Introduction
Anorexia nervosa (AN) commonly emerges during adolescence (Herpertz-Dahlmann, Reference Herpertz-Dahlmann2015) and is a serious psychiatric illness associated with high rates of relapse, an increased risk of mortality, and an often-protracted course (Khalsa et al., Reference Khalsa, Portnoff, McCurdy-McKinnon and Feusner2017). Since the beginning of the COVID-19 pandemic, the incidence of severe AN among adolescents worldwide has soared (Gilsbach et al., Reference Gilsbach, Plana, Castro-Fornieles, Gatta, Karlsson, Flamarique, Raynaud, Riva, Solberg, van Elburg, Wentz, Nacinovich and Herpertz-Dahlmann2022), further heightening the need to understand the neurocognitive processes that contribute to the disorder’s development and, for some, persistence. The disorder is characterized by severe, maladaptive caloric restriction, which results in significant weight loss and associated medical and psychological sequelae of malnourishment (American Psychiatric Association, 2013). Though food is generally considered a primary reward, individuals with AN behave as though high-calorie, high-fat foods are neither rewarding nor reinforcing and should be avoided. The persistence of this behavior, often in the face of serious adverse consequences, has led to interest in understanding whether differences in reinforcement learning processes contribute to the perpetuation of this complex illness (Bernardoni et al., Reference Bernardoni, Geisler, King, Javadi, Ritschel, Murr, Reiter, Rössner, Smolka, Kiebel and Ehrlich2018; DeGuzman, Shott, Yang, Riederer, & Frank, Reference DeGuzman, Shott, Yang, Riederer and Frank2017; Foerde et al., Reference Foerde, Walsh, Dalack, Daw, Shohamy and Steinglass2021; Frank et al., Reference Frank, DeGuzman, Shott, Laudenslager, Rossi and Pryor2018; Murray et al., Reference Murray, Strober, Le Grange, Schauer, Craske and Zbozinek2024; Wierenga, Reilly, Bischoff-Grethe, Kaye, & Brown, Reference Wierenga, Reilly, Bischoff-Grethe, Kaye and Brown2022).
Adaptive behavior relies on the ability to learn from feedback on past choices to guide future actions (Balleine & Dickinson, Reference Balleine and Dickinson1998). Evidence of disturbances in feedback learning have been identified in numerous psychiatric disorders, including depression (Mörkl, Blesl, Jahanshahi, Painold, & Holl, Reference Mörkl, Blesl, Jahanshahi, Painold and Holl2016), anxiety (Khdour et al., Reference Khdour, Abushalbaq, Mughrabi, Imam, Gluck, Herzallah and Moustafa2016), and obsessive-compulsive disorder (Endrass et al., Reference Endrass, Koehne, Riesel and Kathmann2013; Marzuki et al., Reference Marzuki, Tomić, Ip, Gottwald, Kanen, Kaser, Sule, Conway-Morris, Sahakian and Robbins2021). In AN, evidence of impaired learning from feedback has been identified in the acute phase of illness (Foerde & Steinglass, Reference Foerde and Steinglass2017; Foerde et al., Reference Foerde, Walsh, Dalack, Daw, Shohamy and Steinglass2021; Verharen et al., Reference Verharen, Danner, Schröder, Aarts, van Elburg and Adan2019), following weight restoration treatment (Foerde & Steinglass, Reference Foerde and Steinglass2017; Foerde et al., Reference Foerde, Walsh, Dalack, Daw, Shohamy and Steinglass2021), and among individuals who have recovered from illness (Ritschel et al., Reference Ritschel, Geisler, King, Bernardoni, Seidel, Boehm, Vettermann, Biemann, Roessner, Smolka and Ehrlich2017). In some studies, the ability to learn from feedback has also been found to relate to chronicity of illness such that individuals with a longer duration of illness were more impaired in a feedback learning task (Foerde & Steinglass, Reference Foerde and Steinglass2017).
While individuals with AN consistently report heightened sensitivity to punishment (Glashouwer, Bloot, Veenstra, Franken, & de Jong, Reference Glashouwer, Bloot, Veenstra, Franken and de Jong2014; Jappe et al., Reference Jappe, Frank, Shott, Rollin, Pryor, Hagman, Yang and Davis2011; Jonker, Glashouwer, Hoekzema, Ostafin, & de Jong, Reference Jonker, Glashouwer, Hoekzema, Ostafin and de Jong2020; Matton et al., Reference Matton, Goossens, Vervaet and Braet2015; Monteleone, Scognamiglio, Monteleone, Perillo, & Maj, Reference Monteleone, Scognamiglio, Monteleone, Perillo and Maj2014) and, less consistently, reduced sensitivity to reward (Atiye et al., Reference Atiye, Miettunen and Raevuori-Helkamaa2015), it is not clear whether impaired feedback learning in AN reflects problems in learning from positive feedback (i.e., reward), negative feedback (i.e., punishment or loss), or both. One study which used a probabilistic reversal learning task to measure learning from positive and negative feedback found that adolescents and young adults with AN had a higher rate of learning following negative feedback (i.e., monetary loss) relative to healthy control participants (HC), despite not differing in overall accuracy (Bernardoni et al., Reference Bernardoni, Geisler, King, Javadi, Ritschel, Murr, Reiter, Rössner, Smolka, Kiebel and Ehrlich2018). A slightly different pattern emerged in a subsequent study using the same task in a group of adolescents and young adults who had recovered from AN, such that the recovered AN group was less accurate overall and did not differ from HC in learning from negative feedback, despite having a greater difference in learning rates from negative relative to positive feedback (Bernardoni et al., Reference Bernardoni, King, Geisler, Ritschel, Schwoebel, Reiter, Endrass, Rössner, Smolka and Ehrlich2021). Adding yet further complexity, another study examined feedback learning using a probabilistic associative learning task in a group of individuals with AN and HC between 16 and 60 years old and found that the patients with AN had lower learning rates from both positive and negative prediction errors relative to HC (Wierenga et al., Reference Wierenga, Reilly, Bischoff-Grethe, Kaye and Brown2022).
Though data are limited, these studies suggest that stage of illness and duration of illness may be related to learning rates in the setting of both positive and negative feedback. In particular, it is of interest to understand whether feedback learning alterations are present among those who are not marked by longer-term illness, pointing to a need to focus on a narrower range of illness stage and duration. Additionally, the pattern of results underscores the importance of assessing the contributions of both positive and negative feedback in this population. Finally, given differences in how children, adolescents, and adults learn from probabilistic feedback (Cohen et al., Reference Cohen, Asarnow, Sabb, Bilder, Bookheimer, Knowlton and Poldrack2010; Davidow et al., Reference Davidow, Foerde, Galván and Shohamy2016; Jones et al., Reference Jones, Somerville, Li, Ruberry, Powers, Mehta, Dyke and Casey2014; Master et al., Reference Master, Eckstein, Gotlieb, Dahl, Wilbrecht and Collins2020; van den Bos et al., Reference van den Bos, Cohen, Kahnt and Crone2012), it may be important to focus more specifically on adolescence, the time period when illness often emerges, to understand the role of feedback learning in AN. Establishing the presence of feedback learning deficits in a well-powered study of adolescents is important for the pursuit of future longitudinal studies that will be needed to disentangle the issue of illness duration and dysfunctional learning.
In the present study, we examined positive and negative feedback learning among adolescents with AN and HC between the ages of 12 and 18 years using a probabilistic reinforcement learning task which has been shown to capture developmental differences in feedback learning (van den Bos et al., Reference van den Bos, Cohen, Kahnt and Crone2012; van den Bos, Güroğlu, van den Bulk, Rombouts, & Crone, Reference van den Bos, Güroğlu, van den Bulk, Rombouts and Crone2009). Based upon a prior study (Bernardoni et al., Reference Bernardoni, Geisler, King, Javadi, Ritschel, Murr, Reiter, Rössner, Smolka, Kiebel and Ehrlich2018), we hypothesized that adolescents with AN would not differ from HC in overall task performance but would show a higher rate of learning from negative feedback. We also conducted exploratory analyses to examine associations between feedback learning and eating disorder severity, duration of illness, and self/parent-report measures of reward and punishment sensitivity.
Method
Participants
Participants were 76 females with AN and 38 female HC, ages 12–18 years, who completed the probabilistic feedback learning task as part of baseline procedures in a longitudinal neuroimaging study examining neural systems related to course of illness in AN during adolescence (study sample and procedures are described here https://github.com/Columbia-Center-for-EDs/Longitudinal-Assessment-of-Teens-with-Anorexia-Nervosa). Individuals were included if they were assigned female at birth, had no major medical or neurologic illness, had an estimated IQ above 80, and normal (or corrected to normal) vision. Patients with AN met DSM-5 criteria for a diagnosis of AN restricting (ANR) or binge-eating/purging (ANBP) subtype (American Psychiatric Association, 2013) and were receiving inpatient or outpatient treatment (or in rare cases deferring treatment at the time of study enrollment). All participants completed study procedures as part of their initial baseline assessment. Psychotropic medications within 4 weeks of study participation were exclusionary for the patient group, with the exception of antidepressants (i.e., a stable dose of an antidepressant was not exclusionary). All psychotropic medications were exclusionary for HC participants. Additional exclusion criteria for AN included a co-occurring diagnosis that required specialized treatment (e.g., substance use disorder, psychotic or bipolar illness), medical instability, or high/imminent risk for suicide. HC had no current or lifetime history of psychiatric illness (1 HC participant met criteria for a past diagnosis of Specific Phobia (spiders) and 1 had a past diagnosis of encopresis) and had a body mass index (BMI) between the 5th and 85th percentile for sex and age. HC were group-matched for age and ethnicity. The authors assert that all procedures contributing to this work comply with the ethical standards of the relevant national and institutional committees on human experimentation and with the Helsinki Declaration of 1975, as revised in 2008.
Procedures
Eating disorder diagnosis was established via the Eating Disorders Assessment for DSM-5 (Sysko et al., Reference Sysko, Glasofer, Hildebrandt, Klimek, Mitchell, Berg, Peterson, Wonderlich and Walsh2015) and co-occurring psychiatric diagnoses were assessed using the Kiddie Schedule for Affective Disorders and Schizophrenia (K-SADS; Kaufman et al., Reference Kaufman, Birmaher, Brent, Rao, Flynn, Moreci, Williamson and Ryan1997) for participants under age 18 and the Structured Clinical Interview for DSM-5 (SCID; First, Reference First2014) for those participants who were 18 at time of study participation. Eating disorder symptoms and severity were assessed using the Eating Disorder Examination Questionnaire (EDE-Q) (Fairburn, Reference Fairburn2008) and the Age of Onset Questionnaire was used to determine duration of illness (Ranzenhofer et al., Reference Ranzenhofer, Jablonski, Davis, Posner, Walsh and Steinglass2022). Estimated IQ was assessed using the Wechsler Abbreviated Scale Intelligence (WASI, Wechsler, Reference Wechsler2011). Reward and punishment sensitivity were measured using the Behavioral Inhibition System/Behavioral Activation System (BIS/BAS; Carver & White, Reference Carver and White1994), a 24 item self-report measure which was completed by the adolescent, and the Sensitivity to Reward/Sensitivity to Punishment for children (SPSRQ-C; Torrubia, Avile, Molto & Caseras, Reference Torrubia, Avila, Molto and Caseras2001), a 33-item parent-report measure of reward and punishment sensitivity. Height and weight were measured by stadiometer and Detecto scale, respectively, and used to calculate percent median body mass (%mBMI; current BMI/50th percentile BMI for age and sex × 100) to compare the individual’s BMI to the reference population (Society for Adolescent Health and Medicine, 2022).
This study was approved by the Institutional Review Board of the New York State Psychiatric Institute and adult participants provided written informed consent; individuals under 18 years of age gave assent and a parent or guardian provided consent.
Feedback learning task
Participants completed a probabilistic feedback learning task (van den Bos et al., Reference van den Bos, Güroğlu, van den Bulk, Rombouts and Crone2009) previously used in developmental studies (van den Bos et al., Reference van den Bos, Cohen, Kahnt and Crone2012; van den Bos et al., Reference van den Bos, Güroğlu, van den Bulk, Rombouts and Crone2009). In this type of task, participants learn to associate choices and outcomes through trial and error. Due to the probabilistic nature of the feedback, there is no one-to-one mapping between choices and outcomes, and optimal learning involves the use of response-contingent feedback across multiple trials to incrementally learn the most probable outcome. The task was administered using E-Prime 2.0 (Psychology Software Tools, Pittsburgh, PA) and consisted of two runs with 100 trials per run. During each run, two pairs of stimuli (AB and CD) were presented. Stimuli were pictures of everyday items (e.g., AB: bell and bottle, CD: book and bike; see Figure 1) and remained consistent throughout a run but changed between runs. Stimulus pairs were each presented 50 times per run in pseudo-random order. On each trial, participants chose the right or left stimulus by button press within a 2.5 s response window. After a choice was made, feedback was displayed for 1 s via a green checkmark for positive feedback and a red cross for negative feedback, followed by a jittered intertrial interval (min 500 ms, max 6000 ms, mean 1465.25 ms). If participants did not make a choice within the response window, “too slow” appeared on the screen. Feedback was probabilistic such that choice of stimulus A or C led to positive feedback on 80% and 70% of trials, respectively, whereas choice of stimulus B or D was associated with positive feedback on 20% and 30% of trials, respectively. As in previous studies, performance on the probabilistic learning task was assessed in terms of making optimal choices (i.e., the proportion of trials on which participants selected the stimulus most likely to be correct) Knowlton et al., Reference Knowlton, Squire and Gluck1994, Reference Knowlton, Mangels and Squire1996; Poldrack et al., Reference Poldrack, Clark, Paré-Blagoev, Shohamy, Creso Moyano, Myers and Gluck2001; Gluck et al., Reference Gluck, Shohamy and Myers2002; Hopkins et al., Reference Hopkins, Myers, Shohamy, Grossman and Gluck2004; Shohamy et al., Reference Shohamy, Myers, Grossman, Sage, Gluck and Poldrack2004; Foerde et al., Reference Foerde, Knowlton and Poldrack2006;Foerde et al., Reference Foerde, Race, Verfaellie and Shohamy2013). That is, on a given trial, a participant could make the optimal choice and be scored as being correct but experience the receipt of negative feedback. Participants were told that though they would not be able to win points on every trial, they should try to earn as many points as possible.
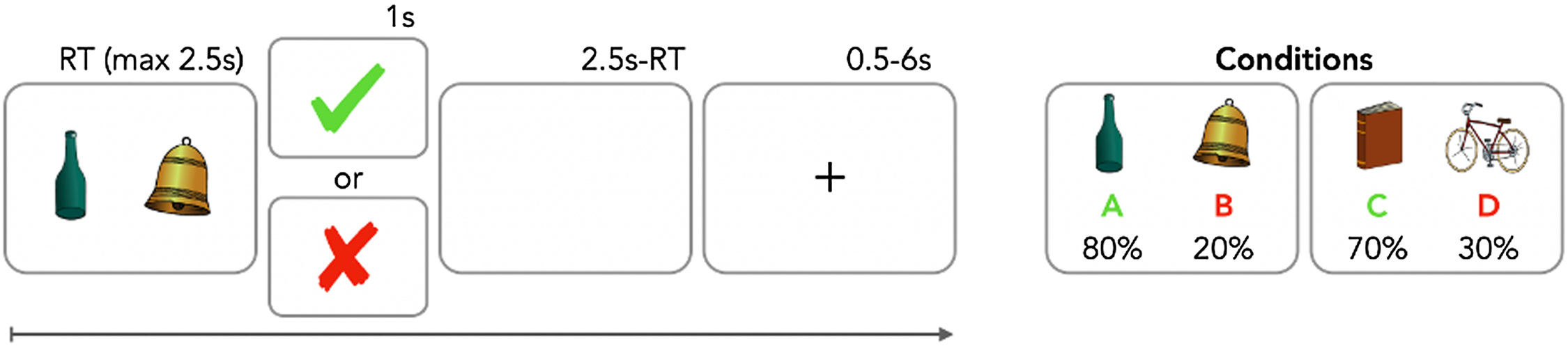
Figure 1. Probabilistic feedback learning task: Two pairs of stimuli are presented to the participants during each trial. Participants choose one stimulus by pressing the (right or left) button and probabilistic feedback with (1) AB pair resulting in 80% positive feedback for A and 20% for B and (2) CD pair resulting in 70% positive feedback for C and 30% for D.
Computational modeling of learning
In order to examine whether there were differences in how individuals learn that may not be apparent in the standard optimal choice measure, a reinforcement learning (RL) model was fit to each participant’s behavioral data to assess subcomponents of reward learning (van den Bos et al., Reference van den Bos, Cohen, Kahnt and Crone2012). The RL model used the prediction error (δ) to update Q-values (Q), or expected values, associated with each stimulus (A, B, C, or D). Q-values represented the (expected) probability (between 0 and 1) that selecting a stimulus (e.g., B) would result in earning a point. Whenever feedback was better than expected, the model generated a positive prediction error, which “increased” the Q-value of the chosen stimulus. When feedback was worse than expected, the model generated a negative prediction error, which “decreased” the decision weight of the chosen stimulus (e.g., stimulus B). The impact of the prediction error was scaled by a learning rate parameter (α), calculated separately for positive (αpos) and negative feedback (αneg). That is, the learning rate indicates the extent to which participants update their expectations about the stimuli in response to feedback. We used the Maximum a posteriori estimation for model fitting (Daw, Gershman, Seymour, Dayan, & Dolan, Reference Daw, Gershman, Seymour, Dayan and Dolan2011; Spektor & Kellen, Reference Spektor and Kellen2018; see supplemental materials for more detail on the model and fitting procedure).
Statistical analysis
Participant demographics and clinical characteristics
Demographic and clinical characteristics were compared using independent samples t-test and chi-square analyses within the IBM SPSS Statistics 28 analysis package. Learning rates were calculated in R (code available on OSF; https://osf.io/tu2xm/). Alpha was set at 0.05.
Behavioral performance
To test for potential group differences in learning over time, the percentage of optimally correct choices (i.e., choice of the stimulus with higher probability of being correct in a pair) per block of 20 trials was calculated for each participant, resulting in a total of five blocks. Consistent with prior studies using this task, the two runs used different stimulus pairs and were thus collapsed for analyses. Independent samples t-test was used to test for group differences in overall performance, performance on AB (high probability 80%/20%) and CD (low probability 70%/30%) pairs, and response time.
Learning performance
Repeated measures analysis of covariance (rmANCOVA) was used to examine overall performance with Group (HC/AN) as a between-subject factor, Block (1–5) and Probability (AB/CD) as within-subject factors, controlled for age and estimated IQ. Mauchly’s test of sphericity was used to assess for violations of the assumption of sphericity and the Greenhouse-Geisser correction was applied when indicated.
Win-stay/lose-shift
Following previous studies (van den Bos et al., Reference van den Bos, Güroğlu, van den Bulk, Rombouts and Crone2009; van den Bos et al., Reference van den Bos, Cohen, Kahnt and Crone2012), feedback sensitivity was investigated by assessing how often participants chose the same stimulus after receiving positive feedback (win-stay) or chose the other stimulus after receiving negative feedback (lose-shift). Win-stay behavior was determined by calculating the proportion of choice repetitions after positive feedback and the total number of positive feedback events. Similarly, lose-shift behavior was determined by calculating the proportion of choice shifts following negative feedback and the total number of negative feedback events. Win-stay and lose-shift behavior were compared in separate ANCOVAs with Group as a between-subject factor and controlling for age and estimated IQ.
Reinforcement learning parameters
Model fit was assessed using Bayesian Information Criterion (BIC) using the formula k*ln(n) -2*loglikelihood [i.e., 3 parameters*ln(200 trials*114 participants)-2*LL]. Potential group differences in model fit, stochasticity variable β, and learning rates (αwin and αloss) were compared in separate ANCOVAs with Group as a between-subject factor and controlling for age and estimated IQ.
Associations with clinical variables
Pearson’s partial correlation controlling for age and IQ was used to test for associations between estimated learning rates and clinical variables (%mBMI, duration of illness, EDE-Q global, SPSRQ-C subscales, and BIS/BAS subscales). Bonferroni correction was used to correct for multiple comparisons.
Results
Participant demographics and clinical characteristics (see Table 1)
Of participants with AN, 73.7% (n = 56) had the restricting subtype and 26.3% (n = 20) had the binge-eating/purging subtype. The groups were well matched in age, estimated IQ and self-reported ethnicity but differed in self-reported racial composition (26 individuals did not provide data for race). Of the 76 participants with AN, 25 (32.9%) met criteria for a co-occurring disorder with 10 participants (13.2%) meeting criteria for more than one comorbid condition. Specifically, 18 participants (23.7%) had a co-occurring anxiety disorder, 13 (17.1%) met criteria for depression, 4 individuals (5.3%) had a secondary diagnosis of obsessive-compulsive disorder, and one participant met criteria for ADHD (1.3%). Fifteen patients with AN (19.7%) were on a stable dose of an antidepressant at time of study participation. Adolescents with AN scored significantly higher on the EDE-Q relative to HC (Mean HC: 0.49 ± 0.7; Mean AN: 3.51 ± 1.7; t 1,108 = −10.5, p < 0.001).
Table 1. Demographicsand clinical characteristics
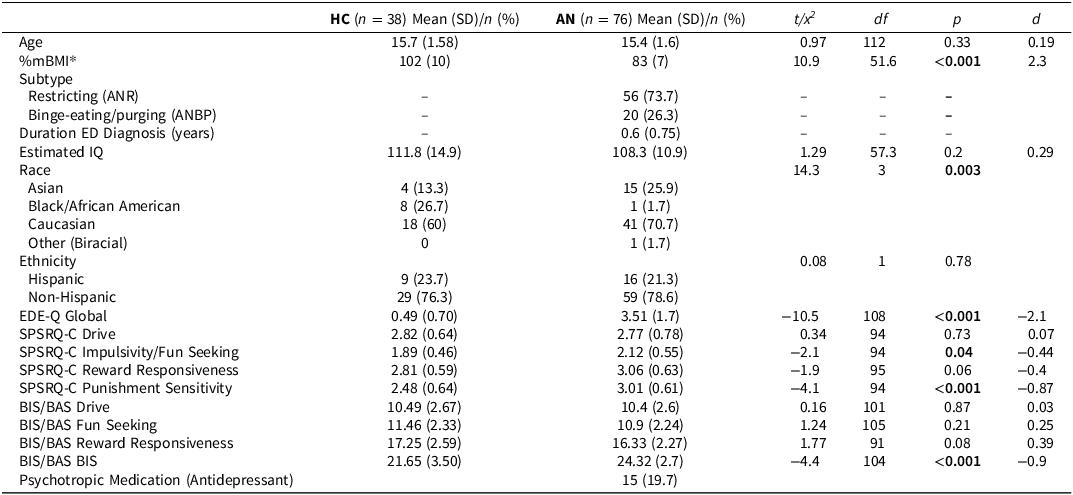
EDE-Q = Eating Disorder Examination Questionnaire; SPSRQ-C = Sensitivity to Punishment and Reward Questionnaire – Child (completed by parent); BIS/BAS = Behavioral Inhibition Scale/Behavioral Activation Scale (completed by participant).
Data are missing for: 4 AN for duration of illness; 8 HC and 18 AN for Race; 1 AN for Ethnicity; 2 HC and 2 AN for the EDE-Q, 3 HC and 8 AN for the BIS/BAS Drive subscale,1 HC and 6 AN for the BIS/BAS Fun-Seeking subscale, 6 HC and 15 AN for the BIS/BAS Reward Responsiveness subscale; 1 AN and 7 AN for the BIS/BAS BIS subscale; 2 HC and 16 AN for the SPSRQ-C Drive subscale; 2 HC and 16 AN for the SPSRQ-C Impulsivity/Fun-seeking subscale; 2 HC and 15 AN for the SPSRQ-C Reward Responsivity subscale; 2 HC and 16 AN for the SPSRQ-Punishment Sensitivity subscale.
* Percent median BMI; Severity of malnutrition: 80–90%: (mild), 70–79% (moderate),<70% (severe) (Society for Adolescent Health and Medicine, 2022).
Reward and punishment sensitivity
Relative to HC, adolescents with AN had significantly greater sensitivity to punishment on both the self-report BIS/BAS BIS subscale (Mean HC: 21.65 ± 3.5; Mean AN: 24.3 ± 2.7; t 1,104 = −4.4, p < 0.001) and the SPSRQ-C Punishment Sensitivity parental report (Mean HC 2.48 ± 0.64; Mean AN: 3.01 ± 0.61; t 1,94 = −4.1, p < 0.001). The patient group also scored higher on the SPSRQ-C Impulsivity/Fun-seeking subscale relative to HC (Mean HC 1.89 ± 0.46; Mean AN: 2.12 ± 0.55; t 1,94 = −2.1, p = 0.04) but this result did not survive correction for multiple comparisons. There were no other significant group differences on either the BIS/BAS or SPSRQ-C (see Table 1).
Feedback learning task behavioral performance
Learning performance
The Block (5) x Probability (AB, CD) x Group (HC, AN) rmANCOVA, controlling for age and estimated IQ, showed a main effect of block (F3.2,357 = 2.6, p = 0.048, η2 = 0.02), with participants making more correct choices over time, suggesting all participants were appropriately learning the task (see Figure 2, Panel a). There was also a significant effect of probability (F1,110 = 6.4, p = 0.01, η2 = 0.06) such that participants were more accurate on AB (80% - 20%) trials than the CD (70% - 30%) trials. In line with our predictions, there was no difference in performance between AN and HC groups (F1,110 = 0.09, p = 0.77, η2 = 0.001). An independent samples t-test found no group differences in overall percentage of correct responses (HC: 64.8% ± 13.8; AN: 64.9% ± 13.4, p = 0.99), percentage of correct responses on AB pairs (HC: 65.2% ± 14.2; AN 65.3% ± 14.4, p = 0.97) or CD pairs (HC: 64.5% ± 14.2; AN 64.4% ±13.9; p = 0.98) or in response time for correct responses (HC: 595.3 ms ± 159.1; AN 630.8 ms ± 208; p = 0.36).
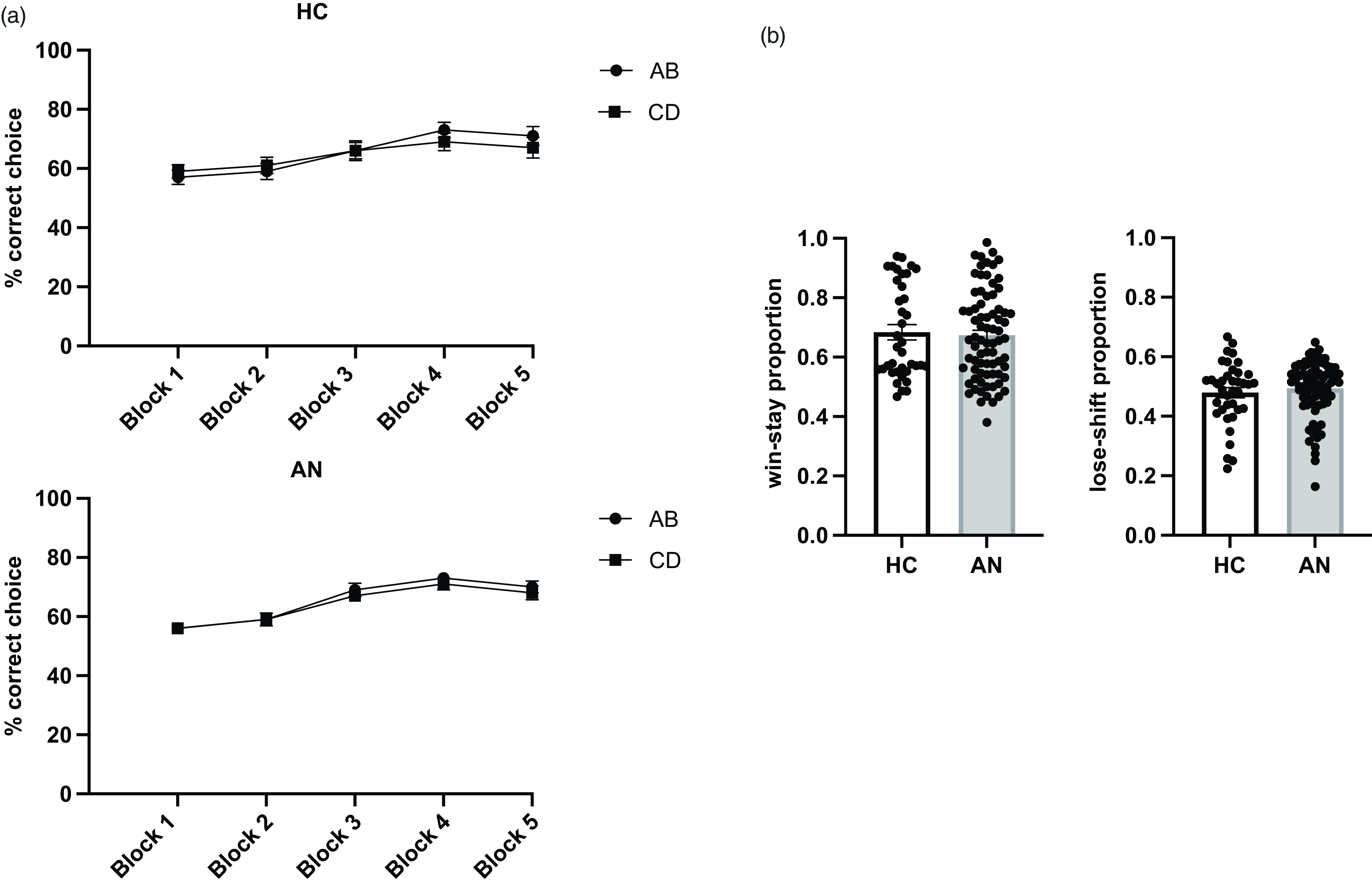
Figure 2. Behavioral Task Performance. (a) Percentage of correct choices (i.e., optimally correct) per block of 20 trials, error bars represent SEM. HC and adolescents with AN similarly learned to make more correct choices over time. (b) Percentages of win-stay and lose-shift choices by group, error bars represent SEM. There were no differences between HC and AN participants in the proportion of choosing the same stimulus after positive feedback (win-stay) or choosing the other stimulus after negative feedback (lose-shift).
Reinforcement learning parameters
Model fit did not differ significantly between HC and AN (HC mean BIC: −423.93 ± 117.1; AN mean BIC: −412.63 ± 154.9; F1,110 = 0.31, p = 0.58, η2 = 0.003), enabling parameter estimates to be compared between groups. Analysis of potential group differences in learning rates (αwin, αloss) identified a main effect of group on αwin (F1,110 = 9.78, p = 0.002, η2 = 0.08) but not αloss (F1,110 = 1.4, p = 0.23, η2 = 0.01). Post-hoc t-tests showed that patients with AN had a significantly lower rate of learning from positive feedback relative to HC (αwin: t1,112 = 3.3, p = 0.001, Cohen’s d = 0.65, see Figure 3a) but did not differ in rate of learning from negative feedback (αloss: t1,112 = 1.2, p = 0.23, Figure 3b). Importantly, there was no main effect of group on the choice stochasticity parameter β (F1,110 = 1.5, p = 0.22, η2 = 0.01), indicating that differences in learning rates were not due to differences in stochasticity.
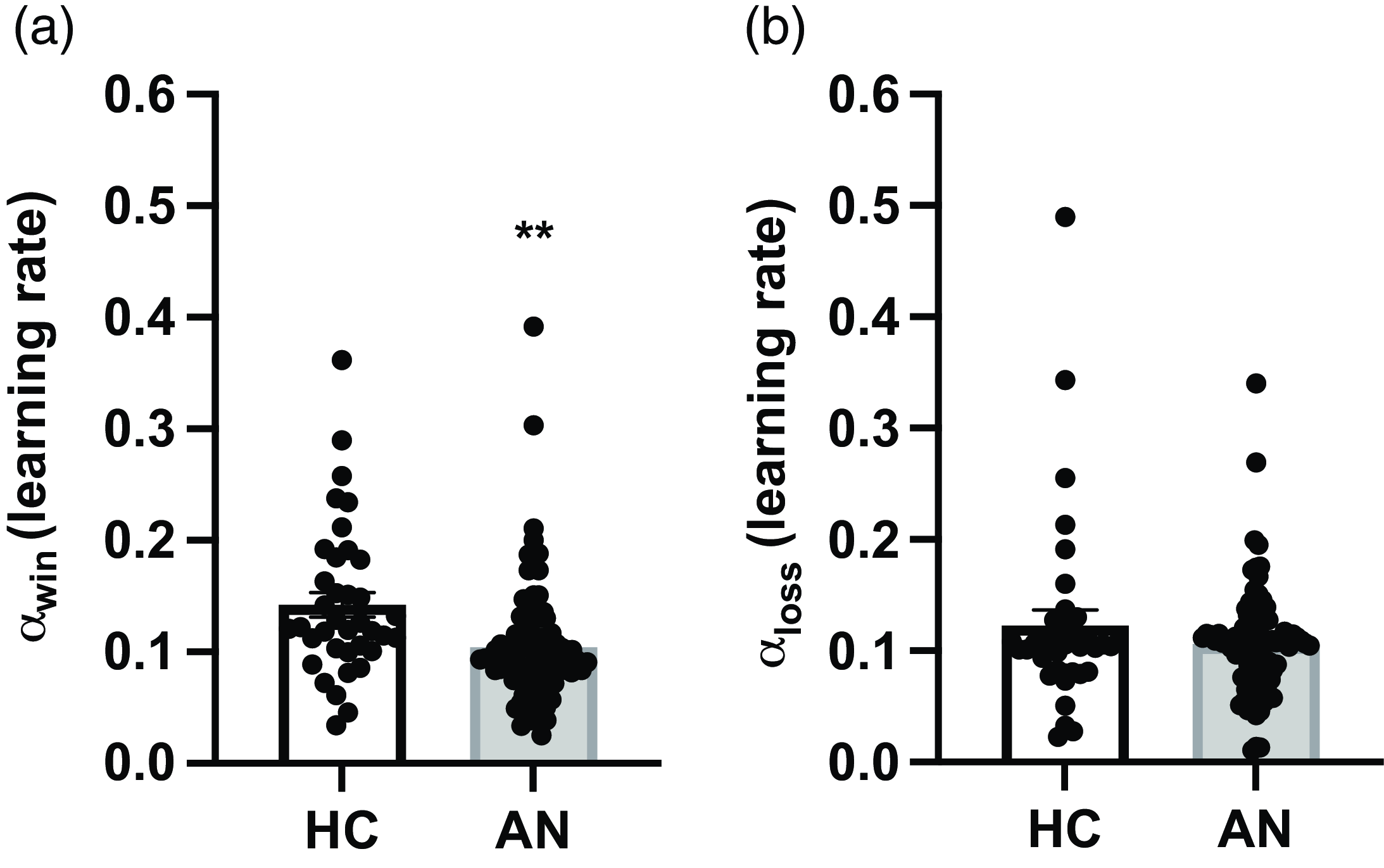
Figure 3. Reinforcement Learning Parameters. Estimated learning rates for positive (αwin) and negative feedback (αloss) by diagnostic group. Error bars represent SEM. (a) Participants with AN had a significantly lower rate of learning from positive feedback (αwin) relative to HC. (b) There was no group difference in rate of learning from negative feedback (αloss).
Associations between learning rate parameters and clinical variables
Among AN, both learning rate parameters (αwin and αloss) were associated with parental report of reward responsivity on the SPSRQ-C. A higher αwin was significantly associated with lower parental report of reward responsivity (r = −0.35, p = 0.02), whereas a higher αloss was associated with a greater parental report of reward responsivity (r = 0.34, p = 0.03), but neither result survived correction for multiple comparisons. There were no other significant associations between either learning rate parameter and any clinical variables, including %mBMI, duration of illness, EDE-Q, or reward or punishment sensitivity on the BIS/BAS or SPSRQ.
Sensitivity analyses
There were no significant differences in task behavior, including reinforcement learning rates, between the 56 patients with ANR and the 20 patients with ANBP (see supplementary materials, Table S1). There were also no significant differences in overall task performance, win-stay/lose-shift behavior, or reinforcement learning parameters between the 25 participants with AN with a comorbid psychiatric disorder and the 51 participants without a co-occurring illness (see supplementary materials, Table S2).
Discussion
This study examined reinforcement learning from both positive and negative feedback in acutely ill adolescents with AN, largely within the first year of illness, as compared with HC. While questionnaire-based reports of sensitivity to punishment by patients and their parents were higher among AN, patients did not show greater learning from negative feedback in a reinforcement learning task. Rather, and contrary to the study hypothesis, adolescents with AN did not differ from HC in learning from negative feedback but had a significantly lower rate of learning from positive feedback.
Although overall task performance did not differ between groups, computational analyses of the learning processes underlying choice behavior identified a circumscribed reduction in learning from positive feedback in the adolescents with AN relative to HC. This finding, which suggests that a difference in reward processing among adolescents with AN is identifiable within the first year of illness, adds a new dimension to understanding potential differences in reinforcement learning in AN. Results from the handful of studies which have examined how individuals with AN learn from positive and negative feedback have been heterogenous: some prior research investigated feedback learning in adolescents and emerging adults with AN (Bernardoni et al., Reference Bernardoni, Geisler, King, Javadi, Ritschel, Murr, Reiter, Rössner, Smolka, Kiebel and Ehrlich2018) and recovered from AN (Bernardoni et al., Reference Bernardoni, King, Geisler, Ritschel, Schwoebel, Reiter, Endrass, Rössner, Smolka and Ehrlich2021) while others included individuals spanning adolescence through age 60 (Wierenga et al., Reference Wierenga, Reilly, Bischoff-Grethe, Kaye and Brown2022). Here we focused on adolescents only, which may be useful to examine a construct known to change across the lifespan (Cutler et al., Reference Cutler, Apps and Lockwood2022; Nussenbaum & Hartley, Reference Nussenbaum and Hartley2019).
In line with prior research examining feedback learning in adolescents and emerging adults with AN (Geisler et al., Reference Geisler, Ritschel, King, Bernardoni, Seidel, Boehm, Runge, Goschke, Roessner, Smolka and Ehrlich2017) or recovered from AN (Ritschel et al., Reference Ritschel, Geisler, King, Bernardoni, Seidel, Boehm, Vettermann, Biemann, Roessner, Smolka and Ehrlich2017), we did not find a difference in overall task performance measures between patients and healthy teens. In contrast, impaired task performance has been identified in adults with AN, who tend to have a longer duration of illness (Foerde & Steinglass, Reference Foerde and Steinglass2017; Verharen et al., Reference Verharen, Danner, Schröder, Aarts, van Elburg and Adan2019). It may be that subtle learning differences which are present early in the course of AN evolve into actual deficits over time with persistent illness, or that more pronounced differences at baseline are associated with a prolonged course of illness. In one study examining feedback learning in adults with AN, participants tended to perform worse the longer they had been ill (Foerde & Steinglass, Reference Foerde and Steinglass2017). While we did not find an association between impaired feedback learning and duration of illness, the constricted age range and very recent onset of illness in our sample (less than one year, on average) likely reduced our ability to assess this relationship.
The present findings differ from some previous studies reporting increased learning from negative feedback (i.e., monetary loss) (Bernardoni et al., Reference Bernardoni, Geisler, King, Javadi, Ritschel, Murr, Reiter, Rössner, Smolka, Kiebel and Ehrlich2018; Bernardoni et al., Reference Bernardoni, King, Geisler, Ritschel, Schwoebel, Reiter, Endrass, Rössner, Smolka and Ehrlich2021) while others have identified reduced learning following both positive and negative feedback (Wierenga et al., Reference Spear2022). To our knowledge, the present study is the largest to date to examine feedback learning in AN as well as the first to focus exclusively on adolescents in an early stage of illness, which may contribute to the diverging patterns. Adolescence is a period characterized by rapid neurodevelopment (Spear, Reference Wierenga, Reilly, Bischoff-Grethe, Kaye and Brown2013) that includes changes in how individuals learn from reward and punishment. Recent evidence from a large study of healthy adolescents found that punishment learning improved with age (Pauli et al., Reference Pauli, Brazil, Kohls, Klein-Flügge, Rogers, Dikeos, Dochnal, Fairchild, Fernández-Rivas, Herpertz-Dahlmann, Hervas, Konrad, Popma, Stadler, Freitag, De Brito and Lockwood2023). If so, persistent illness may impair normal development of feedback learning in adolescents with AN and, over time, result in a deficit in learning from both positive and negative feedback as has been observed in other studies in AN. Future work examining feedback learning longitudinally in adolescents with and without AN will be an important next step in understanding potential differences in reinforcement learning in AN.
Another possibility is that differences between the tasks used across studies, despite overarching similarities, play a role. Some tasks include continuous contingency reversals (Bernardoni et al., Reference Bernardoni, Geisler, King, Javadi, Ritschel, Murr, Reiter, Rössner, Smolka, Kiebel and Ehrlich2018; Bernardoni et al., Reference Bernardoni, King, Geisler, Ritschel, Schwoebel, Reiter, Endrass, Rössner, Smolka and Ehrlich2021), whereas others include distinct gain and loss conditions wherein feedback may be ambiguous with regard to choice accuracy (Wierenga et al., Reference Wierenga, Reilly, Bischoff-Grethe, Kaye and Brown2022). Increasing data suggest that learning parameters do not consistently generalize across tasks (Eckstein et al., Reference Eckstein, Master, Xia, Dahl, Wilbrecht and Collins2022). This is due, at least in part, to the fact that the optimal learning rate depends upon the particular task (e.g., a very high learning rate in a given context may yield suboptimal task performance but may be optimal in a different context) (Nussenbaum & Hartley, Reference Nussenbaum and Hartley2019). Systematic task analysis may reveal further cognitive processes that play a differential role in learning among individuals with AN, such as the influence of uncertainty and the relative role of working memory across tasks (Collins et al., Reference Collins, Ciullo, Frank and Badre2017).
The disconnect between sensitivity to punishment via self-report and learning from negative feedback underscores the possibility that perceptions of behavior do not map directly onto neurocognitive processes. Consistent with numerous prior studies (Frank et al., Reference Frank, DeGuzman, Shott, Laudenslager, Rossi and Pryor2018, Glashouwer et al., Reference Glashouwer, Bloot, Veenstra, Franken and de Jong2014; Jappe et al., Reference Jappe, Frank, Shott, Rollin, Pryor, Hagman, Yang and Davis2011; Jonker et al., Reference Jonker, Glashouwer, Hoekzema, Ostafin and de Jong2020; Matton et al., Reference Matton, Goossens, Vervaet and Braet2015; Monteleone et al., Reference Monteleone, Scognamiglio, Monteleone, Perillo and Maj2014), self-reported sensitivity to punishment (BIS/BAS-BIS) was significantly greater among youth with AN relative to healthy teens. This finding was mirrored in parental assessments (SPSRQ-C), with the parents of teens with AN reporting greater sensitivity to punishment in their children relative to the parents of the control group. Yet, patients with AN were similar to HC in learning from negative feedback and differed only in learning from positive feedback. From a treatment development perspective, this provides constructs for therapeutic targets beyond those suggested by self-report (e.g., taking into account that positive feedback may not be integrated as easily among these patients in addition to a focus on punishment sensitivity). From a mechanistic perspective, reduced learning from positive feedback suggests potential alterations in dopaminergic function which are integral to reinforcement learning (Frank et al., Reference Frank, DeGuzman, Shott, Laudenslager, Rossi and Pryor2004). In illnesses that involve changes in dopamine levels (e.g., Parkinson’s disease) decreased dopamine levels are associated with poorer learning from positive feedback (Frank et al., Reference Frank, DeGuzman, Shott, Laudenslager, Rossi and Pryor2004; Shohamy et al., Reference Shohamy, Myers, Onlaor and Gluck2004). Although empirical data are scarce, dysfunctional dopamine function has been suggested in AN (Kontis & Theochari, Reference Kontis and Theochari2012; Södersten et al., Reference Södersten, Bergh, Leon and Zandian2016) and emerging data point to the importance of diet for dopamine function (Mallick et al., Reference Mallick, Basak and Duttaroy2019; Dyall, Reference Dyall2015).
This study had several strengths, including a large, diverse, and well-characterized sample of adolescents with AN who were largely within the first year of illness. The computational modeling approach allowed us to examine a more subtle alteration in reinforcement learning using a model that fit patients and controls equally well, which can be a challenge when comparing clinical and non-clinical groups. Additionally, none of the participants were receiving dopaminergic medications, which is important when examining reward-based processes that may be influenced by dopaminergic function. The cross-sectional design limits our ability to examine how learning from feedback relates to trajectory of illness, but the participants in the present study are enrolled in an ongoing longitudinal study of adolescent-onset AN, providing further opportunity to assess how differences, and deficits, in learning from feedback relate to severity and course of illness.
Conclusions
This study examined learning from positive and negative feedback on a probabilistic learning task in a large sample of adolescents with AN and age-matched healthy teens. Despite both self- and parental report of increased sensitivity to punishment in the AN group, the teens with AN had a circumscribed alteration in learning from positive feedback as assessed in a computational model but did not differ from HC in overall task performance or learning from negative feedback. These results suggest that differential feedback sensitivity is identifiable early in the course of illness, even in the absence of more global deficits in task performance more commonly observed in older cohorts with AN. Longitudinal research is a key next step to explore how impaired reward-based learning may relate to trajectory of illness in youth with AN.
Supplementary material
For supplementary material accompanying this paper visit https://doi.org/10.1017/S1355617724000237
Acknowledgements
None.
Funding statement
This work was supported by the National Institute of Mental Health (JS, JP, R01 MH110445), (JS, K24 MH113737), (Evelyn Attia, T32 MH096679).
Competing interests
Jonathan Posner has received support from Takeda (formerly Shire), Aevi Genomics, and Innovation Sciences. Joanna E. Steinglass receives royalties from UpToDate, and honoraria from Springer. The remaining authors report no competing interests.