stern, Grimaldi, Muratore, Murrough, Leibu, Fleysher and Burdick2017). The role of the STS in interoceptive awareness might have a bearing on the influence of RNT on anxiety. Altered bodily sensation and interoceptive awareness have been implicated in the pathophysiology of anxiety disorders (Clark et al., Reference Clark, Salkovskis, Ost, Breitholtz, Koehler, Westling and Gelder1997; Khalsa et al., Reference Khalsa, Adolphs, Cameron, Critchley, Davenport and Feinstein2018; Paulus & Stein, Reference Paulus and Stein2010; Wells & Papageorgiou, Reference Wells and Papageorgiou2001), while the ability to control and regulate attention on bodily sensation is thought to help psychological well-being (Farb et al., Reference Farb, Daubenmier, Price, Gard, Kerr, Dunn and Mehling2015). Moreover, the STS is thought to be involved in threat processing. Studies found hyperactivation in the STS for threatening (fear and angry) body expressions (Kret et al., Reference Kret, Pichon, Grezes and de Gelder2011; Pichon et al., Reference Pichon, de Gelder and Grezes2009), and several brain regions showed responses to threat anticipation including the bilateral insula, ACC, and STS (Drabant et al., Reference Drabant, Kuo, Ramel, Blechert, Edge, Cooper and Gross2011). There is increasing evidence to suggest that interoception and threat processing guide our cognitive processes such that fluctuations in bodily arousal and interpretation of threatening stimuli under uncertainty contribute to cognitive processes themselves by feeding back to decision making and RNT [as worry or rumination; (Bechara, Damasio, Tranel, & Damasio, Reference Bechara, Damasio, Tranel and Damasio1997; Bonaz et al., Reference Bonaz, Lane, Oshinsky, Kenny, Sinha, Mayer and Critchley2021; Garfinkel & Critchley, Reference Garfinkel and Critchley2013; Matthews & Wells, Reference Matthews, Wells, Papageorgiou and Well2004; Paulus & Stein, Reference Paulus and Stein2010)].
DMN, RNT, and depression
In our study, we did not find any DMN connectivity pattern differences between the groups (online Supplementary material, Figs S1 and S2). Our results are in line with recent meta-analyses reporting that RNT did not predict hyperconnectivity within the DMN components (Tozzi et al., Reference Tozzi, Zhang, Chesnut, Holt-Gosselin, Ramirez and Williams2021; Yan et al., Reference Yan, Chen, Li, Castellanos, Bai, Bo and Zang2019). To the best of our knowledge, this is the first study investigating the functional connectivity linked to the intensity of RNT taking into account the severity of both depression and anxiety. Our MDD subjects were mostly (81%) unmedicated. This characteristic of our sample needs to be considered for the interpretation of our results in light of prior studies.
Altered functional connectivity of the STS in MDD individuals with high RNT
In summary, altered STS functional connectivity in the high RNT group may potentially relate to dysfunction in several areas, including perceptive, cognitive, and speech processing. Several studies indicated that the functional subdivisions of the STS, e.g., left, right, anterior, and posterior part of the STS, have distinct functions (Hein & Knight, Reference Hein and Knight2008; Lahnakoski et al., Reference Lahnakoski, Glerean, Salmi, Jaaskelainen, Sams, Hari and Nummenmaa2012; Liebenthal et al., Reference Liebenthal, Desai, Humphries, Sabri and Desai2014). On the other hand, the fact that lesions in equivalent STS regions can cause diverse functional deficits could argue against a clear-cut functional subdivision (Akiyama et al., Reference Akiyama, Kato, Muramatsu, Saito, Nakachi and Kashima2006a, Reference Akiyama, Kato, Muramatsu, Saito, Umeda and Kashima2006b; Hein & Knight, Reference Hein and Knight2008; Samson, Apperly, Chiavarino, & Humphreys, Reference Samson, Apperly, Chiavarino and Humphreys2004). Possibly, the role of the STS may vary depending on its functional connectivity with other regions, rather than on the functional subdivision of the STS itself (Hein & Knight, Reference Hein and Knight2008). In our study, individuals with high RNT showed hyperconnectivity between right STS and AI, and between bilateral STS and left DLPFC. Hyperconnectivity between the STS and AI may support a role for the STS in interoceptive and threat processing because the AI serves as a strategic neural node in body integrity, peripheral autonomic output, and appraisal of emotional responses (Craig, Reference Craig2009; Critchley, Melmed, Featherstone, Mathias, & Dolan, Reference Critchley, Melmed, Featherstone, Mathias and Dolan2002; Critchley, Wiens, Rotshtein, Ohman, & Dolan, Reference Critchley, Wiens, Rotshtein, Ohman and Dolan2004; Paulus & Stein, Reference Paulus and Stein2010). Similarly, hyperconnectivity between STS and DLPFC may underlie the role of the STS in speech processing related to emotion regulation (e.g. reappraisal), since those cognitive-behavioral strategies engage prefrontal cortices including the DLPFC (Gilmartin, Balderston, & Helmstetter, Reference Gilmartin, Balderston and Helmstetter2014; Goldin, McRae, Ramel, & Gross, Reference Goldin, McRae, Ramel and Gross2008). We provide additional discussion on the potential implications of altered STS connectivity in MDD and high RNT in the online Supplementary material. The question remains regarding the directionality of connectivity between the STS and other regions since our approach can only inform about coactivated regions. This is a topic that deserves further consideration given the paucity of reports addressing it (Hamilton et al., Reference Hamilton, Furman, Chang, Thomason, Dennis and Gotlib2011; Kumar, Stephan, Warren, Friston, & Griffiths, Reference Kumar, Stephan, Warren, Friston and Griffiths2007; Noppeney, Josephs, Hocking, Price, & Friston, Reference Noppeney, Josephs, Hocking, Price and Friston2008).
Limitations and future directions
This study has several limitations. First, a majority of participants in our sample were female (78%), resulting in a female:male ratio of 3.5:1, while most epidemiological data reported female:male prevalence ratios in MDD are approximately 2:1 (Salk, Hyde, & Abramson, Reference Salk, Hyde and Abramson2017). This overrepresentation of the female sex in our sample could reduce the generalizability of our findings. Second, we used a propensity score matching approach to minimize the effect of potential confounders. Our approach has some advantages, including the statistical efficiency of using a propensity score instead of using multiple covariates, no assumptions of linearity between covariates and outcomes, and a quasi-randomized design without model selections which could be biased by the researcher (Benedetto, Head, Angelini, & Blackstone, Reference Benedetto, Head, Angelini and Blackstone2018). However, the propensity score matching yielded smaller samples than initially obtained in the data collection process, which could lead to a loss of information that would have been held in the original data. We used PHQ as a covariate measuring the severity of depression to match the sample instead of HAMD, and the use of a different set of covariates would lead to a different matching set, although both MDD groups were matched for HAMD score as well. Also, the use of a regression approach might have yielded different results. Third, we relied on a self-report scale to measure the participants' level of RNT. As we stated, the important aspect of RNT is its perseverative nature, or the stickiness of the recurrent negative thoughts. Although the RRS can capture the trait aspect of RNT, it relies on the person's retrospective observation, and may not be enough to measure how RNT instantly varies in face of negative events. Fourth, our cross-sectional findings of altered functional connectivity do not inform directional influence between those brain regions as we stated in 4.3.
An important avenue for future work will be comparing altered functional connectivity prior, during, and after the antidepressant treatments, with the potential to use the current neural findings for the therapeutic monitoring factor as RNT symptoms change over time. From a therapeutic perspective, direct modulation of altered functional connectivity patterns described herein may also be of interest, exploring them as targets of diverse established and emerging neuromodulation techniques.
Supplementary material
The supplementary material for this article can be found at https://doi.org/10.1017/S0033291722002677
Acknowledgements
We would like to express our appreciation to CoBRE NeuroMap Investigators at LIBR, and thank all the research participants. We acknowledge the contributions of Sahib S. Khalsa, MD., Ph.D., Tim Collins, Dara Crittenden, Amy Peterson, Megan Cole, Lisa Kinyon, Lindsey Bailey, Courtney Boone, Natosha Markham, Lisa Rillo, Angela Yakshin, and the LIBR Assessment Team for diagnostic assessments and data collection, and Julie Arterbury, Leslie Walker, Amy Ginn, Bill Alden, Julie DiCarlo, and Greg Hammond for helping with MRI scanning. The authors acknowledge Jerzy Bodurka, Ph.D. (1964–2021) for his intellectual and scientific contributions to the establishment of the EEG, structural and functional MRI and neurofeedback processes that provided the foundation for the data collection, analysis and interpretation of findings for the present work.
Author contributions
Conceptualization and Methodology, AT, RK, MPP, and SMG; Formal analysis, AT; Data Curation, RK; Writing – Original Draft, AT, SMS, and SMG; Writing – Review & Editing, SMS, MM, RK, HP, MPP, and SMG; Resources, RK and MM; Supervision, MPP and SMG; Funding acquisition, MPP.
Financial support
This work has been supported in part by The William K. Warren Foundation, and the National Institute of General Medical Sciences Center Grant Award Number (1P20GM121312). The content is solely the responsibility of the authors and does not necessarily represent the official views of the National Institutes of Health.
Conflict of interest
Dr Paulus is an advisor to Spring Care, Inc., a behavioral health startup, he has received royalties for an article about methamphetamine in UpToDate.