Part III - Making Structural Predictions
Published online by Cambridge University Press: 21 September 2023
Summary
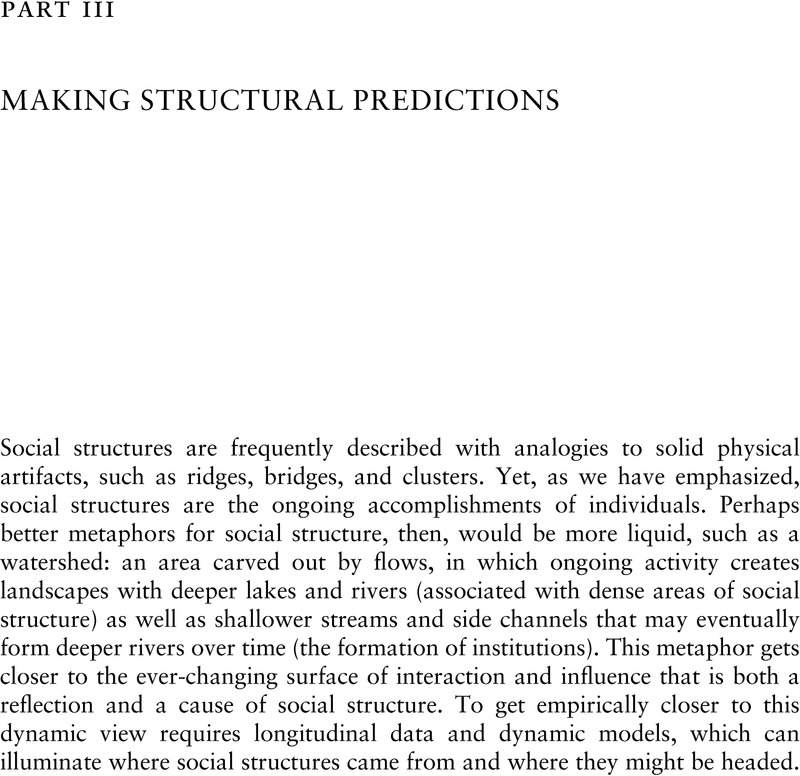
- Type
- Chapter
- Information
- Network AnalysisIntegrating Social Network Theory, Method, and Application with R, pp. 299 - 420Publisher: Cambridge University PressPrint publication year: 2023
References
Suggested Further Reading
The literature on statistical models for networks is vast and has grown dramatically in the last fifteen years. Here, we list a handful of key contemporary references and classic foundational pieces, which should help those interested to launch a deeper exploration.