Introduction
Smart farming (Tyagi, Reference Tyagi2016) is important for tackling various challenges of agricultural production such as productivity, environmental impact, food security and sustainability (Gebbers and Adamchuk, Reference Gebbers and Adamchuk2010). As the global population is growing continuously (Kitzes et al., Reference Kitzes, Wackernagel, Loh, Peller, Goldfinger, Cheng and Tea2008), a large increase of food production must be achieved (FAO, 2009). This must be accompanied with the protection of natural ecosystems by means of using sustainable farming procedures. Food needs to maintain a high nutritional value while its security must be ensured around the world (Carvalho, Reference Carvalho2006).
To address these challenges, complex, multivariate and unpredictable agricultural ecosystems need to be better understood. This would be achieved by monitoring, measuring and analysing various physical aspects and phenomena continuously. The deployment of new information and communication technologies (ICT) for small-scale crop/farm management and larger scale ecosystem observation will facilitate this task, enhancing management and decision-/policy-making by context, situation and location awareness.
Emerging ICT technologies relevant for understanding agricultural ecosystems include remote sensing (Bastiaanssen et al., Reference Bastiaanssen, Molden and Makin2000), the Internet of Things (IoT) (Weber and Weber, Reference Weber and Weber2010), cloud computing (Hashem et al., Reference Hashem, Yaqoob, Anuar, Mokhtar, Gani and Khan2015) and big data analysis (Chi et al., Reference Chi, Plaza, Benediktsson, Sun, Shen and Zhu2016; Kamilaris et al., Reference Kamilaris, Kartakoullis and Prenafeta-Boldú2017). Remote sensing, by means of satellites, planes and unmanned aerial vehicles (UAV, i.e. drones) provides large-scale snapshots of the agricultural environment. It has several advantages when applied to agriculture, being a well-known, non-destructive method to collect information about earth features. Remote-sensing data may be obtained systematically over very large geographical areas, including zones inaccessible to human exploration. The IoT uses advanced sensor technology to measure various parameters in the field, while cloud computing is used for collection, storage, pre-processing and modelling of huge amounts of data coming from various, heterogeneous sources. Finally, big data analysis is used in combination with cloud computing for real-time, large-scale analysis of data stored in the cloud (Waga and Rabah, Reference Waga and Rabah2014; Kamilaris et al., Reference Kamilaris, Gao, Prenafeta-Boldú and Ali2016).
These four technologies (remote sensing, IoT, cloud computing and big data analysis) could create novel applications and services that could improve agricultural productivity and increase food security, for instance by better understanding climatic conditions and changes.
A large sub-set of the volume of data collected through remote sensing and the IoT involves images. Images can provide a complete picture of the agricultural fields, and image analysis could address a variety of challenges (Liaghat and Balasundram, Reference Liaghat and Balasundram2010; Ozdogan et al., Reference Ozdogan, Yang, Allez and Cervantes2010). Hence, image analysis is an important research area in the agricultural domain, and intelligent analysis techniques are used for image identification/classification, anomaly detection, etc., in various agricultural applications (Teke et al., Reference Teke, Deveci, Haliloğlu, Gürbüz, Sakarya, Ilarslan, Ince, Kaynak and Basturk2013; Saxena and Armstrong, Reference Saxena and Armstrong2014).
Of these, the most common sensing method is satellite-based, using multi-spectral and hyperspectral imaging. Synthetic aperture radar, thermal and near infrared cameras have been used to a lesser extent (Ishimwe et al., Reference Ishimwe, Abutaleb and Ahmed2014), while optical and X-ray imaging have been applied in fruit and packaged food grading (Saxena and Armstrong, Reference Saxena and Armstrong2014). The most common techniques used for image analysis include machine learning (K-means, support vector machines (SVM) and artificial neural networks (ANN), amongst others), wavelet-based filtering, vegetation indices such as the normalized difference vegetation index (NDVI) and regression analysis (Saxena and Armstrong, Reference Saxena and Armstrong2014).
Besides the aforementioned techniques, deep learning (DL; LeCun et al., Reference LeCun, Bengio and Hinton2015) is a modern approach with much potential and success in various domains where it has been employed (Wan et al., Reference Wan, Wang, Hoi, Wu, Zhu, Zhang and Li2014; Najafabadi et al., Reference Najafabadi, Villanustre, Khoshgoftaar, Seliya, Wald and Muharemagic2015). It belongs to the research area of machine learning and it is similar to ANN (Schmidhuber, Reference Schmidhuber2015). However, DL constitutes a ‘deeper’ neural network that provides a hierarchical representation of the data by means of various convolutions. This allows better learning capabilities in terms of capturing the full complexity of the real-life task under study, and thus the trained model can achieve higher classification accuracy.
The current survey examines the problems that employ a particular class of DL named convolutional neural networks (CNN), defined as deep, feed-forward ANN. Convolutional neural networks extend classical ANN by adding more ‘depth’ into the network, as well as various convolutions that allow data representation in a hierarchical way (LeCun and Bengio, Reference LeCun, Bengio and Arbib1995; Schmidhuber, Reference Schmidhuber2015) and they have been applied successfully in various visual imagery-related problems (Szegedy et al., Reference Szegedy, Liu, Jia, Sermanet, Reed, Anguelov, Erhan, Vanhoucke and Rabinovich2015).
The motivation for preparing this survey stems from the fact that CNN have been employed recently in agriculture, with growing popularity and success, and the fact that today more than 20 research efforts employing CNN exist for addressing various agricultural problems. As CNN constitute probably the most popular and widely used technique in agricultural research today, in problems related to image analysis, the current survey focuses on this specific sub-set of DL models and techniques. To the authors’ knowledge, this is the first survey in the agricultural domain that focuses on this practice, although a small number of more general surveys do exist (Deng and Yu, Reference Deng and Yu2014; Wan et al., Reference Wan, Wang, Hoi, Wu, Zhu, Zhang and Li2014; Najafabadi et al., Reference Najafabadi, Villanustre, Khoshgoftaar, Seliya, Wald and Muharemagic2015), presenting and analysing related work in other research domains and application areas. For a more complete review on DL approaches in agriculture, please refer to Kamilaris and Prenafeta-Boldú (Reference Kamilaris and Prenafeta-Boldú2018).
The aim of the current research was to introduce the technique of CNN, as a promising and high-potential approach for addressing various challenges in agriculture related to computer vision. Besides analysing the state of the art work at the field, a practical example of CNN applied in identifying missing vegetation based on aerial images is presented in order to further illustrate the benefits and shortcomings of this technique.
Methodology
The bibliographic analysis involved two steps: (a) collection of related work; and (b) detailed review and analysis of these collected works.
In the first step, a keyword-based search for conference papers and articles was performed between August and September 2017. Sources were the scientific databases IEEE Xplore and ScienceDirect, as well as the web scientific indexing services Web of Science and Google Scholar. The following keywords were used in the search query:
[‘deep learning’ | ‘convolutional neural networks’] AND [‘agriculture’ OR ‘farming’].
In this way, papers referring to CNN but not applied to the agricultural domain were filtered out. From this effort, 27 papers were identified initially. Restricting the search for papers to appropriate application of the CNN technique and meaningful findings, the initial number of papers was reduced to 23. The following criteria were used to define appropriate application of CNN:
1. Use of CNN or CNN-based approach as the technique for addressing the problem under study.
2. Target some problem or challenge related to agriculture.
3. Show practical results by means of some well-defined performance metrics that indicate the success of the technique used.
Some performance metrics, as identified in related work under study, are the following:
• Root mean square error (RMSE): Standard deviation of the differences between predicted values and observed values.
• F1 Score: The harmonic mean of precision and recall. For multi-class classification problems, F1 is averaged among all the classes.
• Quality measure (QM): Obtained by multiplying sensitivity (proportion of pixels that were detected correctly) and specificity (which proportion of detected pixels are truly correct; Douarre et al., Reference Douarre, Schielein, Frindel, Gerth and Rousseau2016).
• Ratio of total fruits counted (RFC): Ratio of a predicted count of fruits by a CNN model, v. the actual count performed offline by the authors or by experts (Chen et al., Reference Chen, Shivakumar, Dcunha, Das, Okon, Qu, Taylor and Kumar2017; Rahnemoonfar and Sheppard, Reference Rahnemoonfar and Sheppard2017).
• LifeCLEF metric (LC): A score related to the rank of the correct species in the list of retrieved species during the LifeCLEF 2015 Challenge (Reyes et al., Reference Reyes, Caicedo, Camargo, Cappellato, Ferro, Jones and San Juan2015).
In the second step, the 23 papers selected from the first step were analysed one by one, considering the following research questions: (a) the problem they addressed, (b) approach employed, (c) sources of data used and (d) the overall precision. Also recorded were: (e) whether the authors had compared their CNN-based approach with other techniques, and (f) what was the difference in performance. Examining how CNN performs in relation to other existing techniques was a critical aspect of the current study, as it would be the main indication of CNN effectiveness and performance. It should be noted that it is difficult if not impossible to compare between different metrics for different tasks. Thus, the current paper focuses only on comparisons between techniques used for the same data in the same research paper, using the same metric.
Convolutional neural networks
In machine learning, CNN constitutes a class of deep, feed-forward ANN that has been applied successfully to computer vision applications (LeCun and Bengio, Reference LeCun, Bengio and Arbib1995; Schmidhuber, Reference Schmidhuber2015).
In contrast to ANN, whose training requirements in terms of time are impractical in some large-scale problems, CNN can learn complex problems particularly fast because of weight sharing and more complex models used, which allow massive parallelization (Pan and Yang, Reference Pan and Yang2010). Convolutional neural networks can increase their probability of correct classifications, provided there are adequately large data sets (i.e. hundreds up to thousands of measurements, depending on the complexity of the problem under study) available for describing the problem. They consist of various convolutional, pooling and/or fully connected layers (Canziani et al., Reference Canziani, Paszke and Culurciello2016). The convolutional layers act as feature extractors from the input images whose dimensionality is then reduced by the pooling layers, while the fully connected layers act as classifiers. Usually, at the last layer, the fully connected layers exploit the high-level features learned, in order to classify input images into predefined classes (Schmidhuber, Reference Schmidhuber2015).
The highly hierarchical structure and large learning capacity of CNN models allow them to perform classification and predictions particularly well, being flexible and adaptable in a wide variety of complex challenges (Oquab et al., Reference Oquab, Bottou, Laptev and Sivic2014).
Convolutional neural networks can receive any form of data as input, such as audio, video, images, speech and natural language (Abdel-Hamid et al., Reference Abdel-Hamid, Mohamed, Jiang, Deng, Penn and Yu2014; Karpathy et al., Reference Karpathy, Toderici, Shetty, Leung, Sukthankar and Fei-Fei2014; Kim, Reference Kim2014; Kamilaris and Prenafeta-Boldú, Reference Kamilaris and Prenafeta-Boldú2017), and have been applied successfully by numerous organizations in various domains, such as the web (i.e. personalization systems, online chat robots), health (i.e. identification of diseases from MRI scans), disaster management (i.e. identifications of disasters by remote-sensing images), post services (i.e. automatic reading of addresses), car industry (i.e. autonomous self-driving cars), etc.
An example of CNN architecture (Simonyan and Zisserman, Reference Simonyan and Zisserman2014) is displayed in Fig. 1. As the figure shows, various convolutions are applied at some layers of the network, creating different representations of the learning data set, starting from more general ones at the first, larger layers and becoming more specific at the deeper layers. A combination of convolutional layers and dense layers tends to present good precision results.

Fig. 1. An example of CNN architecture (VGG; Simonyan and Zisserman, Reference Simonyan and Zisserman2014). Colour online.
There exist various ‘successful’ popular architectures which researchers may use to start building their models instead of starting from scratch. These include AlexNet (Krizhevsky et al., Reference Krizhevsky, Sutskever, Hinton, Pereira, Burges, Bottou and Weinberger2012), the Visual Geometry Group (VGG; Simonyan and Zisserman, Reference Simonyan and Zisserman2014) (displayed in Fig. 1), GoogleNet (Szegedy et al., Reference Szegedy, Liu, Jia, Sermanet, Reed, Anguelov, Erhan, Vanhoucke and Rabinovich2015) and Inception-ResNet (Szegedy et al., Reference Szegedy, Ioffe, Vanhoucke and Alemi2017). Each architecture has different advantages and scenarios where it is used more appropriately (Canziani et al., Reference Canziani, Paszke and Culurciello2016). It is also worth noting that almost all the aforementioned architectures come with their weights pre-trained, i.e. their network has already been trained by some data set and has thus learned to provide accurate recognition for some particular problem domain (Pan and Yang, Reference Pan and Yang2010). Common data sets used for pre-training DL architectures include ImageNet (Deng et al., Reference Deng, Dong, Socher, Li, Li and Fei-Fei2009) and PASCAL VOC (http://host.robots.ox.ac.uk/pascal/VOC/).
Moreover, there are various tools and platforms that allow researchers to experiment with DL (Bahrampour et al., Reference Bahrampour, Ramakrishnan, Schott and Shah2015). The most popular ones are Theano, TensorFlow, Keras (which is an Application Programming Interface (API) on top of Theano and TensorFlow), Caffe, PyTorch, TFLearn, Pylearn2 and the Deep Learning Matlab Toolbox. Some of these tools (i.e. Theano, Caffe) incorporate popular architectures such as the ones mentioned above (i.e. AlexNet, VGG, GoogleNet), either as libraries or classes.
Convolutional neural network applications in agriculture
Appendix I lists the relevant works identified, indicating the particular problem they addressed, the agricultural area involved, sources of data used, overall precision achieved and details of the CNN-based implementation as well as comparisons with other techniques, wherever available.
Areas of use
Twelve areas have been identified in total, with the most popular being plant- and leaf-based disease detection (three papers), land cover classification (three papers), plant recognition (three papers), fruit counting (four papers) and weed identification (three papers).
It is remarkable that all papers have been published after 2014, indicating how recent and modern this technique is in the domain of agriculture. More precisely, six of the papers were published in 2017, ten in 2016, six in 2015 and one in 2014.
The majority of these papers dealt with image classification and identification of areas of interest, including detection of obstacles (Christiansen et al., Reference Christiansen, Nielsen, Steen, Jørgensen and Karstoft2016; Steen et al., Reference Steen, Christiansen, Karstoft and Jørgensen2016) and fruit counting (Sa et al., Reference Sa, Ge, Dayoub, Upcroft, Perez and McCool2016; Rahnemoonfar and Sheppard, Reference Rahnemoonfar and Sheppard2017), while some other papers focused on predicting future values such as maize yield (Kuwata and Shibasaki, Reference Kuwata and Shibasaki2015) and soil moisture content in the field (Song et al., Reference Song, Zhang, Liu, Li, Zhao and Yang2016).
From another perspective, most papers (19) targeted crops, while few considered the issues of land cover (three papers) and livestock agriculture (one paper).
Data sources
Observing the sources of data used to train the CNN model for each paper, they mainly used large data sets of images, containing in some cases thousands of images (Reyes et al., Reference Reyes, Caicedo, Camargo, Cappellato, Ferro, Jones and San Juan2015; Mohanty et al., Reference Mohanty, Hughes and Salathé2016). Some of these images and data sets originated from well-known and publicly available resources such as PlantVillage, LifeCLEF, MalayaKew and UC Merced, while others were produced by the authors for their research needs (Xinshao and Cheng, Reference Xinshao and Cheng2015; Sladojevic et al., Reference Sladojevic, Arsenovic, Anderla, Culibrk and Stefanovic2016; Bargoti and Underwood, Reference Bargoti, Underwood and Okamura2017; Rahnemoonfar and Sheppard, Reference Rahnemoonfar and Sheppard2017; Sørensen et al., Reference Sørensen, Rasmussen, Nielsen and Jørgensen2017). Papers dealing with land cover and crop type classification employed a smaller number of images (e.g. 10–100 images), produced by UAV (Lu et al., Reference Lu, Fu, Liu, Li, He and Li2017) or satellite-based remote sensing (Kussul et al., Reference Kussul, Lavreniuk, Skakun and Shelestov2017). One particular paper investigating segmentation of root and soil used images from X-ray tomography (Douarre et al., Reference Douarre, Schielein, Frindel, Gerth and Rousseau2016). Moreover, some projects used historical text data, collected either from repositories (Kuwata and Shibasaki, Reference Kuwata and Shibasaki2015) or field sensors (Song et al., Reference Song, Zhang, Liu, Li, Zhao and Yang2016). In general, the more complicated the problem to be solved (e.g. large number of classes to identify), the more data are required.
Performance metrics and overall precision
Various performance metrics have been employed by the authors, with percentage of correct predictions (CA) on the validation or testing data set being the most popular (16 papers, 69%). Others included RMSE (three papers), F1 Score (three papers), QM (Douarre et al., Reference Douarre, Schielein, Frindel, Gerth and Rousseau2016), RFC (Chen et al., Reference Chen, Shivakumar, Dcunha, Das, Okon, Qu, Taylor and Kumar2017) and LC (Reyes et al., Reference Reyes, Caicedo, Camargo, Cappellato, Ferro, Jones and San Juan2015). The aforementioned metrics have been defined earlier.
The majority of related work employed CA, which is generally high (i.e. above 90%), indicating the successful application of CNN in various agricultural problems. The highest CA statistics were observed in the works of Chen et al. (Reference Chen, Lin, Zhao, Wang and Gu2014), Lee et al. (Reference Lee, Chan, Wilkin and Remagnino2015) and Steen et al. (Reference Steen, Christiansen, Karstoft and Jørgensen2016), with accuracies of 98% or more.
Technical details
From a technical point of view, almost half of the research works (12 papers) employed popular CNN architectures such as AlexNet, VGG and Inception-ResNet. The other half (11 papers) experimented with their own architectures, some combining CNN with other techniques, such as principal component analysis (PCA) and logistic regression (Chen et al., Reference Chen, Lin, Zhao, Wang and Gu2014), SVM (Douarre et al., Reference Douarre, Schielein, Frindel, Gerth and Rousseau2016), linear regression (Chen et al., Reference Chen, Shivakumar, Dcunha, Das, Okon, Qu, Taylor and Kumar2017), large margin classifiers (LMC, Xinshao and Cheng, Reference Xinshao and Cheng2015) and macroscopic cellular automata (Song et al., Reference Song, Zhang, Liu, Li, Zhao and Yang2016).
Regarding the frameworks used, all the studies that employed some well-known architecture had also used a DL framework, with Caffe being the most popular (ten papers, 43%), followed by deeplearning4j (one paper) and Tensor Flow (one paper). Five research works developed their own software, while some authors built their own models on top of Theano (three papers), Pylearn2 (one paper), MatConvNet (one paper) and Deep Learning Matlab Toolbox (one paper). A possible reason for the wide use of Caffe is that it incorporates various CNN frameworks and data sets which can then be used easily.
It is worth mentioning that some of the related works that possessed only small data sets to train their CNN models (Sladojevic et al., Reference Sladojevic, Arsenovic, Anderla, Culibrk and Stefanovic2016; Bargoti and Underwood, Reference Bargoti, Underwood and Okamura2017; Sørensen et al., Reference Sørensen, Rasmussen, Nielsen and Jørgensen2017) exploited data augmentation techniques (Krizhevsky et al., Reference Krizhevsky, Sutskever, Hinton, Pereira, Burges, Bottou and Weinberger2012) to enlarge the number of training images artificially using label-preserving transformations, such as translations, transposing, reflections and altering the intensities of the RGB channels.
Furthermore, the majority of related work included some image pre-processing steps, where each image in the data set was reduced to a smaller size, before being used as input to the model, such as 256 × 256, 128 × 128, 96 × 96, 60 × 60 pixels, or converted to greyscale (Santoni et al., Reference Santoni, Sensuse, Arymurthy and Fanany2015). Most of the studies divided their data randomly between training and testing/verification sets, using a ratio of 80 : 20 or 90 : 10, respectively. Also, various learning rates have been recorded, from 0.001 (Amara et al., Reference Amara, Bouaziz, Algergawy and Mitschang2017) and 0.005 (Mohanty et al., Reference Mohanty, Hughes and Salathé2016) up to 0.01 (Grinblat et al., Reference Grinblat, Uzal, Larese and Granitto2016). Learning rate is about how quickly a network learns. Higher values help to avoid being stuck in local minima. A general approach used by many of the evaluated papers was to start out with a high learning rate and lower it as the training goes on. The learning rate is very dependent on the network architecture.
Finally, most of the research works that incorporated popular CNN architectures took advantage of transfer learning (Pan and Yang, Reference Pan and Yang2010), which leverages the already existing knowledge of some related task in order to increase the learning efficiency of the problem under study, by fine-tuning pre-trained models. When it is not possible to train a network from scratch due to having a small training data set or addressing a complex problem, it is useful for the network to be initialized with weights from another pre-trained model. Pre-trained CNN are models that have already been trained on some relevant data set with possibly different numbers of classes. These models are then adapted to the particular challenge and data set being studied. This method was followed in Lee et al. (Reference Lee, Chan, Wilkin and Remagnino2015), Reyes et al. (Reference Reyes, Caicedo, Camargo, Cappellato, Ferro, Jones and San Juan2015), Bargoti and Underwood (Reference Bargoti, Underwood and Okamura2017), Christiansen et al. (Reference Christiansen, Nielsen, Steen, Jørgensen and Karstoft2016), Douarre et al. (Reference Douarre, Schielein, Frindel, Gerth and Rousseau2016), Mohanty et al. (Reference Mohanty, Hughes and Salathé2016), Sa et al. (Reference Sa, Ge, Dayoub, Upcroft, Perez and McCool2016), Steen et al. (Reference Steen, Christiansen, Karstoft and Jørgensen2016), Lu et al. (Reference Lu, Fu, Liu, Li, He and Li2017) and Sørensen et al. (Reference Sørensen, Rasmussen, Nielsen and Jørgensen2017) for the VGG16, DenseNet, AlexNet and GoogleNet architectures.
Performance comparison with other approaches
The eighth column of Table 1 shows whether the authors of related work compared their CNN-based approach with other techniques used for solving their problem under study. The percentage of CA for CNN was 1–4% better than SVM (Chen et al., Reference Chen, Lin, Zhao, Wang and Gu2014; Lee et al., Reference Lee, Chan, Wilkin and Remagnino2015; Grinblat et al., Reference Grinblat, Uzal, Larese and Granitto2016), 3–11% better than unsupervised feature learning (Luus et al., Reference Luus, Salmon, van den Bergh and Maharaj2015) and 2–44% better than local shape and colour features (Dyrmann et al., Reference Dyrmann, Karstoft and Midtiby2016; Sørensen et al., Reference Sørensen, Rasmussen, Nielsen and Jørgensen2017). Compared with multilayer perceptrons, CNN showed 2% better CA (Kussul et al., Reference Kussul, Lavreniuk, Skakun and Shelestov2017) and 18% lower RMSE (Song et al., Reference Song, Zhang, Liu, Li, Zhao and Yang2016).
Table 1. Applications of deep learning in agriculture

Moreover, CNN achieved 6% higher CA than random forests (Kussul et al., Reference Kussul, Lavreniuk, Skakun and Shelestov2017), 2% better CA than Penalized Discriminant Analysis (Grinblat et al., Reference Grinblat, Uzal, Larese and Granitto2016), 41% improved CA when compared with ANN (Lee et al., Reference Lee, Chan, Wilkin and Remagnino2015) and 24% lower RMSE compared with Support Vector Regression (Kuwata and Shibasaki, Reference Kuwata and Shibasaki2015).
Furthermore, CNN reached 25% better RFC than an area-based technique (Rahnemoonfar and Sheppard, Reference Rahnemoonfar and Sheppard2017), 30% higher RFC than the best texture-based regression model (Chen et al., Reference Chen, Shivakumar, Dcunha, Das, Okon, Qu, Taylor and Kumar2017), 84.3% better F1 Score in relation to an algorithm based on local decorrelated channel features and 3% higher CA compared with a Gaussian Mixture Model (GMM; Santoni et al., Reference Santoni, Sensuse, Arymurthy and Fanany2015).
Convolutional neural networks showed worse performance than other techniques in only one case (Reyes et al., Reference Reyes, Caicedo, Camargo, Cappellato, Ferro, Jones and San Juan2015). This was against a technique involving local descriptors to represent images together with k-nearest neighbours (KNN) as classification strategy (20% lower LC).
Discussion
The current analysis has shown that CNN offer superior performance in terms of precision in the vast majority of related work, based on the performance metrics employed by the authors, with GMM being a technique with comparable performance in some cases (Reyes et al., Reference Reyes, Caicedo, Camargo, Cappellato, Ferro, Jones and San Juan2015; Santoni et al., Reference Santoni, Sensuse, Arymurthy and Fanany2015). Although the current study is relatively small, in most of the agricultural challenges used satisfactory precision has been observed, especially in comparison with other techniques employed to solve the same problem. This indicates a successful application of CNN in various agricultural domains. In particular, the areas of plant and leaf disease detection, plant recognition, land cover classification, fruit counting and identification of weeds belong to the categories where the highest precision has been observed.
Although CNN have been associated with computer vision and image analysis (which is also the general case in this survey), two related works were found where CNN-based models have been trained based on field sensory data (Kuwata and Shibasaki, Reference Kuwata and Shibasaki2015) and a combination of static and dynamic environmental variables (Song et al., Reference Song, Zhang, Liu, Li, Zhao and Yang2016). In both cases, the performance (i.e. RMSE) was better than other techniques under study.
When comparing performance in terms of precision/accuracy, it is of paramount importance to adhere to the same experimental conditions, i.e. data sets and performance metrics (when comparing CNN with other techniques), as well as architectures and model parameters (when comparing studies both employing CNN). From the related work studied, 15 out of the 23 papers (65%) performed direct, valid and correct comparisons among CNN and other commonly used techniques. Hence, it is suggested that some of the findings detailed earlier must be considered with caution.
Advantages/disadvantages of convolutional neural networks
Except from the improvements in precision observed in the classification/prediction problems at the surveyed works, there are some other important advantages of using CNN in image processing. Previously, traditional approaches for image classification tasks was based on hand-engineered features, whose performance and accuracy greatly affected the overall results. Feature engineering (FE) is a complex, time-consuming process which needs to be altered whenever the problem or the data set changes. Thus, FE constitutes an expensive effort that depends on experts’ knowledge and does not generalize well (Amara et al., Reference Amara, Bouaziz, Algergawy and Mitschang2017). On the other hand, CNN do not require FE, as they locate the important features automatically through the training process. Quite impressively, in the case of fruit counting, the model learned explicitly to count (Rahnemoonfar and Sheppard, Reference Rahnemoonfar and Sheppard2017). Convolutional neural networks seem to generalize well (Pan and Yang, Reference Pan and Yang2010) and they are quite robust even under challenging conditions such as illumination, complex background, size and orientation of the images, and different resolution (Amara et al., Reference Amara, Bouaziz, Algergawy and Mitschang2017).
Their main disadvantage is that CNN can sometimes take much longer to train. However, after training, their testing time efficiency is much faster than other methods such as SVM or KNN (Chen et al., Reference Chen, Lin, Zhao, Wang and Gu2014; Christiansen et al., Reference Christiansen, Nielsen, Steen, Jørgensen and Karstoft2016). Another important disadvantage (see earlier) is the need for large data sets (i.e. hundreds or thousands of images), and their proper annotation, which is sometimes a delicate procedure that must be performed by domain experts. The current authors’ personal experimentation with CNN (see earlier) reveals this problem of poor data labelling, which could create significant reduction in performance and precision achieved.
Other disadvantages include problems that might occur when using pre-trained models on similar and smaller data sets (i.e. a few hundreds of images or less), optimization issues because of the models’ complexity, as well as hardware restrictions.
Data set requirements
A considerable barrier in the use of CNN is the need for large data sets, which would serve as the input during the training procedure. In spite of data augmentation techniques, which could augment some data sets with label-preserving transformations, in reality at least some hundreds of images are required, depending on the complexity of the problem under study. In the domain of agriculture, there do not exist many publicly available data sets for researchers to work with, and in many cases, researchers need to develop their own sets of images manually. This could require many hours or days of work.
Researchers working with remote-sensing data have more options, because of the availability of images provided by satellites such as MERIS, MODIS, AVHRR, RapidEye, Sentinel, Landsat, etc. These data sets contain multi-temporal, multi-spectral and multi-source images that could be used in problems related to land and crop cover classification.
Future of deep learning in agriculture
The current study has shown that only 12 agriculture-related problems (see earlier) have been approximated by CNN. It would be interesting to see how CNN would behave in other agriculture-related problems, such as crop phenology, seed identification, soil and leaf nitrogen content, irrigation, plant water stress detection, water erosion assessment, pest detection and herbicide use, identification of contaminants, diseases or defects of food, crop hail damage and greenhouse monitoring. Intuitively, since many of the aforementioned research areas employ data analysis techniques with similar concepts and comparable performance to CNN (i.e. linear and logistic regression, SVM, KNN, K-means clustering, wavelet-based filtering, Fourier transform), then it would be worth examining the applicability of CNN to these problems too.
Other possible application areas could be the use of aerial imagery (i.e. by means of drones) to monitor the effectiveness of the seeding process, increase the quality of wine production by harvesting grapes at the right moment for best maturity levels, monitor animals and their movements to consider their overall welfare and identify possible diseases, and many other scenarios where computer vision is involved.
As noted before, all the papers considered in the current survey made use of basic CNN architectures, which constitute only a specific, simpler category of DL models. The study did not consider/include more advanced and complex models such as Recurrent Neural Networks (RNN; Mandic and Chambers, Reference Mandic and Chambers2001) or Long Short-Term Memory (LSTM) architectures (Gers et al., Reference Gers, Schmidhuber and Cummins2000). These architectures tend to exhibit dynamic temporal behaviour, being able to remember (i.e. RNN) but also to forget after some time or when needed (i.e. LSTM). An example application could be to estimate the growth of plants, trees or even animals based on previous consecutive observations, to predict their yield, assess their water needs or prevent diseases from occurring. These models could find applicability in environmental informatics too, for understanding climatic change, predicting weather conditions and phenomena, estimating the environmental impact of various physical or artificial processes, etc.
Personal experiences from a small study
To better understand the capabilities and effectiveness of CNN, a small experiment was performed, addressing a problem not touched upon by related work: that of identifying missing vegetation from a crop field, as shown in Fig. 2. As depicted in the figure, areas labelled as (1) are examples of sugar cane plants, while areas labelled (2) are examples of soil. Areas labelled as (3) constitute examples of missing vegetation, i.e. it is currently soil where it should have been sugar cane. Finally, areas labelled (4) are examples of irrelevant image segments.

Fig. 2. Identification of missing vegetation from a crop field. Areas labelled as (1) represent examples of sugar cane plants, while areas labelled as (2) constitute examples of soil. Areas labelled as (3) depict missing vegetation examples, i.e. it should have been sugarcane but it is soil. Finally, areas labelled as (4) are examples of ‘others’, being irrelevant image segments. Colour online.
A data set of aerial photos from a sugar cane plantation in Costa Rica, prepared by the company INDIGO Inteligencia Agrícola (https://www.indigoia.com), was used. Data were captured by a drone in May 2017 from a geographical area of 3 ha based on a single crop field. These data were split into 1500 80 × 80 images, and then experts working at INDIGO annotated (labelled) every image as ‘sugar cane’, ‘soil’ or ‘other’, where the latter could be anything else in the image such as fences, irrigation infrastructures, pipes, etc. The VGG architecture (Simonyan and Zisserman, Reference Simonyan and Zisserman2014) was used on the Keras/Theano platform. Data were split randomly into training and testing data sets, using a ratio of 85 : 15. To accelerate training, a pre-trained VGG model was used, based on the ImageNet data set (Deng et al., Reference Deng, Dong, Socher, Li, Li and Fei-Fei2009). The results are presented in a confusion matrix (Fig. 3). The VGG model achieved a CA of 79.2%, after being trained for 15 epochs at a time duration of 11 h using a desktop PC with an Intel Core i7 quad-processor@2 GHz and 6GB of RAM. Trying to investigate the sources of the relatively large error (in comparison to related work under study as listed in Appendix I), the following (main) labelling issues were observed (Fig. 4):
• Some images were mislabelled as ‘other’ while they actually represented ‘sugar cane’ snapshots (4% of the 20.8% total error).
• Some images mislabelled as ‘other’ while they represented ‘soil’ (8% of the error).
• Some images mislabelled as ‘soil’ while being ‘sugar cane’ (2% of the error).
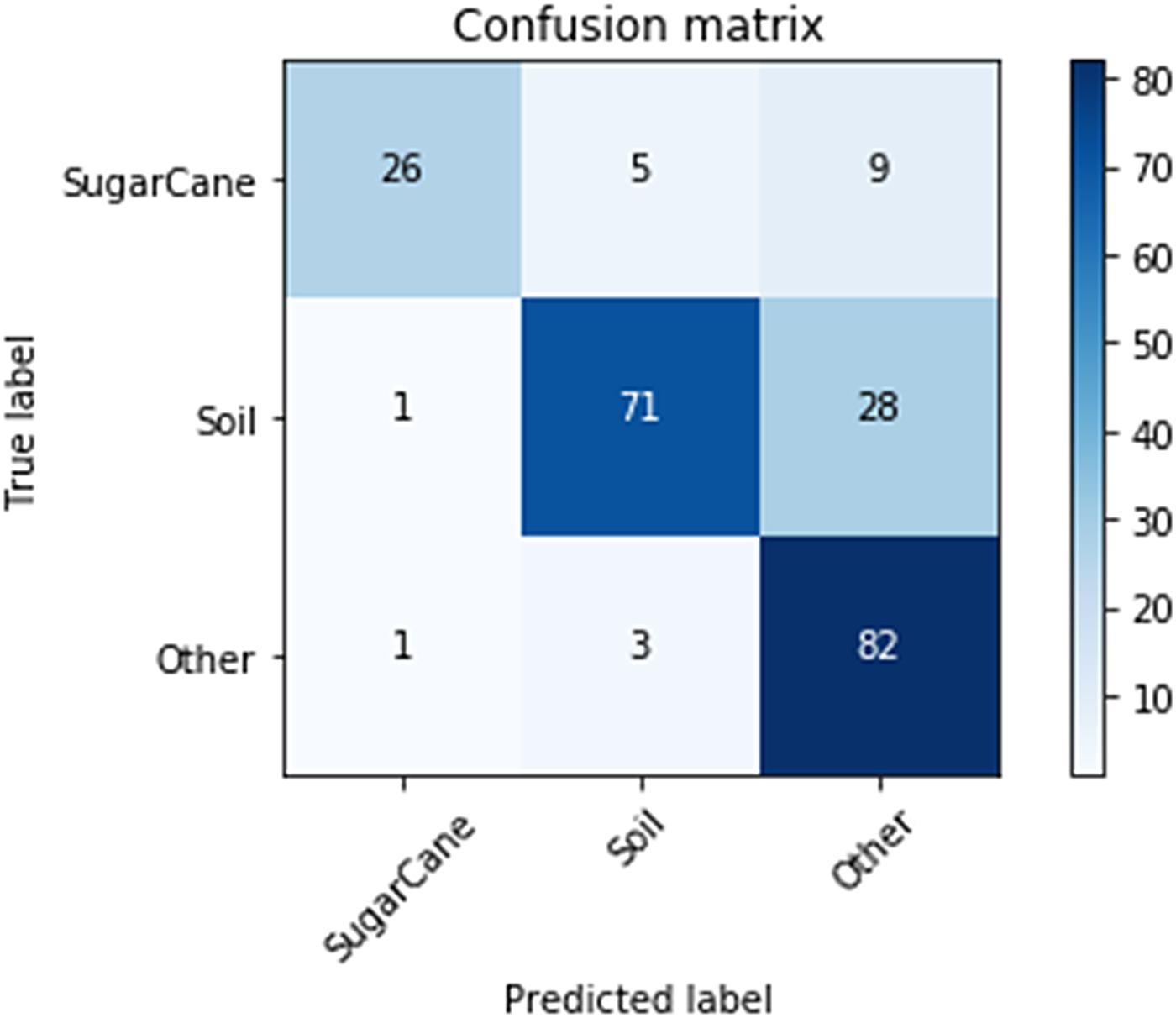
Fig. 3. Confusion matrix of the results of identifying missing vegetation from a crop field. Colour online.

Fig. 4. Incorrect labels of the vegetation data set. Examples of images mislabelled as ‘other’ while should have been labelled ‘soil’ (top). Examples of images mislabelled as ‘other’ while should have been labelled ‘sugar cane’ (middle). Examples of images mislabelled as ‘soil’ while they represented ‘sugar cane’ (bottom). Colour online.
Based on the above, the error would have been reduced from 20.8% to 6–8% by more careful labelling. This experiment emphasizes the importance of proper labelling of the training data set, otherwise CA can deteriorate significantly. Sometimes, as this experiment revealed, the annotation procedure is not trivial because there are images which could belong to multiple labels (Fig. 4). In particular, labelling of images as ‘soil’ or ‘sugar cane’ is sometimes very difficult, because it depends on the proportion of the image covered by plants, or more generally vegetation in the image. Increasing image size makes the problem even worse, as labelling ambiguity increases. Thus, the variation among classes of the training data set is also an important parameter that affects the model's learning efficiency.
Perhaps a solution for future work would be to automate labelling by means of the use of a vegetation index, together with orientation or organization of the plants in the image, which could indicate a pattern of plantation or the soil in between. Still, with these constraints, this experiment indicates that CNN can constitute a reliable technique for addressing this particular problem. The development of the model and its learning process do not require much time, as long as the data set is properly prepared and correctly labelled.
Conclusion
In the current paper, a survey of CNN-based research efforts applied in the agricultural domain was performed: it examined the particular area and problem they focus on, listed technical details of the models employed, described sources of data used and reported the overall precision/accuracy achieved. Convolutional neural networks were compared with other existing techniques, in terms of precision, according to various performance metrics employed by the authors. The findings indicate that CNN reached high precision in the large majority of the problems where they have been used, scoring higher precision than other popular image-processing techniques. Their main advantages are the ability to approximate highly complex problems effectively, and that they do not need FE beforehand. The current authors’ personal experiences after employing CNN to approximate a problem of identifying missing vegetation from a sugar cane plantation in Costa Rica revealed that the successful application of CNN is highly dependent on the size and quality of the data set used for training the model, in terms of variance among the classes and labelling accuracy.
For future work, it is planned to apply the general concepts and best practices of CNN, as described through this survey, to other areas of agriculture where this modern technique has not yet been adequately used. Some of these areas have been identified in the ‘Discussion’ section.
The aim is for the current survey to motivate researchers to experiment with CNN and DL in general, applying them to solve various agricultural problems involving classification or prediction, related not only to computer vision and image analysis, but more generally to data analysis. The overall benefits of CNN are encouraging for their further use towards smarter, more sustainable farming and more secure food production.
Acknowledgements
The authors would like to thank the reviewers for their valuable feedback, which helped to reorganize the structure of the survey more appropriately, as well as to improve its overall quality.
Financial support
This research has been supported by the P-SPHERE project, which has received funding from the European Union's Horizon 2020 research and innovation programme under the Marie Skodowska–Curie grant agreement No 665919. This research was also supported by the CERCA Programme/Generalitat de Catalunya.
Conflicts of interest
None.
Ethical standards
Not applicable.