Introduction
On 31 December 2019, a cluster of cases of pneumonia of unknown aetiology was detected in Wuhan City, Hubei Province, China [Reference Gralinski and Menachery1]. On 7 January 2020, the Chinese authorities identified and announced a novel type of coronavirus as the cause of the disease [2]. On 30 January 2020, the World Health Organization (WHO) declared the 2019-nCoV outbreak a Public Health Emergency of International Concern [Reference Sohrabi3] and a few days later announced the official name of the virus as Severe Acute Respiratory Syndrome Coronavirus 2 (SARS-CoV-2) and the disease as Coronavirus Disease 2019 (COVID-19) [Reference Zhao4]. COVID-19 was declared a pandemic on 11 March 2020 by the WHO.
The first case of COVID-19 in Nigeria was confirmed on 27 February 2020. The case was a 44-year old Italian citizen who arrived Nigeria through the Murtala Mohammed International Airport, Lagos, on a flight via Milan, Italy [5]. This index case led to the activation of COVID-19 Public Health Emergency Operation Centers (PHEOC) at national and sub-national levels, with associated active case finding via contact tracing. By 9 March 2020, 217 contacts were linked to this index case [5], out of which 136 (63.0%) were under follow-up, with one contact confirmed positive [Reference Ebenso and Otu6]. The 14-day follow-up for contacts of the index case ended on 12 March 2020. During this period, two additional unlinked cases were reported in Nigeria. In addition, 42 suspected cases were identified across seven states in Nigeria namely the Federal Capital Territory (FCT), Edo, Kano, Lagos, Ogun, Rivers and Yobe [5].
Since the confirmation of the first COVID-19 case in Nigeria, cases and deaths have risen steadily in the country, although the government has implemented public health interventions – e.g. advocacy for physical distancing, complete and partial lockdown, and ban on large public gatherings including at churches and mosques – to contain or mitigate spread. As of 6 June 2020, 35 (out of 36) states, plus the FCT, have reported at least one confirmed COVID-19 case. A descriptive analysis of the clinical characteristics, treatment modalities and outcomes of the first 32 COVID-19 patients admitted to Mainland Hospital in Lagos State, Nigeria, found that two-thirds of patients were male, and the mean age was 38.1 years [Reference Bowale7]. This early analysis however is insufficient to provide a national overview of COVID-19 epidemiology in Nigeria. The Nigeria Centre for Disease Control (NCDC) coordinates the public health response to COVID-19 in the country. Through NCDC's surveillance and laboratory network as well as coordination of state PHEOCs, epidemiological information on COVID-19 cases are captured into a real-time networked platform called Surveillance Outbreak Response Management and Analysis System (SORMAS). This forms the basis for the release of daily situation reports for COVID-19 on NCDC COVID-19 microsite [8]. By 6 June, thousands of individual records with laboratory diagnosis contained on SORMAS offered opportunities to expand and explore country-specific epidemiologic and clinical characteristics of COVID-19 from the onset of the outbreak. This study aims to provide the initial descriptive epidemiology of COVID-19 in Nigeria, with emphasis on the disease magnitude and patterns in terms of person, place and time.
Methods
Study design, period and settings
We conducted a retrospective analysis of Nigeria surveillance data between 27 February and 6 June 2020. Nigeria is administratively divided into 36 states plus the FCT, which are zoned across six geopolitical areas: South-South; South-West; South-East; North-East; North-West and North-Central. During the study period, 36 states plus FCT had reported confirmed COVID-19 cases; all states were actively monitoring for cases through the Integrated Disease Surveillance and Response system (IDSR) system [Reference Fall9].
Data source
SORMAS, an open-source real-time electronic health surveillance database, was the primary data source for this study. In 2017, NCDC adopted SORMAS as its primary digital surveillance platform for implementing the IDSR system [Reference Fall9], and customised it for the surveillance of priority diseases of public health importance in Nigeria. As part of the country's preparedness activities, a COVID-19 module was developed and added to SORMAS in January 2020. All the surveillance data generated through SORMAS is owned by NCDC, processed and stored in a central server at the NCDC headquarters in Abuja, Nigeria.
Study population and data collection
The study population was persons investigated for SARS-CoV-2 infection and captured on SORMAS during the study period. Samples were collected from suspect cases in line with the NCDC case definitions (which were in turn derived from WHO case definitions) in Table 1 [10]. However, these guidelines were not strictly adhered to as samples were also collected from some asymptomatic cases and contacts of cases. Trained healthcare personnel (and rapid response team members) investigated suspected COVID-19 cases, completed a detailed case investigation form (CIF) and collected a minimum of one nasopharyngeal or nasal swab, and one oropharyngeal swab using synthetic fibre swabs with plastic shafts. Collected specimens were triple-packaged and aseptically transported in viral transport media, under appropriate temperature conditions (2–4 °C) to a designated NCDC-certified laboratory in the country, usually based on proximity. Laboratory diagnosis of COVID-19 was done by real-time polymerase chain reaction (RT-PCR) in accordance with the WHO interim guidelines [11]. In addition to clinical samples, information on patients' sociodemographic characteristics, signs and symptoms in the 14 days prior to diagnosis, laboratory findings and clinical outcome as detailed in the national CIF was captured on SORMAS. Surveillance and laboratory data were submitted by trained data collectors (i.e. healthcare personnel) in real time to the NCDC through the SORMAS platform (configured on mobile devices (e.g. tablets and smartphones) and laptops) by each reporting State Epidemiologist and testing laboratory, respectively. All laboratory-confirmed COVID-19 cases were managed according to the NCDC case management protocol [10], while adherence to infection prevention and control measures for both health workers and patient was ensured. Testing for COVID-19 during this study period is free of charge in Nigeria.
Table 1. Definition of key study variables

a Initially, some of the returnees from abroad were tested for COVID-19 even in the absence of symptoms.
b For confirmed asymptomatic cases, period of contact was measured as the 2 days before, through the 14 days after the date on which the sample was taken which led to confirmation; for symptomatic cases, it was presumably 2 days before symptom onset through 14 days after.
c For more information on the criteria for urban/rural classification in Nigeria, see [Reference Ofem12].
d All negative values following the subtraction of date variables were dropped.
Data management and definition of study variables
De-identified data were retrieved from SORMAS. COVID-19 classifications (suspect, probable and confirmed case) were entered by trained data collectors as per the NCDC case definitions [10]. Data management and definitions of key study variables are presented in Table 1. The missing indicator approach was used to address missing data.
Definition of outcome variables
The primary outcome variables for this study were cumulative incidence (CI) and case fatality (CF). CI was defined as the ratio of COVID-19 cases in a defined area to the estimated population of that area. Based on a national average growth rate of 3.2%, CI for each reporting state was calculated using the projected Nigerian population of 2020 from the 2006 national census and was multiplied by 100 000 for ease of interpretation. CF was defined as the proportion of persons diagnosed with COVID-19 who died during the study period, expressed as a percentage (%). Both CI and CF were calculated for Nigeria and for each state separately.
Statistical analyses
Binary/categorical variables were described using frequencies and percentages (%), normally distributed continuous variables by means and standard deviations (s.d.), and non-normally distributed continuous variables by medians and interquartile ranges (IQR). Pearson χ 2 test was used to assess how the sociodemographic and clinical characteristics between COVID-19 cases (confirmed cases vs. non-cases) and clinical outcome (alive vs. dead). A P-value of <0.05 was considered statistically significant. All statistical analyses were carried out in STATA version 13 (Stata Corp. LP, College Station, TX, United States of America). The report of this study was structured in accordance with the STROBE statement.
Ethics
The study protocol was approved by the Nigeria National Health Research Ethics Committee (NHREC/01/01/2007-22/06/2020).
Results
Characteristics of the study population in relation to COVID-19 infection
Between 27 February and 6 June 2020, 60 839 records were entered in the COVID-19 SORMAS database in Nigeria, these were classified as follows: 18 790 suspected cases (30.9%), 73 probable cases (0.1%), 12 289 confirmed cases (20.2%), 28 637 non-cases (47.1%) and 1050 non-classified cases (1.7%). This study focuses on individuals with definitive diagnostic classification (40 926): confirmed cases (n = 12 289) and non-cases (n = 28 637). The daily incidence of cases is shown in the epicurve in Figure 1.
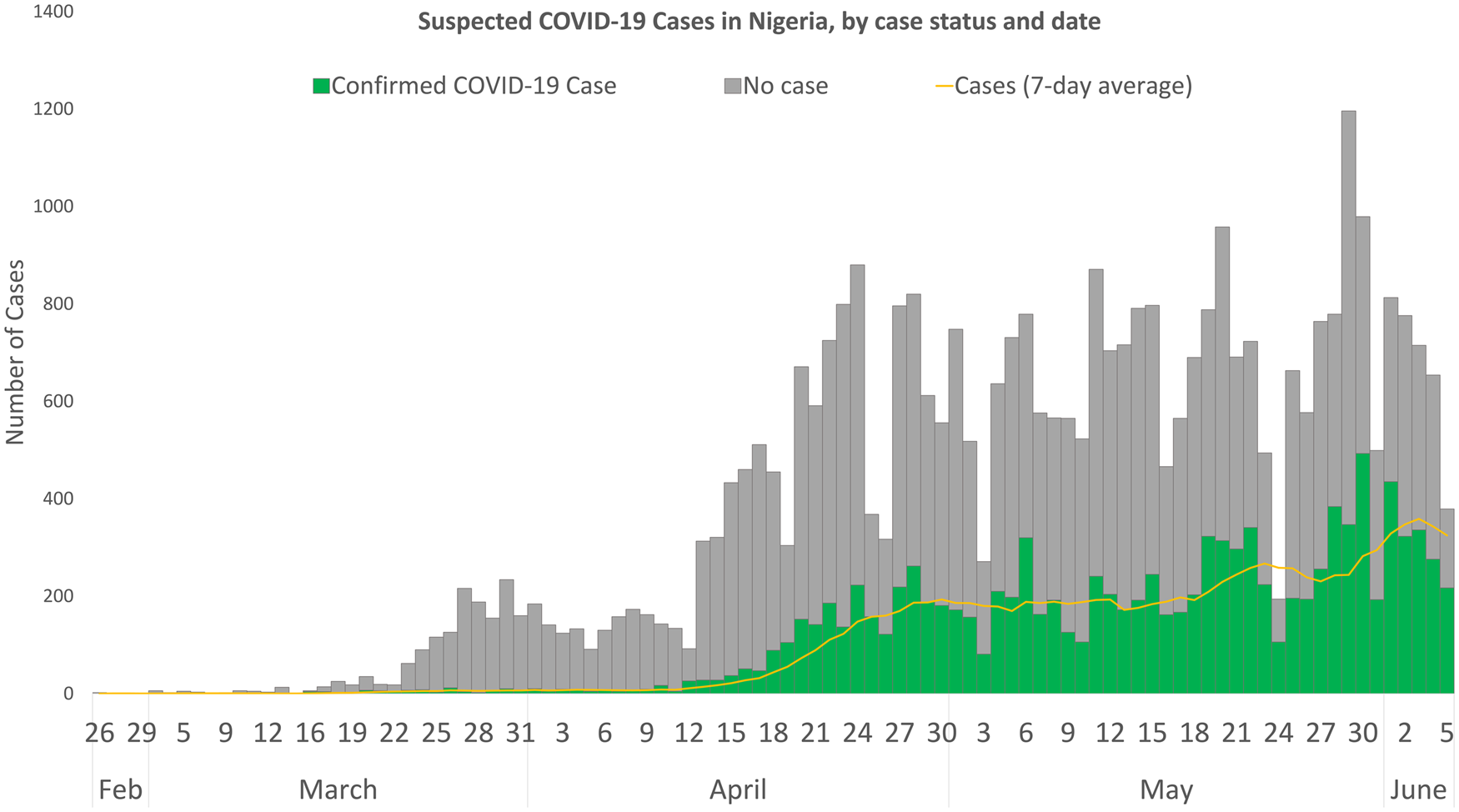
Fig. 1. Suspected COVID-19 cases in Nigeria by case status and date, 26 February to 5 June 2020.
Males (65.8%) constituted a higher proportion of confirmed COVID-19 cases than females (31.6%) (Fig. 2). The mean (s.d.) age of confirmed COVID-19 cases was 37.1 (15.7) years, with the highest proportion of these cases recorded among persons aged 31–40 years (25.5%) and 21–30 years (21.0%) (Table 2). Despite the high proportion of confirmed cases with missing information on education (53.1%), 30.6% reported completing tertiary education, followed by secondary school certificate holders at 8.6%. For confirmed cases with occupation information available, 9.3% were healthcare workers, while pupil/students and traders accounted for 6.7% each. The proportion of confirmed cases who reported history of travel 14 days prior to diagnosis was generally low, with local and international travels at 4.3% and 1.6%, respectively. Sixty-six per cent (8150/12 289) of confirmed COVID-19 cases were asymptomatic in the 14 days prior to diagnosis. Among confirmed COVID-19 cases with symptoms (n = 4139; 33.7%), fever (56.4%) and cough (55.5%) were the most common signs and symptoms reported. Other symptoms commonly reported among confirmed COVID-19 cases were runny nose (23.8%), sore throat (19.8%), difficulty in breathing (18.6%), headache (14.1%), diarrhoea (7.9%), nausea (7.5%), vomiting (5.5%) and fatigue (5.2%); other symptoms were less than 5.0% including loss of smell (4.1%) and loss of taste (3.1%).

Fig. 2. Distribution of non-cases and confirmed cases of COVID-19 by age and sex in Nigeria, 26 February to 6 June 2020.
Table 2. Distribution of sociodemographic and clinical characteristics of the study population in relation to COVID-19 infection status in Nigeria, 27 February–6 June 2020

a Percentages in some instances may be greater than 100.0% due to rounding up.
b Based on 39 145 observations.
c Analyses were restricted to individuals who showed symptoms during the study period: non-cases (9307), confirmed cases (4139) and total cases (13 446).
† P-value <0.05.
‡ P-value <0.001.
Characteristics of the study population in relation to clinical outcome among confirmed COVID-19 cases
Overall, 3467 out of 12 289 confirmed COVID-19 cases had complete records on clinical outcome over the period covered by this study: 3125 surviving vs. 342 dead (Table 3). Mean age of persons who died from COVID-19 was 55.5 (16.4) years. Overall, death from COVID-19 infection increased with increasing age, reaching its highest proportion at 26.6% among persons aged 61–70 years. More deaths were among males (79.0%) than females (19.9%), similar to the gender distribution of COVID-19 cases. Regarding the occupation of those who died, 7.3% of death was recorded among traders, while 40.6% had missing data. Among those who died from COVID-19, 62.0% (212/342) had shown at least one clinical symptom in the 14 days prior to diagnosis (results not shown in Table 3). Of these, cough (72.6%), fever (64.6%) and difficulty in breathing (51.4%) were the most commonly recorded signs and symptoms. Other common symptoms recorded at diagnosis were sore throat (16.5%), runny nose (15.1) and vomiting (12.3%).
Table 3. Distribution of sociodemographic and clinical characteristics of the study population in relation to clinical outcome from COVID-19 infection

ARDS, acute respiratory distress syndrome.
a 8822 persons diagnosed with COVID-19 did not yet have a clinical outcome during the study period.
b Percentages in some instances may be greater than 100.0% due to rounding up.
c Only for symptomatic confirmed COVID-19 cases with records of clinical outcome: survivor (n = 1366), dead (n = 212), and total (n = 1578).
d 692 total records were used for the assessment of temperature.
†P-value <0.05; ‡ P-value <0.001; NS = P-value not statistically significant (i.e. >0.05).
Φ: P-value from t-test was <0.0001; mean difference was 19.9 years.
Description of clinical time variables
Table 4 describes the timelines for clinical variables from available records during this study period. Based on the records of 8370 confirmed COVID-19 cases, the median (IQR) turnaround time for laboratory diagnosis was 2 (1–4) days, whereas it was 1 (1–3) day among 17 817 non-cases. Median (IQR) time from self-reported onset of symptom to sample collection for laboratory diagnosis among 2426 confirmed COVID-19 cases with symptom presentation was 7 (2–17) days. Among 186 deaths from COVID-19 infection, the median (IQR) time from sample collection for laboratory diagnosis to death was 4 (1–8) days.
Table 4. Description of time of available clinical variables among COVID-19 cases

Cumulative incidence of COVID-19 and case fatality in Nigeria, 27 February–6 June 2020
The overall CI of COVID-19 infection and CF in Nigeria during the study period was 5.6 per 100 000 population and 2.8%, respectively (Table 5). Lagos State (39.9 per 100 000), followed by the FCT (19.4 per 100 000), recorded the highest CI in Nigeria during this study period. Other States with CI higher than the national figure include Edo (8.6 per 100 000), Kano (6.8 per 100 000), Ogun (5.9 per 100 000) and Gombe (5.7 per 100 000). Regarding CF across the various Nigerian States, Ondo recorded the highest figure at 16.7%, followed by Yobe State (13.5%), Kebbi State (11.4%) and Bayelsa State (10.0%). The spatial distribution of confirmed COVID-19 cases and death by state is presented in Figure 3.

Fig. 3. (a) Spatial distribution of confirmed COVID-19 cases by Nigerian State, 28 February–6 June 2020. (b) Spatial distribution of confirmed COVID-19 deaths by Nigerian State, 28 February–6 June 2020.
Table 5. CI of COVID-19 and CF rate in Nigeria, 27 February–6 June 2020

a Excluding Cross River State with no official report of COVID-19 during the study period.
b 0.04 when values were not rounded to one decimal place.
c Based on the 2020 projected Nigerian population.
Discussion
Summary of key findings
We have provided a description of the first national epidemiology of COVID-19 cases and associated clinical features and outcomes for Nigeria. There were 12 289 confirmed COVID-19 cases and 28 637 non-cases in 35 states plus the FCT in Nigeria between 27 February and 6 June 2020. During this period, there were 342 deaths, a CI of 5.6 per 100 000 and a CF of 2.8% overall.
Interpretation of key findings
After South Africa, Nigeria is the second most-affected African country in terms of recorded confirmed COVID-19 cases and death as of 7 June 2020 [14]. However, the CI of COVID-19 in Nigeria during the study period, at 5.6 per 100 000 population, is substantially lower than in some non-African countries at a similar stage in their epidemic. For example, about three months after the first confirmed case in the United States, CI was 119⋅6 per 100 000 population, far more than that of Nigeria's; with Minnesota, the State with the lowest CI, having a CI of 20.6 per 100 000 population [15]. Additionally, many European countries reached a CI of at least 4.0 confirmed cases per 100 000 population over a period of less than 1 month [Reference Kinross16]. A possible reason for lower CI in Nigeria could be due to a relatively low testing capacity in the country as compared to the US and European countries.
There was substantial variability in COVID-19 incidence among the states in Nigeria. The heterogeneity in CIs within Nigeria could be attributable, in part, to international travels as indicated by the figures recorded by Lagos State (39.9 per 100 000) and the FCT (19.4 per 100 000) with the two major international airports in the country. Another possible explanation might be due to variations in the estimated population of states in Nigeria, with smaller population recording a higher CI and vice-versa. For example, Ekiti State (3 655 663 population) and Enugu State (4 926 955 population) each recorded 29 confirmed COVID-19 cases during this study period; but the latter recorded a lower CI (0.6 per 100 000 population) than the former (0.8 per 100 000). Moreover, all the Nigerian states did not have a similar testing capacity during the study period, and this might have contributed to the observed findings in terms of the numerator figures for calculating CIs. Similarly, the CF of 2.8% in this study is lower than several other countries which have been hard hit by the COVID-19 pandemic. There is a wide range of CFs among non-African countries (from 0.1% in Singapore to 16.2% in Belgium [17]) and in African countries (from 0.0% in Uganda to 8.2% in Chad) during this study period [14]. Nigeria's observed CF of 2.8% is on the lower end of the range reported outside and within Africa, but higher than the 2.4% (3210 deaths/133 119 confirmed cases) recorded for the entire Africa as of 7 June 2020 [14]. The variation in CF in Nigeria could be an indication of varying health system capacity and preparedness across the country. An unpublished study indicates that Lagos State – with the highest CI but a CF of 1.3% – invested substantially in case management of COVID-19 patients as part of its preparedness activities. The overall CF in Nigeria could be partly due to its much younger population compared to the United States and most countries in Europe [Reference Dowd18]; similar trends in deaths by age from COVID-19 have been reported in China [Reference Liu19]. Just as cases are potentially underestimated due to inadequate testing, it is likely that deaths from COVID-19 are also underestimated, especially in places like Kano, which reported significant increases in deaths in April [20].
In contrast to deaths from COVID-19, a higher proportion of COVID-19 cases was recorded among economically active age groups, suggesting potential role of socio-economic or work-related activities rather than immunological capacity. Children under 5 years of age and those aged 5–13 years, respectively, accounted for 1.7% and 3.9% of confirmed COVID-19 cases in this study. These findings are comparable to those from a recent global systematic review [Reference Ludvigsson21]. Although it remains unclear why children are less affected by COVID-19 than older individuals, evidence suggests differences in immune system function [Reference Balasubramanian22]. The higher infection rate among males in this study corresponds to evidence reported in the WHO African region, where males in the 31–39 and 40–49 age groups accounted for 62% of 5178 recorded cases [14]. Outside Africa, early findings of the clinical characteristics of 41 confirmed COVID-19 cases in Wuhan, China, reported males to have accounted for 30 (73.0%) of the cases [Reference Huang23]. A study in Italy also reported male preponderance [Reference Grasselli24]. A combination of genetic and physiological factors has been hypothesised as possible explanations for the potential male bias. For example, the wider distribution of SARS-CoV-2 cellular receptor, angiotensin-converting enzyme 2 (ACE-2), in male over females has been postulated [Reference Patel25]. In a patriarchal system such as seen in Nigeria, men are more likely to engage in economic activities outside of the household and potentially become more exposed to SARS-CoV-2 infection than women. While this may be more feasible during a controlled economy, such as that seen during the suspension of non-essential economic activities in the early phase of COVID-19 outbreak in Nigeria, it may not be applicable when socio-economic activities are functional. This is because women are increasingly partaking in the workforce in Nigeria, such that the traditional trends of ‘male breadwinner and female family support’ are fast eroding [Reference Akanle26].
The median length of stay of 111 patients with COVID-19 in hospital in this study was 19 days, which is within the range outside of China (4–21 days), but comparatively lower than that from China (4–53 days) [Reference Rees27]. In general, differences in the length of hospital stay may be attributable to variations in criteria for admission and discharge across different countries as well as timing within the pandemic [Reference Rees27]. Early diagnosis is fundamental for effective management of COVID-19 cases; thus, a median turnaround time of 2 (1–4) days for laboratory diagnosis as noted in the current study seems impressive, and possibly an indication of ongoing measures being championed by the NCDC to strengthen molecular diagnostic capacity in Nigeria. However, we lacked information on when laboratory test was received by a COVID-19 suspected case, as turnaround time only included the time from sample collection to availability of result.
The symptomatic status of confirmed COVID-19 cases in this analysis is noteworthy, as over half of them were asymptomatic at testing. A scoping review of the literature found that between 5% and 80% of people testing positive for SARS-CoV-2 may be asymptomatic [Reference Heneghan28], placing the 66% in the current study closer to the maximum range. It is possible that the case investigation approach adopted during testing might have underestimated symptoms: patients were initially asked whether they were symptomatic and probed about individual symptoms only if they answered in the affirmative. Stigma associated with COVID-19 in Nigeria might contribute to people not reporting symptoms when they get tested [29]. Furthermore, it is possible for asymptomatic status at diagnosis to change in the course of an illness, in which case such persons could be better classified as pre-symptomatic cases, so the proportion of truly asymptomatic cases cannot be described by these data. Nevertheless, this scenario could pose a challenge to community surveillance activities and implementation of public health interventions (e.g. quarantine and isolation). Thus, the possibility of COVID-19 transmission by asymptomatic cases in Nigeria needs to be explored and addressed, both in terms of research and community risk communication activities.
The most common signs and symptoms among symptomatic confirmed COVID-19 cases in the 14 days prior to diagnosis were fever (56.4%) and cough (55.5%). This trend is similar to that recorded in a recent systematic review of the literature for China [Reference Fu30]; however, while fatigue was the third most frequently recorded symptom in China, its frequency was low in our study at 5.2%. Similarly, cough, fever and difficulty in breathing, in that order, were the most commonly recorded symptoms at diagnosis among persons who died from COVID-19 infection. The common occurrence of difficulty in breathing in deceased patients has been identified as a major driver of adverse clinical outcomes among COVID-19 patients [Reference Guan31]. Although relatively small in proportion due to late recording during the study period, loss of smell and loss of taste among confirmed COVID-19 cases in this study are consistent with available evidence [Reference Printza and Constantinidis32]. However, being a descriptive study, these data do not have the capacity to establish a causal association between observed clinical symptoms and COVID-19 infection or death. Thus, a follow-up study aimed at exploring these associations is recommended. It is also worth noting that the fever which is one of the common symptoms noted in this study is often common in endemic febrile illnesses in Nigeria including malaria, Lassa fever and yellow fever. As such, in the case of a co-infection, misclassification of illnesses is likely if symptoms alone are used for COVID-19 case definitions [Reference Chanda-Kapata33]. The symptomatic and geographic convergence of COVID-19 and common febrile diseases in Nigeria therefore requires continuous strengthening of definitive diagnostic approaches in the country. About 9% of COVID-19 infections occurred in healthcare workers during this study period. COVID-19 infection among health workers is of prominent public health importance as it could potentially enhance disease transmission [Reference Barranco and Ventura34] and further weaken a health system that already struggles with insufficient human resources for health.
Strengths and limitations
This study has provided the first national epidemiological evidence on COVID-19 in Nigeria, necessary for public health planning and health system strengthening. However, this study is limited by the substantial proportion of missing data within some of the sociodemographic (e.g. residential setting and health facility) and clinical (e.g. malaise, pharyngeal exudate, rapid breathing, loss of smell and taste) variables studied. The late addition of loss of smell and taste to the CIF in Nigeria may partly explain why data recorders were not accustomed to capturing them. The high proportion of missing data on some key indicators has prompted a systemic effort to improve the quality of SORMAS data, and a dedicated Data Quality Improvement Project (DQIP) was initiated in April to improve completeness of key variables to above 90%.
In conclusion, this study has provided an early insight into the epidemiology of COVID-19 in Nigeria. Evidence from this study, such as the high proportion of cases among the active age group and high proportion of asymptomatic cases at diagnosis, will be useful for policymakers and stakeholders in the health and other sectors in contextualising public health planning and response as well as for scientific activities in the country. Such measures could include intensifying NPIs at work and commercial places where this age group is mostly found, and adapting case finding protocols to include routine testing of asymptomatic contacts of confirmed cases.
Acknowledgement
We wish to thank all the Nigerian frontline health personnel for the contribution to the collection of the data used for this study. The leadership and coordination of all the State Commissioners for Health, Epidemiologists and Disease Notification and Surveillance Officers are very much appreciated. Last but not least, we are grateful to colleagues who provided technical and administrative support for this manuscript.
Financial support
This research received no specific grant from any funding agency, commercial or not-for-profit sectors.
Conflict of interest
None.
Data availability statement
Readers can contact the corresponding author at chinwe.ochu@ncdc.gov.ng for access to the data used for this study.