Book contents
- Grasslands and Climate Change
- Ecological Reviews
- Grasslands and Climate Change
- Copyright page
- Contents
- Contributors
- Preface
- Introduction
- CHAPTER ONE Grasslands and climate change: an overview
- CHAPTER TWO Methodology I: Detecting and predicting grassland change
- CHAPTER THREE Methodology II: Remote sensing of change in grasslands
- Part I Grassland dynamics and climate change
- Part II Species traits, functional groups, and evolutionary change
- Part III Dealing with climate change effects
- Index
- Plate Section (PDF Only)
- References
CHAPTER THREE - Methodology II: Remote sensing of change in grasslands
Published online by Cambridge University Press: 22 March 2019
- Grasslands and Climate Change
- Ecological Reviews
- Grasslands and Climate Change
- Copyright page
- Contents
- Contributors
- Preface
- Introduction
- CHAPTER ONE Grasslands and climate change: an overview
- CHAPTER TWO Methodology I: Detecting and predicting grassland change
- CHAPTER THREE Methodology II: Remote sensing of change in grasslands
- Part I Grassland dynamics and climate change
- Part II Species traits, functional groups, and evolutionary change
- Part III Dealing with climate change effects
- Index
- Plate Section (PDF Only)
- References
Summary
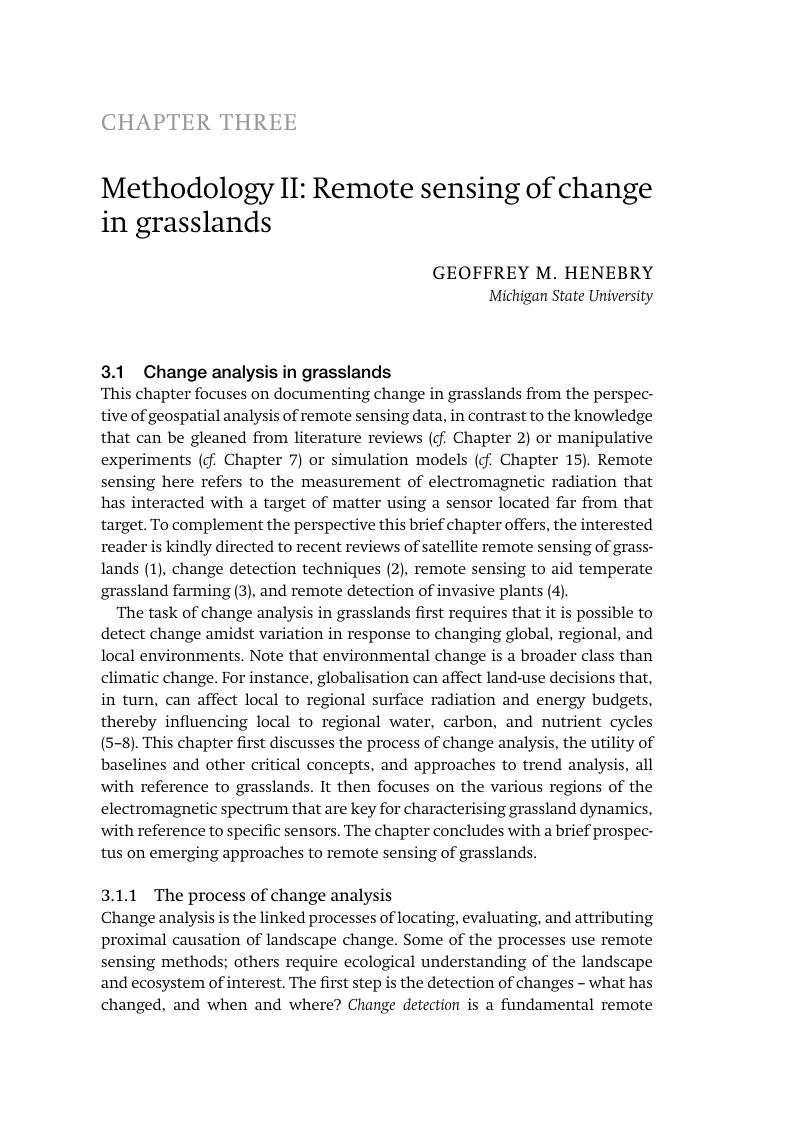
- Type
- Chapter
- Information
- Grasslands and Climate Change , pp. 40 - 64Publisher: Cambridge University PressPrint publication year: 2019
References
3.5 References
- 2
- Cited by